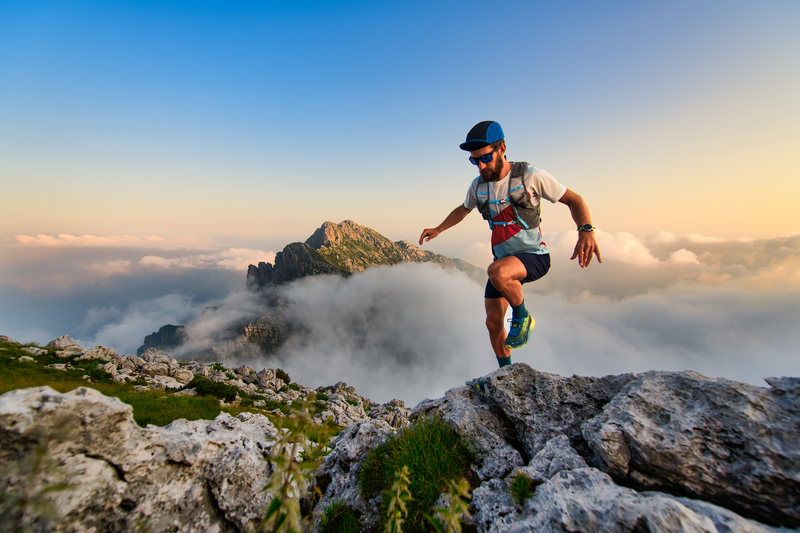
95% of researchers rate our articles as excellent or good
Learn more about the work of our research integrity team to safeguard the quality of each article we publish.
Find out more
ORIGINAL RESEARCH article
Front. Neurorobot.
Volume 19 - 2025 | doi: 10.3389/fnbot.2025.1582995
This article is part of the Research Topic Advancing Neural Network-Based Intelligent Algorithms in Robotics: Challenges, Solutions, and Future Perspectives - Volume II View all articles
The final, formatted version of the article will be published soon.
You have multiple emails registered with Frontiers:
Please enter your email address:
If you already have an account, please login
You don't have a Frontiers account ? You can register here
Introduction: Understanding human actions in complex environments is crucial for advancing applications in areas such as surveillance, robotics, and autonomous systems. Identifying actions from UAV-recorded videos becomes more challenging as the task presents unique challenges, including motion blur, dynamic background, lighting variations, and varying viewpoints. The presented work develops a deep learning system that recognizes multi-person behaviors from data gathered by UAVs.The proposed system provides higher recognition accuracy while maintaining robustness along with dynamic environmental adaptability through the integration of different features and neural network models. The study supports the wider development of neural network systems utilized in complicated contexts while creating intelligent UAV applications utilizing neural networks.The proposed study uses deep learning and feature extraction approaches to create a novel method to recognize various actions in UAV-recorded video. The proposed model improves identification capacities and system robustness by addressing motion dynamic problems and intricate environmental constraints, encouraging advancements in UAV-based neural network systems.We proposed a deep learning-based framework with feature extraction approaches that may effectively increase the accuracy and robustness of multi-person action recognition in the challenging scenarios. Compared to the existing approaches, our system achieved 91.50% on MOD20 dataset and 89.71% on Okutama-Action. These results do, in fact, show how useful neural networkbased methods are for managing the limitations of UAV-based application.Discussion: Results how that the proposed framework is indeed effective at multi-person action recognition under difficult UAV conditions.
Keywords: Unmanned Aerial Vehicle, neural network models, deep learning, Human action recognition, CNN, RNN, image processing, action classification Neural network models
Received: 25 Feb 2025; Accepted: 28 Mar 2025.
Copyright: © 2025 Alshehri, Zahoor, Alqahtani, Alshahrani, Al-Hammadi, Jalal and Liu. This is an open-access article distributed under the terms of the Creative Commons Attribution License (CC BY). The use, distribution or reproduction in other forums is permitted, provided the original author(s) or licensor are credited and that the original publication in this journal is cited, in accordance with accepted academic practice. No use, distribution or reproduction is permitted which does not comply with these terms.
* Correspondence:
Ahmad Jalal, Air University, Islamabad, Pakistan
Hui Liu, University of Bremen, Bremen, 28359, Bremen, Germany
Disclaimer: All claims expressed in this article are solely those of the authors and do not necessarily represent those of their affiliated organizations, or those of the publisher, the editors and the reviewers. Any product that may be evaluated in this article or claim that may be made by its manufacturer is not guaranteed or endorsed by the publisher.
Research integrity at Frontiers
Learn more about the work of our research integrity team to safeguard the quality of each article we publish.