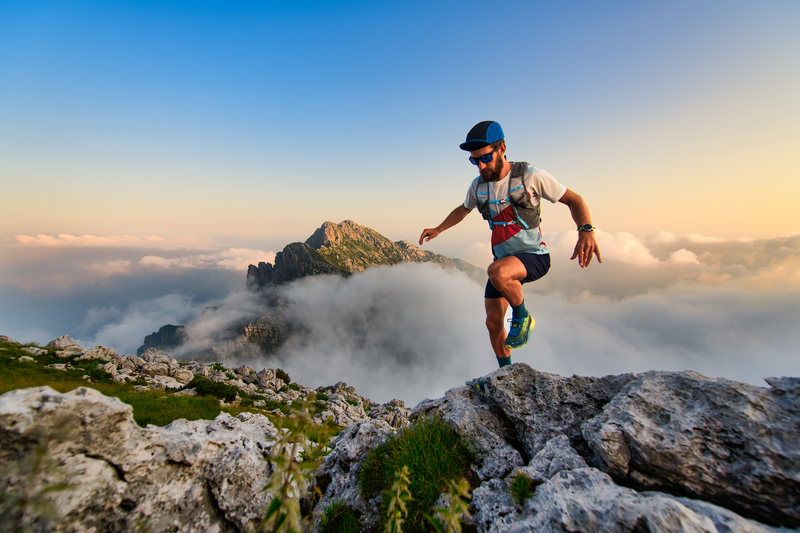
94% of researchers rate our articles as excellent or good
Learn more about the work of our research integrity team to safeguard the quality of each article we publish.
Find out more
ORIGINAL RESEARCH article
Front. Neurorobot.
Volume 19 - 2025 | doi: 10.3389/fnbot.2025.1531894
This article is part of the Research Topic Recent Advances in Image Fusion and Quality Improvement for Cyber-Physical Systems, Volume III View all 8 articles
The final, formatted version of the article will be published soon.
You have multiple emails registered with Frontiers:
Please enter your email address:
If you already have an account, please login
You don't have a Frontiers account ? You can register here
In the field of collaborative robotics, precise human pose recognition is a key technology for achieving seamless human-robot interaction, especially in complex dynamic environments.Traditional methods face limitations in handling occlusions, lighting variations, and motion continuity. To address these challenges, we propose a deep learning-based pose recognition model, PoseRL-Net, designed to enhance accuracy and robustness in human pose estimation.PoseRL-Net integrates components such as a Spatial-Temporal Graph Convolutional Network (STGCN), attention mechanism, Gated Recurrent Unit(GRU) module, pose refinement, and symmetry constraints to build a robust pose estimation framework. The STGCN effectively extracts spatial and temporal features, the attention mechanism improves focus on key pose features, the GRU module ensures temporal consistency in actions, and the pose refinement and symmetry constraints enhance structural plausibility and stability in predictions. Extensive experiments on the Human3.6M and MPI-INF-3DHP datasets demonstrate that PoseRL-Net surpasses existing state-of-the-art models on key metrics such as MPJPE and P-MPJPE, highlighting its superior performance across various pose recognition tasks. PoseRL-Net not only improves pose estimation accuracy but also provides crucial support for intelligent decision-making and motion planning for robots in dynamic and complex scenarios, offering significant practical value.
Keywords: Human Pose Estimation, 3D Skeleton Modeling, Spatial-temporal graph convolution, attention mechanism, Robot-Assisted Motion Analysis
Received: 21 Nov 2024; Accepted: 17 Feb 2025.
Copyright: © 2025 Liu and Wang. This is an open-access article distributed under the terms of the Creative Commons Attribution License (CC BY). The use, distribution or reproduction in other forums is permitted, provided the original author(s) or licensor are credited and that the original publication in this journal is cited, in accordance with accepted academic practice. No use, distribution or reproduction is permitted which does not comply with these terms.
* Correspondence:
Hui Wang, Shanghai Jianqiao University, Shanghai, 201315, Shanghai, China
Disclaimer: All claims expressed in this article are solely those of the authors and do not necessarily represent those of their affiliated organizations, or those of the publisher, the editors and the reviewers. Any product that may be evaluated in this article or claim that may be made by its manufacturer is not guaranteed or endorsed by the publisher.
Research integrity at Frontiers
Learn more about the work of our research integrity team to safeguard the quality of each article we publish.