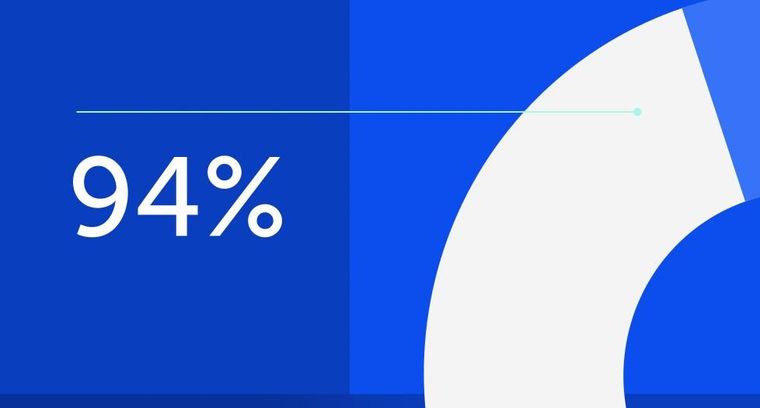
94% of researchers rate our articles as excellent or good
Learn more about the work of our research integrity team to safeguard the quality of each article we publish.
Find out more
ORIGINAL RESEARCH article
Front. Neurorobot.
Volume 19 - 2025 | doi: 10.3389/fnbot.2025.1576438
This article is part of the Research TopicPerceiving the World, Planning the Future: Advanced Perception and Planning Technology in RoboticsView all 3 articles
The final, formatted version of the article will be published soon.
Select one of your emails
You have multiple emails registered with Frontiers:
Notify me on publication
Please enter your email address:
If you already have an account, please login
You don't have a Frontiers account ? You can register here
Early and accurate diagnosis of pneumonia is crucial to improve cure rates and reduce mortality.Traditional chest X-ray analysis relies on physician experience, which can lead to subjectivity and misdiagnosis. To address this, we propose a novel pneumonia diagnosis method using the Fast-YOLO deep learning network that we introduced. First, we constructed a pneumonia dataset containing five categories and applied image enhancement techniques to increase data diversity and improve the model's generalization ability. Next, the YOLOv11 network structure was redesigned to accommodate the complex features of pneumonia X-ray images. By integrating the C3k2 module, DCNv2, and DynamicConv, the Fast-YOLO network effectively enhanced feature representation and reduced computational complexity (FPS increased from 53 to 120). Experimental results subsequently show that our method outperforms other commonly used detection models in terms of accuracy, recall, and mAP, offering better real-time detection capability and clinical application potential.X-ray images, providing clinicians with an efficient and accurate auxiliary tool. This helps accelerate the diagnosis process and improve diagnostic accuracy. Related program will be open source in the future: https://github.com/Zhaobin7/Fast-Yolo. 2 Pneumonia Dataset and Evaluation Metrics 2.1 Pneumonia Dataset MIMIC-CXR (MIMIC Chest X-ray) is an open-source chest X-ray dataset, and the dataset is designed to provide data support for medical image analysis, disease prediction, and the development of automated diagnostic systems. It contains a large number of accurately annotated chest X-ray images along with corresponding pathology reports, covering a variety of pulmonary diseases, with pneumonia being one of the key categories. The MIMIC-CXR dataset includes over 200,000 chest Xray images, representing a diverse range of chest diseases, such as pneumonia, tuberculosis, and pneumothorax, with the majority of the images sourced from hospitalized patients. All images are stored in DICOM (Digital Imaging and Communications in Medicine) format, ensuring highresolution and clear image quality [13][14][15] . However, despite the detailed disease labels provided in the pathology reports, the reports are written by different radiologists, which may introduce subjectivity and label inconsistency. This variance can potentially affect the training performance of disease
Keywords: Classification detection, pneumonia detection, YOLO model, deep learning, pneumonia diagnosis
Received: 14 Feb 2025; Accepted: 17 Mar 2025.
Copyright: © 2025 Xie, Zhu, Zhao and Jiang. This is an open-access article distributed under the terms of the Creative Commons Attribution License (CC BY). The use, distribution or reproduction in other forums is permitted, provided the original author(s) or licensor are credited and that the original publication in this journal is cited, in accordance with accepted academic practice. No use, distribution or reproduction is permitted which does not comply with these terms.
* Correspondence:
Yanchun Xie, Department of Orthopaedics, General Hospital of Northern Theater Command, Shenyang, China
Binbin Zhu, The Fourth People's Hospital of Shenyang, Shenyang, Liaoning Province, China
Disclaimer: All claims expressed in this article are solely those of the authors and do not necessarily represent those of their affiliated organizations, or those of the publisher, the editors and the reviewers. Any product that may be evaluated in this article or claim that may be made by its manufacturer is not guaranteed or endorsed by the publisher.
Supplementary Material
Research integrity at Frontiers
Learn more about the work of our research integrity team to safeguard the quality of each article we publish.