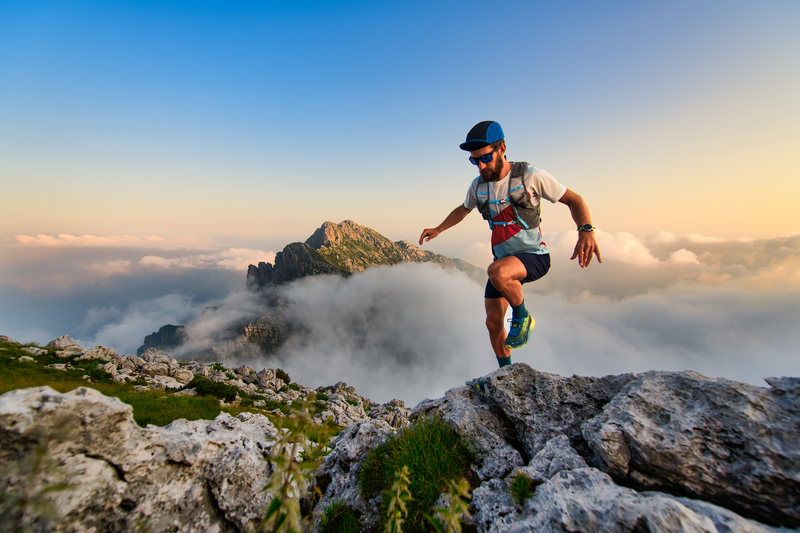
95% of researchers rate our articles as excellent or good
Learn more about the work of our research integrity team to safeguard the quality of each article we publish.
Find out more
ORIGINAL RESEARCH article
Front. Neurorobot.
Volume 19 - 2025 | doi: 10.3389/fnbot.2025.1482327
The final, formatted version of the article will be published soon.
You have multiple emails registered with Frontiers:
Please enter your email address:
If you already have an account, please login
You don't have a Frontiers account ? You can register here
The research and application of air traffic control automation systems in the field are constrained by the scarcity and low quality of radiotelephony communication data, especially in complex low-altitude airspace where communication data is less standardized. This study propose a deep reinforcement learning model based on BART, named BART-Reinforcement Learning (BRL), for standardizing non-standard control instructions encountered during training or operational scenarios. Leveraging the BART pre-trained language model, the BRL model is optimized through transfer learning and reinforcement learning techniques. This study evaluated the model's performance across various control datasets, including training flight data, civil aviation control operation data, and a dataset of generated control instructions derived from the "Radiotelephony Communications for Air Traffic Services" standard. Under ROUGE evaluation criteria, the BRL model exhibited significant improvements across all datasets, validating its efficacy. Additionally, we conducted a comprehensive evaluation of the model's performance based on the intent of land-air communication instructions, revealing a marked enhancement in standardizing instructions across diverse types. Compared to the baseline model, the overall accuracy increased by 10.5%, effectively alleviating the generalization challenges of deep learning models across disparate datasets.
Keywords: Radiotelephony communication, Air Traffic Control, Bart model, Low-altitude airspace, deep reinforcement learning
Received: 18 Aug 2024; Accepted: 17 Mar 2025.
Copyright: © 2025 Han, Pan and Jiang. This is an open-access article distributed under the terms of the Creative Commons Attribution License (CC BY). The use, distribution or reproduction in other forums is permitted, provided the original author(s) or licensor are credited and that the original publication in this journal is cited, in accordance with accepted academic practice. No use, distribution or reproduction is permitted which does not comply with these terms.
* Correspondence:
Boyuan Han, Civil Aviation Flight University of China, Guanghan, China
Disclaimer: All claims expressed in this article are solely those of the authors and do not necessarily represent those of their affiliated organizations, or those of the publisher, the editors and the reviewers. Any product that may be evaluated in this article or claim that may be made by its manufacturer is not guaranteed or endorsed by the publisher.
Research integrity at Frontiers
Learn more about the work of our research integrity team to safeguard the quality of each article we publish.