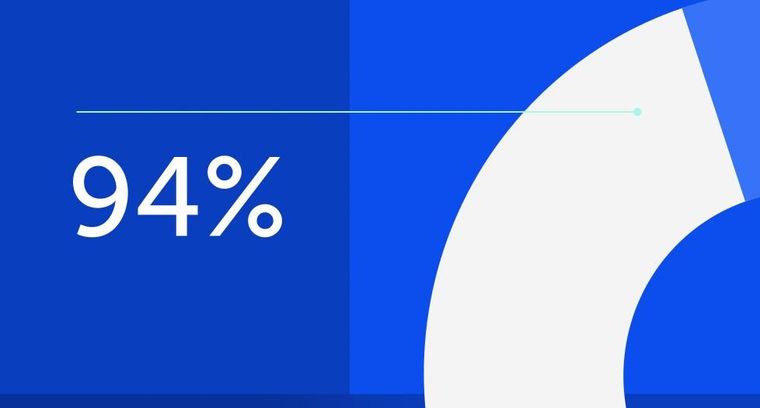
94% of researchers rate our articles as excellent or good
Learn more about the work of our research integrity team to safeguard the quality of each article we publish.
Find out more
ORIGINAL RESEARCH article
Front. Neurosci., 21 June 2011
Sec. Decision Neuroscience
volume 5 - 2011 | https://doi.org/10.3389/fnins.2011.00081
This article is part of the Research TopicNeurobiology of ChoiceView all 12 articles
Motivational theories of choice focus on the influence of goal values and strength of reinforcement to explain behavior. By contrast relatively little is known concerning how the cost of an action, such as effort expended, contributes to a decision to act. Effort-based decision making addresses how we make an action choice based on an integration of action and goal values. Here we review behavioral and neurobiological data regarding the representation of effort as action cost, and how this impacts on decision making. Although organisms expend effort to obtain a desired reward there is a striking sensitivity to the amount of effort required, such that the net preference for an action decreases as effort cost increases. We discuss the contribution of the neurotransmitter dopamine (DA) toward overcoming response costs and in enhancing an animal’s motivation toward effortful actions. We also consider the contribution of brain structures, including the basal ganglia and anterior cingulate cortex, in the internal generation of action involving a translation of reward expectation into effortful action.
“So she was considering in her own mind […], whether the pleasure of making a daisy-chain would be worth the trouble of getting up and picking the daisies…”
Alice in “Alice’s Adventures in Wonderland,” Carroll, (1865, p. 11)
Effort is commonly experienced as a burden, and yet we readily expend effort to reach a desired goal. Many classical and contemporary studies have assessed the effect of effort expenditure on response rates, by varying experimental parameters such as the weight of a lever press, the height of a barrier to scale, or the number of handle turns needed to generate a unit of reward (Lewis, 1964; Collier and Levitsky, 1968; Kanarek and Collier, 1973; Collier et al., 1975; Walton et al., 2006; Kool et al., 2010). There is general agreement that animals, including humans, are disposed to avoid effortful actions. It is paradoxical then that effort is not always treated as a nuisance, and there are instances where its expenditure enhances outcome value as observed in food palatability (Johnson and Gallagher, 2010), likability (Aronson, 1961), and indeed the propensity to choose a previously effortful option (Friedrich and Zentall, 2004). What is most surprising is the observation that effort often biases future choice toward effortful actions (Eisenberger et al., 1989).
Laboratory results show that if reward magnitude is held constant then high-effort tasks tend to be avoided (Kool et al., 2010). Yet, in daily life most organisms seem superficially indifferent to the varying costs of action and readily choose challenging tasks to achieve a desired goal (Duckworth et al., 2007). Such observations point to the presence of a mechanism that integrates effort costs with benefits in order to implement desired actions (see Floresco et al., 2008b for review on various cost–benefit analyses and Salamone et al., 2007 for an earlier review on dopamine and effort). This perspective has been addressed by optimal foraging theory. It is known that animals will strive to maximize gain whilst minimizing energy expenditure (Bautista et al., 2001; Stevens et al., 2005). Thus, ducks choose between walking or flying depending on optimal solution of net gain between energy requirements in walking or flying and the food gained (Bautista et al., 2001).
In what follows we discuss a literature that has endeavored to understand the neural mechanisms of effort and reward integration, including the involvement of dopamine (DA) in effort-based behavior. This literature points to the basal ganglia, particularly dorsal and ventral striatum, and anterior cingulate cortex (ACC) as the principal substrates in both representing and integrating effort and action implementation. Finally, we suggest that pathologies in effort expenditure, the paradigmatic instance being the clinical phenomenon of apathy, can be characterized behaviorally as impairment in representing action–outcome association and neurally as a disruption of core cortico-subcortical circuitry.
Over the past three decades, theories concerning the role of midbrain DA on behavior have changed dramatically. The hedonic hypothesis of DA (Wise, 1980) is now challenged by empirical evidence revealing that global DA depletion (including within the accumbens, a major recipient for DA) does not impair hedonic (‘liking’) responses to primary rewards such as orofacial reactions, the preference for sucrose over water, or discrimination among reinforcement (Berridge et al., 1989; Cousins and Salamone, 1994; Cannon and Palmiter, 2003). On the other hand the same lesions profoundly impair performance of instrumental tasks necessary to obtain rewards that are liked (Berridge and Robinson, 1998). These observations have led to a formulation that the contribution of DA includes an effect on motivated behaviors toward desired goals, a concept referred to as “wanting” (Berridge and Robinson, 1998). “Wanting” (Table 1) can be expressed in simple instrumental responses, such as button or lever presses or in a more expanded form of behaviors which require an agent to overcome action costs. As demonstrated unequivocally by Salamone and colleagues(Salamone and Correa, 2002; Salamone et al., 2003; Salamone, 2007), accumbens DA depletion disrupts instrumental responding if the responses require an energetic cost such as climbing a barrier (Salamone et al., 1994), but leaves reward preference intact when effort is minimal. This has led to an hypothesis that DA plays a role in overcoming “costs” (Salamone and Correa, 2002; Phillips et al., 2007).
There are several alternative views to dopamine which we summarize in Figure 1. Aside from a role in the expression of motivated behavior, DA is also involved in its acquisition through learning. An influential view on how dopamine influences behavior comes from reinforcement learning (Sutton and Barto, 1998). Reinforcement learning offers ways to formalize the process of reward maximization through learned choices and has a close resonance with the neuroscience of decision making (Montague et al., 1996; Montague and Berns, 2002; Niv et al., 2005; Daw and Doya, 2006). In particular, phasic responses of macaque and rodent midbrain dopaminergic neurons to rewards, and reward-associated stimuli, are akin to a reward prediction error signal within reinforcement learning algorithms, responding to unexpected rewards and stimuli that predict rewards but not to fully predicted rewards (Schultz et al., 1997; Bayer and Glimcher, 2005; Morris et al., 2006; Roesch et al., 2007). Moreover, functional magnetic resonance imaging (fMRI) studies report that the BOLD signal in the striatum, a major target of the dopaminergic system, correlates with the prediction error signals derived from fitting subject’s behavior to a reinforcement learning model (Mcclure et al., 2003; O’Doherty et al., 2003, 2004). In support of such a role for dopamine in reinforcement learning processes, stimulation of the substantia nigra (using intracranial self-stimulation paradigm) has been shown to induce a potentiation within corticostriatal synapses at the site where nigral output cells terminate, with these effects in turn being blocked by systemic administration of a DA D1/D5 antagonist (Reynolds et al., 2001). Importantly, the magnitude of potentiation is negatively correlated with the time taken by an animal to learn the self-stimulation paradigm.
Figure 1. The figure illustrates a range of views regarding the role of dopamine in facilitating motivated behavior. Clockwise from top left corner: a wealth of evidence shows that DA acts to invigorate an agent’s effortful action, integrating ideas about overcoming effort costs, agents’ choice for high-effort options as well as modeling work on vigor. Another influential view pertains to DA acting as a signal for a prediction in reward as exemplified by its role in reinforcement learning. An alternative view interprets this signal as a saliency signal which allows agents to implement associative learning. Finally, dopamine may facilitate flexible switching and re-engagement in relation to reward-driven behavior. The summarized perspectives are not mutually exclusive, nor do they represent the entire literature on dopamine, but are useful in understanding what determines motivated behavior.
Dopamine is also proposed to signal stimulus salience, as opposed to reward prediction error (Redgrave et al., 1999). Redgrave et al. (1999) have discussed the stereotypical latency and duration of phasic bursts of nigral dopaminergic neurons, as well as the connectivity between nigral dopaminergic neurons and sensory subcortical structures such as the superior colliculus. They argue that activity of dopamine neurons can be interpreted as reporting biological salient events, either due to novelty or unpredictability. From this perspective, salient events generate short-latency bursts of dopaminergic activity that reinforce actions occurring immediately preceding the unpredictable event. This signal allows an agent to learn that an action caused the salient event (see Redgrave and Gurney, 2006 for an elegant discussion on signal transmission in tecto-nigral and cortico-subcortical pathways for learning of action–outcome associations). According to this view, unpredictable rewarding events are just one among many exemplars of a salient event.
Finally, Nicola (2010)recently suggested that DA is required to flexibly initiate goal-directed instrumental responses. This view is based on observations that the effects of DA depletion in the rat nucleus accumbens (NAc) are dependent on inter-trial interval, such that when this is short instrumental responses are not affected but disruption increases as a function of increasing time between responses. Detailed behavioral analysis shows these effects of time are explained by the fact that as the duration between responses increases animals tend to engage with behaviors different from the required instrumental response, with depleted animals unable to flexibly reinitiate execution of the instrumental responses. On the other hand, depleted rats can perform complex sequences of behavior in situations where these are not interrupted. Such findings suggest that rather than impairing lever presses, dopamine depletion disrupts an animal’s ability to flexibly re-engage with a task after engaging in a task-irrelevant behavior.
The most compelling attempt to link the known role of DA in reward learning to effort is that of Niv et al. (2007) who have developed a model that specifies the vigor (defined as the inverse latency) of action. This model realizes a trade-off between two costs: one stemming from the harder work assumed necessary to emit faster actions and the other from the opportunity cost inherent in acting more slowly. The latter arises out of the ensuing delay to the next, and indeed to all subsequent, rewards. Niv et al. (2007) suggested that agents should choose latencies (and actions) to maximize the rate of accumulated reward per unit time, and showed that the resulting optimal latencies would be inversely proportional to the average reward rate. Based on a review of experimental evidence, Niv et al. (2007) proposed that tonic levels of DA report the average rate of reward, thus tying together prediction error (Montague et al., 1996; Schultz et al., 1997; Mcclure et al., 2003), incentive salience (Berridge and Robinson, 1998), and invigoration (Salamone and Correa, 2002) theories of DA. As defined by Niv et al. (2007), vigor can be thought of as a specific manifestation of effort expenditure in the time domain. Future work might usefully extend this temporal computational concept of vigor into other aspects of physical effort.
Considerable evidence points to midbrain DA depletion discouraging animals from choosing effortful actions (Cousins and Salamone, 1994; Aberman and Salamone, 1999; Denk et al., 2005; Phillips et al., 2007). A series of experiments in rats has pointed to the crucial role of cortico-subcortical networks for cost–benefit decision making as highlighted in depletion effects (Cousins and Salamone, 1994; Aberman and Salamone, 1999; Salamone et al., 2007). In these experiments, rats are trained on a T-maze that requires choosing between two actions; one yields high reward (four pellets of food) but requires higher effort (climb a 30-cm barrier or higher lever press fixed-ratio schedule), the other yields low reward (two pellets of food) but requires less effort. DA depletion in the NAc changes a rat’s preference away from the high-effort/high reward option, but does not impact on reward preference when it is readily available, nor does it alter response selection based on reward alone (Cousins and Salamone, 1994; Salamone et al., 1994). This finding has been replicated in other laboratories with a variety of depletion methods (Denk et al., 2005; Floresco et al., 2008a), where some studies point to a stronger effect from depletion in the core as opposed to shell of the NAc (Ghods-sharifi and Floresco, 2010; Nicola, 2010).
The impact of DA elevation on effort is much less conclusive. Enhancing DA function is commonly realized through injection of amphetamine, an indirect DA agonist that increases synaptic DA levels (but also that of other neuromodulators). Floresco et al. (2008a) revealed a dose-dependent effect of amphetamine such that low-doses of amphetamine increased effortful choice, but high dose decreased it. This dose-dependent effect is difficult to interpret. First of all, it is unclear what the precise effect of a high dose of amphetamine is on DA concentration level since amphetamine also results in increased extracellular serotonin and noradrenaline (Salomon et al., 2006). Moreover, it is unclear whether a low dose of amphetamine acts by increasing the value of the reward, decreasing the cost of an action, modifying the integration of both, or by affecting other components of behavioral control such as impulsivity (see Pine et al., 2010 in relation to the latter). Nevertheless, the data suggest that increasing DA levels per se does not invariably enhance preference for a high reward/high-effort option, ruling out a simple monotonic relationship between DA and effort.
Another study showed an interactive effect of haloperidol, a DA receptor blocker, and amphetamine. While an injection of haloperidol 48 h before treatment, followed by saline 10 min before test, significantly reduced preference for high reward/high-effort arm, giving the same haloperidol injection followed by amphetamine 10 min before testing blunted the effect of haloperidol, and completely recovered preference for high reward/high-effort arm (Bardgett et al., 2009). Evidence therefore points to amphetamine’s ability to overcome the effects of DA blockade induced by haloperidol. However, as indicated amphetamine also increases the levels of serotonin and noradrenaline as well as DA levels, making it difficult to completely outrule a possibility that the effect might relate to elevations of other amines aside from DA. We also know that amphetamine increases locomotor activity (Salomon et al., 2006) and it is impossible to dismiss the possibility that a recovered preference for the high-effort arm found might be due to enhanced locomotion.
Recent advances in neurochemical assay techniques, particularly in vivo fast scan cyclic voltammetry, allow detection of DA transients with a temporal resolution of milliseconds in awake behaving animals (Robinson et al., 2003; Roitman et al., 2004). Gan et al. (2010) performed in vivo voltammetry while rats selected between two options in a task where there was an independent manipulation of the amount of reward and effort. These authors found that rats had the expected preference for higher magnitude of reward when costs were held constant and higher preference for options which require less effort when reward magnitude was constant. This study also included a separate set of trials which offered rats either option, while measuring the amount of DA released in the core of the NAc elicited by cues predicting reward and effort. By having this set of non-choice trials the authors ensured that the dopaminergic response was not confounded by the presentation of the second option. Whereas DA release reliably reflected the magnitude of the reward available in these trials, the amount of effort required to obtain the goal was not coded in the amount of DA released in the core of the NAc. This lack of evidence for an integration between costs and benefits in the dopaminergic signal was surprising given the extent of prior evidence (discussed above) pointing to a link between DA and the expenditure of effort in overcoming costs.
Overall, there is evidence that DA is required to overcome costs when high levels of effort are necessary to obtain a desired goal. However, the precise mechanism by which DA supports a cost-overcoming function, and how effort is integrated into a dopaminergic modulation of the striatum and prefrontal cortex, is much less clear. In addition, dopamine depleted animals can engage in high-effort responding given a limited, inflexible set of possible responses but exhibit difficulties and are slower in re-engaging with simple one-lever presses where multiple responses are allowed (Nicola, 2010). Whilst dopamine may be key to the computation and execution of highly effortful tasks, its role in strategic flexibility (Nicola, 2010) suggests it exerts a more subtle contribution to the complex relationship between task demands and the integration of task-relevant and task-irrelevant behavior.
We next consider the likely contribution of basal ganglia (BG) and ACC, and the formation of action–outcome association necessary for motivated behavior.
The basal ganglia are a set of subcortical nuclei comprising dorsal (putamen and caudate nucleus) and ventral aspects (often synonymous with NAc), the internal (GPi) and external (GPe) segments of globus pallidus, substantia nigra pars compacta (SNc), and reticulata (SNr) as well as the subthalamic nucleus (STN). The BG receives afferents from almost all cortical areas, especially the frontal lobe. Information processed within the BG network is sent via output nuclei (the internal segment of the globus pallidus and substantia nigra pars reticulata) to the thalamus, which eventually feeds back to frontal cortex (Alexander and Crutcher, 1990; Bolam et al., 2002). This basic circuitry is reproduced in different parallel and integrative corticostriatal loops, with their origin in different frontal domains, and is held to play a critical role in cognitive functions that span motor generation to more cognitive aspects of causal learning, executive function and working memory (Frank et al., 2001; Frank, 2005; Haber and Knutson, 2010; Vitay and Hamker, 2010).
Neurons in the striatum project either to output nuclei of the BG (GPi and SNr) or to an intermediate relay involving GPe neurons which ultimately project to BG output nuclei. These two populations provide the origin of BG direct and indirect pathways which funnel information, conveyed in parallel to striatum by cortical afferents, to BG output nuclei (Alexander and Crutcher, 1990; Frank et al., 2004; Frank, 2005; Frank and Fossella, 2011). Under basal conditions, the output nuclei of the BG have a high level of firing and maintain thalamic inhibition that serve to dampen activity in corticostriatal loops (Frank, 2005). The distinct connectivity of direct and indirect pathways (see Figure 2) results in opposite effects: the direct pathway promotes inhibition of BG output nuclei and release of inhibition in thalamic activity whereas the indirect pathway promotes excitation of BG output structure and drives thalamic inhibition.
Figure 2. A schematic model of direct and indirect pathways of BG (adapted from Frank et al., 2004). The principal input of BG is the striatum, receiving excitatory inputs from most cortical areas. The output nuclei of BG are GPi/SNr, which direct processed information to the thalamus to eventually feed back an excitatory projection to the cortex. Within this circuitry, there are two pathways: a direct pathway expresses D1 receptors and indirect pathway expresses D2 receptors. D1 striatal neurons inhibit GPi/SNr cells forming the direct pathway. D2 striatal cells inhibit an intermediate relay, the GPe which ultimately provides inhibition to GPi/SNr. Under basal conditions, GPi/SNr cells fire at high level and maintain inhibition of the thalamus which in turn dampens corticostriatal loops activity. The different direct/indirect connectivity results in opposite effects: inhibitory effect on GPi/SNr and release of inhibition in thalamic activity by the direct pathway and excitatory effect on GPi/SNr and inhibitory effect on thalamus by the indirect pathway.
Neurons within the direct pathway express D1 dopamine receptors, promoting cell activity and long-term potentiation (LTP), whereas neurons of the indirect pathway mainly express D2 dopamine receptors which promote cell inhibition (when activated) and long-term depression (LTD). This scheme means that an increase in striatal DA tends to promote activity in the direct pathway and inhibition of the indirect pathway, resulting in net disinhibition of the thalamo-cortical connections and the generation of behavioral output. On the other hand a decrease in striatal DA promotes excitation of the indirect pathway resulting in dampening of activity in thalamo-cortical loops and behavioral inhibition. A segregation between striatal neurons that form the direct and indirect pathway means increases in dopamine potentiate the direct pathway while inhibiting the indirect pathway, thus facilitating thalamic output and corticostriatal flow. On the other hand a decrease in dopamine promotes inhibition of the direct pathway and an excitation of the indirect pathway, dampening thalamic output and shutting down corticostriatal information flow. Finally, to complete a picture of the BG circuitry we need to include reference to an hyperdirect pathway from inferior frontal cortex to the STN, a circuit that sends excitatory projections to output nuclei of the BG (Frank, 2006). The contribution of this pathway to behavioral control has been discussed extensively (Aron and Poldrack, 2006; Frank, 2006) and is beyond the scope of the present review.
The functional organization of BG along the direct and indirect pathways, as described above, applies to the full extent of the striatum, forming an integral re-iterated processing matrix which performs common operations across different subdivisions (Wickens et al., 2007). Although there are suggestions of a dorsal–ventral segregation, the consensus favors a dorsolateral–ventromedial gradient (Voorn et al., 2004) with no sharp anatomical distinction between dorsal–ventral areas. Indeed, based on the cytology of spiny projection neurons, dopaminergic inputs, and dopamine-modulated plasticity and inhibition, dorsal and ventral striatum are strikingly similar (Wickens et al., 2007). However, there is evidence for a functional segregation such that dorsolateral striatum, receiving sensorimotor afferents, supports habitual, stimulus–reward associations. This contrasts with ventromedial striatum, receiving afferents from orbito and medial prefrontal cortex, hippocampus, and amygdala, which supports formation of stimulus–action–reward associations (Voorn et al., 2004; Haber and Knutson, 2010).
A functional gradient in dopamine signaling is also described in BG (Wickens et al., 2007). DA release is determined by density of DA innervation (densities reduce the distance between release and receptor sites), such that higher innervation densities are necessary for rapid DA signaling. DA clearance is regulated by density of DA transporters (DAT), hence affecting distance and time course of volume transmission. Wickens et al. (2007) has documented greater DA innervation and higher DAT densities in dorsolateral striatum with these densities decreasing along a ventromedial gradient (also Haber and Knutson, 2010). High densities of release sites and DAT result in fast clearance in dorsolateral striatum, which may be related to encoding of discrete events involving reinforced responding, or even automatized and habitualized behaviors. Ventromedially, lower densities of DA innervation and DAT result in slow clearance in NAc core, and even slower clearance in NAc shell, which may be related to slower time course of action–outcome evaluation (Wickens et al., 2007; Humphries and Prescott, 2010).
Moreover, it is noteworthy that within the ventromedial subdivisions of the striatum, the NAc has interesting particularities. The NAc is subdivided, on the basis of anatomical and histochemical features, into the core and the shell, with the latter more medial and ventral in location than the former (Voorn et al., 2004; Ikemoto, 2007; Humphries and Prescott, 2010). This core/shell distinction is particularly important when considering the role of BG in motivated behavior.
The NAc core is similar to dorsal striatum (Humphries and Prescott, 2010, but see Nicola, 2007 on role of dorsal–ventral striatum in temporal predictability). Functionally, NAc core seems critical in the translation of raw, unconditioned stimulus value, into a conditioned response. Thus, NAc core plays an important role in conditioned behavior (Ikemoto, 2007), such as autoshaping in classical conditioning paradigms and conditioned reinforced responses in instrumental learning paradigms. On the other hand, the NAc shell, the most ventromedial aspect of striatum, has unique features compared to the rest of striatum. First, it is involved in unconditioned responding in the appetitive and aversive domains, spanning feeding (Kelley et al., 2005), and maternal behavior (Li and Fleming, 2003) to defensive treading (Reynolds and Berridge, 2002). Moreover, the NAc shell is involved in invigorating effects of dopamine on conditioned behaviors controlled by the NAc core (Parkinson et al., 1999). Second, the shell is the only striatal subdivision projecting to lateral hypothalamus (Pennartz et al., 1994, and reviewed by Humphries and Prescott, 2010), a key structure in an “action–arousal” network. Note lateral hypothalamus also exerts an influence over autonomic function and contains orexin-producing cells which influence arousal and energy balance control (see Ikemoto, 2007 for a comprehensive review). Third, whereas amygdala has extensive projections to both the core and shell (Humphries and Prescott, 2010), the NAc shell is the only recipient of hippocampal afferents within the striatal complex (Wickens et al., 2007; Haber and Knutson, 2010). This restricted projection from hippocampus has generated extensive discussion concerning the unique role of ventral BG in spatial navigation, fear-modulated free-feeding, and acquisition of stimulus value through stimulus–outcome pairings (Humphries and Prescott, 2010). These lines of evidence point to the shell as critical in forming linkages between an object/event in the environment and the agent’s natural response toward it.
An alternative interpretation of the anatomical and physiological organization of the BG is a selection and control model (Gurney et al., 2001). In this model inputs for selection and control are received separately by striatal D1 receptors and D2-like receptors, respectively. D1 transmission is then projected as inhibition to GPi/SNr which acts as an action selection output, whereas D2 transmission inhibits GPe which acts as an output layer for a control mechanism. The control output layer, in turn modulates action selection: GPe inhibits activity GPi/SNr output nuclei. Akin to inhibitory mechanisms described in the direct/indirect BG model, this selection/control BG model also describes inhibitory relationships between nuclei in BG. It is not clear what the thalamic inhibitory/excitatory impacts are on movement. Nevertheless this model highlights an important role for BG in action selection and control. More recently, Nicola (2007) has discussed the potential role of NAc in such a model, particularly in disinhibiting motor efferents for one action and inhibiting motor efferents for another, thereby allowing action selection.
To facilitate execution of motivated behavior, one needs to internally represent action costs and benefits. Using fMRI, Croxson et al. (2009) investigated where in the human brain effort and reward are represented. Participants saw a discriminative stimulus signaling an action with a particular cost and benefit and then completed a series of finger movements using a computer mouse, to gain secondary reinforcers. The cost, in terms of effort and time, increased as more finger movements were completed, whilst the benefit increased as the secondary reinforcer was larger. When anticipating these actions, striatum activity correlated with both anticipated costs and anticipated reward of effortful actions.
More recent fMRI studies have replicated an involvement of striatum in effort-related processes, reporting higher dorsolateral striatal activity for choosing low compared to high-effort options in a physical effort task (Kurniawan et al., 2010) and higher ventral striatal activity in a low cognitive demand block compared to a high cognitive demand block in a mental effort task (Botvinick et al., 2009). Whilst, it is still unclear whether physical and cognitive mechanisms of effortful actions reflect similar psychological and neural processes, together these studies provide support for the importance of striatum in effort-related processes. In the following section, we assess the type of association formed when an organism performs a motivated goal-directed behavior.
Linking a chosen action to its outcome is central for optimal goal-directed behavior. When a monkey travels a distance to forage for food, not only does it need to link contextual cues to food consumption, for example associating a tree full of ripe fruits with eating fruits, it also needs to associate the action (climbing a tree) with the consequences of the action, namely the energetic cost of climbing. Neurons in primate dorsal striatum, can be categorized into those that encode the action made by the monkey (direction of saccade made) and neurons sensitive to the outcome of the monkey’s choice (reward/unrewarded; Lau and Glimcher, 2007). However, these neurons do not appear to support the kind of action–outcome association required for goal-directed behavior.
Using reinforcement learning models, similar to those used to characterize activity in DA neurons, Samejima et al. (2005) reported neurons in the striatum whose activity correlated with the value of an action. These action value neurons are important because they track the value of say, a left handle turn in a probabilistic two-choice task, independent of whether the monkey ultimately selects the action, and thus provide input information for action selection. Furthermore, in a subsequent study, Lau and Glimcher (2008) found action value neurons, including neurons which traced the value of the chosen action, in the striatum. These chosen value neurons show enhanced activity when the tracked action has a higher value and, on this basis, was subsequently chosen. Using similar reinforcement learning models, human fMRI studies also report that BOLD signal in the dorsal striatum correlate with the relative advantage of taking one action over an alternative (O’Doherty et al., 2004).
These action and chosen value representations in the striatum are precisely the kind of association between action and outcome required for goal-directed behavior. However, the unanswered question is where does the information needed for this computation come from? One possibility is ACC, a region suggested to represent this action–outcome association (Rushworth et al., 2007). For example, Hadland et al. (2003) trained macaque monkeys to pull a joystick upward after receiving a type of food, say a peanut, in order to obtain a second peanut and to turn a joystick to the side after obtaining a different food type, say a raisin, to receive a second raisin. They found that while control monkeys could select an action based on this reward–response association, monkeys with a lesion to ACC were impaired in selecting the correct response. Interestingly, the impairment was not due to an inability to make an association between visual cues and reward as tested in a second visual discrimination task, but instead was specific to an inability to utilize reward–action association to make the correct response. In a different experiment, monkeys with ACC lesion were impaired in selecting a set of response when the correct responses were determined by an integration across past contingencies between action and reward (Kennerley et al., 2006). In addition, using fMRI, human ACC was found to be most active when participants had to simultaneously internally generate a sequence of actions whilst monitoring the outcome of their actions (Walton et al., 2004).
Lesions studies with rodents using the T-maze consistently show impairments in effort-based decision making following removal of ACC. As with dopamine depletion experiments, these lesions result in a shift of preference away from an option with a larger food reward that requires scaling a high barrier, thus requiring more effort. This reduced preference for larger/effortful arm was not due to lethargy or immobility as it is immediately restored when both arms have equal effort costs (Denk et al., 2005; Floresco and Ghods-Sharifi, 2007; Walton et al., 2009, but see Floresco et al., 2008b for a discussion the extent to which ACC plays a role in effort-based tasks).
Human ACC lesions provide a more subtle interpretation for the role of ACC in effort processing. Naccache et al. (2005) tested a patient with a large lesion to left mesial frontal region including the left ACC using a, cognitively demanding, Stroop task (Stroop, 1935). This patient could not verbally recognize nor express discriminatory skin conductance responses in difficult trials where greater mental effort was required, but could perform as well as healthy controls. This case study suggests dissociability of objective cognitive performance from a physiological response and from the subjective appraisal of mental effortfulness (Naccache et al., 2005, but see McGuire and Botvinick, 2010 for the involvement of lateral prefrontal cortex, instead of ACC, in a closer inspection of subjective experience of mental effort through intentional and behavioral avoidance from mentally challenging tasks).
The ACC is implicated in a host of cognitive processes, ranging from cognitive control to suppression of prepotent responses such as in Stroop or go–nogo tasks, tasks that induce negative emotions, and tasks that predict delivery of painful stimuli (see a review by Shackman et al., 2011). Shackman et al. (2011) discussed a challenge in advancing knowledge of its functional organization being the complexity of its anatomical organization and variability across individuals. For example, a tertiary sulcus in dorsal ACC, the paracingulate sulcus, is present in one-third of the population, and its presence causes location change of architectonic Brodmann area 32′, and a volumetric reduction of Brodmann areas 24a′ and 24b′. Consequently, spatially normalized cingulate premotor regions differ across subjects, and an unmodeled cingulate sulcal variability may inflate the spread of activation clusters found across studies, rendering complex a clear functional dissociation within ACC.
Bush et al. (2000) proposed the rostro-ventral cingulate could be functionally segregated into cognitive and affective components located to dorsal and ventral ACC, respectively. This segregation seems too broad. Shackman et al. (2011) using a sample of almost 200 neuroimaging experiments that included negative affect, pain, and cognitive control reported strongly overlapping activation clusters in dorsal ACC, or what they termed as middle cingulate cortex (MCC), challenging a strict segregationist view of ACC (see Figure 3). These authors also pointed to evidence that the dorsal ACC might be involved in affective control, including autonomic regulation (Critchley et al., 2003) and pain processing, suggesting these findings may reflect an agent’s need for behavioral control when habitual responses are not sufficient under uncertain action–outcome contingencies.
Figure 3. Views on the psychological function of ACC. Left: ACC function has been suggested as anatomically segregated into a dorsal cognitive division and a ventral affective division (Bush et al., 2000). Right: more than a decade later, a meta-analysis on almost 200 fMRI experiments suggested a strong overlap in clusters of activation in studies of cognitive control, negative affect, and pain (Shackman et al., 2011). Figures adapted from Shackman et al. (2011).
Anatomically, the ACC projects to striatum, particularly the caudate nucleus and portions of ventral striatum (Haber and Knutson, 2010). Moreover, ACC has bilateral connections to motor and prefrontal cortex fulfilling a role as a hub where action and outcome associations might be represented. In human and non-human primates, the ventral cingulate have strong interconnections with ventral striatum including the NAc, whilst the dorsal cingulate connects more strongly to dorsal striatum including putamen and caudate (Kunishio and Haber, 1994; Beckman et al., 2009), potentially facilitating transmission of reward-related information. Furthermore, dorsal ACC is interconnected with premotor cortex and a more posterior part constitutes the cingulate motor area (Beckman et al., 2009) implicated in action selection (Picard and Strick, 2001). Shima and Tanji (1998) reported that cingulate motor areas in monkeys respond to selection of voluntary movement based on reward, supporting a role in linking internally generated action to reward. Indeed, a working hypothesis is that ACC could support adaptive control, integrating aversive, biologically relevant information in order to bias motor regions toward a contextually appropriate action (Shackman et al., 2011).
This wide-ranging anatomical connectivity between BG, ACC, and other cortical regions provide a neuroanatomical foundation for establishing action and outcome representations, of a type needed for motivated behavior. Normal function of this circuitry can be inferred to facilitate willingness to execute effortful actions. On the other hand, disruption of this circuitry, as in people with apathy, would discourage execution of such actions. This account has a resemblance to phenomena in a case study of a patient with a lesion to mesial prefrontal cortex (which included ACC) that led to profound apathy (Eslinger and Damasio, 1985). This patient was severely impaired in execution of real-life events such as holding a job, although various measures of logical reasoning, general knowledge, planning, and social and moral judgments proved intact. The authors discussed how the lesion did not impact on pure action execution, but on the analysis and integration of the costs and benefits pertaining to real-life situations.
Several distinct types of brain insult are associated with apathy in humans. For example, bilateral ACC lesions can present with akinetic mutism, a wakeful state characterized by prominent apathy, indifference to painful stimulation, lack of motor and psychological initiative (Tekin and Cummings, 2002). Apathy is also often present in patients with subcortical brain lesions (involving BG), but is more commonly found in those with prefrontal, mainly ACC, lesions (van Reekum et al., 2005). In this section we draw upon findings with apathy to understand cost benefit integration and implementation of effortful choices.
Effort is a salient variable in individuals with apathy who lack the ability to initiate simple day-to-day activities (van Reekum et al., 2005; Levy and Dubois, 2006). This lack of internally generated actions may stem from impaired incentive motivation: the ability to convert basic valuation of reward into action execution (Schmidt et al., 2008). Patients with auto-activation deficit (AAD), the most severe form of apathy, are characterized by lack of self-initiated action (van Reekum et al., 2005) or a quantitative reduction in self-generated voluntary behaviors (Levy and Dubois, 2006). Thus, the key feature in AAD is an inability to internally generate goal-based actions, a deficit that may variously reflect an ability to (1) encode the consequence of an action as pleasurable or as having hedonic value (e.g., to attain reward, “liking”); (2) execute the action; and (3) represent the association between action and reward. We now discuss a proposal that the behavioral and neural mechanisms underlying AAD are mostly intimately linked to the third sub-process.
Auto-activation deficit is not associated with impaired “liking” as patients with AAD have a normal skin conductance response to receipt of rewards and verbally distinguish between different magnitudes of monetary reward (Schmidt et al., 2008). In addition, the most prominent damage in AAD pertains to BG and the dopaminergic system. Secondly, AAD is probably not linked to specific impairments of action execution. Schmidt et al. (2008) tested patients with bilateral BG lesions with the history of AAD and found that, compared to normal and Parkinson’s disease control groups, patients with AAD are worse when generating voluntary vigorous actions based on contingent reward, but are equally able to generate the same motor response if based on external instructions. This provides evidence against AAD being explicable in terms of an impairment in pure motor action execution.
We suggest that AAD reflects an impairment in linking reward anticipation to action. Damage to BG in AAD most commonly involves a focal bilateral insult to the internal portion of pallidum (Levy and Dubois, 2006). Pessiglione et al. (2007) investigated the role of ventral pallidum in incentive motivation employing a task where individuals voluntarily squeezed a handgrip device in response to different reward magnitudes. Notably, the amount of voluntary force during squeezing was proportional to reward magnitude, suggesting that participants were able to identify a reward context where it was advantageous to produce more physical effort. Furthermore, ventral pallidal activity correlated with outcome context, providing a neural basis for enhanced effort as a response to increased payoff. Similarly, damage to BG in AAD may have caused a failure to recognize an advantageous context to make an adaptive action (Walton et al., 2004; Levy and Dubois, 2006). These data suggest that bilateral BG damage, at least in AAD, produces a syndrome that arises out of a deficit in translating reward cues into appropriate action selection and execution.
In light of Schmidt et al.’s (2008) findings that AAD patients were mostly impaired in the execution of actions, when an internal link between a reward and action is required, it is noteworthy that AAD may cause impairments beyond simple abstract action–reward association. In other words, AAD may cause impairments in the actual execution of reward-based actions. This highlights the importance of BG in energizing individuals to persevere with acting, a deficit commonly found in patients with Parkinson’s disease (which is largely associated with a dysfunction in BG). Schneider (2007) tested Parkinson’s disease patients in solving a difficult cognitive task, and found that the patients were making significantly fewer attempts to solve the task than normal controls, pointing to a deficit in mental persistence in such patients. It may well be that persistence is linked to a higher tendency to generate internal motivation or arousal which then energizes individuals to persevere (Gusnard et al., 2003), or perhaps lessens a tendency to distraction (see Nicola, 2010).
Taken together, apathy, as a manifestation of impaired motivation to overcome the cost of an action, is associated with damage to a cortico-subcortical network (either lesions in the ACC or BG) that generates internal association between action and its consequences. This highlights a key involvement of the ACC and BG in the anticipation and execution of effortful actions.
We provide evidence for an intimate interplay between ACC, BG, and dopaminergic pathways in enabling animals, including humans, to choose and execute effortful action. We suggest that effort may act as a discounting factor for action value, and that integrative mechanisms between cost and benefit facilitate a willingness to incur costs. Our review of reinforcement learning, empirical findings on the relationship between dopaminergic coding and cost–benefit parameters of an action, and the organization of BG and ACC point to these latter structures as critical in linking a stimulus to an action and the consequences of that action. Notably, patients with apathy often manifest a pathology that disrupts this ACC–BG network. This fractures a link between action and outcomes resulting in lack of drive to execute potentially valuable actions.
Our review highlights the psychological and neural mechanisms through which an organism is willing and capable of executing an effortful act to attain a goal. The core process appears to involve coding of specific action requirements, an analysis and integration of costs and benefits, and a decision to expend effort and to implement an action. We do not dissect a potentially important distinction between cognitive and physical types of effort (Kool et al., 2010; Kurniawan et al., 2010). Future research might usefully endeavor to examine how one makes a trade-off between both effort types and examine how we determine when investing in one type of effort (mental) is more appropriate than investing in the other (physical).
The authors declare that the research was conducted in the absence of any commercial or financial relationships that could be construed as a potential conflict of interest.
This work was supported by a Wellcome Trust Programme Grant to Ray J. Dolan. Marc Guitart-Masip is funded by a Max Plank Society Award to Ray J. Dolan. The authors would like to thank Fred H. Hamker and Julien Vitay for their helpful suggestions.
Aberman, J. E., and Salamone, J. D. (1999). Nucleus accumbens dopamine depletions make rats more sensitive to high ratio requirements but do not impair primary food reinforcement. Neuroscience 92, 549–552.
Alexander, G. E., and Crutcher, M. D. (1990). Functional architecture of basal ganglia circuits: neural substrates of parallel processing. Trends Neurosci. 13, 266–271.
Aron, A. R., and Poldrack, R. A. (2006). Cortical and subcortical contributions to Stop signal response inhibition: role of the subthalamic nucleus. J. Neurosci. 26, 2424–2433.
Aronson, E. (1961). The effect of effort on the attractiveness of rewarded and unrewarded stimuli. J. Abnorm. Soc. Psychol. 63, 375–380.
Bardgett, M. E., Depenbrock, M., Downs, N., Points, M., and Green, L. (2009). Dopamine modulates effort-based decision making in rats. Behav. Neurosci. 123, 242–251.
Bautista, L. M., Tinbergen, J., and Kacelnik, A. (2001). To walk or to fly? How birds choose among foraging modes. Proc. Natl. Acad. Sci. U.S.A. 98, 1089–1094.
Bayer, H. M., and Glimcher, P. W. (2005). Midbrain dopamine neurons encode a quantitative reward prediction error signal. Neuron 47, 129–141.
Beckman, M., Johansen-Berg, H., and Rushworth, M. F. S. (2009). Connectivity-based parcellation of human cingulate cortex and its relation to functional specialization. J. Neurosci. 29, 1175–1190.
Berridge, K. C., and Robinson, T. E. (1998). What is the role of dopamine in reward: hedonic impact, reward learning, or incentive salience? Brain Res. Brain Res. Rev. 28, 309–369.
Berridge, K. C., Venier, I. L., and Robinson, T. E. (1989). Taste reactivity analysis of 6-hydroxydopamine-induced aphagia: implications for arousal and anhedonia hypotheses of dopamine function. Behav. Neurosci. 103, 36–45.
Bolam, J. P., Magill, P. J., and Bevan, M. D. (2002). “The functional organisation of the basal ganglia: new insights from anatomical and physiological analyses,” in Basal Ganglia VII, eds L. Nicholson and R. Faull (New York, NY: Kluwer Academic/Plenum Publishers), 3711–3378.
Botvinick, M. M., Huffstetler, S., and McGuire, J. (2009). Effort discounting in human nucleus accumbens. Cogn. Affect. Behav. Neurosci. 9, 16–27.
Bush, G., Luu, P., and Posner, M. (2000). Cognitive and emotional influences in anterior cingulate cortex. Trends Cogn. Sci. 4, 215–222.
Collier, G., Hirsch, E., Levitsky, D., and Leshner, A. I. (1975). Effort as a dimension of spontaneous activity in rats. J. Comp. Physiol. Psychol. 88, 89–96.
Collier, G., and Levitsky, D. A. (1968). Operant running as a function of deprivation and effort. J. Comp. Physiol. Psychol. 66, 522–523.
Cousins, M. S., and Salamone, J. D. (1994). Nucleus accumbens dopamine depletions in rats affect relative response allocation in a novel cost/benefit procedure. Pharmacol. Biochem. Behav. 49, 85–91.
Critchley, H. D., Mathias, C. J., Josephs, O., O’Doherty, J., Zanini, S., Dewar, B.-K., Cipolotti, L., Shallice, T., and Dolan, R. J. (2003). Human cingulate cortex and autonomic control: converging neuroimaging and clinical evidence. Brain 126, 2139–2152.
Croxson, P. L., Walton, M. E., O’Reilly, J. X., Behrens, T. E. J., and Rushworth, M. F. S. (2009). Effort-based cost-benefit valuation and the human brain. J. Neurosci. 29, 4531–4541.
Daw, N. D., and Doya, K. (2006). The computational neurobiology of learning and reward. Curr. Opin. Neurobiol. 16, 199–204.
Denk, F., Walton, M. E., Jennings, K. A., Sharp, T., Rushworth, M. F. S., and Bannerman, D. M. (2005). Differential involvement of serotonin and dopamine systems in cost-benefit decisions about delay or effort. Psychopharmacology (Berl.) 179, 587–596.
Duckworth, A. L., Peterson, C., Matthews, M. D., and Kelly, D. R. (2007). Grit: perseverance and passion for long-term goals. J. Pers. Soc. Psychol. 92, 1087–1101.
Eisenberger, R., Weier, F., Masterson, F. A., and Theis, L. Y. (1989). Fixed-ratio schedules increase generalized self-control: preference for large rewards despite high effort or punishment. J. Exp. Psychol. Anim. Behav. Process. 15, 383–392.
Eslinger, P. J., and Damasio, A. R. (1985). Severe disturbance of higher cognition after bilateral frontal lobe ablation. Neurology 35, 1731–1741.
Floresco, S. B., and Ghods-Sharifi, S. (2007). Amygdala-prefrontal cortical circuitry regulates effort-based decision making. Cereb. Cortex 17, 251–260.
Floresco, S. B., Tse, M. T. L., and Ghods-sharifi, S. (2008a). Dopaminergic and glutamatergic regulation of effort- and delay-based decision making. Neuropsychopharmacology 33, 1966–1979.
Floresco, S. B., St Onge, J. R., Ghods-sharifi, S., and Winstanley, C. A. (2008b). Cortico-limbic-striatal circuits subserving different forms of cost-benefit decision making. Cogn. Affect. Behav. Neurosci. 8, 375–379.
Frank, M. J. (2005). Dynamic dopamine modulation in the basal ganglia: a neurocomputational account of cognitive deficits in medicated and non-medicated Parkinsonism. J. Cogn. Neurosci. 17, 51–72.
Frank, M. J. (2006). Hold your horses: a dynamic computational role for the subthalamic nucleus in decision making. Neural Netw. 19, 1120–1136.
Frank, M. J., and Fossella, J. A. (2011). Neurogenetics and pharmacology of learning, motivation, and cognition. Neuropsychopharmacology 36, 133–152.
Frank, M. J., Loughry, B., and O’Reilly, R. C. (2001). Interactions between frontal cortex and basal ganglia in working memory: a computational model. Cogn. Affect. Behav. Neurosci. 1, 137–160.
Frank, M. J., Seeberger, L. C., and O’Reilly, R. C. (2004). By carrot or by stick: cognitive reinforcement learning in parkinsonism. Science 306, 1940–1943.
Friedrich, A. M., and Zentall, T. R. (2004). Pigeons shift their preference toward locations of food that take more effort to obtain. Behav. Processes 67, 405–415.
Gan, J. O., Walton, M. E., and Phillips, P. E. M. (2010). Dissociable cost and benefit encoding of future rewards by mesolimbic dopamine. Nat. Neurosci. 13, 25–27.
Ghods-sharifi, S., and Floresco, S. B. (2010). Differential effects on effort discounting induced by inactivations of the nucleus accumbens core or shell. Behav. Neurosci. 124.
Gurney, K., Prescott, T. J., and Redgrave, P. (2001). A computational model of action selection in the basal ganglia. I. A new functional anatomy. Biol. Cybern. 84, 401–410.
Gusnard, D. A., Ollinger, J. M., Shulman, G. L., Cloninger, C. R., Price, J. L., Van Essen, D. C., and Raichle, M. E. (2003). Persistence and brain circuitry. Proc. Natl. Acad. Sci. U.S.A. 100, 3479–3484.
Haber, S. N., and Knutson, B. (2010). The reward circuit: linking primate anatomy and human imaging. Neuropsychopharmacology 35, 4–26.
Hadland, K. A., Rushworth, M. F. S., Gaffan, D., and Passingham, R. E. (2003). The anterior cingulate and reward-guided selection of actions. J. Neurophysiol. 89, 1161–1164.
Humphries, M. D., and Prescott, T. J. (2010). The ventral basal ganglia, a selection mechanism at the crossroads of space, strategy, and reward. Prog. Neurobiol. 90, 385–417.
Ikemoto, S. (2007). Dopamine reward circuitry: two projection systems from the ventral midbrain to the nucleus accumbens-olfactory tubercle complex. Brain Res. Rev. 56, 27–78.
Johnson, A. W., and Gallagher, M. (2010). Greater effort boosts the affective taste properties of food. Proc. Biol. Sci. 278, 1450–1456.
Kanarek, R. B., and Collier, G. (1973). Effort as a determinant of choice in rats. J. Comp. Physiol. Psychol. 84, 332–338.
Kelley, A. E., Baldo, B. A., Pratt, W. E., and Will, M. J. (2005). Corticostriatal-hypothalamic circuitry and food motivation: integration of energy, action and reward. Physiol. Behav. 86, 773–795.
Kennerley, S. W., Walton, M. E., Behrens, T. E. J., Buckley, M. J., and Rushworth, M. F. (2006). Optimal decision making and the anterior cingulate cortex. Nat. Neurosci. 9, 940–947.
Kool, W., McGuire, J. T., Rosen, Z. B., and Botvinick, M. M. (2010). Decision making and the avoidance of cognitive demand. J. Exp. Psychol. Gen. 139, 665–682.
Kunishio, K., and Haber, S. N. (1994). Primate cingulostriatal projection: limbic striatal versus sensorimotor striatal input. J. Comp. Neurol. 390, 337–356.
Kurniawan, I. T., Seymour, B., Talmi, D., Yoshida, W., Chater, N., and Dolan, R. J. (2010). Choosing to make an effort: the role of striatum in signaling physical effort of a chosen action. J. Neurophysiol. 104, 313–321.
Lau, B., and Glimcher, P. W. (2007). Action and outcome encoding in the primate caudate nucleus. J. Neurosci. 27, 14502–14514.
Lau, B., and Glimcher, P. W. (2008). Value representations in the primate striatum during matching behavior. Neuron 58, 451–463.
Levy, R., and Dubois, B. (2006). Apathy and the functional anatomy of the prefrontal cortex-basal ganglia circuits. Cereb. Cortex 16, 916–928.
Lewis, M. (1964). Effect of effort on value: an exploratory study of children. Child Dev. 35, 1337–1342.
Li, M., and Fleming, A. (2003). The nucleus accumbens shell is critical for normal expression of pup-retrieval in postpartum female rats. Behav. Brain Res. 145, 99–111.
Mcclure, S. M., Berns, G. S., and Montague, P. R. (2003). Temporal prediction errors in a passive learning task activate human striatum. Neuron 38, 339–346.
McGuire, J. T., and Botvinick, M. M. (2010). Prefrontal cortex, cognitive control, and the registration of decision costs. Proc. Natl. Acad. Sci. U.S.A. 107, 7922–7926.
Montague, P. R., and Berns, G. S. (2002). Neural economics and the biological substrates of valuation. Neuron 36, 265–284.
Montague, P. R., Dayan, P., and Sejnowski, T. J. (1996). A framework for mesencephalic predictive Hebbian learning. J. Neurosci. 16, 1936–1947.
Morris, G., Nevet, A., Arkadir, D., Vaadia, E., and Bergman, H. (2006). Midbrain dopamine neurons encode decisions for future action. Nat. Neurosci. 9, 1057–1063.
Naccache, L., Dehaene, S., Cohen, L., Habert, M.-O., Guichart-Gomez, E., Galanaud, D., and Willer, J.-C. (2005). Effortless control: executive attention and conscious feeling of mental effort are dissociable. Neuropsychologia 43, 1318–1328.
Nicola, S. M. (2007). The nucleus accumbens as part of a basal ganglia action selection circuit. Psychopharmacology (Berl.) 191, 521–550.
Nicola, S. M. (2010). The flexible approach hypothesis: unification of effort and cue-responding hypotheses for the role of nucleus accumbens dopamine in the activation of reward-seeking behavior. J. Neurosci. 30, 16585–16600.
Niv, Y., Daw, N., and Dayan, P. (2005). “How fast to work: response vigor, motivation and tonic dopamine,” in Neural Information Processing, eds Y. Weiss, B. Scholkopf, and J. Platt (Cambridge, MA: MIT Press), 1019–1026.
Niv, Y., Daw, N. D., Joel, D., and Dayan, P. (2007). Tonic dopamine: opportunity costs and the control of response vigor. Psychopharmacology (Berl.) 191, 507–520.
O’Doherty, J., Dayan, P., Schultz, J., Deichmann, R., Friston, K., and Dolan, R. J. (2004). Dissociable roles of ventral and dorsal striatum in instrumental conditioning. Science 304, 452–454.
O’Doherty, J. P., Dayan, P., Friston, K., Critchley, H., and Dolan, R. J. (2003). Temporal difference models and reward-related learning in the human brain. Neuron 38, 329–337.
Parkinson, J. A., Olmstead, M. C., Burns, L. H., Robbins, T. W., and Everitt, B. J. (1999). Dissociation in effects of lesions of the nucleus accumbens core and shell on appetitive pavlovian approach behavior and the potentiation of conditioned reinforcement and locomotor activity by D-amphetamine. J. Neurosci. 19, 2401–2411.
Pennartz, C. M., Groenewegen, H. J., and Lopes da Silva, F. H. (1994). The nucleus accumbens as a complex of functionally distinct neuronal ensembles: an integration of behavioural, electrophysiological and anatomical data. Prog. Neurobiol. 42, 719–761.
Pessiglione, M., Schmidt, L., Draganski, B., Kalisch, R., Lau, H., Dolan, R. J., and Frith, C. D. (2007). How the brain translates money into force: a neuroimaging study of subliminal motivation. Science 316, 904–906.
Phillips, P. E. M., Walton, M. E., and Jhou, T. C. (2007). Calculating utility: preclinical evidence for cost-benefit analysis by mesolimbic dopamine. Psychopharmacology (Berl.) 191, 483–495.
Picard, N., and Strick, P. L. (2001). Imaging the premotor areas. Curr. Opin. Neurobiol. 11, 663–672.
Pine, A., Shiner, T., Seymour, B., and Dolan, R. J. (2010). Dopamine, time, and impulsivity in humans. J. Neurosci. 30, 8888–8896.
Redgrave, P., and Gurney, K. (2006). The short-latency dopamine signal: a role in discovering novel actions? Nat. Rev. Neurosci. 7, 967–975.
Redgrave, P., Prescott, T. J., and Gurney, K. (1999). Is the short-latency dopamine response too short to signal reward error? Trends Neurosci. 2236, 146–151.
Reynolds, J. N., Hyland, B. I., and Wickens, J. R. (2001). A cellular mechanism of reward-related learning. Nature 413, 67–70.
Reynolds, S. M., and Berridge, K. C. (2002). Positive and negative motivation in nucleus accumbens shell: bivalent rostrocaudal gradients for GABA-elicited eating, taste “liking”/“disliking” reactions, place preference/avoidance, and fear. J. Neurosci. 22, 7308–7320.
Robinson, D. L., Venton, B. J., Heien, M. L. A. V., and Wightman, R. M. (2003). Detecting subsecond dopamine release with fast-scan cyclic voltammetry in vivo. Clin. Chem. 49, 1763–1773.
Roesch, M. R., Calu, D. J., and Schoenbaum, G. (2007). Dopamine neurons encode the better option in rats deciding between differently delayed or sized rewards. Nat. Neurosci. 10, 1615–1624.
Roitman, M. F., Stuber, G. D., Phillips, P. E. M., Wightman, R. M., and Carelli, R. M. (2004). Dopamine operates as a subsecond modulator of food seeking. J. Neurosci. 24, 1265–1271.
Rushworth, M. F. S., Behrens, T. E. J., Rudebeck, P. H., and Walton, M. E. (2007). Contrasting roles for cingulate and orbitofrontal cortex in decisions and social behaviour. Trends Cogn. Sci. (Regul. Ed.) 11, 168–176.
Salamone, J. D. (2007). Functions of mesolimbic dopamine: changing concepts and shifting paradigms. Psychopharmacology (Berl.) 191, 389.
Salamone, J. D., and Correa, M. (2002). Motivational views of reinforcement: implications for understanding the behavioral functions of nucleus accumbens dopamine. Behav. Brain Res. 137, 3–25.
Salamone, J. D., Correa, M., Farrar, A., and Mingote, S. M. (2007). Effort-related functions of nucleus accumbens dopamine and associated forebrain circuits. Psychopharmacology (Berl.) 191, 461–482.
Salamone, J. D., Correa, M., Mingote, S., and Weber, S. M. (2003). Nucleus accumbens dopamine and the regulation of effort in food-seeking behaviour: implications for studies of natural motivation, psychiatry, and drug abuse. J. Pharmacol. Exp. Ther. 305, 1–8.
Salamone, J. D., Cousins, M. S., and Bucher, S. (1994). Anhedonia or anergia? Effects of haloperidol and nucleus accumbens dopamine depletion on instrumental response selection in a T-maze cost/benefit procedure. Behav. Brain Res. 65, 221–229.
Salomon, L., Lanteri, C., Glowinski, J., and Tassin, J.-P. (2006). Behavioral sensitization to amphetamine results from an uncoupling between noradrenergic and serotonergic neurons. Proc. Natl. Acad. Sci. U.S.A. 103, 7476–7481.
Samejima, K., Ueda, Y., Doya, K., and Kimura, M. (2005). Representation of action-specific reward values in the striatum. Science 310, 1337–1340.
Schmidt, L., d’Arc, B. F., Lafargue, G., Galanaud, D., Czernecki, V., Grabli, D., Schüpbach, M., Hartmann, A., Lévy, R., Dubois, B., and Pessiglione, M. (2008). Disconnecting force from money: effects of basal ganglia damage on incentive motivation. Brain 131, 1303–1310.
Schneider, J. S. (2007). Behavioral persistence deficit in Parkinson’s disease patients. Eur. J. Neurol. 14, 300–304.
Schultz, W., Dayan, P., and Montague, P. R. (1997). A neural substrate of prediction and reward. Science 275, 1593–1599.
Shackman, A. J., Salomons, T. V., Slagter, H. A., Fox, A. S., Winter, J. J., and Davidson, R. J. (2011). The integration of negative affect, pain and cognitive control in the cingulate cortex. Nat. Rev. Neurosci. 12, 154–167.
Shima, K., and Tanji, J. (1998). Role for cingulate motor area cells in voluntary movement selection based on reward. Science 282, 1335–1338.
Stevens, J. R., Rosati, A. G., Ross, K. R., and Hauser, M. D. (2005). Will travel for food: spatial discounting in two new world monkeys. Curr. Biol. 15, 1855–1860.
Stroop, J. R. (1935). Studies of interference in serial verbal reactions. J. Exp. Psychol. 18, 643–662.
Sutton, R. S., and Barto, A. G. (1998). Reinforcement Learning: An Introduction. Cambridge, MA: MIT Press.
Tekin, S., and Cummings, J. L. (2002). Frontal-subcortical neuronal circuits and clinical neuropsychiatry: an update. J. Psychosom. Res. 53, 647–654.
van Reekum, R., Stuss, D. T., and Ostrander, L. (2005). Apathy: why care? J. Neuropsychiatry Clin. Neurosci. 17, 7–19.
Vitay, J., and Hamker, F. H. (2010). A computational model of basal ganglia and its role in memory retrieval in rewarded visual memory tasks. Front. Comput. Neurosci. 4:13. doi: 10.3389/fncom.2010.00013
Voorn, P., Vanderschuren, L. J., Groenewegen, H. J., Robbins, T. W., and Pennartz, C. M. (2004). Putting a spin on the dorsal-ventral divide of the striatum. Trends Neurosci. 27, 468–474.
Walton, M. E., Devlin, J. T., and Rushworth, M. F. S. (2004). Interactions between decision making and performance monitoring within prefrontal cortex. Nat. Neurosci. 7, 1259–1265.
Walton, M. E., Groves, J., Jennings, K. A., Croxson, P. L., Sharp, T., Rushworth, M., and Bannerman, D. M. (2009). Comparing the role of the anterior cingulate cortex and 6-hydroxydopamine nucleus accumbens lesions on operant effort-based decision making. Eur. J. Neurosci. 29, 1678–1691.
Walton, M. E., Kennerley, S. W., Bannerman, D. M., Phillips, P. E. M., and Rushworth, M. F. S. (2006). Weighing up the benefits of work: behavioral and neural analyses of effort-related decision making. Neural. Netw. 19, 1302–1314.
Wickens, J. R., Budd, C. S., Hyland, B. I., and Arbuthnott, G. W. (2007). Striatal contributions to reward and decision making: making sense of regional variations in a reiterated processing matrix. Ann. N. Y. Acad. Sci. 1104, 192–212.
Keywords: basal ganglia, vigor, effort, ACC, dopamine, apathy
Citation: Kurniawan IT, Guitart-Masip M and Dolan RJ (2011) Dopamine and effort-based decision making. Front. Neurosci. 5:81. doi: 10.3389/fnins.2011.00081
Received: 14 January 2011;
Accepted: 06 June 2011;
Published online: 21 June 2011.
Edited by:
Julia Trommershaeuser, New York University, USAReviewed by:
Joseph W. Kable, University of Pennsylvania, USACopyright: © 2011 Kurniawan, Guitart-Masip and Dolan. This is an open-access article subject to a non-exclusive license between the authors and Frontiers Media SA, which permits use, distribution and reproduction in other forums, provided the original authors and source are credited and other Frontiers conditions are complied with.
*Correspondence: Irma Triasih Kurniawan, Cognitive, Perceptual, and Brain Sciences, University College London, Gower Street, London WC1E 6BT, UK. e-mail:aS5rdXJuaWF3YW5AdWNsLmFjLnVr
Disclaimer: All claims expressed in this article are solely those of the authors and do not necessarily represent those of their affiliated organizations, or those of the publisher, the editors and the reviewers. Any product that may be evaluated in this article or claim that may be made by its manufacturer is not guaranteed or endorsed by the publisher.
Research integrity at Frontiers
Learn more about the work of our research integrity team to safeguard the quality of each article we publish.