- 1Rehab Technologies, Istituto Italiano di Tecnologia, Genoa, Italy
- 2CNRS, Univ Rennes, Inria, IRISA, Rennes, France
Introduction
Among the most recent enabling technologies, Digital Twins (DTs) emerge as data-intensive network-based computing solutions in multiple domains—from Industry 4.0 to Connected Health (Pires et al., 2019; Bagaria et al., 2020; Juarez et al., 2021; Phanden et al., 2021). A DT works as a virtual system for replicating, monitoring, predicting, and improving the processes and the features of a physical system—the Physical Twin (PT), connected in real-time with its DT (Grieves and Vickers, 2017; Kaur et al., 2020; Mourtzis et al., 2021; Volkov et al., 2021). Such a technology, based on advances in fields like the Internet of Things (IoT) and machine learning (Kaur et al., 2020), proposes novel ways to face the issues of complex systems as in Human-Robot Interaction (HRI) (Pairet et al., 2019) domains.
This position paper aims at proposing a physical-digital twinning approach to improve the understanding and the management of the PT in contexts of HRI according to the interdisciplinary perspective of neuroergonomics (Parasuraman, 2003; Frederic et al., 2020).
Approaching and Adopting Digital Twins
The DT definition is still an object of debate, and reaching one could be a necessary step for efficiently managing its technical requirements in terms of computing and connectivity (Shafto et al., 2012; Haag and Anderl, 2018; Jones et al., 2020; Kuehner et al., 2021; Singh et al., 2021; Botín-Sanabria et al., 2022; Wang D. et al., 2022). However, we can ignite our discussion by considering how Fuller et al. (2020) highlighted that a DT is not just a digital model or an offline simulation of a physical object. Nor does a DT correspond to a digital shadow, depicting the real-time states and changes of a PT that can just be manually modified. The changes in a DT automatically mirror and affect the status of its PT: the data flows bi-directionally (Van der Valk et al., 2020) and in real time between twins in digital and physical worlds, possibly without any human intervention (Liu et al., 2022) through the DT-driven control of an actuated PT. However, a DT is typically “played” by experts like managers, engineers, and designers as a complex interactive simulation to predict future issues in the PT according to its past and current behavior (Semeraro et al., 2021). This leads to new policies as feedback to the real system, even with the assistance of artificial intelligence layers (Umeda et al., 2019; Gichane et al., 2020). Considering their functions (Khan et al., 2022) each DT can focus on (i) monitoring a PT, (ii) simulating the future states of a PT, (iii) directly interacting—as an “operational DT”—with a cyber-physical system as PT.
Among the fields of DT application, robotics certainly offers several examples (Girletti et al., 2020; Matulis and Harvey, 2021) of twinning solutions, especially in conditions of HRI like human-robot collaboration (Malik and Bilberg, 2018; Maruyama et al., 2021; Tuli et al., 2021). In particular, literature in robotics offers interesting solutions of intuitive extended reality interfaces (Alfrink and Rossmann, 2019; Burghardt et al., 2020) to ease the interaction of an expert with a DT. In the next section, we propose that such an approach can be further enhanced by emulating certain PT components through a DT and others through a physical replica of the robotic system.
Phygital Twins in Human-Robot Interaction
Performing holistic, physical, and reality-based interaction with a robotic system is more intuitive for the user than contactless gestures to program or command the device and change its state to accomplish a task (Jacob et al., 2008; Heun et al., 2013; Blackler et al., 2019; Ravichandar et al., 2020). Following this reasoning, we decided to highlight the opportunity of emulating a PT through what we labeled as a “Phygital Twin.” This term has already been used by Sarangi et al. (2018) to describe an IoT setup designed to collect data and represent an environment (even through portable devices) to assist a farmer in precision agriculture paradigms. However, we envisioned the usage of this label for a wider class of solutions by pondering the meaning of the “phygital” attribute outside the domain of twinning processes.
As a neologism (merging two words: physical and digital), this attribute has been typically adopted across various domains like design and marketing, blending real and virtual dimensions as in its etymology (Gaggioli, 2017; Mikheev et al., 2021). This term was used, for instance, to define Tactile User Interfaces (TUIs) like the “phygital map” in Nakazawa and Tokuda (2007), the paradigms of “phygital play” (Lupetti et al., 2015) in mixed reality-based robotic games (MRRGs) (Prattico and Lamberti, 2020), and interactive solutions for work and education proposed during the COVID-19 pandemic (Chaturvedi et al., 2021; Burova et al., 2022).
Overall, these are just examples in a general virtual-real convergence trend (Tao and Zhang, 2017), like cyber-physical twins (Czwick and Anderl, 2020). This trend occurs in healthcare too (Gregory, 2022) about managing chronic conditions and predicting their progress or the therapeutic outcome (Voigt et al., 2021; Barresi et al., 2022). Furthermore, we must highlight how intrinsically phygital are the recent definitions of the metaverse, a digital world embracing cyber-physical systems and also DTs in its connection with the real world (Yoon et al., 2021).
Exploiting the phygital approach we foresee a Phygital Twin (PDT, highlighting both its physical and digital elements) as in the example in Figure 1. Within a PDT, certain components of the PT are replicated by digital objects and others by physical objects within an integrated extended reality model. These physical objects would be secondary instances of the same products (not necessarily a robot) in the PT. In Figure 1, an example of the human-exoskeleton system in a real context is the PT emulated by a DT (in green, on the left), based on a fully virtual model of the HRI system. On the other hand, the same PT can be represented (on the right) by a PDT, based on a virtual human “wearing” a real exoskeleton (identical to the one in the real-world context and, possibly, sustained by a mannequin) into a laboratory. Both settings, visualized by an expert through a mixed reality headset, enable the live visualization of anomalies in the right shoulder of the worker in this example.
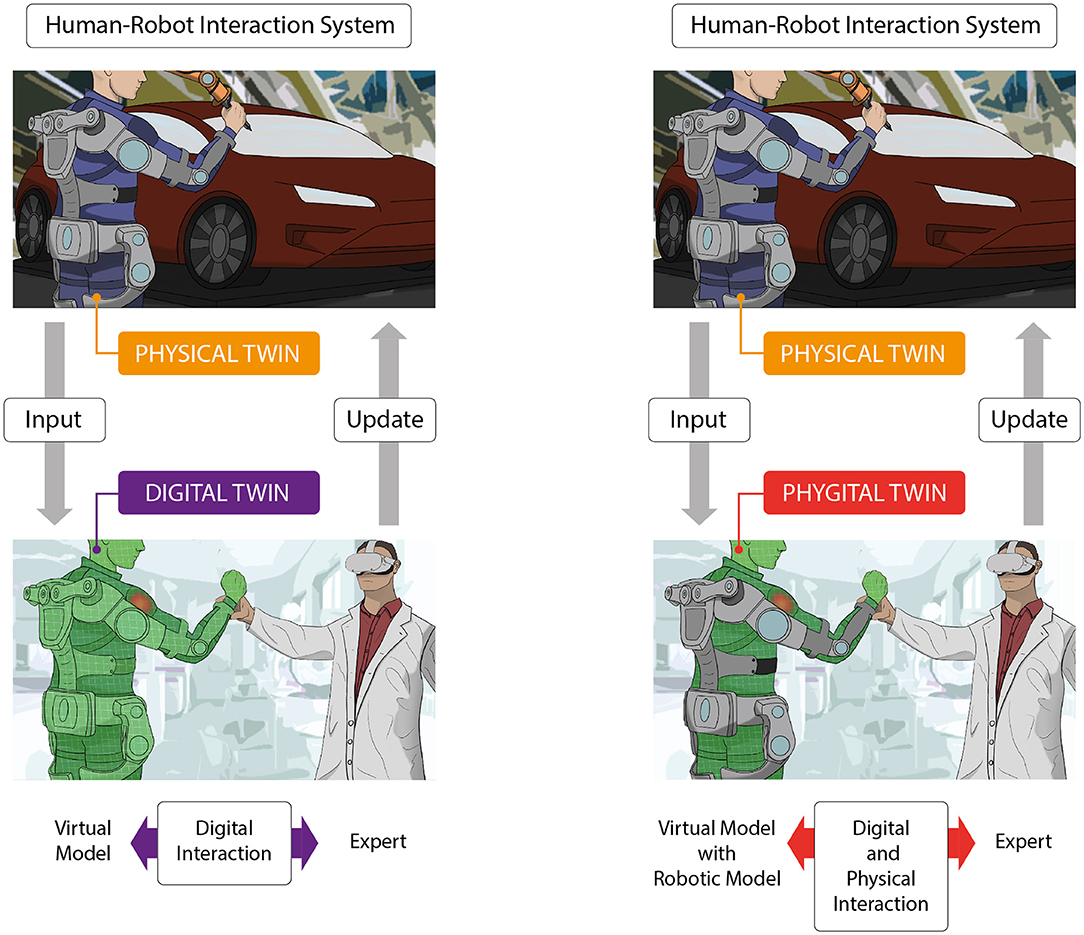
Figure 1. A Physical Twin (PT), based on a human-exoskeleton system in a context of usage, connected (on the left) to its Digital Twin (DT) in green, based on a virtual model of the human-robot interaction (HRI) system, or (on the right) to its Phygital Twin (PDT), based on a holographic human “wearing” a real exoskeleton in a laboratory.
Different from the case of the fully virtual model on the left, the expert on the right can decide to alter the phygital model through intuitive physical interactions with the lab exoskeleton (working as a TUI), performing tests according to past and current data from the PT. Indeed, the expert receives visual feedback from the DT and more intuitive visuotactile feedback from the PDT. After obtaining the informed consent of the worker in the PT system, the experts can also update the remote wearable robot software according to their predictions.
Thus, the PDTs enable intuitive phygital interactions with experts to assess and improve the PT. Furthermore, its physical components can emulate the ones of the PT more reliably than a virtual simulacrum because they are based on the same products. The PDT computer-generated elements may also be visualized through a virtual reality headset instead of a mixed reality one, according to the need of depicting the PT context as a whole. However, focusing further on the virtual human component can also be greatly advantageous to deepen our knowledge of the user's conditions, especially in terms of neuromotor and neurocognitive processes, as the next section will propose.
Neuroergonomic Twinning of HRI Systems
Through digital human modeling (Paul et al., 2021), DTs can contribute to monitoring, assessing, and designing different human-system interactions (Caputo et al., 2019; Greco et al., 2020; Sharotry et al., 2022; Wang B. et al., 2022) according to the perspective of human factors. In particular, neuroergonomics (Mehta and Parasuraman, 2013)—especially computational neuroergonomics (Farahani et al., 2019)—can advantageously exploit twinning for understanding how the human nervous system works in real contexts (Cheng et al., 2022), and improving the design of any item interacting with it. This is certainly true about neuroergonomics in HRI contexts (Cassioli et al., 2021) for applications like monitoring motor control difficulties (Memar and Esfahani, 2018), providing robots with adaptive features (Lim et al., 2021), and improving brain-robot interfaces (Mao et al., 2019). Overall, the exploitation of DTs in this field can inherit the corpus of knowledge in neuroscience, especially when human-machine interactions are investigated (Gaggioli, 2018; Ramos et al., 2021). Interestingly, literature in this area already shows several approaches presenting analogies with PDTs, which can contribute to neuroergonomics in HRI by offering intuitive interactions with a phygital emulation of the human-robot system.
For instance, the field of bionic prosthetics (Frossard and Lloyd, 2021) offers this kind of solution, with emphasis on twinning the residual limb more than the device. Interestingly, Chen et al. (2022) labeled as “mechatronics-twin” a framework integrating a 6-DoF manipulator with biomechanical models to explore, through simulations, the operational behaviors of prosthetic sockets with amputees. Such an example sounds quite close to the concept of PDT, which can have additional features of real-time bidirectionality, intuitive physical interaction, and ecological validity (resemblance with real contexts).
Furthermore, Pizzolato et al. (2019) proposed human neuromusculoskeletal (NMS) system models for DTs to improve the outcome of the interactions between users and assistive or rehabilitative machines. NMS models implemented in robot control solutions can offer phygital features. For instance, the output of the interaction between a user and a mechatronic device (possibly enriched by extended reality solutions) can become a quantifiable index of healthy and pathological conditions and responses to treatments. This would make such an output a peculiar type of digital biomarker (Wright et al., 2017): a “phygital biomarker” or possibly, a “neurophygital biomarker” (a promising step in this direction is based on neuromechanical biomarkers for rehabilomics) (Garro et al., 2021). In line with this reasoning, we could think about “neurophygital twins” to extract biomarkers from the activity of their PTs: mechatronic devices like rehabilitative exoskeletons (Buccelli et al., 2022) or, possibly, any other robot (including humanoids) designed to interact with humans wearing sensors.
Through intuitive phygital interactions between the researcher or the clinician and the lab replica of the same machine in the real world, neuroergonomic hypotheses on psychophysiological and motor processes underlying HRIs can be tested in simulated experiments based on a PDT. We could also envision the development of neurorobotic systems (Li et al., 2019) mimicking neurocognitive and neuromotor processes to physically replace a virtual human model in a PDT: in this case, the neurorobotic model would be validated through its interaction with another machine within the same PDT. However, before addressing such challenges, the current constraints in our knowledge and know-how must be pondered. Besides the technical limitations in twinning (first of all, the computational burden of emulating neural processes in ecologically valid settings, without considering the connectivity issues to approach the real-time standards), we must also highlight how both DTs and PDTs raise ethical issues on privacy and consent in data representation and storage, and on concepts like “normality” and enhancement (Bruynseels et al., 2018; Braun, 2021; Nyholm, 2021). These issues should be discussed within the frame of the enablers and the barriers to twinning adoption (Perno et al., 2020), even pondering the opportunities offered by novel technological frameworks (Yi et al., 2022).
Conclusion
This position paper presented a novel “twinning design” concept: PDT, based on physical replicas of PT components enriched with virtual models and computational features to establish intuitive and reliable phygital interactions with experts. Thus, a PDT would facilitate the experts' task of assessing and improving the PT conditions. Furthermore, PDTs provide neuroergonomics with tools for iterative human-centered design and evaluation of robotic systems into a “metalaboratory” before and after their deployment.
Author Contributions
GB devised the conceptual contents and structure of the paper and wrote the initial draft. CP, ML, and LDM improved the document and considering further potential applications of the proposed approach. All authors revised and approved the manuscript.
Conflict of Interest
The authors declare that the research was conducted in the absence of any commercial or financial relationships that could be construed as a potential conflict of interest.
Publisher's Note
All claims expressed in this article are solely those of the authors and do not necessarily represent those of their affiliated organizations, or those of the publisher, the editors and the reviewers. Any product that may be evaluated in this article, or claim that may be made by its manufacturer, is not guaranteed or endorsed by the publisher.
Acknowledgments
The authors thank Lorenzo Cavallaro (Rehab Technologies) for his help with the figure.
References
Alfrink, M., and Rossmann, J. (2019). “Enhanced interaction with industrial robots through extended reality relying on simulation-based digital twins,” in Proceedings of the Industrial Simulation Conference 2019 (Lisbon).
Bagaria, N., Laamarti, F., Badawi, H. F., Albraikan, A., Martinez Velazquez, R. A., and El Saddik, A. (2020). “Health 4.0: digital twins for health and well-being,” in Connected Health in Smart Cities (Cham: Springer), 143–152.
Barresi, G., Zenzeri, J., Tessadori, J., Laffranchi, M., Semprini, M., and Michieli, L. D. (2022). “Neuro-gerontechnologies: applications and opportunities,” in Internet of Things for Human-Centered Design (Singapore: Springer), 123–153.
Blackler, A., Desai, S., McEwan, M., Popovic, V., and Diefenbach, S. (2019). “Perspectives on the nature of intuitive interaction,” in Intuitive Interaction Research and Application, 19–39. doi: 10.1201/b22191-2
Botín-Sanabria, D. M., Mihaita, A. S., Peimbert-García, R. E., Ramírez-Moreno, M. A., Ramírez-Mendoza, R. A., and Lozoya-Santos, J. D. J. (2022). Digital twin technology challenges and applications: A comprehensive review. Remot. Sens. 14, 1335. doi: 10.3390/rs14061335
Braun, M. (2021). Represent me: please! towards an ethics of digital twins in medicine. J. Med. Ethics 47, 394–400. doi: 10.1136/medethics-2020-106134
Bruynseels, K., Santoni de Sio, F., and Van den Hoven, J. (2018). Digital twins in health care: ethical implications of an emerging engineering paradigm. Front. Genet. 9, 31. doi: 10.3389/fgene.2018.00031
Buccelli, S., Tessari, F., Fanin, F., De Guglielmo, L., Capitta, G., Piezzo, C., et al. (2022). A gravity-compensated upper-limb exoskeleton for functional rehabilitation of the shoulder complex. Appl. Sci. 12, 3364. doi: 10.3390/app12073364
Burghardt, A., Szybicki, D., Gierlak, P., Kurc, K., Pietruś, P., and Cygan, R. (2020). Programming of industrial robots using virtual reality and digital twins. Appl. Sci. 10, 486. doi: 10.3390/app10020486
Burova, N. V., Molodkova, E. B., Nikolaenko, A. V., and Popazova, O. A. (2022). “Remote work as a societal incentive for creativity: phygital initiative for self-actualization,” in Technology, Innovation and Creativity in Digital Society. PCSF 2021. Lecture Notes in Networks and Systems, Vol. 345, eds D. Bylieva and A. Nordmann (Cham: Springer). doi: 10.1007/978-3-030-89708-6_15
Caputo, F., Greco, A., Fera, M., and Macchiaroli, R. (2019). Digital twins to enhance the integration of ergonomics in the workplace design. Int. J. Ind. Ergon. 71, 20–31. doi: 10.1016/j.ergon.2019.02.001
Cassioli, F., Fronda, G., and Balconi, M. (2021). Human–co-bot interaction and neuroergonomics: co-botic vs. robotic systems. Front. Robot. AI. 8, 659319. doi: 10.3389/frobt.2021.659319
Chaturvedi, S., Purohit, S., and Verma, M. (2021). Effective teaching practices for success during COVID 19 pandemic: towards phygital learning. Front. Educ. 6, 646557. doi: 10.3389/feduc.2021.646557
Chen, D., Ottikkutti, S., and Tahmasebi, K. N. (2022). “A mechatronics-twin framework based on Stewart platform for effective exploration of operational behaviors of prosthetic sockets with amputees,” in Paper Presented at the BIODEVICES-16th International Conference on Biomedical Electronics and Devices (Lisbon). Available online at: https://www.scitepress.org/Link.aspx?doi=10.5220/0010838600003123
Cheng, B., Fan, C., Fu, H., Huang, J., Chen, H., and Luo, X. (2022). Measuring and computing cognitive statuses of construction workers based on electroencephalogram: a critical review. IEEE Transac. Comput. Soc. Syst. 1–6. doi: 10.1109/TCSS.2022.3158585
Czwick, C., and Anderl, R. (2020). Cyber-physical twins-definition, conception and benefit. Procedia CIRP. 90, 584–588. doi: 10.1016/j.procir.2020.01.070
Farahani, F. V., Karwowski, W., and Lighthall, N. R. (2019). Application of graph theory for identifying connectivity patterns in human brain networks: a systematic review. Front. Neurosci. 13, 585. doi: 10.3389/fnins.2019.00585
Frederic, D., Waldemer, K., and Hasan, A. (2020). Brain at work and in everyday life as the next frontier: grand field challenges for neuroergonomics. Front. Neuroergonom. 1, 583733. doi: 10.3389/fnrgo.2020.583733
Frossard, L., and Lloyd, D. (2021). The future of bionic limbs. Res. Feat. 134, 74–77. doi: 10.26904/RF-134-7477
Fuller, A., Fan, Z., Day, C., and Barlow, C. (2020). Digital twin: enabling technologies, challenges and open research. IEEE Access. 8, 108952–108971. doi: 10.1109/ACCESS.2020.2998358
Gaggioli, A. (2017). Phygital spaces: when atoms meet bits. Cyberpsychol. Behav. Soc. Netw. 20, 774–774. doi: 10.1089/cyber.2017.29093.csi
Gaggioli, A. (2018). Digital twins: an emerging paradigm in cyberpsychology research? Cyberpsychol. Behav. Soc. Netw. 21, 468–469. doi: 10.1089/cyber.2018.29118.csi
Garro, F., Chiappalone, M., Buccelli, S., De Michieli, L., and Semprini, M. (2021). Neuromechanical biomarkers for robotic neurorehabilitation. Front. Neurorobot. 15, 742163. doi: 10.3389/fnbot.2021.742163
Gichane, M. M., Byiringiro, J. B., Chesang, A. K., Nyaga, P. M., Langat, R. K., Smajic, H., et al. (2020). Digital triplet approach for real-time monitoring and control of an elevator security system. Designs 4, 9. doi: 10.3390/designs4020009
Girletti, L., Groshev, M., Guimarães, C., Bernardos, C. J., and de la Oliva, A. (2020). “An intelligent edge-based digital twin for robotics,” in Paper Presented at the 2020 IEEE Globecom Workshops (GC Wkshps) (Taipei). Available online at: https://ieeexplore.ieee.org/document/9367549
Greco, A., Caterino, M., Fera, M., and Gerbino, S. (2020). Digital twin for monitoring ergonomics during manufacturing production. Appl. Sci. 10, 7758. doi: 10.3390/app10217758
Gregory, D. (2022). Healthcare Goes Digital: Designing for the Convergence of the Digital and Physical Environment—Implications for Design Professionals. Los Angeles, CA: SAGE Publications Sage CA.
Grieves, M., and Vickers, J. (2017). “Digital twin: mitigating unpredictable, undesirable emergent behavior in complex systems,” in Transdisciplinary Perspectives on Complex Systems (Springer), 85–113.
Haag, S., and Anderl, R. (2018). Digital twin–Proof of concept. Manuf. Lett. 15, 64–66. doi: 10.1016/j.mfglet.2018.02.006
Heun, V., Kasahara, S., and Maes, P. (2013). “Smarter objects: using AR technology to program physical objects and their interactions,” in Extended Abstracts on Human Factors in Computing Systems (New York, NY: Association for Computing Machinery), 961–966. doi: 10.1145/2468356.2468528
Jacob, R. J., Girouard, A., Hirshfield, L. M., Horn, M. S., Shaer, O., Solovey, E. T., et al. (2008). “Reality-based interaction: a framework for post-WIMP interfaces,” in Paper Presented at the Proceedings of the SIGCHI Conference on Human Factors in Computing Systems (Florence). Available online at: https://dl.acm.org/doi/abs/10.1145/1357054.1357089
Jones, D., Snider, C., Nassehi, A., Yon, J., and Hicks, B. (2020). Characterising the Digital Twin: a systematic literature review. CIRP J. Manuf. Sci. Technol. 29, 36–52. doi: 10.1016/j.cirpj.2020.02.002
Juarez, M. G., Botti, V. J., and Giret, A. S. (2021). Digital Twins: review and challenges. J. Comput. Inform. Sci. Eng. 21, 030802. doi: 10.1115/1.4050244
Kaur, M. J., Mishra, V. P., and Maheshwari, P. (2020). “The convergence of digital twin, IoT, and machine learning: transforming data into action,” in Digital Twin Technologies and Smart Cities (Cham: Springer), 3–17.
Khan, L. U., Saad, W., Niyato, D., Han, Z., and Hong, C. S. (2022). Digital-twin-enabled 6G: vision, architectural trends, and future directions. IEEE Commun. Mag. 60, 74–80. doi: 10.1109/MCOM.001.21143
Kuehner, K. J., Scheer, R., and Strassburger, S. (2021). Digital twin: finding common ground–a meta-review. Proc. CIRP. 104, 1227–1232. doi: 10.1016/j.procir.2021.11.206
Li, J., Li, Z., Chen, F., Bicchi, A., Sun, Y., and Fukuda, T. (2019). Combined sensing, cognition, learning, and control for developing future neuro-robotics systems: a survey. IEEE Trans. Cogn. Dev. Syst. 11, 148–161. doi: 10.1109/TCDS.2019.2897618
Lim, Y., Pongsakornsathien, N., Gardi, A., Sabatini, R., Kistan, T., Ezer, N., et al. (2021). Adaptive human-robot interactions for multiple unmanned aerial vehicles. Robotics 10, 12. doi: 10.3390/robotics10010012
Liu, Y., Ong, S., and Nee, A. (2022). State-of-the-art survey on digital twin implementations. Adv. Manuf. 10, 1–23. doi: 10.1007/s40436-021-00375-w
Lupetti, M. L., Piumatti, G., and Rossetto, F. (2015). “Phygital play HRI in a new gaming scenario,” in Paper Presented at the 2015 7th International Conference on Intelligent Technologies for Interactive Entertainment (INTETAIN) (Turin). Available online at: https://ieeexplore.ieee.org/abstract/document/7325480
Malik, A. A., and Bilberg, A. (2018). Digital twins of human robot collaboration in a production setting. Proc. Manuf. 17, 278–285. doi: 10.1016/j.promfg.2018.10.047
Mao, X., Li, W., Lei, C., Jin, J., Duan, F., and Chen, S. (2019). A brain–robot interaction system by fusing human and machine intelligence. IEEE Trans. Neural Syst. Rehabil. Eng. 27, 533–542. doi: 10.1109/TNSRE.2019.2897323
Maruyama, T., Ueshiba, T., Tada, M., Toda, H., Endo, Y., Domae, Y., et al. (2021). Digital Twin-driven human robot collaboration using a digital human. Sensors 21, 8266. doi: 10.3390/s21248266
Matulis, M., and Harvey, C. (2021). A robot arm digital twin utilising reinforcement learning. Comput. Graph. 95, 106–114. doi: 10.1016/j.cag.2021.01.011
Mehta, R. K., and Parasuraman, R. (2013). Neuroergonomics: a review of applications to physical and cognitive work. Front. Hum. Neurosci. 7, 889. doi: 10.3389/fnhum.2013.00889
Memar, A. H., and Esfahani, E. T. (2018). EEG correlates of motor control difficulty in physical human-robot interaction: a frequency domain analysis. Paper Presented at the 2018 IEEE Haptics Symposium (HAPTICS) (San Francisco, CA). Available online at: https://ieeexplore.ieee.org/abstract/document/8357181
Mikheev, A. A., Krasnov, A., Griffith, R., and Draganov, M. (2021). The interaction model within Phygital environment as an implementation of the open innovation concept. J Open Innov. Technol. Mark. Complex. 7, 114. doi: 10.3390/joitmc7020114
Mourtzis, D., Angelopoulos, J., Panopoulos, N., and Kardamakis, D. (2021). A Smart IoT platform for oncology patient diagnosis based on AI: towards the human digital twin. Proc. CIRP. 104, 1686–1691. doi: 10.1016/j.procir.2021.11.284
Nakazawa, J., and Tokuda, H. (2007). Phygital map: accessing digital multimedia from physical map. Paper Presented at the 21st International Conference on Advanced Information Networking and Applications Workshops (AINAW'07) (Ontario Falls, ON). Available online at: https://ieeexplore.ieee.org/abstract/document/4224132
Nyholm, S. (2021). Should a medical digital twin be viewed as an extension of the patient's body? J. Med. Ethics 47, 401–402. doi: 10.1136/medethics-2021-107448
Pairet, È., Ardón, P., Liu, X., Lopes, J., Hastie, H., and Lohan, K. S. (2019). A digital twin for human-robot interaction. Paper Presented at the 2019 14th ACM/IEEE International Conference on Human-Robot Interaction (HRI) (Daegu). Available online at: https://ieeexplore.ieee.org/abstract/document/8673015
Parasuraman, R. (2003). Neuroergonomics: research and practice. Theoret. Issues Ergon. Sci. 4, 5–20. doi: 10.1080/14639220210199753
Paul, G., Abele, N. D., and Kluth, K. (2021). A review and qualitative meta-analysis of digital human modeling and cyber-physical-systems in Ergonomics 4.0. IISE Trans. Occup. Ergon. Hum. Fact. 9, 111–123. doi: 10.1080/24725838.2021.1966130
Perno, M., Hvam, L., and Haug, A. (2020). Enablers and barriers to the implementation of digital twins in the process industry: a systematic literature review. Paper Presented at the 2020 IEEE International Conference on Industrial Engineering and Engineering Management (IEEM) (Singapore). Available online at: https://ieeexplore.ieee.org/abstract/document/9309745
Phanden, R. K., Sharma, P., and Dubey, A. (2021). A review on simulation in digital twin for aerospace, manufacturing and robotics. Mater. Today Proc. 38, 174–178. doi: 10.1016/j.matpr.2020.06.446
Pires, F., Cachada, A., Barbosa, J., Moreira, A. P., and Leitão, P. (2019). “Digital twin in industry 4.0: Technologies, applications and challenges,” in 2019 IEEE 17th International Conference on Industrial Informatics (IEEE), 721–726.
Pizzolato, C., Saxby, D. J., Palipana, D., Diamond, L. E., Barrett, R. S., Teng, Y. D., et al. (2019). Neuromusculoskeletal modeling-based prostheses for recovery after spinal cord injury. Front. Neurorobot. 13, 97. doi: 10.3389/fnbot.2019.00097
Prattico, F. G., and Lamberti, F. (2020). Mixed-reality robotic games: design guidelines for effective entertainment with consumer robots. IEEE Consumer Electr. Mag. 10, 6–16. doi: 10.1109/MCE.2020.2988578
Ramos, D., Gonçalves, G., Faria, R., and Sanches, M. P. (2021). “Building a drone operator digital twin using a brain-computer interface for emotion recognition,” in 2021 20th International Conference on Advanced Robotics (IEEE), 824–829.
Ravichandar, H., Polydoros, A. S., Chernova, S., and Billard, A. (2020). Recent advances in robot learning from demonstration. Ann. Rev. Control Robot. Auton. Syst. 3, 297–330. doi: 10.1146/annurev-control-100819-063206
Sarangi, S., Choudhury, S. B., Jain, P., Bhatt, P. V., Ramanath, S., Sharma, R., et al. (2018). Development and deployment of a scalable IoT Framework for digital farming applications. Paper Presented at the 2018 IEEE Global Humanitarian Technology Conference (GHTC) (San Jose, CA). Available online at: https://ieeexplore.ieee.org/abstract/document/8601742
Semeraro, C., Lezoche, M., Panetto, H., and Dassisti, M. (2021). Digital twin paradigm: a systematic literature review. Comput. Industry. 130, 103469. doi: 10.1016/j.compind.2021.103469
Shafto, M., Conroy, M., Doyle, R., Glaessgen, E., Kemp, C., LeMoigne, J., et al. (2012). Modeling, simulation, information technology and processing roadmap. Nat. Aeronaut. Space Administr. 32, 1–38.
Sharotry, A., Jimenez, J. A., Mediavilla, F. A. M., Wierschem, D., Koldenhoven, R. M., and Valles, D. (2022). Manufacturing operator ergonomics: a conceptual digital twin approach to detect biomechanical fatigue. IEEE Access 10, 12774–12791. doi: 10.1109/ACCESS.2022.3145984
Singh, M., Fuenmayor, E., Hinchy, E. P., Qiao, Y., Murray, N., and Devine, D. (2021). Digital twin: origin to future. Appl. Syst. Innov. 4, 36. doi: 10.3390/asi4020036
Tao, F., and Zhang, M. (2017). Digital twin shop-floor: a new shop-floor paradigm towards smart manufacturing. IEEE Access 5, 20418–20427. doi: 10.1109/ACCESS.2017.2756069
Tuli, T. B., Kohl, L., Chala, S. A., Manns, M., and Ansari, F. (2021). “Knowledge-based digital twin for predicting interactions in human-robot collaboration,” in 2021 26th IEEE International Conference on Emerging Technologies and Factory Automation (IEEE), 1–8.
Umeda, Y., Ota, J., Kojima, F., Saito, M., Matsuzawa, H., Sukekawa, T., et al. (2019). Development of an education program for digital manufacturing system engineers based on ‘Digital Triplet'concept. Proc. Manuf. 31, 363–369. doi: 10.1016/j.promfg.2019.03.057
Van der Valk, H., Haße, H., Möller, F., Arbter, M., Henning, J.-L., and Otto, B. (2020). A taxonomy of digital twins. Paper Presented at the AMCIS (Salt Lake City, UT). Available online at: https://aisel.aisnet.org/amcis2020/org_transformation_is/org_transformation_is/4/
Voigt, I., Inojosa, H., Dillenseger, A., Haase, R., Akgün, K., and Ziemssen, T. (2021). Digital twins for multiple sclerosis. Front. Immunol. 12, 1556. doi: 10.3389/fimmu.2021.669811
Volkov, I., Radchenko, G., and Tchernykh, A. (2021). Digital twins, internet of things and mobile medicine: a review of current platforms to support smart healthcare. Prog. Comp. Softw. 47, 578–590. doi: 10.1134/S0361768821080284
Wang, B., Zhou, H., Yang, G., Li, X., and Yang, H. (2022). Human Digital Twin (HDT) driven human-cyber-physical systems: key technologies and applications. Chin. J. Mech. Eng. 35, 1–6. doi: 10.1186/s10033-022-00680-w
Wang, D., Li, B., Song, B., Liu, Y., Muhammad, K., and Zhou, X. (2022). Dual-driven resource management for sustainable computing in the blockchain-supported digital twin IoT. IEEE Internet Things J. doi: 10.1109/JIOT.2022.3162714
Wright, J. M., Regele, O. B., Kourtis, L. C., Pszenny, S. M., Sirkar, R., Kovalchick, C., et al. (2017). Evolution of the digital biomarker ecosystem. Dig. Med. 3, 154. doi: 10.4103/digm.digm_35_17
Yi, C., Park, S., Yang, C., Jiang, F., Ding, Z., Zhu, J., et al. (2022). Muscular human cybertwin for internet of everything: a pilot study. IEEE Transac. Industr. Inform. doi: 10.1109/TII.2022.3153305
Yoon, K., Kim, S.-K., Jeong, S. P., and Choi, J.-H. (2021). Interfacing cyber and physical worlds: introduction to IEEE 2888 standards. Paper Presented at the 2021 IEEE International Conference on Intelligent Reality (ICIR) (Piscataway, NJ). Available online at: https://ieeexplore.ieee.org/abstract/document/9480910
Keywords: neuroergonomics, robotics, human-robot interaction, Digital Twin, Phygital Twin, human-machine interfaces, neurorobotics
Citation: Barresi G, Pacchierotti C, Laffranchi M and De Michieli L (2022) Beyond Digital Twins: Phygital Twins for Neuroergonomics in Human-Robot Interaction. Front. Neurorobot. 16:913605. doi: 10.3389/fnbot.2022.913605
Received: 05 April 2022; Accepted: 23 May 2022;
Published: 28 June 2022.
Edited by:
Hang Su, Fondazione Politecnico di Milano, ItalyReviewed by:
Mingchuan Zhou, Zhejiang University, ChinaXuanyi Zhou, Zhejiang University of Technology, China
Xinxing Chen, Southern University of Science and Technology, China
Copyright © 2022 Barresi, Pacchierotti, Laffranchi and De Michieli. This is an open-access article distributed under the terms of the Creative Commons Attribution License (CC BY). The use, distribution or reproduction in other forums is permitted, provided the original author(s) and the copyright owner(s) are credited and that the original publication in this journal is cited, in accordance with accepted academic practice. No use, distribution or reproduction is permitted which does not comply with these terms.
*Correspondence: Giacinto Barresi, Z2lhY2ludG8uYmFycmVzaUBpaXQuaXQ=