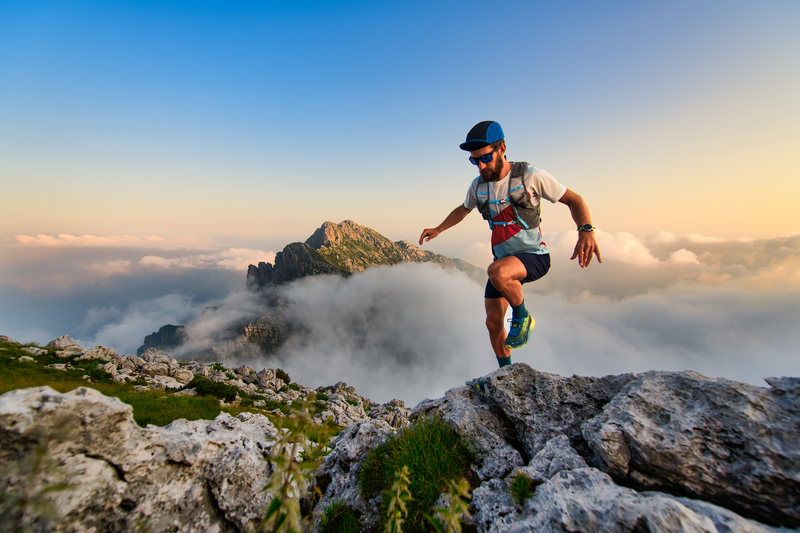
94% of researchers rate our articles as excellent or good
Learn more about the work of our research integrity team to safeguard the quality of each article we publish.
Find out more
EDITORIAL article
Front. Neurorobot. , 29 May 2024
Volume 18 - 2024 | https://doi.org/10.3389/fnbot.2024.1422982
This article is part of the Research Topic Recent Advances in Image Fusion and Quality Improvement for Cyber-Physical Systems, Volume II View all 6 articles
Editorial on the Research Topic
Recent advances in image fusion and quality improvement for cyber-physical systems, volume II
Multi-source visual information fusion and quality improvement can help the robotic system to perceive the real world. Image fusion is a computational technique fusing multi-source images from multiple sensors into a synthesized image that provides a comprehensive or reliable description. Quality improvement techniques can be used to address the challenge of low-quality image analysis tasks (Jin et al., 2017, 2023a, 2024; Liu et al., 2022; Wang G. et al., 2022; Guo et al., 2024). At present, a lot of brain-inspired algorithm methods (or models) are aggressively proposed to accomplish these two tasks, and the artificial neural network has become one of the most popular techniques in processing image fusion and quality improvement techniques in this decade, especially deep convolutional neural networks (Kong et al., 2022; Liu et al., 2022; Wang G. et al., 2022; Chen et al., 2023; Jin et al., 2023a). This is an exciting research field for the research community of image fusion, and many interesting Research Topics remain to be explored, such as deep few-shot learning, unsupervised learning, application of embodied neural systems, and industrial applications.
How to develop a sound biological neural network and embedded system to extract the multiple features of source images are two key questions that need to be addressed in the fields of image fusion and quality improvement. Hence, studies in this field can be divided into two aspects: new end-to-end neural network models for merging constituent parts during the image fusion process and the embodiment of artificial neural networks for image processing systems. In addition, current booming techniques, including deep neural systems and embodied artificial intelligence systems, are considered potential future trends for reinforcing image fusion performance and quality improvement (Wang W. et al., 2022; Yang et al., 2022; Zhang et al., 2022, 2023; Jin et al., 2023b; Liu et al., 2023; Mi et al., 2023).
The paper of Zhang et al. introduces a palmprint recognition method based on a gating mechanism and adaptive feature fusion. They propose a new network structure, GLGAnet, for extracting local and global features of palmprints. The method incorporates a gating mechanism to control features extracted by deep convolutional layers and Transformer modules, along with an adaptive convolution fusion module for multi-level feature fusion. Experimental results demonstrate that their method outperforms existing approaches on two datasets.
Many previous works overlooked the crucial support-query set interaction and the deeper information that needs to be explored. Zeng et al. propose a duplex network model utilizing the suppression and focus concept to address this issue. Their network includes dynamic convolution, prototype matching structure, and a hybrid attention module called DAAConv. The DPMCN model demonstrates superior performance over traditional prototype-based methods in dataset experiments.
In the third work, Peng et al. proposed a network structure called Context-Aware Lightweight Super-Resolution Network, which enhances the resolution of remote sensing images. This network combines local and global features and includes a Dynamic Weight Generation Branch to improve image quality while maintaining computational efficiency. Compared to existing methods, the proposed approach can reconstruct high-quality images at a lower cost.
In the fourth study, titled “Feature fusion network based on few-shot fine-grained classification,” Yang et al. introduced the Feature Fusion Similarity Network (FFSNet). This model employs global measures to accentuate the differences between classes while utilizing local measures to consolidate intra-class data, greatly enhancing the model's generalization ability. The method proposed in this paper has been validated to be effective.
In the fifth work, Chen et al. introduced ID-YOLOv7, a convolutional neural network specifically designed for insulator defect detection in power distribution networks, by introducing several novel methods, including Edge Detailed Shape Data Augmentation, Cross-Channel and Spatial Multi-Scale Attention module and Re-BiC module, to enhance the model's sensitivity to subtle defect features and fuse multi-scale contextual features. Experimental results demonstrate that the algorithm significantly boosts detection performance.
XJ: Writing – original draft. S-JL: Writing – review & editing. MW: Writing – review & editing. QJ: Writing – review & editing, Conceptualization.
The author(s) declare that financial support was received for the research, authorship, and/or publication of this article. This study was supported by the National Natural Science Foundation of China (Nos. 62101481 and 62261060), Yunnan Fundamental Research Projects (Nos. 202301AW070007, 202201AU070033, 202201AT070112, 202301AU070210, and 202401AT070470), and Major Scientific and Technological Project of Yunnan Province (No. 202202AD080002).
The authors declare that the research was conducted in the absence of any commercial or financial relationships that could be construed as a potential conflict of interest.
All claims expressed in this article are solely those of the authors and do not necessarily represent those of their affiliated organizations, or those of the publisher, the editors and the reviewers. Any product that may be evaluated in this article, or claim that may be made by its manufacturer, is not guaranteed or endorsed by the publisher.
Chen, S., Li, Z., Shen, D., An, Y., Yang, J., Lv, B., et al. (2023). Multi-exposure electric power monitoring image fusion method without ghosting based on exposure fusion framework and color dissimilarity feature. Front. Neuror. 16:1105385. doi: 10.3389/fnbot.2022.1105385
Guo, H., Jin, X., Jiang, Q., Wozniak, M., Wang, P., and Yao, S. (2024). “DMF-net: a dual remote sensing image fusion network based on multi-scale convolutional dense connectivity with performance measure,” in IEEE Transactions on Instrumentation and Measurement.
Jin, X., Hou, J., Zhou, W., and Lee, S. J. (2023b). Recent advances in image fusion and quality improvement for cyber-physical systems. Front. Neurorobot. 17:1201266. doi: 10.3389/fnbot.2023.1201266
Jin, X., Jiang, Q., Yao, S., Zhou, D., Nie, R., Hai, J., et al. (2017). A survey of infrared and visual image fusion methods. Infrar. Phys. Technol. 85, 478–501. doi: 10.1016/j.infrared.2017.07.010
Jin, X., Liu, L., Ren, X., Jiang, Q., Lee, S. J., Zhang, J., et al. (2024). “A restoration scheme for spatial and spectral resolution of panchromatic image using convolutional neural network,” in IEEE Journal of Selected Topics in Applied Earth Observations and Remote Sensing.
Jin, X., Xi, X., Zhou, D., Ren, X., Yang, J., and Jiang, Q. (2023a). An unsupervised multi-focus image fusion method based on transformer and U-Net. IET Image Proc. 17, 733–746. doi: 10.1049/ipr2.12668
Kong, W., Li, C., and Lei, Y. (2022). Multimodal medical image fusion using convolutional neural network and extreme learning machine. Front. Neuror. 16:1050981. doi: 10.3389/fnbot.2022.1050981
Liu, R., Jin, X., Hu, D., Zhang, J., Wang, Y., Zhang, J., et al. (2023). DualFlow: generating imperceptible adversarial examples by flow field and normalize flow-based model. Front. Neurorobot. 17:1129720. doi: 10.3389/fnbot.2023.1129720
Liu, S., Peng, W., Jiang, W., Yang, Y., Zhao, J., and Su, Y. (2022). Multi-focus image fusion dataset and algorithm test in real environment. Front. Neuror. 16:1024742. doi: 10.3389/fnbot.2022.1024742
Mi, Z., Chen, R., and Zhao, S. (2023). Research on steel rail surface defects detection based on improved YOLOv4 network. Front. Neurorobot. 17:1119896. doi: 10.3389/fnbot.2023.1119896
Wang, G., Li, W., Du, J., Xiao, B., and Gao, X. (2022). Medical image fusion and denoising algorithm based on a decomposition model of hybrid variation-sparse representation. IEEE J. Biomed. Health Inf. 26, 5584–5595. doi: 10.1109/JBHI.2022.3196710
Wang, W., Wang, X., Chen, G., and Zhou, H. (2022). Multi-view SoftPool attention convolutional networks for 3D model classification. Front. Neurorobot. 16:1029968. doi: 10.3389/fnbot.2022.1029968
Yang, Z., Li, X., and Li, J. (2022). Transformer-based progressive residual network for single image dehazing. Front. Neurorobot. 16:1084543. doi: 10.3389/fnbot.2022.1084543
Zhang, C., Xu, F., Wu, C., and Xu, C. (2022). A lightweight multi-dimension dynamic convolutional network for real-time semantic segmentation. Front. Neurorobot. 16:1075520. doi: 10.3389/fnbot.2022.1075520
Keywords: artificial neural networks, embedded learning system, feature extraction, image quality improvement, image fusion, robot vision
Citation: Jin X, Lee S-J, Wozniak M and Jiang Q (2024) Editorial: Recent advances in image fusion and quality improvement for cyber-physical systems, volume II. Front. Neurorobot. 18:1422982. doi: 10.3389/fnbot.2024.1422982
Received: 25 April 2024; Accepted: 06 May 2024;
Published: 29 May 2024.
Edited and reviewed by: Florian Röhrbein, Technische Universität Chemnitz, Germany
Copyright © 2024 Jin, Lee, Wozniak and Jiang. This is an open-access article distributed under the terms of the Creative Commons Attribution License (CC BY). The use, distribution or reproduction in other forums is permitted, provided the original author(s) and the copyright owner(s) are credited and that the original publication in this journal is cited, in accordance with accepted academic practice. No use, distribution or reproduction is permitted which does not comply with these terms.
*Correspondence: Xin Jin, eGlueGluX2ppbkAxNjMuY29t
Disclaimer: All claims expressed in this article are solely those of the authors and do not necessarily represent those of their affiliated organizations, or those of the publisher, the editors and the reviewers. Any product that may be evaluated in this article or claim that may be made by its manufacturer is not guaranteed or endorsed by the publisher.
Research integrity at Frontiers
Learn more about the work of our research integrity team to safeguard the quality of each article we publish.