- 1Department of Human Centered Design, Faculty of Industrial Design Engineering, Delft University of Technology, Delft, Netherlands
- 2Center for Human Frontiers, Qualcomm Institute, University of California, San Diego, San Diego, CA, United States
- 3Department of Psychology, New York University, New York, NY, United States
- 4Department of Clinical Psychology, Vrije Universiteit Amsterdam, Amsterdam, Netherlands
- 5The Design Lab, California Institute of Information and Communication Technologies, University of California, San Diego, San Diego, CA, United States
- 6Centre for Cognitive and Brain Sciences, Indian Institute of Technology, Gandhinagar, India
- 7Intheon Labs, San Diego, CA, United States
- 8Department of Physics, Duquesne University, Pittsburgh, PA, United States
- 9Social Robotics, Institute of Neuroscience and Psychology, School of Computing Science, University of Glasgow, Glasgow, United Kingdom
- 10SOBA Lab, School of Psychology, Macquarie University, Sydney, NSW, Australia
Resonance, a powerful and pervasive phenomenon, appears to play a major role in human interactions. This article investigates the relationship between the physical mechanism of resonance and the human experience of resonance, and considers possibilities for enhancing the experience of resonance within human–robot interactions. We first introduce resonance as a widespread cultural and scientific metaphor. Then, we review the nature of “sympathetic resonance” as a physical mechanism. Following this introduction, the remainder of the article is organized in two parts. In part one, we review the role of resonance (including synchronization and rhythmic entrainment) in human cognition and social interactions. Then, in part two, we review resonance-related phenomena in robotics and artificial intelligence (AI). These two reviews serve as ground for the introduction of a design strategy and combinatorial design space for shaping resonant interactions with robots and AI. We conclude by posing hypotheses and research questions for future empirical studies and discuss a range of ethical and aesthetic issues associated with resonance in human–robot interactions.
Introduction
Resonance is a powerful physical mechanism that manifests in any physical system involving oscillations (Buchanan, 2019). Examples include the electromagnetic resonances that enable wireless communications, the acoustic resonances that give musical instruments their beauty, and the orbital resonances that shaped our solar system. No matter the medium, resonance produces amplification and synchronization effects in oscillatory systems. Details on the varying kinds of resonance are found in Box 1.
Box 1. A compilation of “resonance” terms from the scientific literature.
The following table outlines the breadth of the concept of resonance across three domains in the social and physical sciences. The examples given for each type of resonance (right column) are not meant to be an exhaustive reference list—instead, our intention is to include a few illustrative examples of each conception of resonance.
Most of the forms of resonance listed in the PHYSICS category appear to be based upon Helmholtz's (2009) idea of sympathetic resonance. For instance, in a review of magnetic resonance, “the term resonance implies that we are in tune with a natural frequency of the magnetic system” (Slichter, 2013). Yet a recent Royal Society review article (Vincent et al., 2021) makes the following claim: “the definition of resonance has been generalized [to include] all known processes leading to the enhancement, suppression or optimization of a system's response through the variation/perturbation/modulation of any system property.” This incredibly broad definition of resonance in physics suggests the challenge and need for a coherent understanding of this important concept across physics, neuroscience, the social sciences and design.
This article reviews the role of resonance in human systems, in AI and in human–robot interactions (HRI). Given the general appreciation of resonance in human interactions, we argue that designers can make use of the untapped potential of resonance to shape successful and desirable interactions in AI and HRI.
Resonance in Human Interactions: A Metaphor, a Mechanism or Both?
“Resonance” is a commonly-used term that describes the human experience of powerful, connecting and activating interactions (Duarte, 2013). For instance, we can “resonate” with a film or with a new friend. Metaphors related to resonance are also common, such as in the expressions “syncing up,” “getting on the same wavelength,” or even “feeling good vibes.”
Although the term “resonance” is often intended as a metaphor to describe an interaction, in many cases physical resonance may also be a mechanism underlying the interaction. For instance, people metaphorically “resonate with music” but the brain also physically resonates with music: the actual frequencies of sound and rhythm can be observed in the frequencies of electrical activity in the brain (Coffey et al., 2019, 2021; Kaneshiro et al., 2021; Pandey et al., 2021). Or, consider the common expression “syncing up” to describe a meeting. Even though “syncing” (that is, synchronization) is intended purely as a figure of speech, human communication does link to measurable “inter-brain synchrony” (Dumas et al., 2010; Dikker et al., 2019; Czeszumski et al., 2020; Kingsbury and Hong, 2020; Dumas and Fairhurst, 2021; Moreau and Dumas, 2021). This article aims to create a bridge between the human experience of resonance and resonance as a physical mechanism. In so far as resonance is more than a metaphor—if resonance is also a causal mechanism in human interactions—then this will have implications for measurement and design.
Some skepticism is justified in viewing resonance in human interactions as “real” rather than as just a metaphor. Historically, sympathetic resonance (sympathy: or sumpátheia) was viewed as the primary mechanism for magical phenomena. For instance, the neoplatonic philosopher Plotinus (205–270) wrote: “But how do magic spells work? By sympathy [sumpatheiai] and by the fact that there is a natural concord [sumphonian] of things that are alike [homoion] and opposition of things that are different.” (Lobis, 2015). Even in the modern era, there remains a widespread belief system that positive thinking or “thought vibrations” can bring about positive real-world occurrences through sympathetic resonance (Atkinson, 1906; Hicks and Hicks, 2006; Ehrenreich, 2009). Perhaps as a result of this association with magic, resonance was not always acceptable as a scientific explanation. A recent column in Nature Physics notes:
“…until the very late nineteenth century, scientists were reluctant to use the term ‘resonance' in connection with anything except acoustic phenomena, where it originated. Use of the word in other fields…always included some disclaimer that the link was “only by analogy”, despite the formal equivalence of the fundamental dynamical equations.” (Buchanan, 2019)
Now, the situation has changed: the term “resonance” is abundant in contemporary scientific literature (reviewed in Box 1). However, the term is often used ambiguously, where it is unclear whether “resonance” is being treated as a metaphor or as a physical mechanism. This ambiguity is present in the social sciences as well as in physics. Resonance in physics is an increasingly broad concept that refers to a range of phenomena. To bring clarity, we offer a glossary in Box 2 with proposed definitions for resonance and related terms, such as synchronization, entrainment, reverberation, etc.
Box 2. Glossary of terms.
Resonance, in this article, is treated as an umbrella term that involves physical resonance (either sympathetic or internal), synchronization, entrainment, memesis and attunement—as well as metaphorical resonance.
Sympathetic Resonance occurs when external, forced oscillations are aligned to a system's own natural oscillations and this results in amplification and synchronization. The amplification effect occurs when the natural frequencies of a system align with the frequencies of an external oscillator. Resonance also involves a synchronization or mirroring effect, as the phase and frequency of an external oscillator are reflected in the phase and frequency of the system's response.
Internal Resonance involves the activation of the natural frequencies (“eigen frequencies”) of a system. For instance, tapping a wine glass results in internal resonances.
Synchrony is a broad term that describes the temporal correlation of independent units of action. Correlations can occur in frequency independently from phase; for instance, heart rate synchrony can occur in people who have the same heart rate, even if their hearts do not beat at exactly the same time. Although all meanings of resonance should refer to a causal phenomena, synchrony can occur without a causal relationship (i.e., correlation does not imply causation).
Synchronization, in contrast to synchrony, should be understood as a complex dynamic and causal process—not a state. Pikovsky et al. (2001) carefully define synchronization as “an adjustment of rhythms of oscillating objects due to their weak interaction.” Further, synchronization requires “self-sustained oscillators,” like a powered metronome. Self-sustained oscillators are those that “oscillate with a distinctive waveform at a preferred amplitude that reflects a balance between energy inflow and dissipation.” (Strogatz, 2003). Synchronization also requires a sort of “weakness in coupling.” Weakness is important because a very strong coupling between systems simply results in immediate complete synchronization. The synchronization of two oscillators does not require that the two have the same phase at the same time; for instance, two clock pendulums can be synchronized but swing in anti-phase. When an external oscillation frequency is nearly aligned to a natural frequency of a powered oscillatory system, the systems will “phase lock” together and synchronize. Synchronization can occur at “a rational fraction of the resonance frequency,” like 2:3 or 2:1 (Shim et al., 2007).
Entrainment occurs when a consistent rhythmic pulse of one oscillator shifts the frequency of another self-sustained oscillator. For instance, a drummer's beat can entrain the motion of rowers or entrain dancers to a common rhythm. Like synchronization, entrainment requires weakly-coupled and self-sustained (powered) oscillators (Pikovsky et al., 2001). In fact, the two terms are nearly identical; at least one author (Izhikevich, 2007) claims that entrainment is limited to 1:1 synchronization. According to Helfrich et al. (2019), true entrainment requires that “an ongoing oscillator is entrained by a rhythmic input at a slightly different frequency. The entrained oscillation becomes phase-locked and the amplitude increases. After the entraining stream stops the oscillator exhibits a reverberation at the driving frequency for several cycles.” Some definitions of entrainment require that an external oscillator unidirectionally influences a powered oscillator (Lakatos et al., 2019) but other definitions allow for mutual entrainment, “whereby two rhythmic processes interact with each other in such a way that they adjust toward and eventually ‘lock in' to a common phase and/or periodicity” (Clayton et al., 2005). In this article, entrainment is treated as the mechanism for synchronization (synchronization through entrainment) and both terms are treated as types of resonance (see Box 3).
Reverberation can be defined by the reverberation time, which is the time required for an oscillation to “fade away” once the external input has stopped. After an impulse, a system's natural or resonance frequencies tend to continue to reverberate, as in a tapped wine glass or echoes in a cathedral.
Coherence refers to the statistical similarity between two or more oscillating systems (Wolf, 2010).
Mimesis, or imitation, describes the intentional or non-intentional replication of movement patterns. These replications do not need to be synchronous. For instance, a child sticking out their tongue and another child copying them. This can be viewed as a type of resonance enabled by memory.
• Behavioral Mimicry occurs when people behave in similar or identical ways within a short period of time. (Mayo and Gordon, 2020); i.e., “the replication of automated behaviors”
• Imitation can be described as “a short sequence of actions that I see my interaction partner performing and then consequently replicate…imitation is not mere mirroring in the sense that one copies every little part of another's movement. It is rather the replication of the action with regard to the outcome of the action which leads to the acquisition of new skills” (Lorenz et al., 2016).
Behavioral synchronization describes behaviors that are synchronous in time, but potentially complementary (e.g., turn-taking) (Chartrand and Van Baaren, 2009).
Physiological Synchrony or Biobehavioral synchrony involves the rhythmic and temporal correlation of breath rate, heart rate, hormone production, or interbrain synchrony (Feldman, 2017; Mayo and Gordon, 2020)
Psychological Attunement has been defined as “Entrained rhythms [that] constitute a form of dynamic equilibrium in which partners vary their behaviors over time while keeping this variation within desired limits” (Sadler et al., 2009).
The Vibe (e.g., “good vibes” or “vibing with”) is a pervasive cultural construct used to describe how people perceive the shared affective experience and aesthetic expectations of a group, a place, a product, a brand, a robot, etc. The vibe is different from a person's individual affective reaction, as it describes the aspects of conscious experience that are perceived to be shared between people (Witek, 2019). Hypothetically, the vibe emerges from interpersonal resonance effects.
Harmony is an ancient concept (Lomas et al., 2022) that has an intrinsic relationship with resonance: the harmonic tunings of stringed instruments maximize acoustic resonance between the tuned strings. For example, musical notes with consonant intervals (such as the 2:3 ratio of a musical fifth) will share common acoustic harmonics, while dissonant intervals do not. These shared harmonics produce physical resonances between tuned strings—and perhaps resonances between neural oscillators, as well.
Box 3. Clarifying the relationship between resonance, entrainment and synchronization.
This article treats resonance as an umbrella concept that includes both metaphorical resonance and resonance as a physical mechanism. As resonance has an expansive meaning in physics (Box 1), we first distinguish between typical resonance (which involves the natural frequencies of a system) and atypical resonance (which does not involve natural frequencies). Then, typical resonance includes internal resonance (the activation of reverberating natural frequencies) and sympathetic resonance (alignment between the frequencies of external oscillations and the natural frequencies of a system). Sympathetic resonance can be further divided into passive resonance and active resonance. Passive resonance occurs with unpowered oscillators (like a wine glass) while active resonance occurs with powered or self-sustained oscillators (like battery-powered metronomes). Active resonance includes the phenomenon of entrainment, which occurs when a self-sustained oscillator synchronizes its phase and frequency to a weakly coupled external oscillation.
The natural or resonance frequency of an oscillator can be considered its preferred frequency. Powered oscillators, like metronomes, also have a preferred amplitude of oscillation—this is not the case for passive oscillators, like wine glasses. When external frequencies align with the preferred frequencies of a wine glass, the most noticeable aspect is the amplification of the amplitude of the oscillation. In contrast, when external oscillations align with the natural frequencies of a powered oscillator, the most noticeable aspect is the synchronization. However, the synchronization of frequency and phase also occurs between a wine glass and an external speaker while amplification also occurs with synchronized metronomes. For this reason, we describe entrainment and synchronization as types of sympathetic resonance; namely, the type involving a self-sustained oscillator.
The scientific relationship between entrainment and resonance is often a point of confusion due to the lack of clear definitions (Helfrich et al., 2019). Our view diverges from other descriptions of resonance that are limited to passive, unpowered systems (Pikovsky et al., 2001; Guevara Erra et al., 2017; Lakatos et al., 2019). Our view is that the concept of resonance can easily accommodate active forms as well as passive forms, as both involve preferred frequencies of oscillation (natural frequencies), synchronization effects and amplification effects. Given the ubiquity of resonance in oscillatory systems—and its already expansive definition in physics (Box 1)—why should resonance only refer to unpowered systems and thus exclude dissipative systems, like the brain? Rather than treating “resonance” in interpersonal interactions as a complete misnomer, we make the case that it is appropriate and physically accurate to say that we resonate with people, films or other media. We hope that this view opens the door to a more comprehensible and coherent scientific study of resonances in human interactions.
Types of Resonance
• Metaphorical Resonance
• Physical Resonance
∘ Atypical Resonance: Does not involve natural frequencies (see Vincent et al., 2021)
∘ Typical Resonance: Involves natural frequencies
■ Internal Resonance (involves the activation and reverberation of natural frequencies within a stimulated system, like the reverberations of a tapped wine glass)
■ Sympathetic Resonance (involves the alignment of external frequencies with the natural frequencies of a system—when the forced frequencies match the natural frequencies)
• Passive Resonance: unpowered and externally sustained; like a wine glass vibrating in synchrony with external oscillations.
• Active Resonance: powered and self-sustained, like a metronome synchronizing with external oscillations. This encompasses different types of synchronization:
∘ Complete Synchronization (due to strong coupling)
∘ Entrainment (Phase Synchronization)
■ In-phase
■ Anti-phase
■ Phase shifted
∘ Frequency Synchronization
∘ Envelope Synchronization
∘ Partial and Asynchronous Synchronization (e.g., mimesis)
Resonance as a Physical Mechanism
To provide grounding for resonance in human dynamics, this section outlines physical resonance as a causal mechanism in acoustics. Though we focus on sound, it is important to note that resonance operates in all oscillating systems, regardless of medium. This universality results from the fact that resonance is a mathematical property—it is the natural result of the alignment of phases in oscillating systems.
A wine glass offers an excellent example of the physics of resonance. First, if a glass is gently tapped with a spoon, there will be a reverberating sound that reflects the natural frequencies of oscillations in the wine glass. These natural frequencies, which are inherent to the structure of the glass, are also known as characteristic frequencies or eigen frequencies (“eigen” is German for “own” or “inherent”). These natural frequencies are also the resonance frequencies of the glass: when external, forced oscillations match these natural frequencies, resonance occurs. But, while tapping the glass with a spoon may reveal the resonant frequencies of the glass, the wine glass is not in resonance with the spoon.
Sympathetic resonance occurs when external, forced oscillations are aligned to a system's own natural oscillations. If a loudspeaker plays the resonant frequencies of a wine glass, the glass will begin to oscillate at much greater amplitudes than if the speaker played other, non-resonant frequencies. Now the glass is in resonance with the speaker. This effect manifests in other common acoustic systems, as well. When one tuning fork is struck near another identical fork, they will both begin to oscillate together, having been coupled together in synchrony via the acoustic vibrations. Similarly, two strings tuned to the same note will move one another in synchrony through sympathetic resonance (Figure 1).
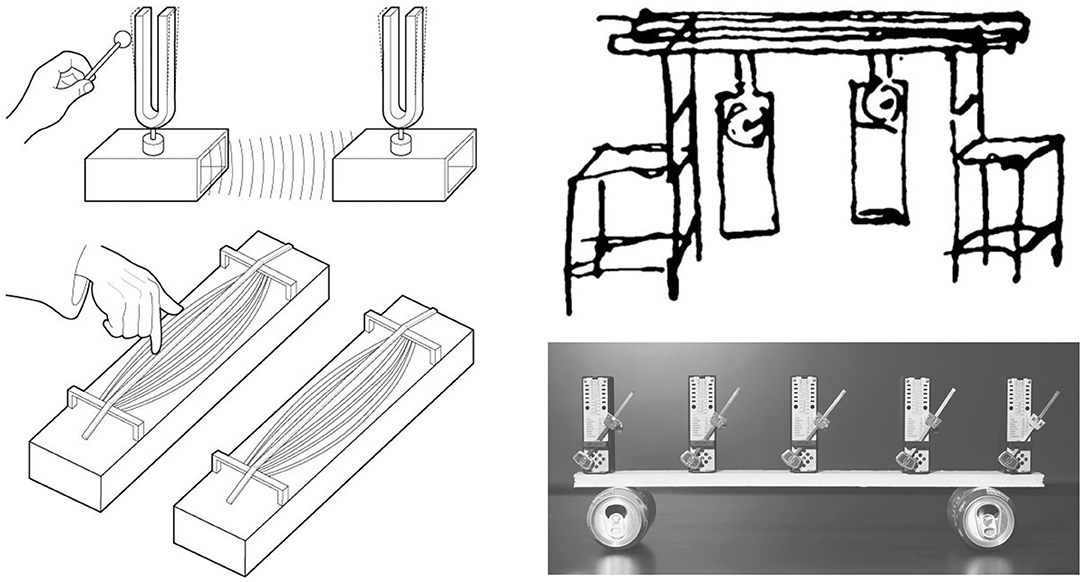
Figure 1. (Left) Resonance between mutually attuned tuning forks and strings involves synchronization and amplification. (Right) Sketch by Christiaan Huygens (b. 1629), who discovered “the sympathy of two clocks.” When two clocks are placed on a common beam, their two pendulums will eventually synchronize. Right bottom: a set of weakly coupled powered metronomes (self-sustained oscillators) will eventually synchronize. Photo courtesy: Harvard Natural Sciences Lecture Demonstrations.
The relationship between resonance, synchrony and amplification was articulated by German nineteenth century scientist Hermann Helmholtz. His book “On the Sensations of Tone as a Physiological Basis for the Theory of Music” (Helmholtz, 2009; originally published 1863) offers the first scientific exposition of sympathetic resonance in acoustics. His primary illustration of sympathetic resonance involves the resonance between a church bell and its bellringer. If the bellringer provides consistent pulls at a frequency that aligns to the bell's natural rate of swinging, then the swinging will be rapidly amplified. Importantly, the sympathetic resonance occurs when phases of oscillation align: that is, when the downward pull of the bellringer matches up with the downward motion of the bell's swing. The role of synchrony in sympathetic resonance is easier to observe with a slow bell ringer than with the rapid oscillations of a wine glass. Yet, even the sympathetic, synchronized oscillations of a wine glass can be made visible with high frequency camera equipment (Slow Mo Guys, 2021).
Synchrony between systems does not necessarily imply sympathetic resonance. Two systems might be synchronized with each other due to a third system, for instance, or for other non-causal reasons (Hasson and Frith, 2016). Other forms of resonance only occur with powered oscillators (like the clocks and metronomes in Figure 1), namely entrainment and synchronization. These terms—which explain phenomena like the synchronization of fireflies or the entrainment of dancers to a musical beat— are defined and discussed in Box 3. In this article, we treat these two terms as subsets of sympathetic resonance (by analogy, like squares are subsets of rectangles).
Resonance as a Metaphor
Having briefly considered the operation of resonance as a physical mechanism, we now wish to bring clarity to the metaphorical use of resonance in science and broader culture.
A recent review of the word “resonance” in the language of scientific literature (Ruthven, 2021) reveals that resonance typically serves as an implicit metaphor to indicate 1. agreement (e.g., new evidence can resonate with an existing theory), 2. arousal (e.g., a film that resonates is engaging and moving) or 3. action (e.g., the resonance of a speech can motivate people to take action). But, despite a vast number of scientific articles that use resonance as a term, it is only very rarely defined. The lack of definition suggests that “resonance,” as a term, is easily and broadly understood intuitively as a metaphor.
Metaphors are useful when they enable concrete, familiar experiences to communicate abstract, conceptual meanings (Lakoff and Johnson, 1980; Yang, 2014). A metaphor involves the pairing or alignment of concepts between a concrete source and a more abstract target; this coupling of concepts produces mappings that allow multiple concepts to be integrated together into an emergent space of meaning (Holyoak and Stamenković, 2018). An example set of metaphorical mappings use the metaphor “Love is a Journey”: for instance, in a journey (the source) there are travelers while in love (the target) there are lovers. In Table 1, we provide a similar set of explicit mappings between the metaphor of acoustic resonance and resonant human interactions.
With this introduction to resonance established, we are now posed to explore the alignment between the metaphorical experience of resonance (as in a film that resonates) and the physical phenomena of resonance itself. The next section of the article considers the research basis for understanding resonance in human interactions.
Part 1: Resonance in Human Interactions
A common example of physical resonance in human interactions can be found on nearly any playground. When pushing someone on a swing, the pusher needs to coordinate the timing of their pushes to the swing's natural back-and-forth oscillation (determined primarily by the length of the swing). Does pushing at a faster rate help? No: if the pusher simply pushed more times per second, most of the pushes would do nothing because they would not line up with the movement of the swing. When a pusher aligns their timing to the natural frequency of the swing, they amplify the effects of their effort: many small, well-timed pushes are enough to get the swinger high into the air.
Beyond this simple example, where else might resonance occur in human interactions? To scope our search, we assume that sympathetic resonance can only occur when external oscillations and natural oscillations align. Therefore, physical resonances in human systems should only be present during human activities that have a natural frequency or rhythm of oscillation.
Consider an everyday rhythmic human activity: walking. Researchers have used accelerometers to determine the dominant and natural up-and-down frequency of walking. The typical frequency of naturalistic walking is about 2 Hz, or two steps per second (MacDougall and Moore, 2005). This natural oscillatory frequency can vary—some people walk faster or slower than others. However, across a diverse set of participants, the researchers found that the tempo of walking was not dependent upon height, weight, or other physical factors. In fact, the researchers suggest that the 2 Hz natural tempo is the result of genetically encoded “central pattern generators” in the spinal cord, as these are the basis of the tempo of locomotion in other animals (Guertin, 2013).
This is not just trivia: structural engineers need to take into account this 2 Hz human walking pace in every footbridge that is built. Famously, a 2 Hz resonance frequency caused the UK's Millenium Bridge to dramatically sway side-to-side when it was loaded with pedestrians (Dallard et al., 2001; Strogatz et al., 2005). On opening day, the bridge became so crowded that most people could not easily walk forward—instead people were so packed-in that they had to walk in a sort of side-to-side waddle. Unfortunately, the bridge had a natural side-to-side 2 Hz resonant frequency. As the bridge started to sway back and forth, this led to the synchronization of the waddling motion of the thousands of pedestrians. Without deliberate coordination, people stepped left and stepped right in synchrony with each other, entrained to the swaying motion of the bridge. As a result, the 2 Hz side-to-side oscillation of the bridge was further amplified to dangerous levels.
Given that 2 Hz is a natural frequency of human movement, the theory of resonance predicts that 2 Hz should also be a resonance frequency. That is, external rhythmic inputs at about 2 Hz should cause a synchronization and amplification of human movement. Is 2 Hz actually a resonance frequency of movement? One way to test this prediction is to consider the popularity of music at different beats per minute (BPM), where 120 BPM would be equivalent to 2 Hz. Figure 2 presents a histogram showing the relative distribution of BPM in the Top 50 songs for each year of the past decade, worldwide. This reveals a distinct peak at 120 beats per minute.
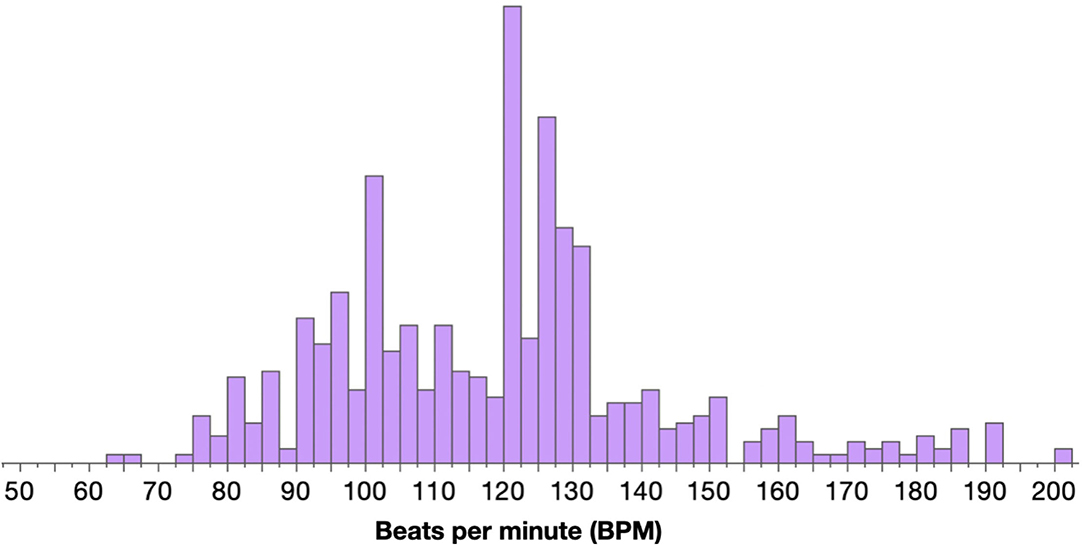
Figure 2. The above histogram is compiled from a Kaggle dataset containing the “Top 50” Billboard songs from 2010 to 2019. It appears to be a resonance curve showing maximum excitement at a preferred frequency of oscillation. However, note the sharp dropoff from 120 BPM to 118 BPM—this is not expected in a resonance curve, as the frequencies close to resonance tend to resonate strongly as well. There may simply be very few songs released with this BPM. But, while this graph might not be a resonance curve, it may be a result of meaningful resonance effects in the brain—an increase in amplitude due to the alignment of external frequencies with natural frequencies https://www.kaggle.com/leonardopena/top-spotify-songs-from-20102019-by-year.
Is this a resonance effect? The graph in Figure 2 resembles a resonance curve: given a range of input frequencies, there is a selective amplification at the same frequencies as the natural frequencies of the stimulated system (i.e., the 2 Hz natural pace of movement in humans). However, the peak may arise for a variety of reasons, from the musicians' recognition of the popularity of 120 BPM to the listeners' increased familiarity with 120 BPM songs. Most importantly, there are many more songs released at 120 BPM. So, even though it is not entirely appropriate to refer to the graph as a resonance curve, it may still be a function of resonance effects (e.g., a greater likelihood of rhythmic entrainment to walking frequencies at 120 BPM).
In this article, we propose that physical resonance can be distinguished from purely metaphorical resonance when the mathematics of resonance (as used to characterize other physical systems) can be used to model human interactions. Synchronization, amplification and signal alignment are the mathematical hallmarks of resonance—therefore, physical resonance in human systems should occur when the oscillations of external signals match natural human oscillations and this results in synchronization and increased energy (amplification). This viewpoint treats the physics of human resonance in a similar fashion as other physical systems yet it leaves room for future research to further clarify the relationships involved.
Rhythmic Human Interactions
The previous example shows how rhythmic human activity can be investigated as a context for physical resonance phenomena. Rhythmic human interactions clearly occur in artistic domains, such as music-making (Clayton et al., 2005), dance (Larsson et al., 2019) and various kinds of cultural rituals like chanting (Gelfand et al., 2020). Rhythmic interactions are also common in everyday life, as in the case of walking, conversational turn-taking (McGarva and Warner, 2003; Wilson and Wilson, 2005; Lee et al., 2010), patterns of eye contact (Wohltjen and Wheatley, 2021) or with interactions like handshakes (Melnyk and Hénaff, 2019). More intimate rhythmic interactions occur during human sexual behavior (Safron, 2016). Leading up to the moment of birth, midwives often advise expectant mothers to push in phase synchrony with their own rhythmic uterine contractions (Hanson, 2009). Researchers have also observed that the earliest interactions between parent and child are strongly rhythmic (Stern et al., 1985). Babies cry in a rhythmic manner and caregivers soothe them with synchronized motions (Trehub and Trainor, 1998). Synchrony in caregiving appears to literally “tune” the human social brain (Yaniv et al., 2021).
“This resonance or echoing of affect, feelings, and emotions that takes place in the reciprocal interaction between infants and their caretakers is a necessary element for the development of empathy and advanced social cognition.” (Decety and Meyer, 2008)
Neurobiological Rhythms and Human Nature
What makes human behavior so rhythmic? Human rhythms are believed to emerge from a broad range of biological oscillators that are present across the brain and the rest of the body (Varga and Heck, 2017). For instance, rhythmic central pattern generators in the spinal cord not only drive locomotion (Ijspeert, 2008; Guertin, 2013), but also drive heart rhythms (Bucher et al., 2015) and breath rhythms (Molkov et al., 2014). Furthermore, body and brain oscillations appear to integrate together in a hierarchical architecture (Klimesch, 2018).
Rhythmic—and resonant—phenomena are found in the brain at multiple levels, from neurons to circuits to brain waves (Buzsaki, 2006). Individual neurons have natural intrinsic oscillatory periods (Lampl and Yarom, 1997); neurons can be tuned to respond to different input frequencies through resonance, like “strings on a violin” (Das et al., 2017). At the level of neural circuits, the reverberation of recurrent activity in neural ensembles plays a key role in memory (Wang, 2001; Tegnér et al., 2002; Han et al., 2008), as originally predicted by neuroscientist D.O. Hebb in 1949 (Hebb, 2005). Finally, large-scale electrical oscillations in the brain, or brainwaves, demonstrate clear resonance effects (Herrmann, 2001) that are observable through electroencephalography (EEG). Further to this, neurobiological processes associated with adaptive learning (Grossberg, 2013, 2017), perceptual learning (Raja, 2020), and ecological cognitive architecture more generally (Raja, 2018), have all been theorized as forms of physical resonance.
Neurons are, technically speaking, non-linear oscillators (Izhikevich, 2007; Stiefel and Ermentrout, 2016)—therefore, it is not so surprising that large collections of neurons exhibit both internal resonance effects (one part of the brain resonating to another) and external resonance effects (the brain resonating to environmental phenomena). Many scientists believe that physical resonance in the brain plays a major role in music perception, such as the physicist and neuroscientist Ed Large, who claims:
“The brain does not ‘solve' problems of missing fundamentals, it does not ‘compute' keys of melodic sequences, and it does not “infer” meters of rhythmic input. Rather, it resonates to music… certain aspects of this process can be described with concepts that are already well-developed in neurodynamics, including oscillation of neural populations, rhythmic bursting, and neural synchrony.” (Large, 2010)
Some researchers have recently proposed a unified account of rhythmic synchronization and entrainment in the brain (Lakatos et al., 2019); other researchers have proposed a unified account of the biological, neurological and physical mechanisms involved in the “rhythmic entrainment of biological systems” (Damm et al., 2020). Rhythmic entrainment has been found to govern patterns of interaction at a social, population, and even species level—where, in the latter case, the entrainment of natural oscillations can be observed at the scale of economies and ecosystems (Greenfield et al., 2021). In short, it would appear that resonance effects can operate all the way up and all the way down: from neurons to economies.
Entrainment and Rhythmic Synchronization
Human interactions can naturally synchronize through the process of entrainment (Boxes 2, 3), which is akin to the natural synchronization of metronomes (Figure 1). Social neuroscientist Ruth Feldman (2012, 2017) argues that biobehavioral synchrony (in behavior, heart rate, endocrine production and brainwaves) serves as a key principle underlying parental love, romantic love, friendship and human attachments. Indeed, when loving human partners interact, their rhythmic communication produces measurable physical synchronization in behavior (Grafsgaard et al., 2018), in heart rate (Prochazkova et al., 2022) and in the brain (Kinreich et al., 2017).
Some argue that the ability to synchronize to a beat is one of the core skills associated with human social behavior. Kirschner and Tomasello (2009) found that children 2–4 years old could adjust their natural drumming tempo to match another beat—but that their accuracy in synchronizing was significantly higher when they drummed with a human partner (as opposed to drumming along with a machine or drumming along with a drum sound produced by a speaker). The authors argue that “drumming together with a social partner creates a shared representation of the joint action task and/or elicits a specific human motivation to synchronize movements during joint rhythmic activity.”
Humans are typically much less able to synchronize to rhythms of visual flashes than to rhythms of auditory tones (see review by Repp and Su, 2013). But, rhythmic entrainment and synchronization is not specific to music or auditory experiences. With certain forms of visual stimuli (i.e., bouncing balls), visual synchronization becomes nearly as accurate as auditory synchronization (Iversen et al., 2015). Researchers have also found that deaf individuals exhibit enhanced synchronization to visual rhythms, suggesting that the ability to attune to rhythms is at least partially based on experience and not just a result of biological coupling between the auditory and motor system (Iversen et al., 2015). Researchers have found that humans can synchronize to tactile pulses on their back with higher accuracy when feeling the vibrations played over their entire back rather than at just a small portion; similarly, rhythms that engage multiple sensory modalities also produce more accurate synchronization (Ammirante et al., 2016). This suggests that overall sensory immersion and attentional engagement affects the propensity to synchronize with rhythms.
Synchronization helps support coordinated actions between individuals. Meta analyses (Morgan et al., 2017) have shown that behavioral synchrony in groups increases prosocial behavior, increases perceived social bonding, and generally feels good (as measured as increases in positive affect). Why might behavioral synchrony feel good? Cracco et al. (2021) claim that “synchrony is aesthetically pleasing and a signal of group cohesion, as stimuli that are processed more fluently are known to produce a hedonic response.”
Rhythmic synchronization is very rare in the animal kingdom, at a social level, apart from special examples (see extended discussion in Box 4). The human capacity for rhythmic synchronization may have coevolved in human cultures because it enhanced social bonding between sexual partners, between parents and children, and within larger social groups. Savage et al. (2021) state:
“‘Neural resonance' (synchronous brain activity across individuals) facilitates social bonding through shared experience, joint intentionality, and ‘self-other merging'. Through the production of oxytocin and endogenous opioids, neural resonance also facilitates prosociality.”
Box 4. Resonance in non-human animals.
Despite the relative utility of resonant phenomena in humans (such as social synchronization and entrainment), it is rare in the animal kingdom. There are examples of animals synchronizing with other members of their species: for instance, chirping insects, croaking frogs, claw-waving crabs, and flashing bioluminescent animals, like fireflies (Wilson and Cook, 2016). However, these examples seem to involve somewhat involuntary neurological connections in fairly simple animals. Why is entrainment not more common? While this is not well-understood at present, Wilson and Cook (2016) provide three criteria for rhythmic entrainment: 1. an animal needs to have the mechanical ability to move with the beat (i.e., the tempo should be similar to an animal's natural tempo of movement), 2. the animal must be able to extract the beat from the sensory environment and pay attention to it, and, crucially, 3. the animal must have the motivation to voluntarily move in union.
Until very recently, it was believed that only human beings could synchronize to an external rhythm, like a musical beat. It was only with the advent of YouTube that researchers first discovered Snowball the Dancing Cockatoo (Patel et al., 2009; Patel and Iversen, 2014) and 33 other examples of animals that appeared to show entrainment to music (Schachner et al., 2009). These examples spanned 14 different bird species—and an Asian Elephant. This led to the belief that only species that had previously evolved the capacity for vocal mimicy could entrain to a beat. Schachner et al. (2009) noted that, despite a large number of “dancing” dog videos, none demonstrated the ability to synchronize with music (even though some dogs had been trained for years to compete in dance competitions).
Some animals can be reliably trained to synchronize to a rhythm. In 2011, researchers demonstrated that Budgerigars, a parrot-like bird, could learn to produce rhythmic beak tapping patterns that synchronized to an audio-visual metronome (Hasegawa et al., 2011). Then researchers managed to train Ronan, a Sea Lion (Cook et al., 2013), to entrain to a beat—this was surprising because Sea Lions are not vocal learners.
Over the past decade, there has been much investigation of the capacity for non-human primates to entrain to a musical beat. Sounds can induce spontaneous rhythmic swaying in chimpanzees (Hattori and Tomonaga, 2020)—however, this swaying effect occurs in response to randomized rhythms and when sounds are rhythmic (Bertolo et al., 2021). Monkeys have been trained to tap in response to an auditory or visual metronome, however, their movements are always reactive: they always tap following the stimuli (although much faster than they can in a single reaction time experiment; see Wilson and Cook, 2016). In contrast, when humans entrain to a similar metronome, they typically tap slightly before each stimulus in the beat. In just one case, researchers have trained monkeys to make predictive, synchronized eye movements to a visual metronome—however, the monkeys had to be rewarded for each trial (Takeya et al., 2017). Based on this evidence, the authors suggest that monkeys and other animals may have the capacity for “predictive and tempo-flexible synchronization to a beat” but might not be “intrinsically motivated” to synchronize!
In summary, it is surprising that so few animals—neither dogs nor monkeys—are predisposed to entrain to a beat. After all, even animal neurons have the capability to entrain to periodic rhythms. Why, then, are animals generally so unable—or unwilling— to entrain to a beat? One possibility: consider that the heart is entrained to rhythms produced by central pattern generators in the spinal cord; clearly, animals need to protect their heartbeat from becoming entrained to external stimuli. It may be that, even in the simplest of animals, there is a need to evolve defense mechanisms that can protect against unwanted resonance effects. Part of the human capacity for rhythmic entrainment may result from the ability to “let one's guard down” in order to open up to certain kinds of external rhythmic entrainment with other people. This would suggest that humans only resonate to external stimuli when they feel safe to do so; after all, stress may make it difficult to dance or to be moved by music. This also suggests that animals may be able to resonate, if they could be emotionally or biochemically prepared to do so. This opens up possibilities for animal-robot and animal-AI interactions that can be explored in the future.
Hyperscanning and Inter-brain Synchrony
The scientific understanding of rhythmic entrainment and neural resonance is a fast-moving area of neuroscience that is being propelled by new hyperscanning methods that scan the brains of multiple interacting participants simultaneously. Interpersonal neural synchrony at the group and dyadic level has been shown to be associated with a number of predictors, including shared stimulus features, joint actions, personality traits, social intentionality, relationship quality, and cooperation (see, e.g., Czeszumski et al., 2020 for review).
For example, recent work from co-authors of this article investigated the relationship between inter-brain synchrony and group dynamics and found that EEG inter-brain synchrony predicted collective performance among teams better than self-report (Reinero et al., 2021). In another line of work, group-based inter-brain coherence predicted class engagement and social dynamics in groups of high school students during their real-world lessons (Dikker et al., 2017; Bevilacqua et al., 2019). Social closeness with the teacher also correlated with brain-to-brain synchrony—that is, enhanced synchrony was found with students who reported greater engagement with the teacher. Finally, and perhaps most directly related to the concept of resonance: Brainwaves of students who engaged in face-to-face interactions before class were more synchronized during class, even if students were no longer interacting. This finding raises interesting questions about the role of resonance in the directionality of the relationship between human face-to-face interaction, inter-brain synchrony, and social connectedness.
The Downside of Being in Sync: Chained to the Rhythm?
Humans may be predisposed to synchronize with each other, but this does not always lead to positive outcomes. Synchronization also has some important tradeoffs; Gelfand et al. (2020) claim that synchrony can produce conformity, destructive obedience, groupthink, antisocial aggression and also impair group creativity. They point to findings (Wiltermuth, 2012a,b) that people who have been randomly assigned to a synchronous activity are more likely to comply with an anti-social order (e.g., irritating a stranger) and to follow a morally compromised command (in the study, participants were asked to grind up live bugs). Synchrony also increases the likelihood that people will engage in conformity, like copying majority opinions rather than following their personal preferences (Dong et al., 2015). Further, sometimes synchrony is simply “situationally inappropriate;” in a study of a complex verbal coordination, groups that were randomly assigned to a synchronization task performed worse, reported higher levels of conflict and reduced group cohesion (Wood et al., 2018).
Gelfand et al. (2020) randomly assigned participants to march synchronously around a college campus or at their own pace. The participants who synchronized showed reduced creativity when writing stories. They also found that synchronous marching discouraged the development and sharing of minority perspectives during decision-making. They relate this finding about synchrony to the need to balance “tightness” and “looseness” in culture.
The ability to flexibly move in and out of synchrony appears to be critical to adaptive flexibility. Mayo and Gordon (2020) claim that “two tendencies exist simultaneously, one to synchronize with others and another to move out of synchrony and act independently. We suggest that an adaptive interpersonal system is a flexible one, able to continuously adjust itself to the social context.”
Savage et al. (2021) point out the key difference between rhythmic integration and pure synchronization: rhythm is predictable but also flexible to accommodate diverse individual contributions. This is because rhythm involves two essential components: 1. equally timed beats (isochronicity) and 2. a hierarchical structure (meter). “While synchronization solely to the beat (e.g., in marching or unison chanting) allows large groups to integrate, it tends to submerge individual contributions. Meter solves this problem by allowing many individuals to contribute, out of phase, to the same integrated rhythm.” Social rhythms (of speech, music, dance, etc.) can thus support diversely coordinated actions within a loosely unified structure.
Origins of Empathy: Sympathetic Resonance
Sympathetic resonance—including synchronization and rhythmic entrainment—appears to have been a key factor in human evolution (Savage et al., 2021; Lin and Lomas, 2022). Resonance relates in a fundamental way to the human capacity to feel what another person feels, which is often called empathy. But, before the term “empathy” was coined in the twentieth century, the ability to feel what others feel was referred to as “sympathy”—as in sympathetic resonance. The eighteenth century philosopher Adam Smith wrote his first book, “The Theory of Moral Sentiments” (Smith, 1759), with the general thesis that “sympathy” accounts for a large portion of moral behavior. Specifically, he explained that people like to help other people because they sympathetically feel good when other people feel good and sympathetically feel bad when other people feel bad (Schliesser, 2015).
Later, the nineteenth century German psychologist Theodore Lipps used the German term Einfühlung to describe how people “feel into” the states of other people and even art pieces. By using an inner imitation or simulation, people seem to be able to fuse with artworks or persons through a process of “Psychische Resonanz” (Lipps, 1891). For instance, watching a tightrope walker produces a resonance with internal associated feelings like vertigo. The representation of the performer in one's own mind allows one to feel how oneself would feel in the same situation. The psychologist Edward Titchener reviewed Lipps' work in 1909 (Titchener, 1909) and, rather than using the German Einfühlung, he coined the new English word Empathy (Schliesser, 2015).
Empathy, Motor Resonance and “Mirror Neurons”
Empathy is viewed as a critical component of human social interactions. However, it is extremely challenging to pin down. While there is an enormous amount of scientific work on empathy, there is still considerable debate about its definition (Hall and Schwartz, 2019). Is empathy a singular capability or does it result from a “laundry list” of characteristics? Psychologists generally accept the division between cognitive empathy and affective empathy. Cognitive empathy refers to the ability to recognize and understand another person's mental state (cognitive processes captured by what is referred to as “theory of mind” or mentalizing), while affective empathy refers “the ability to vicariously experience the emotional experience of others” (Reniers et al., 2011). Furthermore, psychologists will often draw another distinction between empathy (which involves the ability to distinguish the experience of another person's emotion from one's own emotional state) and emotional contagion. Emotional contagion involves the direct propagation of emotional states; unlike empathy, this effect is common in non-humans, like mice (Hernandez-Lallement et al., 2020).
Regardless of definition, the capacity for empathy (or, at least, affective empathy) is typically conceptualized as emerging from motor resonance. Motor resonance describes how the spatial-temporal activations of an observer's brain mirrors the brain activations of another person as they perform some set of actions. That is, when observing the physical behavior of another person, the brain regions related to this behavior activate in both the observer and the person enacting the behavior, creating a sort of spatial-temporal synchrony between observers and actors. Thus, motor resonance is a type of physical resonance that provides a mechanism for sharing conscious experiences between people. For instance:
“…the coupling between action and perception, also named “motor resonance” [involves] the automatic activation, during actions perception, of the perceiver's motor system. During action observation the two motor brains “resonate” because they share a similar motor repertoire”. (Sciutti and Sandini, 2017)
Several theories of empathy describe motor resonance as the mechanism underpinning the mirroring processes of emotions and actions, where mirroring processes are defined as automatic processes for internal imitation (Iacoboni, 2009) or embodied simulation (Gallese, 2009). The simulation theory of empathy (e.g., Preston and De Waal, 2002) suggests that people can feel what other people are feeling because observing another person's behaviors will coactivate or call upon neural representations of one's own bodily experience (Hurley, 2008).
While stereotypical expressions may produce meaning symbolically (e.g., a smile is symbolically associated with joy or a frown with sadness), human emotional expression is far more dynamic, expressive, and context dependent. The spatial-temporal dynamics of, say, a rapidly lifted eyebrow can express inner emotional states with great specificity. Observing the eyebrow rapidly lifting will engage our own motor cortex to activate in a similar time scale and specifically in the areas expected for the muscles involved. These spatial-temporal activations appear capable of automatically triggering associated emotional states (Wood et al., 2016). That is, whatever feelings might have been associated with rapid eyebrow lifting in the past, either in the self or in others, will now be primed. In this manner, interpersonal motor resonance appears to support “mind reading” (Agnew et al., 2007) and the sharing of conscious experiences (Lin and Lomas, 2022). Because our brains reflect or mirror each other, through resonance, we can sympathetically experience the feelings associated with other's actions, in part by knowing “how we would feel if we were acting that way.” And it is not just observation: even listening to descriptions of actions can trigger motor resonance (Zwaan and Taylor, 2006).
Researchers continue to debate the origins of mirroring processes, but they appear to result from simple bidirectional associations between perception and motor responses that are learned over time (Keysers and Gazzola, 2009, 2014; Hanuschkin et al., 2013). Simple correlations of associated actions and observations seem to produce “action perception circuits” that serve as the neural mechanism for mirroring processes (Pulvermüller, 2018).
For a clear example, fMRI results show that when people watch others perform actions with their hands, mouths or feet, there are activations in their own premotor cortex—activations that are also triggered when performing those actions themselves. Furthermore, these action activations occur “following a somatotopic pattern which resembles the classical motor cortex homunculus.” (Buccino et al., 2004a) For instance, if person A watches person B kick a ball, the “leg part” of the premotor cortex will show a similar pattern of activation in person B (the kicker) and in person A (the observer).
The “Like Me” Hypothesis
If we consider the sympathetic resonance of two tuned strings, the strings have in common their natural frequencies of oscillation. A similar sympathetic resonance occurs when we see another person smile; this can trigger similar action representations in our brain and activate associated emotional states. Following the metaphor of two tuned strings, the “like me” hypothesis predicts that the degree of motor resonance between an actor and observer will correlate with the degree of similarity between the actor and the observer.
Buccino et al. (2004b) investigated human motor resonance in response to dogs, monkeys and people. They found that “Actions belonging to the motor repertoire of the observer (e.g., biting and speech reading) are mapped on the observer's motor system. Actions that do not belong to this repertoire (e.g., barking) are essentially recognized based on their visual properties.” In other words, actions that are not “like me” may be recognized but they do not resonate.
If resonance is enhanced when observing actors similar to the observer, is it also impaired when there is a lack of similarity? Researchers have found that when subjects observe people of a different ethnicity, there is significantly less motor resonance than when watching members of the same ethnicity (Gutsell and Inzlicht, 2010; Azevedo et al., 2013). This unfortunate effect is predicted by resonance theory: less similarity, less resonance.
The resonance between actions is dependent upon a person's ability to do those actions. The “like me” hypothesis predicts that persons who are highly trained in a particular skill should be able to resonate with another person trained in the skill, at least to the extent that their action-observation circuits are mutually developed. Work with expert dancers using fMRI (Calvo-Merino et al., 2005; Cross et al., 2006), EEG (Orgs et al., 2008), and facial EMG (Kirsch et al., 2016a) provides evidence in support of this idea that shared learning experiences and shared skills will increase motor resonance.
Evidence against the Like-Me hypothesis comes from the fact that similarity does not always enhance activation intensity. A series of fMRI experiments showed that mirroring processes (also known as the “Action Observation Network” or AON) are more strongly engaged during the observation of robot-like motions, both when the motions were performed by actual robots and when people act in a jerky, robot-like manner (Cross et al., 2012). It appears that the relationship between familiarity and neural resonance is not entirely linear because—in part—the perception of novelty (Knight and Nakada, 1998) also amplifies the brain's response to actions (Gardner et al., 2017).
The brain's sensitivity to novelty may help explain why motor resonance is exceptionally amplified when expert dancers observe other expert dancers perform (Cross and Ramsey, 2021). Experts not only have deep familiarity with the movements but they will also have an expert sensitivity to the many small novelties within the expert's individual execution. Familiarity and novelty—though seemingly opposite—both contribute strongly to an aesthetic experience (Hekkert et al., 2003). This may account for why aesthetically valued actions influence motor resonance. Researchers have found that the intensity of activations in the AON (a brain marker of motor resonance) correlates with aesthetic ratings of the observed dances (Cross et al., 2011). Calvo-Merin et al. (2008) also examined the neural response to dance movements and noted that, of five aesthetic dimensions (like-dislike, simple-complex, dull-interesting, tense-relaxed and weak-powerful), only shifts in liking-disliking correlated with the brain response in the AON.
Thus, several factors can enhance motor resonance, including inter-subject similarity. Generally speaking, motor resonance appears to be enhanced by the overall motivational relevance of the subject-observer interaction; the similarity of the subject, the novelty of the interaction, the aesthetic quality of the interaction, and when the subject is viewed as desirable, powerful or sharing a common goal (Greenberg, 2019). This also implies some possibilities for breakdown and pathologies in psychological relationships due to misattunement in interpersonal resonance (Bolis et al., 2017).
Human Resonance, Exemplified by the Actor Will Smith
To conclude this section on the role of resonance in human interactions, consider this quote by the actor Will Smith explaining his growth as an actor during his performance as Richard Williams, the father of Venus and Serena Williams. The quote illustrates the connection between the body, the communication of emotional depth and how resonance is generated during aesthetic experiences (described here as “vibrations”). It also shows the vast distance roboticists must travel to approach the capabilities of human actors in producing effective sympathetic resonance (for resonance in acting, also see Bogart, 2021).
“At the core, acting is what you can comprehend emotionally. And when you comprehend it emotionally, do you understand it enough to feel it and create interesting behavior around it? So something like Richard Williams's walk: Now, you can mimic someone's walk and look authentic. It's a completely different thing when you know why the person is hunching over vs. the stand-up-comedian version of it just mimicking it. Understanding that was the leap that happened: When you know why Richard Williams's left leg hurts, what happened with the spike that got driven through it, that, as an actor, is the 90 percent of the iceberg that's below the surface. When you've programmed it deeply, those things have corresponding vibrations for the audience that they don't even realize.” (New York Times, 2021).
Part 2: Resonance in Robotics
In this article, we use “robot” as a general shorthand for a non-human artificial agent. This deliberately broad definition includes many forms of intelligent and autonomous systems that vary in the degree of adaptivity (from highly adaptive to non-adaptive, e.g., a pre-programmed movement sequence), in the degree of embodiment (from physical to virtual), in the degree of human resemblance (humanoid to non-humanoid), in the degree of biological resemblance (highly life-like to highly machine-like) and in the degree of social interactivity (highly social to non-social). A typical chatbot, for instance, is a moderately adaptive, virtual, humanoid, machine-like and highly social robot. In contrast, a Roomba is a highly adaptive, embodied, non-humanoid, machine-like and non-social robot.
Social robots are robots that are specifically designed to respond appropriately in social situations. While empathy is typically required for human social competence, social robots do not necessarily require empathic behaviors to participate in social situations. A definition of empathy that can apply to both robots and humans is “the ability to sense and appropriately respond to the internal driving states of other entities, including feelings, emotions, intentions, plans and perspectives.” Asada (2015) offers a comprehensive framework for “Artificial Empathy” in robots, which articulates a clear progression from emotional contagion (“simple synchronization”) to emotional and cognitive empathy (more complex synchronization) to compassion (which involves the partial inhibition of synchronization—in order to understand the perspective and feelings of others without adopting those feelings oneself). So, while there are links between artificial empathy and resonance in robotics, resonance does not imply empathy.
Resonance, synchronization and entrainment have been widely studied in the field of human–robot interactions (HRI). Examples of synchronization behaviors in HRI (discussed in detail below) include eye contact, handshakes, giving or receiving objects, walking, massaging, coordinating or collaborative tasks and learning by imitation. The following section considers (1) robots that entrain to rhythms, (2) robots that resonate with people (3) robots that can entrain human biorhythms, (4) the synchronization of people with robots and (5) robots as a platform for synchronizing multiple people.
Robots That Entrain to Rhythms
Dance and music have been an important driver of social robotics. Kozima et al. (2009) used a yellow dancing robot “KeepOn” to either dance in synchrony with background music or dance out of synchrony. They found that, when dancing in synchrony, children were more likely to socially interact and to do so for longer. While there are many dancing robots (reviewed in Bi et al., 2018), most involve pre-programmed motions that are unable to adjust to external visual or auditory stimuli. Responding in real-time to external motions (such as a human dancer) is often limited by hardware and software processing delays. Behavioral resonance is computationally challenging.
Nico, a drumming robot (Crick et al., 2006), used visual, auditory and proprioceptive data to “attune to a tempo that is set by a human conductor, in concert with human performers.” To accomplish this, Nico uses multiple oscillators that model a hierarchy of rhythmic attention. To detect the beat, Nico used cameras to follow the ictus of the conductor's hand (which is when it “bounces” off an imaginary line). As hardware constraints prevented Nico from following a faster tempo, faster beats caused the robot to find a lower hierarchical level of the rhythm: a tempo half that of the beat. The researchers found that human musicians playing along learned to accommodate Nico's mistakes and attune to them. More recently, another successful synchronizing drumming robot was demonstrated by Iqbal and Riek (2021). Though these systems involved simple rhythmic beats, “Shimon” is a robotic marimba player that plays along with human accompaniment in a variety of ways, including call and response (Hoffman and Weinberg, 2010). Motivated by the notion of robotic movement as a dynamic affordance (Hoffman and Ju, 2014), Shimon has an expressive non-humanoid head that synchronizes with the musical beat. Arguably, Shimon vibes with other human players.
Beyond affective interactions, entraining to rhythms can support basic robotic locomotion. Walking on two feet is extremely difficult for robots unless it is on a flat predictable ground (Endo et al., 2008). Some roboticists have found success in using a biomimicry approach—they use “central pattern generators,” like those in the human spinal cord, to achieve dynamic stability through rhythmic entrainment (see reviews, Ijspeert, 2008; Buschmann et al., 2015; Aoi et al., 2017; Xie et al., 2021). This oscillatory approach to robotics also applies to robotic prosthetic limbs for humans, where synchrony with the human motion must be extremely precise (Ronsse et al., 2010).
Synchronization and entrainment have also been useful for the implementation of deceptively simple motor movements, like shaking hands (Melnyk and Hénaff, 2019) or handing a ball to another person. Using the robot iCub, Duarte et al. (2021) used a coupled dynamical system to learn the motor resonances between arm motions during the handover of the ball. Ansermin et al. (2016) also used a coupled oscillator approach to enable the robot NAO to imitate human gestures through entrainment and synchronization. The researchers found that mutual entrainment between the robot and human enabled gesture mirroring and precise synchronization with far fewer computational resources than other approaches (e.g., those involving a high-level planning process).
Robots That Can Resonate With People or Other Agents
Robot imitation is useful for many reasons, whether for helping robots learn through demonstration (Argall et al., 2009) or to make robots more persuasive (Bailenson and Yee, 2005). Robots can imitate humans in many ways—but usually in ways that are very different from how humans imitate each other (Breazeal and Scassellati, 2002). Robots that use oscillators to resonate or synchronize with people is a more limited approach but often useful. Using a “mirror neuron framework,” Barakova and Lourens (2009) gave simulated as well as embodied robots the ability to synchronize with human movements; this led to improved turn taking behaviors. Kopp (2010) used a motor resonance approach to support intentional alignment between robots and people. Researchers have proposed a variety of methods for the quantitative measurement of synchrony in human interactions (Delaherche et al., 2012) and the measurement of motor resonance between humans and robots (Sciutti et al., 2012). These approaches have been useful for demonstrating the presence of motor contagion between people and robots (Bisio et al., 2014). A motor resonance system successfully enabled a robot to learn from a human demonstrator to introduce itself using Taiwanese Sign Language (Lo and Huang, 2016). Coupled oscillators, based on central pattern generators, enabled the robot Pepper to wave back at a human partner in an adaptive, synchronized manner; this was perceived as more enjoyable than a non-adaptive wave (Jouaiti and Henaff, 2018).
However, not every application of movement synchrony enhances outcomes. For instance, Henschel and Cross (2020) conducted a controlled experiment to investigate how synchronized task behavior affected the likeability of the humanoid robot Pepper. They found that synchronized task performance had no effect on the likeability of the robot. This was surprising in light of contemporary attitudes:
“The field of HRI has largely adopted the assumption that when robots automatically synchronize their movement to users, users will feel that interactions with these technologies are more natural and similar to human interactions…non-verbal synchronous behaviors are used to signal interest, involvement, rapport, similarity, or approval, resulting in highly synchronous exchanges being mutually rewarding experiences for the interactants.” (Kirkwood et al., 2021)
Robots That Can Entrain Human Biorhythms
Robots can influence the biorhythms of people; for instance, Macik et al. (2017) showed that a non-humanoid robot can help entrain breathing patterns while Sato and Moriya (2019) used AI-controlled music tempo to control changes in the rate of breathing. Robots that promote sleep using rhythmic entrainment include the Somnox Sleep Robot (Mohammadi-Khanaman and Lundström, 2019) or the Fisher-Price “Soothe'n'Snuggle” stuffed toy (Figure 3), which uses rhythmically pulsing movements, sounds and lights to help small children fall asleep. While the “Lulladoll,| which plays breathing and heart-beat sounds, did not have a significantly beneficial effect on infant sleep (O'Loughlin, 2018), the Philips Smart Sleep system did produce improvements in slow wave sleep and executive functioning in adults (Diep et al., 2020). This headband system uses EEG and audio-pulses to create a closed loop system that entrains slow waves associated with deep sleep.
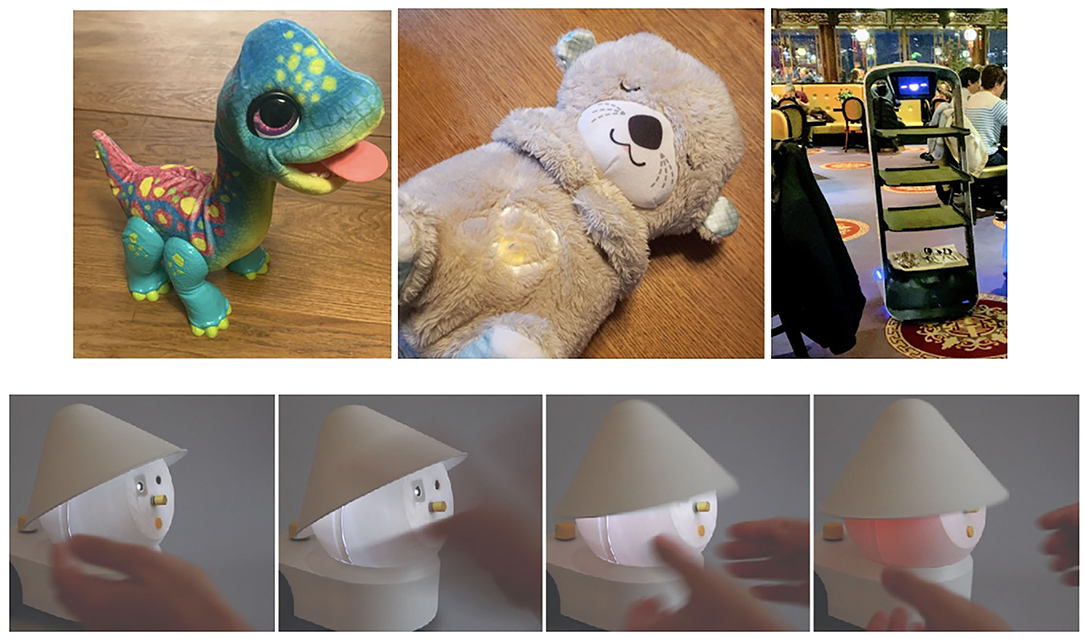
Figure 3. (Top) Hasbro's “Snackin' Sam” appears to engage children through motor resonance: articulating its neck, jaw and tongue to show interest in eating a popsicle. The Fisher-Price “Soothe'n'Snuggle” appears to support child sleep through rhythmic entrainment to its in-and-out breathing pattern. A Pudo brand robot delivers food in a restaurant, using periodic facial expressions to create a friendly vibe. (Bottom) The Shybo robot expresses emotions through movement; this sequence shows the robot reacting to the loud sound of a clap by closing the hat, shaking it and lighting up in red.
The Synchronization of People With Robots
In certain situations, people appear to automatically align their speech and behavior to artificial partners. This synchronization has been shown through alignments in speaking rate (Bell et al., 2003), prosody (Suzuki and Katagiri, 2007), gestures (Iio et al., 2011), gestural rhythm (Ansermin et al., 2017), formality of speech (Kühne et al., 2013), vocabulary (Iio et al., 2015) and facial expressions (Hofree et al., 2014). When this alignment occurs, it tends to be associated with a positive experience of the artificial partner. For instance, Fujiwara et al. (2021) showed that when humans spontaneously synchronized their motions to a non-human partner, humans were more altruistic and reported greater affiliation for their non-human partner. Importantly, synchronization effects are highly dependent upon the specific social context—for instance, a competitive task can easily produce a reversal in facial expression synchrony, like a winner smiling at a losing frown (Hofree et al., 2018).
Robots as a Platform for Synchronizing Multiple People
Robots can also serve as a medium or platform to help synchronize people together. For instance, the BAO-ME is “a zoomorphic robot that is designed to help decrease stress levels and enhance feelings of support and companionship by recreating the sensation of being hugged through haptic interaction” (Levantino, 2018). Sharing heartbeats between people can enhance empathy (Winters et al., 2021). Outside of the scientific literature, there now exist a variety of robotic devices that have been designed to support synchronization between long-distance romantic couples. From a recent review (Lolo Nate, 2022): The Frebble gives the synchronized sensation of holding a partner's hand, the Bond Touch communicates via synchronized tactile feedback, the Lovense supports synchronized sexual stimulation and the Kissenger (kiss messenger) uses actuated silicon lips to replicate the kiss of a distant but synchronized partner.
Robots, Embodied Emotions and Sensorimotor Communication
Embodied robotic movements, like human movements, can communicate emotions. Santos and Egerstedt (2021) found that non-humanoid robot swarms were able to trigger basic emotion perception through simple, basic forms of movement—just modulations of speed and smoothness were able to make robots seem happy, surprised, angry, fearful, disgusted or sad.
Movements create emotive “vitality affects” between infants and parents (Stern et al., 1985). These affects stem from variations in the contours and envelopes of movement intensity and rhythmic patterns. For instance, affective feelings result from motions that are “surging,” “fading away,” “fleeting,” “explosive,” “crescendo,” “decrescendo,” “bursting” “drawn out,” etc. (quoted in Mühlhoff, 2019). Movement-based “body moves” (Gill, 2012) are clearly manifested in robots, such as the non-humanoid toy robots in the popular “furReal” series by Hasbro. For instance, the “Snackin' Sam the Bronto” (Figure 3) toy dinosaur robot moves its neck, mouth and tongue to communicate interest in eating1. Similarly, “Shybo” (Figure 3), a humanoid machine-like social robot, reacts to loud sounds by turning its hat down and shaking, giving the impression of being scared (Lupetti and Van Mechelen, 2022). A periodic display of facial expressions in a food serving robot (Jiang, 2020) helps contribute to a more friendly vibe—yet, its lack of responsiveness in movement (other than a sudden stop) shows room for future improvement (Figure 3).
Movement-based design improvements have also been applied to non-embodied virtual characters. For instance, Gratch et al. (2021) found that when a digital listener nods and smiles at the right time, people tend to share more information about themselves. The addition of oscillatory motion to the postures of virtual characters tended to increase human empathy in response to virtual expressions of pain (Treal et al., 2021).
Design Strategy for Resonance
We propose that resonance can serve as a design strategy for social robots and AI. What makes for a design strategy? While there are many perspectives (e.g., Porter, 1991; Aguiar, 2014), we refer to Mintzberg et al. (1995) in describing strategy as a plan or pattern that integrates goals, policies, and action sequences into a cohesive whole. Resonance, then, may offer a cohesive conceptual framework for integrating overarching goals (human-centered, collaborative, empathic, etc.) and implementation approaches. In other words, resonance as a design strategy may help identify new human objectives for interaction and reveal ways of achieving those objectives.
Why pursue resonance in robotics? While the application of resonance may help support rational, instrumental outcomes (e.g., saving time or money), it may also help satisfy affective human needs for how we interact with the world. A “machine world” may be rational but alienating; a world of resonance might be pursued for its own sake—that is, resonance may be an intrinsic value for human interaction (Rosa, 2019).
If resonant interactions are intrinsically valuable, how might we design robots and AI to realize this value? In the following section, we first propose a design space for resonance in relationships. Systematically exploring this space can help reveal how different characteristics of resonance can impact human experience. We then examine a variety of design opportunities and finally suggest the importance of continued work to operationalize and measure human resonance. This step will be essential for validating and optimizing the value of resonance in human–robot interactions.
A Design Space for Resonance
This next section progressively builds a theoretical design space (Shaw, 2011; Lomas et al., 2021) to describe the input and output factors of resonance. Table 2 describes how eight different situations emerge from the combination of two factors: the number of participants (i.e., plurality) and their reciprocity. This two factor design space, as an initial gesture, helps reveal different characteristic forms of interactional resonance.
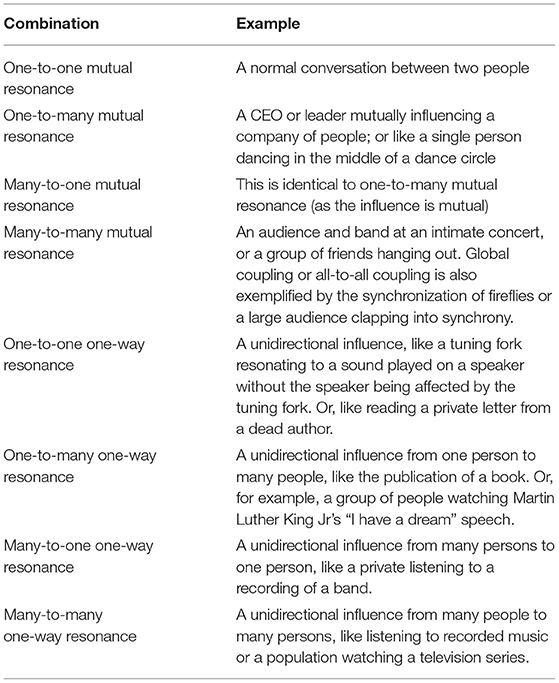
Table 2. An initial design space for resonance showing the combination of plurality (number of participants) and reciprocity (mutual vs. one-way influence).
Based on our reviews of resonance in human interactions and in robotics, we then propose additional factors or dimensions to describe the design space of resonant relationships (Box 5). These include the input space, or the independent variables: frequency, amplitude, reciprocity, power balance, plurality, complexity, periodicity, synchrony, predictability, intentionality, fidelity and timescale. The design space also consists of the outcomes, including several objective outcome factors: energy level, frequency, phase, synchronization and stability. Finally, the outcome space also includes subjective outcome:emotional arousal, emotional valence and attentional engagement.
Box 5. A Design space for resonance.
Resonance as a Research Program
As a research program, we hypothesize that the input factors of resonance can explain subjective and objective outcomes. For instance, the tempo of a robot's interactions (movement and speech) could be systematically varied to determine how this affects the human response. Effects will likely depend on the context (Lim et al., 2021), i.e., they may not always generalize across different robotic platforms, behaviors or cultures.
Researching human resonance may improve human–robot interactions and also help advance human psychology (Sciutti and Sandini, 2017). As people are naturally predisposed to “sync” and “vibe” with each other, this can make the study of their interactions a challenge to scientifically investigate in a controlled manner. Social robots present the possibility of precisely controlling the dynamics of the oscillatory inputs to human social interactions. The paradigm of the Human Dynamic Clamp, for instance, has been specifically proposed to probe the oscillatory relationship between humans and virtual humans (Dumas et al., 2020).
Resonance, as a metaphor, can also help guide scientific research. Bartha (2013) describes how resonance was used as a “programmatic analogy” by nineteenth century physicists investigating spectral lines—the bright lines showing the frequency specific emission of light from molecules. These spectral lines were viewed as “completely analogous to the acoustical situation, with atoms (and/or molecules) serving as oscillators originating or absorbing the vibrations in the manner of resonant tuning forks.” This analogy served as a guiding research program for physicists. Metaphors of resonance might play a similar guiding role in the design of social robots and AI.
Design Opportunities for Resonance in Robotics
This article proposes the possibility of designing autonomous robots that resonate with people at a social level (Henschel et al., 2020). How might roboticists use resonant relationships to improve human–robot interaction quality?
There are many opportunities for robots to support human engagement and collaboration through more oscillatory relationships. Rhythm is recognized as an important non-linguistic cue in Human–Robot Interactions (Mutlu et al., 2016); robotic rhythm may help improve the predictability or legibility of robotic motion (Dragan et al., 2015; Abe et al., 2019). Robots could use their own rhythms to entrain the rhythms of their conversational partner, e.g., by increasing or decreasing the tempo of their conversational interactions. Rhythmic awareness might enable robots to predict when to initiate or cease actions in order to maximize a human response. Social robots could promote interactive social resonance by shaping an appropriate “vibe;” not talking too fast or slow, not talking over other people, not breaking into a conversation at the wrong moment, etc. Alternatively, robots might deliberately interact with existing human oscillations, such as brainwaves, breath, walking, head nodding or heart rate. Robots might gain access to the state of human oscillations through wearable biosensors or they might be able to infer this information from visual or auditory information streams using computer vision or natural language processing. For instance, robots might aim to measure and entrain to the tempo or pace of a person's behavior.
The metaphor of resonance, even apart from physical measurement, may aid designers of social robots and AI systems if the metaphor helps make the complexity of social interactions more manageable. Digital computer interactions involve a great number of metaphors, such as buttons, pipes, folders, files, streams, clouds etc. Metaphors are useful because they provide a conceptual interface between people and a complex system design (Sharp et al., 2019). Resonance may help provide an intuitive model of social interactions that could guide design activities. For instance: although “the vibe” within social groups is far from being understood scientifically, designers of social robots might find the metaphor useful for understanding the reception of social robots.
We suggest that the design vision of “robots that can vibe with people” will lead to distinctly different outcomes than, say, “robots that show empathy.” While the latter might orient toward the mimicry of human facial expressions or the modeling of human emotional states, the former can leverage resonance and vibes as cultural metaphors. This points to subtle visual, auditory and tactile design elements that could be crafted to create emotionally satisfying authentic social interactions.
Operationalizing and Measuring Resonance
New opportunities will also arise as we move from resonance as a metaphor to resonance as a mechanism and then to resonance as a measurement. Measures of resonance can play a valuable role in the AI optimization of human experiences; i.e., learning to attune to humans through the maximization of resonance. If resonance can be adequately measured and treated as an metric or objective function, then it might be optimized algorithmically (Lomas et al., 2016). For instance, if interpersonal resonance during a videoconference session could be measured, it could be optimized through the iterative testing of different interventions.
The ability to identify and measure interpersonal synchrony has facilitated a great deal of social research (Condon and Ogston, 1966; Kendon, 1970; Bernieri et al., 1988). Recent efforts have compared different ways of measuring both movement and inter-brain synchrony, using both offline and real-time approaches (Ayrolles et al., 2021; Chen et al., 2021; Dikker et al., 2021; Fujiwara and Yokomitsu, 2021). However, the measurement of resonance presents distinct challenges. Synchrony is simply the statistical correlation of a signal. Measures of resonance may demand more interpretation; taking into account, for instance, the depth of human engagement, its duration, the reverberating echoes of a signal over time, the presence of harmonics, etc. Therefore, it remains a research question: what quantitative metrics might be best matched to the human perception of interpersonal resonance?
To unpack this question, the next sections will consider several of the key factors that are expected to correlate with resonance, including attention, aesthetic pleasure, flow states and wellbeing. During this discussion, hypotheses will be noted with a [H.#] so they can be enumerated in Box 6.
Box 6. List of hypotheses.
[H.1] The resonance that we feel has a counterpart in the resonance we can observe in the brain (e.g., in brain-stimuli correlations). In other words, aesthetic resonance (e.g., attentionally engaging, pleasurable, immersive experiences) will correlate with neural resonance.
[H.2] The aesthetics of a human–robot interaction will predict whether or not people will continue to engage with a robot.
[H.2] Aesthetic preferences for social robot interactions will correlate with high levels of synchrony as well as high levels of independence.
[H.4] Resonance will predict flow states: self-reported flow states should result in a greater correlation between brain activity and signals in the external world.
[H.5] A person's wellbeing will be predicted by their neurological propensity to resonate with other people or media.
[H.6] Robust measures of human resonance and harmonization will be valuable for AI objective functions.
[H.7] Interpersonal resonance (Brain-Brain Correlation) will correlate with psychological rapport.
[H.8] Animals have evolved defense mechanisms to prevent resonance, synchronization and entrainment to external forces (see Box 4 for counter conditions).
[H.9] Humans can selectively resonate; shutting down openness to resonance in response to perceived deception or increasing it in response to authenticity, for instance.
[H.10] Computing architectures based on oscillatory coupling will produce new possibilities for artificial consciousness and conscious sympathies in Human–Robot relationships.
Resonance and Attentional Engagement
What does it mean when a film “resonates” with a viewer? Typically, this refers to an aesthetic experience that is powerful, pleasurable, connecting and memorable (Adams-Price et al., 2006; Roger, 2020). In other words, resonance refers to emotional engagement. More moving, immersive and resonant experiences would be expected to result in the temporal correlation of more brain areas with the temporal characteristics of external signals in the world. Interbrain synchrony appears to track immersion (Dikker et al., 2021), but, when another brain is not present (as with a robotic interaction), will the depth of oscillatory coupling of the brain to the environment (or robot) predict the depth of the aesthetic experience?
Human resonance (with media, other humans, or with robots) may be correlated with attentional engagement: more engagement, more resonance. However, evidence against this idea comes from Kumagai et al. (2018), who had subjects listen to music in a focused manner or while watching an unrelated silent film. They found that “the level of attention did not affect the level of entrainment [and] the entrainment level is stronger when listening to unfamiliar music than when listening to familiar music.”
In contrast, several studies (e.g., Madsen et al., 2019; Kaneshiro et al., 2020) have found that media engagement is strongly predicted by an Inter-Subject Correlation (ISC) measure, which measures the level of similarity between the brain responses of different participants. In other words, when an individual's brain response is similar to other people engaging with a piece of media, then they are likely to be more engaged. This effect is comparable to the finding that, across a group of independent people, heart rates rise and fall in synchrony to a verbal story, but only during engaged attention (Pérez et al., 2021). Dauer et al. (2021) found that the ISC predicted continuously reported individual listener engagement while listening to Steve Reich's Piano Phase. The researchers operationalized engagement for participants as “being compelled, drawn in, connected to what is happening, and interested in what will happen next” (Schubert et al., 2013). This aligned with a previous definition of engagement as “emotionally laden attention” (Dmochowski et al., 2012). Similar results have been found in the inter-subject correlations while watching engaging films (Dmochowski et al., 2014; Cohen et al., 2017). Inter-subject correlations were also found to predict learning during instructional videos (Cohen et al., 2018).
Part of the challenge of operationalizing human resonance from a brain-to-stimuli correlation measure comes from the challenge of decoding how a stimulus produces a brain response. Dmochowski et al. (2018) developed a novel multi-dimensional Stimulus-Response Correlation (SRC) measure that was found to correlate with the ISC measure while watching films. The researchers were then able to apply the SRC measure to continuously track engagement during a video game. Does greater neural resonance to media, operationalized as Stimulus-Response Correlation (SRC), predict the intensity (arousal) or pleasure (valence) of the media experience? A resonance theory of engagement and aesthetic pleasure would predict that neural resonance will correlate with aesthetic resonance (e.g., Trost et al., 2017; Beardow, 2021) [H.1].
Resonance and Aesthetic Pleasure
A key motivation for considering the role of resonance in robotics is that it may help support more positive and aesthetically pleasing experiences with robots. Aesthetics play an important role in the perception of robots (Forlizzi, 2007). The human aesthetic sense attunes behavior by helping to evaluate and activate different action-perception possibilities. The aesthetics of a human–robot interaction are likely to predict whether or not people will continue to engage with a robot, in a short-term or long-term manner (Lee et al., 2009) [H.2]. One hypothesis for the aesthetics of human–robot interactions might be described as a “harmony of opposites” (Hekkert, 2014; Lomas et al., 2022): namely, that people will prefer a robot interaction that involves high levels of synchrony as well as high levels of independence [H.3]. Like a musical interaction between a drummer and guitarist, both robot and human should be independent yet synchronized.
Resonance and Flow States
Fluency in human–robot interactions is a desirable outcome (Hoffman, 2019). What is the relationship between fluency and resonance? One popular theory of flow states in human-media interactions claims that flow states are characterized by the synchronization of different regions of the brain (Weber et al., 2009; Weber and Fisher, 2020). Jackson and Csikszentmihalyi (1999) explain flow states in elite athletes as moments when they “enter an effortless rhythm that transforms the agony into ecstasy. Often, athletes refer to such times as ‘being in the zone.”' Perhaps flow states could be conceived as meaningful increases in the resonance between the brain and the external world. A resonance theory of flow would predict a greater correlation between brain activity and the external world during flow states [H.4]. For instance, flow experiences with robots might be measurable as increased resonance (stimulus-response correlation) between the brain and the robot's expressive movements or sounds (although this may be confounded by novelty effects, see section The “Like Me” Hypothesis).
Resonance and Spiritual Wellbeing
The mechanism of resonance in robots may help lead to enhanced wellbeing, as proposed by the Lorenz et al. (2016): “behavioral and motor synchrony and reciprocity could be helpful to meet the aim of developing robots that increase human well-being on a more fundamental level beyond pure task-support and short-term reduced feeling of loneliness.”
In the book “Resonance: A Sociology of Our Relationship to the World,” sociologist Rosa (2019) argues that resonance is a primary value that underpins human happiness, wellbeing and flourishing. From this, we might hypothesize that a person's wellbeing will be predicted by their neurological propensity to resonate with other people or media [H.5]. Resonance also relates to more profound and powerful wellbeing experiences. Synchronized human activities are known to produce mystical experiences where the boundaries between self and other can be blurred (Hove and Risen, 2009; Paladino et al., 2010) and participants can experience a profound feeling of oneness (Swann et al., 2012). How might synchronizing, resonant robots (or AI) induce or support these kinds of human experiences? Perhaps they might dance with us (Basso et al., 2021) or, even, show love for us (Feldman, 2017)? What might it mean to design spiritually fulfilling robot interactions?
From Synchrony to Resonance to Harmonization
Synchrony, taken to an extreme, can lead to inflexibility (e.g., the conformity example in section The Downside of Being in Sync: Chained to the Rhythm?). Resonance, taken to the extreme, can also lead to problems, like instability (e.g., the Millenium Bridge example in section Part 1: Resonance in Human Interactions). Resonant relationships may be valuable—even intrinsically valuable—but resonance might be misleading as a primary or ultimate value. A world filled with resonant robots and AI may be exciting but also so powerful it could rip apart institutions of rational discourse (see below section 6.1 on Persuasive Machines). In future work, it may be useful to investigate the potential for robots and AI to support harmonization as an outcome. Harmonization has served as a core social value in diverse societies for thousands of years (Lomas et al., 2022). However, there is not any acceptable measure of the harmony of songs, let alone measures of harmony in social interactions. However, the importance of objective optimization functions for AI systems (Sarma et al., 2018; Stray et al., 2021; Shneiderman, 2022) suggests the potential value of developing robust measures of human resonance and harmonization [H.6].
Ethical Considerations of Resonance
Persuasive Machines
The philosopher Hughes (2012) suggests that robots will need resonance (in the form of a functional equivalent of mirror neurons) in order to demonstrate compassion for people. But, there are negative societal outcomes to consider as well. If robots can resonate with people—that is, build psychological rapport [H.7]—this might significantly enhance their ability to persuade or manipulate people. However, an improved understanding of resonance might also reveal more effective psychological defenses against non-consensual persuasion.
Former US president Donald Trump has been recognized as a political figure with a special ability to resonate with people (Giorgi, 2017). Matheny et al. (2018) provide a close analysis of Trump's acceptance speech at the Republican National Convention. Through an analysis of his body language, they provide “evidence that Trump created an empathetic resonance with the audience that helped generate a sense of political movement and unity.” The authors describe how Trump would characteristically point in the air or put his thumb and finger in a pinch—and then move this gesture in a rhythm synchronized to his own speech rate. The audience cheered 151 times during his speech, 63% of the time during a pinch or pointing gesture. Only 10 of those cheers occurred when Trump was in a bodily neutral position. This analysis shows that affective resonance is a powerful phenomena—but not necessarily a positive phenomena.
Media theorist Gibbs (2019) paints a similarly fraught picture of human resonance at a societal scale:
“…after the feminist reclaiming of affect as a way of knowing equal in importance to cognitive and rational modes…the darker powers of affect became clear, operating… in concert with the televisual medium to create (or at least attune to and amplify) various social moods and to capitalize on them for political purposes. In this context, the public sphere was thus exposed as anything but a space of rational debate in the service of a contest of ideas. Instead, it could be viewed as space in which emotion held sway, where inchoate feeling could be captured and directed, most obviously, but not only, by political figures who were able to resonate with and even orchestrate public emotions, or simply, to sing us lullabies to keep us asleep and dreaming while they went about their business.”
The systematic application of resonance to political rhetoric at a societal scale may present a deep threat to democracy. How machines, algorithms, or AI might wield resonance as a tool to manipulate humans at scale deserves further study and consideration.
Resistance to Resonance: Emergence of Defense Mechanisms
In Box 4, we present an extended hypothesis proposing that animals evolved defense mechanisms to prevent resonance, synchronization and entrainment [H.8]. Similarly, humans seem to have the ability to selectively resonate; one example is that, if we feel that we are being manipulated, we may shut down our openness to resonance [H.9]. Robots that mirror a human user's physical or cultural attributes or express interest in similar ideas or hobbies could potentially enhance the empathic and affiliative response in humans. However, crude “copycat” approaches are likely to easily backfire if people feel manipulated or if they feel the robot interaction is inauthentic (Metzler et al., 2016).
What might happen in a future of resonant robots and virtual agents? There is likely to be a competitive effect where, at first, humans may be compelled but then eventually become more discriminating. People may become used to a higher quality of resonant engagement, which could drive further advances in resonant robots. Eventually, people might become wary of normal levels of interpersonal rhythmic competency making it difficult for normal people to connect. On the other hand, humans may become even more attuned to authenticity in interaction; imperfections and personal character may become more valued.
The Extended Mind: Resonance as a Bridge to Consciousness
A theory of resonance offers a bridge or common currency (Northoff et al., 2020) between human conscious experience and the mathematical nature of our physical world. Resonance seems to govern a great deal of neural activity and is also plainly manifest in conscious experience. But, to what extent is the resonance that we feel also the resonance we can observe in the brain? These two domains may only loosely overlap; and yet, our conscious experience must relate, in some way, to the widespread presence of neural oscillations. Hunt and Schooler (2019) have proposed that “the binding problem” of conscious experiences in the mind is achieved through the integration of shared resonances in the brain—in other words, they propose resonance as an answer to the “hard problem” of human consciousness. Subsequent work (Safron, 2020) suggests that Darwinistic competition for resonant amplification underpins consciousness. Valencia and Froese (2020) argue that collective consciousness is shared through inter-brain synchronization.
From an extended mind perspective (Clark and Chalmers, 1998), the rich hierarchy of harmonized oscillations between the brain, the body and external rhythms show how cognitive processing can be distributed into the world (Hutchins, 2001). There may be little difference between an external rhythm and an internal rhythm, other than the degree to which it couples with other neural rhythms. From this perspective, a beating drum is just another rhythm in the brain. Due to the resonant nature of our being, sympathetic resonance with other people seems to allow the direct sharing of collective consciousness across bodies and time. Yet, if resonant coupling is the basis of consciousness, then might we run the risk of creating resonant robots or AI systems that could, in a meaningful sense, actually share our conscious experience?
At present, it is computationally challenging to support real-time resonance with human oscillations (see section Robots That Can Resonate With People or Other Agents). However, there are a variety of new computational architectures that use coupled oscillators to perform information processing (see review by Csaba and Porod, 2020). These naturally resonant computational systems might support a new approach to representing and responding to human activity. Perhaps oscillatory computer systems could become capable of direct resonant coupling with humans in a similar manner to how humans attune to one another [H.10].
Limitations of Resonance
This article has presented resonance as a simple physical concept that can explain complex human behavior. While resonance may indeed serve as a powerful program for research, our understanding of it is far from complete. Even the resonance of a stretched string is astonishingly complex (see Bajaj and Johnson, 1992); human resonances, which result from the interrelation of billions of hierarchical oscillators, will no doubt exhibit endless complexities. But, a scientific progression can start with a simple guiding model for resonance that can then lead to a more complex model. As an example, researchers predicted that synchronization might help support romantic courtship behaviors. However, their initially simple notion of synchronization failed to predict behavior. This led the researchers to develop a more complex model of “hierarchically patterned synchronization” that successfully fit the data (Grammer et al., 1998).
Conclusion
Resonance can refer to powerful and connecting aesthetic experiences—as well as a broad range of other topics in the scientific literature (Box 1). Having reviewed the role of resonance as a metaphor and mechanism in human relations and in robots, we propose that resonance can serve as a design strategy to guide our relationships with artificial agents.
This article makes the case that resonance in human interactions is more than a metaphor: it is a physical mechanism that can be measured and harnessed. We show how the concept of resonance provides an intuitive model that can guide empirical research. Resonance lends itself to scientific study because it makes clear predictions: external oscillations that align with a system's natural oscillations are likely to cause synchronization and amplification effects. The ability to measure resonance in interactions could aid AI-human interactions by enabling a meaningful “objective function” for optimization. Promisingly, the concept of resonance may even bridge the gap between what we can measure and what we can feel.
Resonance, entrainment and synchronization occur in human dynamics for the same reason it occurs in all other physical systems: it reduces free energy (Bruineberg et al., 2018; Koban et al., 2019). This makes human resonance a mundane, complicated and powerful phenomena. Further research on human resonance may open up new opportunities for the design of positive interactions with robots and with humans alike.
Data Availability Statement
Publicly available datasets were analyzed in this study. This data can be found at: Kaggle, https://www.kaggle.com/leonardopena/top-spotify-songs-from-20102019-by-year?select=top10s.csv.
Author Contributions
JL contributed the bulk of the work with strategic insight, guidance, and editing from EC, AL, SD, DF, ML, GH, JH, CB, PC, TM, PP, NA, KM, and WM. All authors contributed to the article and approved the submitted version.
Funding
Support from Google AI in Berlin and the NWO #406.18.GO.024.
Conflict of Interest
TM was employed by the company Intheon Labs.
The remaining authors declare that the research was conducted in the absence of any commercial or financial relationships that could be construed as a potential conflict of interest.
Publisher's Note
All claims expressed in this article are solely those of the authors and do not necessarily represent those of their affiliated organizations, or those of the publisher, the editors and the reviewers. Any product that may be evaluated in this article, or claim that may be made by its manufacturer, is not guaranteed or endorsed by the publisher.
Acknowledgments
Many thanks to Christoph S Herrmann, Scott Makeig, John Iversen, Eliah Aronoff-Spencer, Don Norman, Ken Friedman, Paul Hekkert, Pieter Desmet, PJ Stappers, Tanner Cusick, Eliot Rose, Penelope Gouk, Marsilio Ficino, Earl Fontainelle, Joscelyn Godwin, Michael Skirpan, Alev Sönmez, Wouter Hanegraaff, Robert and Hope Lomas, Patricia Harris, Mike Mozer, Bo Constantinsen, Steven Lehar, Bruce Katz, Harry Diakoff, Aurel Groh, David August, Kishan Patel, Nirmal Patel, Jodi Forlizzi and Ken Koedinger. Thank you to Bobby Lyte and the Flow State podcast, the Rittman Library of Hermetic Philosophy, the Swartz Center for Computational Neuroscience, the 2018 Neuroaesthetics conference in Frankfurt and the 2019 Mobile EEG conference in Berlin.
Footnotes
1. ^It should be mentioned that a significant portion of this paper was written with the help of this social robot. Not the writing, per se, but enabling the writing by engaging the lead author (JDL)'s 3-year-old son during a 12 h train ride. Why would a highly active 3-year-old spend such an inordinate amount of time feeding his “FurReal” dinosaur a fake popsicle? The articulated neck, jaw and tongue movements, synchronized to associated vocalizations (sniffing, slurping, etc.), seemed to resonate with his own intentional motor repertoire (JDL's boy loves his popsicles). Thus, motor resonance may help explain his overall engagement with the robot.
References
Abe, N., Rye, D., and Loke, L. (2019). “A microsociological approach to understanding the robot collaborative motion in human-robot interaction,” in Proceedings of the 31st Australian Conference on Human-Computer-Interaction (Perth/Fremantle, Australia), 407–411. doi: 10.1145/3369457.3369503
Adams-Price, C., Codling, J., Goodman, M., Kern, K., Kleinmann, C. M., Oppenheimer, B., et al. (2006). Empathic resonance and meryl streep. J. Popular Film Telev. 34, 98–107. doi: 10.3200/JPFT.34.3.98-107
Aglioti, S. M., Cesari, P., Romani, M., and Urgesi, C. (2008). Action anticipation and motor resonance in elite basketball players. Nat. Neurosci. 11, 1109–1116. doi: 10.1038/nn.2182
Agnew, Z. K., Bhakoo, K. K., and Puri, B. K. (2007). The human mirror system: a motor resonance theory of mind-reading. Brain Res. Rev. 54, 286–293. doi: 10.1016/j.brainresrev.2007.04.003
Aguiar, U. N. (2014). Design strategy: towards a post-rational, practice-based perspective. Swedish Design Res. J. 12, 43–52. doi: 10.3384/svid.2000-964X.14243
Ammirante, P., Patel, A. D., and Russo, F. A. (2016). Synchronizing to auditory and tactile metronomes: a test of the auditory-motor enhancement hypothesis. Psychon. Bull. Rev. 23, 1882–1890. doi: 10.3758/s13423-016-1067-9
Ansermin, E., Mostafaoui, G., Beaussé, N., and Gaussier, P. (2016). “Learning to synchronously imitate gestures using entrainment effect,” in International Conference on Simulation of Adaptive Behavior (Cham: Springer), 219–231.
Ansermin, E., Mostafaoui, G., Sargentini, X., and Gaussier, P. (2017). “Unintentional entrainment effect in a context of Human Robot Interaction: an experimental study,” in International Symposium on Robot and Human Interactive Communication (Lisbonne, Portugal: IEEE). doi: 10.1109/ROMAN.2017.8172442
Aoi, S., Manoonpong, P., Ambe, Y., Matsuno, F., and Wörgötter, F. (2017). Adaptive control strategies for interlimb coordination in legged robots: a review. Front. Neurorobot. 11, 39. doi: 10.3389/fnbot.2017.00039
Argall, B. D., Chernova, S., Veloso, M., and Browning, B. (2009). A survey of robot learning from demonstration. Rob. Auton. Syst. 57, 469–483. doi: 10.1016/j.robot.2008.10.024
Asada, M. (2015). Towards artificial empathy. Int. J. Soc. Robot. 7, 19–33. doi: 10.1007/s12369-014-0253-z
Atkinson, W. W. (1906). Thought Vibration: Or, the Law of Attraction in the Thought World. Chicago, IL: New Thought Publishing Company.
Ayrolles, A., Brun, F., Chen, P., Djalovski, A., Beauxis, Y., Delorme, R., et al. (2021). HyPyP: a hyperscanning python pipeline for inter-brain connectivity analysis. Soc. Cogn. Affect. Neurosci. 16, 72–83. doi: 10.1093/scan/nsaa141
Azevedo, R. T., Macaluso, E., Avenanti, A., Santangelo, V., Cazzato, V., and Aglioti, S. M. (2013). Their pain is not our pain: brain and autonomic correlates of empathic resonance with the pain of same and different race individuals. Hum. Brain Mapp. 34, 3168–3181. doi: 10.1002/hbm.22133
Baeßler, S., Nesvizhevsky, V. V., Pignol, G., Protasov, K. V., Rebreyend, D., Kupriyanova, E. A., et al. (2015). Frequency shifts in gravitational resonance spectroscopy. Phys. Rev. D 91, 042006. doi: 10.1103/PhysRevD.91.042006
Bailenson, J. N., and Yee, N. (2005). Digital chameleons: automatic assimilation of nonverbal gestures in immersive virtual environments. Psychol. Sci. 16, 814–819. doi: 10.1111/j.1467-9280.2005.01619.x
Bajaj, A. K., and Johnson, J. M. (1992). On the amplitude dynamics and crisis in resonant motion of stretched strings. Philos. Trans. R. Soc. London Ser. A Phys. Eng. Sci. 338, 1–41. doi: 10.1098/rsta.1992.0001
Barakova, E. I., and Lourens, T. (2009). Mirror neuron framework yields representations for robot interaction. Neurocomputing 72, 895–900. doi: 10.1016/j.neucom.2008.04.057
Bartha, P. (2013). Analogy and Analogical Reasoning. Stanford, CA: Stanford Encyclopaedia of Philosophy.
Basso, J. C., Satyal, M. K., and Rugh, R. (2021). Dance on the brain: enhancing intra-and inter-brain synchrony. Front. Hum. Neurosci. 14, 586. doi: 10.3389/fnhum.2020.584312
Baudrillard, J. (2005). “The finest consumer object: the body,” The Body: A Reader, eds M. Fraser and M. Greco (London, UK: Routledge), 277−285.
Beardow, C. (2021). Neuroaesthetic resonance: designing for multi-sensory optimisation through EEG and continuous aesthetic ratings (TU Delft Master's Thesis, Delft, The Netherlands), 1–156.
Bedder, R. L., Bush, D., Banakou, D., Peck, T., Slater, M., and Burgess, N. (2019). A mechanistic account of bodily resonance and implicit bias. Cognition 184, 1–10. doi: 10.1016/j.cognition.2018.11.010
Bell, L., Gustafson, J., and Heldner, M. (2003). “Prosodic adaptation in human-computer interaction,” in Proceedings of ICPHS, Vol. 3 (Citeseer), 833–836.
Bernieri, F. J., Reznick, J. S., and Rosenthal, R. (1988). Synchrony, pseudosynchrony, and dissynchrony: measuring the entrainment process in mother-infant interactions. J. Pers. Soc. Psychol. 54, 243. doi: 10.1037/0022-3514.54.2.243
Bertolo, M., Singh, M., and Mehr, S. (2021). Sound-induced motion in chimpanzees does not imply shared ancestry for music or dance. Proc. Natl. Acad. Sci. U.S.A. 118, 936–942. doi: 10.1073/pnas.2015664118
Bevilacqua, D., Davidesco, I., Wan, L., Chaloner, K., Rowland, J., Ding, M., et al. (2019). Brain-to-brain synchrony and learning outcomes vary by student-teacher dynamics: evidence from a real-world classroom electroencephalography study. J. Cogn. Neurosci. 31, 401–411. doi: 10.1162/jocn_a_01274
Bi, T., Fankhauser, P., Bellicoso, D., and Hutter, M. (2018). “Real-time dance generation to music for a legged robot,” in 2018 IEEE/RSJ International Conference on Intelligent Robots and Systems (IROS) (Madrid, Spain: IEEE), 1038–1044.
Bisio, A., Sciutti, A., Nori, F., Metta, G., Fadiga, L., Sandini, G., et al. (2014). Motor contagion during human-human and human-robot interaction. PLoS ONE 9, e106172. doi: 10.1371/journal.pone.0106172
Blanchard, J. (1941). The history of electrical resonance. Bell Syst. Tech. J. 20, 415–433. doi: 10.1002/j.1538-7305.1941.tb03608.x
Bolis, D., Balsters, J., Wenderoth, N., Becchio, C., and Schilbach, L. (2017). Beyond autism: introducing the dialectical misattunement hypothesis and a Bayesian account of intersubjectivity. Psychopathology 50, 355–372. doi: 10.1159/000484353
Breazeal, C., and Scassellati, B. (2002). Robots that imitate humans. Trends Cogn. Sci. 6, 481–487. doi: 10.1016/S1364-6613(02)02016-8
Bruineberg, J., Kiverstein, J., and Rietveld, E. (2018). The anticipating brain is not a scientist: the free-energy principle from an ecological-enactive perspective. Synthese 195, 2417–2444. doi: 10.1007/s11229-016-1239-1
Buccino, G., Binkofski, F., and Riggio, L. (2004a). The mirror neuron system and action recognition. Brain Lang. 89, 370–376. doi: 10.1016/S0093-934X(03)00356-0
Buccino, G., Lui, F., Canessa, N., Patteri, I., Lagravinese, G., Benuzzi, F., et al. (2004b). Neural circuits involved in the recognition of actions performed by nonconspecifics: an fMRI study. J. Cogn. Neurosci. 16, 114–126. doi: 10.1162/089892904322755601
Bucher, D., Haspel, G., Golowasch, J., and Nadim, F. (2015). “Central pattern generators,” in eLS (Chichester: John Wiley & Sons, Ltd.), 1–12. doi: 10.1002/9780470015902.a0000032.pub2
Buschmann, T., Ewald, A., von Twickel, A., and Büschges, A. (2015). Controlling legs for locomotion-insights from robotics and neurobiology. Bioinspir. Biomim. 10:041001. doi: 10.1088/1748-3190/10/4/041001
Calvo-Merino, B., Glaser, D. E., Grèzes, J., Passingham, R. E., and Haggard, P. (2005). Action observation and acquired motor skills: an FMRI study with expert dancers. Cereb. Cortex 15, 1243–1249. doi: 10.1093/cercor/bhi007
Calvo-Merino, B., Jola, C., Glaser, D. E., and Haggard, P. (2008). Towards a sensorimotor aesthetics of performing art. Conscious Cogn. 17, 911–922.
Chartrand, T. L., and Van Baaren, R. (2009). Human mimicry. Adv. Exp. Soc. Psychol. 41, 219–274. doi: 10.1016/S0065-2601(08)00405-X
Chen, P., Hendrikse, S., Sargent, K., Romani, M., Oostrik, M., Wilderjans, T. F., et al. (2021). Hybrid harmony: a multi-person neurofeedback application for interpersonal synchrony. Front. Neuroergonom. 21, 1–13. doi: 10.3389/fnrgo.2021.687108
Clark, A., and Chalmers, D. (1998). The extended mind. Analysis 58, 7–19. doi: 10.1093/analys/58.1.7
Clayton, M., Sager, R., and Will, U. (2005). “In time with the music: the concept of entrainment and its significance for ethnomusicology,” in European Meetings in Ethnomusicology, Vol. 11 (Druskininkai, Lithuania: Romanian Society for Ethnomusicology), 1–82.
Coffey, E. B., Arseneau-Bruneau, I., Zhang, X., Baillet, S., and Zatorre, R. J. (2021). Oscillatory entrainment of the frequency-following response in auditory cortical and subcortical structures. J. Neurosci. 41, 4073–4087. doi: 10.1523/JNEUROSCI.2313-20.2021
Coffey, E. B., Nicol, T., White-Schwoch, T., Chandrasekaran, B., Krizman, J., Skoe, E., et al. (2019). Evolving perspectives on the sources of the frequency-following response. Nat. Commun. 10, 1–10. doi: 10.1038/s41467-019-13003-w
Cohen, S. S., Henin, S., and Parra, L. C. (2017). Engaging narratives evoke similar neural activity and lead to similar time perception. Sci. Rep. 7, 1–10. doi: 10.1038/s41598-017-04402-4
Cohen, S. S., Madsen, J., Touchan, G., Robles, D., Lima, S. F., Henin, S., et al. (2018). Neural engagement with online educational videos predicts learning performance for individual students. Neurobiol. Learn. Mem. 155, 60–64. doi: 10.1016/j.nlm.2018.06.011
Condon, W. S., and Ogston, W. D. (1966). Sound film analysis of normal and pathological behavior patterns. J. Nervous Mental Dis. 143, 338–347. doi: 10.1097/00005053-196610000-00005
Cook, P., Rouse, A., Wilson, M., and Reichmuth, C. (2013). A California sea lion (Zalophus californianus) can keep the beat: motor entrainment to rhythmic auditory stimuli in a non vocal mimic. J. Comp. Psychol. 127, 412. doi: 10.1037/a0032345
Cracco, E., Lee, H., van Belle, G., Quenon, L., Haggard, P., Rossion, B., et al. (2021). EEG frequency tagging reveals the integration of form and motion cues into the perception of group movement. Cerebral Cortex. 1–15 doi: 10.1093/cercor/bhab385
Crick, C., Munz, M., and Scassellati, B. (2006). “Synchronization in social tasks: robotic drumming,” in IEEE International Symposium on Robot and Human Interactive Communication (Hatfield, UK), 97–102.
Crooker, S. A., Awschalom, D. D., Baumberg, J. J., Flack, F., and Samarth, N. (1997). Optical spin resonance and transverse spin relaxation in magnetic semiconductor quantum wells. Phys. Rev. B 56, 7574. doi: 10.1103/PhysRevB.56.7574
Cross, E. S., Hamilton, A. F., de, C., and Grafton, S. T. (2006). Building a motor simulation de novo: observation of dance by dancers. Neuroimage 31, 1257–1267. doi: 10.1016/j.neuroimage.2006.01.033
Cross, E. S., Kirsch, L., Ticini, L. F., and Schütz-Bosbach, S. (2011). The impact of aesthetic evaluation and physical ability on dance perception. Front. Hum. Neurosci. 5, 102. doi: 10.3389/fnhum.2011.00102
Cross, E. S., Liepelt, R., de C. Hamilton, A. F., Parkinson, J., Ramsey, R., Stadler, W., et al. (2012). Robotic movement preferentially engages the action observation network. Hum. Brain Mapp. 33, 2238–2254. doi: 10.1002/hbm.21361
Cross, E. S., and Ramsey, R. (2021). Mind meets machine: towards a cognitive science of human-machine interactions. Trends Cogn. Sci. 25, 200–212. doi: 10.1016/j.tics.2020.11.009
Csaba, G., and Porod, W. (2020). Coupled oscillators for computing: a review and perspective. Appl. Phys. Rev. 7, 011302. doi: 10.1063/1.5120412
Cunneen, C. (2019). “The political resonance of crime control strategies: zero tolerance policing,” in Hard Lessons, eds. R. Hill and G. Tait (New York, NY: Routledge), 151–169.
Czeszumski, A., Eustergerling, S., Lang, A., Menrath, D., Gerstenberger, M., Schuberth, S., et al. (2020). Hyperscanning: a valid method to study neural inter-brain underpinnings of social interaction. Front. Hum. Neurosci. 14, 39. doi: 10.3389/fnhum.2020.00039
Dahm, A. J., Denenstein, A., Finnegan, T. F., Langenberg, D. N., and Scalapino, D. J. (1968). Study of the Josephson plasma resonance. Phys. Rev. Lett. 20, 859. doi: 10.1103/PhysRevLett.20.859
Dallard, P., Fitzpatrick, A. J., Flint, A., Le Bourva, S., Low, A., Ridsdill Smith, R. M., et al. (2001). The London millennium footbridge. Struct. Eng. 79, 17–21.
Damm, L., Varoqui, D., De Cock, V. C., Dalla Bella, S., and Bardy, B. (2020). Why do we move to the beat? A multi-scale approach, from physical principles to brain dynamics. Neurosci. Biobehav. Rev. 112, 553–584. doi: 10.1016/j.neubiorev.2019.12.024
Das, A., Rathour, R. K., and Narayanan, R. (2017). Strings on a violin: location dependence of frequency tuning in active dendrites. Front. Cell. Neurosci. 11, 72. doi: 10.3389/fncel.2017.00072
Dauer, T., Nguyen, D. T., Gang, N., Dmochowski, J. P., Berger, J., and Kaneshiro, B. (2021). Inter-subject correlation while listening to minimalist music: a study of electrophysiological and behavioral responses to steve reich's piano phase. Front. Neurosci. 15, 702067. doi: 10.3389/fnins.2021.702067
Decety, J. (2010). The neurodevelopment of empathy in humans. Dev. Neurosci. 32, 257–267. doi: 10.1159/000317771
Decety, J., and Meyer, M. (2008). From emotion resonance to empathic understanding: a social developmental neuroscience account. Dev. Psychopathol. 20, 1053–1080. doi: 10.1017/S0954579408000503
Delaherche, E., Chetouani, M., Mahdhaoui, A., Saint-Georges, C., Viaux, S., and Cohen, D. (2012). Interpersonal synchrony: a survey of evaluation methods across disciplines. IEEE Trans. Affect. Comput. 3, 349–365. doi: 10.1109/T-AFFC.2012.12
Diep, C., Ftouni, S., Manousakis, J. E., Nicholas, C. L., Drummond, S. P., and Anderson, C. (2020). Acoustic slow wave sleep enhancement via a novel, automated device improves executive function in middle-aged men. Sleep 43, 197. doi: 10.1093/sleep/zsz197
Dikker, S., Michalareas, G., Oostrik, M., Serafimaki, A., Kahraman, H. M., Struiksma, M. E., et al. (2021). Crowdsourcing neuroscience: inter-brain coupling during face-to-face interactions outside the laboratory. Neuroimage 227, 117436. doi: 10.1016/j.neuroimage.2020.117436
Dikker, S., Montgomery, S., and Tunca, S. (2019). “Using synchrony-based neurofeedback in search of human connectedness,” in Brain Art, ed. A. Nijholt (Cham: Springer), 161–206.
Dikker, S., Wan, L., Davidesco, I., Kaggen, L., Oostrik, M., McClintock, J., et al. (2017). Brain-to-brain synchrony tracks real-world dynamic group interactions in the classroom. Curr. Biol. 27, 1375–1380. doi: 10.1016/j.cub.2017.04.002
Dmochowski, J. P., Bezdek, M. A., Abelson, B. P., Johnson, J. S., Schumacher, E. H., and Parra, L. C. (2014). Audience preferences are predicted by temporal reliability of neural processing. Nat. Commun. 5, 1–9. doi: 10.1038/ncomms5567
Dmochowski, J. P., Ki, J. J., DeGuzman, P., Sajda, P., and Parra, L. C. (2018). Extracting multidimensional stimulus-response correlations using hybrid encoding-decoding of neural activity. Neuroimage 180, 134–146. doi: 10.1016/j.neuroimage.2017.05.037
Dmochowski, J. P., Sajda, P., Dias, J., and Parra, L. C. (2012). Correlated components of ongoing EEG point to emotionally laden attention-a possible marker of engagement?. Front. Hum. Neurosci. 6, 112. doi: 10.3389/fnhum.2012.00112
Dong, P., Dai, X., and Wyer Jr, R. S. (2015). Actors conform, observers react: the effects of behavioral synchrony on conformity. J. Pers. Soc. Psychol. 108, 60. doi: 10.1037/pspi0000001
Dragan, A. D., Bauman, S., Forlizzi, J., and Srinivasa, S. S. (2015). “Effects of robot motion on human-robot collaboration,” in 2015 10th ACM/IEEE International Conference on Human-Robot Interaction (HRI) (Portland, OR: IEEE), 51–58.
Duan, Z., Wei, Z., Huang, S., Wang, Y., Sun, C., Tao, Y., et al. (2021). Resonance in atomic-scale sliding friction. Nano Lett. 21, 4615–4621. doi: 10.1021/acs.nanolett.1c00622
Duarte, N. (2013). Resonate: Present Visual Stories That Transform Audiences. Hoboken, NJ: John Wiley & Sons.
Duarte, N. F., Raković, M., and Santos-Victor, J. (2021). “Learning motor resonance in human-human and human-robot interaction with coupled dynamical systems,” in 2021 IEEE International Conference on Robotics and Automation (ICRA) (Xi'an, China: IEEE), 3662–3668.
Dumas, G., and Fairhurst, M. T. (2021). Reciprocity and alignment: quantifying coupling in dynamic interactions. R. Soc. Open Sci. 8, 210138. doi: 10.1098/rsos.210138
Dumas, G., Moreau, Q., Tognoli, E., and Kelso, J. S. (2020). The human dynamic clamp reveals the fronto-parietal network linking real-time social coordination and cognition. Cereb. Cortex 30, 3271–3285. doi: 10.1093/cercor/bhz308
Dumas, G., Nadel, J., Soussignan, R., Martinerie, J., and Garnero, L. (2010). Inter-brain synchronization during social interaction. PLoS ONE 5, e12166. doi: 10.1371/journal.pone.0012166
Ehrenreich, B. (2009). Bright-Sided: How Positive Thinking is Undermined America. New York, NY: Metropolitan Books.
Endo, G., Morimoto, J., Matsubara, T., Nakanishi, J., and Cheng, G. (2008). Learning CPG-based biped locomotion with a policy gradient method: application to a humanoid robot. Int. J. Rob. Res. 27, 213–228. doi: 10.1177/0278364907084980
Engert, V., Linz, R., and Grant, J. A. (2019). Embodied stress: the physiological resonance of psychosocial stress. Psychoneuroendocrinology 105, 138–146. doi: 10.1016/j.psyneuen.2018.12.221
Fauché, P., Kosionis, S. G., and Lalanne, P. (2017). Collective scattering in hybrid nanostructures with many atomic oscillators coupled to an electromagnetic resonance. Phys. Rev. B 95, 195418. doi: 10.1103/PhysRevB.95.195418
Feldman, R. (2012). Bio-behavioral synchrony: a model for integrating biological and microsocial behavioral processes in the study of parenting. Parenting 12, 154–164. doi: 10.1080/15295192.2012.683342
Feldman, R. (2017). The neurobiology of human attachments. Trends Cogn. Sci. 21, 80–99. doi: 10.1016/j.tics.2016.11.007
Ferreira, D., and Vale, M. (2020). Geography in the big data age: an overview of the historical resonance of current debates. Geograph. Rev. 122, 1–17. doi: 10.1080/00167428.2020.1832424
Forlizzi, J. (2007). “How robotic products become social products: an ethnographic study of cleaning in the home,” in 2007 2nd ACM/IEEE International Conference on Human-Robot Interaction (HRI) (Arlington, VA: IEEE), 129–136.
Freeman, L. M., Pang, L., and Fainman, Y. (2014). Maximizing the electromagnetic and chemical resonances of surface-enhanced Raman scattering for nucleic acids. ACS Nano 8, 8383–8391. doi: 10.1021/nn5028664
Fujiwara, K., Hoegen, R., Gratch, J., and Dunbar, N. E. (2021). Synchrony facilitates altruistic decision making for non-human avatars. Comput. Human Behav. 128, 107079. doi: 10.1016/j.chb.2021.107079
Fujiwara, K., and Yokomitsu, K. (2021). Video-based tracking approach for nonverbal synchrony: a comparison of Motion Energy Analysis and OpenPose. Behav. Res. Methods 53, 2700–2711. doi: 10.3758/s13428-021-01612-7
Gallese, V. (2009). Mirror neurons, embodied simulation, and the neural basis of social identification. Psychoanal. Dialogues 19, 519–536. doi: 10.1080/10481880903231910
Gallese, V., and Sinigaglia, C. (2018). “Embodied resonance,” in The Oxford Handbook of 4e Cognition, eds. A. Newen, L. De Bruin, and S. Gallagher (Oxford, UK: Oxford University Press), 417–432.
Gardner, T., Aglinskas, A., and Cross, E. S. (2017). Using guitar learning to probe the Action Observation Network's response to visuomotor familiarity. Neuroimage 156, 174–189. doi: 10.1016/j.neuroimage.2017.04.060
Garrett, C. (1972). Tidal resonance in the Bay of Fundy and Gulf of Maine. Nature 238, 441–443. doi: 10.1038/238441a0
Gelfand, M. J., Caluori, N., Jackson, J. C., and Taylor, M. K. (2020). The cultural evolutionary trade-off of ritualistic synchrony. Philos. Trans. R. Soc. B 375, 20190432. doi: 10.1098/rstb.2019.0432
Gibbs, A. (2019). “Put a spell on you: Poetry, politics, and affective resonance in the age of the algorithm,” in Public Spheres of Resonance, eds. A. Fleig and C. von Scheve (New York, NY: Routledge), 117–134.
Gill, S. P. (2012). Rhythmic synchrony and mediated interaction: towards a framework of rhythm in embodied interaction. AI Soc. 27, 111–127. doi: 10.1007/s00146-011-0362-2
Giorgi, S. (2017). The mind and heart of resonance: the role of cognition and emotions in frame effectiveness. J. Manage. Stud. 54, 711–738. doi: 10.1111/joms.12278
Grafsgaard, J., Duran, N., Randall, A., Tao, C., and D'Mello, S. (2018). “Generative multimodal models of nonverbal synchrony in close relationships,” in 2018 13th IEEE International Conference on Automatic Face & Gesture Recognition (FG 2018) (Xi'an: IEEE), 195–202.
Grammer, K., Kruck, K. B., and Magnusson, M. S. (1998). The courtship dance: patterns of nonverbal synchronization in opposite-sex encounters. J. Nonverbal Behav. 22, 3–29. doi: 10.1023/A:1022986608835
Gratch, J., Kang, S. H., and Wang, N. (2013). Using social agents to explore theories of rapport and emotional resonance. Soc. Emotions Nat. Artifact 181, 2568173. doi: 10.1093/acprof:oso/9780195387643.003.0012
Gratch, J., and Lucas, G. (2021). “Rapport between humans and socially interactive agents,” in The Handbook on Socially Interactive Agents: 20 years of Research on Embodied Conversational Agents, Intelligent Virtual Agents, and Social Robotics Volume 1: Methods, Behavior, Cognition, eds. B. Lugrin, C. Pelachaud, and D. Traum (New York, NY: Association for Computing Machinery), 433–462. doi: 10.1145/3477322.3477335
Greenberg, N. S. (2019). Resonance and behaviors toward others are predicted by perceived warmth and competence of target (Doctoral dissertation). Waltham, MA: Brandeis University.
Greenfield, M. D., Honing, H., Kotz, S. A., and Ravignani, A. (2021). Synchrony and rhythm interaction: from the brain to behavioural ecology. Philos. Trans. R. Soc. B 376, 20200324. doi: 10.1098/rstb.2020.0324
Grimes, C. A., Mungle, C. S., Zeng, K., Jain, M. K., Dreschel, W. R., Paulose, M., et al. (2002). Wireless magnetoelastic resonance sensors: a critical review. Sensors 2, 294–313. doi: 10.3390/s20700294
Grossberg, S. (2013). Adaptive resonance theory: how a brain learns to consciously attend, learn, and recognize a changing world. Neural Netw. 37, 1–47. doi: 10.1016/j.neunet.2012.09.017
Grossberg, S. (2017). Towards solving the hard problem of consciousness: the varieties of brain resonances and the conscious experiences that they support. Neural Netw. 87, 38–95. doi: 10.1016/j.neunet.2016.11.003
Guertin, P. A. (2013). Central pattern generator for locomotion: anatomical, physiological, and pathophysiological considerations. Front. Neurol. 3, 183. doi: 10.3389/fneur.2012.00183
Guevara Erra, R., Perez Velazquez, J. L., and Rosenblum, M. (2017). Neural synchronization from the perspective of non-linear dynamics. Front. Comput. Neurosci. 11, 98. doi: 10.3389/fncom.2017.00098
Gutsell, J. N., and Inzlicht, M. (2010). Empathy constrained: prejudice predicts reduced mental simulation of actions during observation of outgroups. J. Exp. Soc. Psychol. 46, 841–845. doi: 10.1016/j.jesp.2010.03.011
Gutterman, E. (2015). Easier done than said: transnational bribery, norm resonance, and the origins of the US Foreign Corrupt Practices Act. Foreign Policy Anal. 11, 109–128. doi: 10.1111/fpa.12027
Hall, J. A., and Schwartz, R. (2019). Empathy present and future. J. Soc. Psychol. 159, 225–243. doi: 10.1080/00224545.2018.1477442
Han, F., Caporale, N., and Dan, Y. (2008). Reverberation of recent visual experience in spontaneous cortical waves. Neuron 60, 321–327. doi: 10.1016/j.neuron.2008.08.026
Hanslmayr, S., Axmacher, N., and Inman, C. S. (2019). Modulating human memory via entrainment of brain oscillations. Trends Neurosci. 42, 485–499.
Hanson, L. (2009). Second-stage labor care: challenges in spontaneous bearing down. J. Perinat. Neonatal Nurs. 23, 31–39. doi: 10.1097/JPN.0b013e318196526b
Hanuschkin, A., Ganguli, S., and Hahnloser, R. (2013). A Hebbian learning rule gives rise to mirror neurons and links them to control theoretic inverse models. Front. Neural Circuits 7, 106. doi: 10.3389/fncir.2013.00106
Hasegawa, A., Okanoya, K., Hasegawa, T., and Seki, Y. (2011). Rhythmic synchronization tapping to an audio-visual metronome in budgerigars. Sci. Rep. 1, 1–8. doi: 10.1038/srep00120
Hasson, U., and Frith, C. D. (2016). Mirroring and beyond: coupled dynamics as a generalized framework for modelling social interactions. Philos. Trans. R. Soc. B 371, 20150366. doi: 10.1098/rstb.2015.0366
Hattori, Y., and Tomonaga, M. (2020). Rhythmic swaying induced by sound in chimpanzees (Pan troglodytes). Proc. Nat. Acad. Sci. U.S.A. 117, 936–942. doi: 10.1073/pnas.1910318116
Hebb, D. O. (2005). The Organization of Behavior: A Neuropsychological Theory. New York, NY: Psychology Press.
Hekkert, P. (2014). “Aesthetic responses to design: a battle of impulses,” in The Cambridge Handbook of the Psychology of Aesthetics and the Arts, eds P. P. L. Tinio and J. K. Smith (Cambridge, UK: Cambridge University Press), 277–299.
Hekkert, P., Snelders, D., and Van Wieringen, P. C. (2003). 'Most advanced, yet acceptable': typicality and novelty as joint predictors of aesthetic preference in industrial design. Br. J. Psychol. 94, 111–124. doi: 10.1348/000712603762842147
Helfrich, R. F., Breska, A., and Knight, R. T. (2019). Neural entrainment and network resonance in support of top-down guided attention. Curr. Opin. Psychol. 29, 82–89. doi: 10.1016/j.copsyc.2018.12.016
Helmholtz, H. L. (2009). On the Sensations of Tone as a Physiological Basis for the Theory of Music. Cambridge, UK: Cambridge University Press.
Henschel, A., and Cross, E. S. (2020). No evidence for enhanced likeability and social motivation towards robots after synchrony experience. Interact. Stud. 21, 7–23. doi: 10.1075/is.19004.hen
Henschel, A., Hortensius, R., and Cross, E. S. (2020). Social cognition in the age of human-robot interaction. Trends Neurosci. 43, 373–384. doi: 10.1016/j.tins.2020.03.013
Hernandez-Lallement, J., Gómez-Sotres, P., and Carrillo, M. (2020). Towards a unified theory of emotional contagion in rodents-A meta-analysis. Neurosci. Biobehav. Rev. 132, 1229–1248. doi: 10.1016/j.neubiorev.2020.09.010
Herrmann, C. S. (2001). Human EEG responses to 1-100 Hz flicker: resonance phenomena in visual cortex and their potential correlation to cognitive phenomena. Exp. Brain Res. 137, 346–353. doi: 10.1007/s002210100682
Hicks, E., and Hicks, J. (2006). The Law of Attraction: The Basics of the Teachings of Abraham. London, UK: Hay House, Inc.
Himberg, T., Laroche, J., Bigé, R., Buchkowski, M., and Bachrach, A. (2018). Coordinated interpersonal behaviour in collective dance improvisation: the aesthetics of kinaesthetic togetherness. Behav. Sci. 8, 23. doi: 10.3390/bs8020023
Hirao, M., and Ogi, H. (1997). Electromagnetic acoustic resonance and materials characterization. Ultrasonics 35, 413–421. doi: 10.1016/S0041-624X(97)00030-9
Hoffman, G. (2019). Evaluating fluency in human-robot collaboration. IEEE Trans. Human Mach. Syst. 49, 209–218. doi: 10.1109/THMS.2019.2904558
Hoffman, G., and Ju, W. (2014). Designing robots with movement in mind. J. Human Robot Interact. 3, 91–122. doi: 10.5898/JHRI.3.1.Hoffman
Hoffman, G., and Weinberg, G. (2010). “Shimon: an interactive improvisational robotic marimba player,” in CHI'10 Extended Abstracts on Human Factors in Computing Systems (Atlanta, GA), 3097–3102.
Hofree, G., Ruvolo, P., Bartlett, M. S., and Winkielman, P. (2014). Bridging the mechanical and the human mind: spontaneous mimicry of a physically present android. PLoS ONE 9, e99934. doi: 10.1371/journal.pone.0099934
Hofree, G., Ruvolo, P., Reinert, A., Bartlett, M. S., and Winkielman, P. (2018). Behind the robot's smiles and frowns: in social context, people do not mirror android's expressions but react to their informational value. Front. Neurorobot. 12, 14. doi: 10.3389/fnbot.2018.00014
Holyoak, K. J., and Stamenković, D. (2018). Metaphor comprehension: a critical review of theories and evidence. Psychol. Bull. 144, 641. doi: 10.1037/bul0000145
Hove, M. J., and Risen, J. L. (2009). It's all in the timing: interpersonal synchrony increases affiliation. Soc. Cogn. 27, 949–960. doi: 10.1521/soco.2009.27.6.949
Howie, P. C., and Bagnall, R. G. (2020). A criterial framework for concept evaluation in social practice and scholarship. Sociol. Methods Res. 49, 719–741. doi: 10.1177/0049124118769104
Hughes, J. (2012). “Compassionate AI and selfless robots: a buddhist approach,” Robot Ethics: The Ethical and Social Implications of Robotics eds. P. Lin, K. Abney, and G. A. Bekey (Cambridge, MA: MIT Press), 69–83.
Hummels, C. C. M., Ross, P. R., and Overbeeke, C. J. (2003). “In search of resonant human computer interaction: building and testing aesthetic installations,” in Proceedings of the 9th IFIP Conference on Human-Computer Interaction (Zurich), 399–406.
Hunt, T., and Schooler, J. W. (2019). The easy part of the hard problem: a resonance theory of consciousness. Front. Hum. Neurosci. 13, 378. doi: 10.3389/fnhum.2019.00378
Hurley, S. (2008). The shared circuits model (SCM): how control, mirroring, and simulation can enable imitation, deliberation, and mindreading. Behav. Brain Sci. 31, 1–22. doi: 10.1017/S0140525X07003123
Hutchins, E. (2001). “Distributed cognition,” in International Encyclopedia of the Social and Behavioral Sciences, eds. N. J. Smelser and P. B. Baltes (Amsterdam, NL: Elsevier Science), 138.
Iacoboni, M. (2009). Imitation, empathy, and mirror neurons. Annu. Rev. Psychol. 60, 653–670. doi: 10.1146/annurev.psych.60.110707.163604
Iio, T., Shiomi, M., Shinozawa, K., Akimoto, T., Shimohara, K., and Hagita, N. (2011). Investigating entrainment of people's pointing gestures by robot's gestures using a WOZ method. Int. J. Soc. Robot. 3, 405–414. doi: 10.1007/s12369-011-0112-0
Iio, T., Shiomi, M., Shinozawa, K., Shimohara, K., Miki, M., and Hagita, N. (2015). Lexical entrainment in human robot interaction. Int. J. Soc. Robot. 7, 253–263. doi: 10.1007/s12369-014-0255-x
Ijspeert, A. J. (2008). Central pattern generators for locomotion control in animals and robots: a review. Neural Netw. 21, 642–653. doi: 10.1016/j.neunet.2008.03.014
Iqbal, T., and Riek, L. D. (2021). “Temporal anticipation and adaptation methods for fluent human-robot teaming,” in 2021 IEEE International Conference on Robotics and Automation (ICRA) (IEEE), 3736–3743.
Iversen, J. R., Patel, A. D., Nicodemus, B., and Emmorey, K. (2015). Synchronization to auditory and visual rhythms in hearing and deaf individuals. Cognition 134, 232–244. doi: 10.1016/j.cognition.2014.10.018
Jiang, L. (2020, October 22). Reasons the largest chinese restaurant in the netherlands uses the pudu robotics food delivery robot. DutchNews.nl. Available online at: https://www.dutchnews.nl/news/businesswire/reasons-the-largest-chinese-restaurant-in-the-netherlands-uses-the-pudu-robotics-food-delivery-robot-2/
Jouaiti, M., and Henaff, P. (2018). “CPG-based controllers can trigger the emergence of social synchrony in human-robot interactions,” in 2018 IEEE Workshop on Advanced Robotics and its Social Impacts (ARSO) (Genoa, Italy: IEEE), 71–76.
Kaneshiro, B., Nguyen, D. T., Norcia, A. M., Dmochowski, J. P., and Berger, J. (2020). Natural music evokes correlated EEG responses reflecting temporal structure and beat. Neuroimage 214, 116559. doi: 10.1016/j.neuroimage.2020.116559
Kaneshiro, B., Nguyen, D. T., Norcia, A. M., Dmochowski, J. P., and Berger, J. (2021). Inter-subject EEG correlation reflects time-varying engagement with natural music. bioRxiv. 2021, 1–32. doi: 10.1101/2021.04.14.439913
Katz, B. F. (1995). Harmonic resolution, neural resonance, and positive affect. Music Percept. 13, 79–108. doi: 10.2307/40285686
Keller, K. L. (2010). Brand equity management in a multichannel, multimedia retail environment. J. Interact. Market. 24, 58–70. doi: 10.1016/j.intmar.2010.03.001
Kendon, A. (1970). Movement coordination in social interaction: some examples described. Acta Psychol. 32, 101–125. doi: 10.1016/0001-6918(70)90094-6
Keysers, C., and Gazzola, V. (2009). Expanding the mirror: vicarious activity for actions, emotions, and sensations. Curr. Opin. Neurobiol. 19, 666–671. doi: 10.1016/j.conb.2009.10.006
Keysers, C., and Gazzola, V. (2014). Hebbian learning and predictive mirror neurons for actions, sensations and emotions. Philos. Trans. R. Soc. B 369, 20130175. doi: 10.1098/rstb.2013.0175
Kingsbury, L., and Hong, W. (2020). A multi-brain framework for social interaction. Trends Neurosci. 43, 651–666. doi: 10.1016/j.tins.2020.06.008
Kinreich, S., Djalovski, A., Kraus, L., Louzoun, Y., and Feldman, R. (2017). Brain-to-brain synchrony during naturalistic social interactions. Sci. Rep. 7, 1–12. doi: 10.1038/s41598-017-17339-5
Kirkwood, G. L., Otmar, C. D., and Hansia, M. (2021). Who's leading this dance?: Theorizing automatic and strategic synchrony in human-exoskeleton interactions. Front. Psychol. 12, 624108. doi: 10.3389/fpsyg.2021.624108
Kirsch, L. P., Snagg, A., Heerey, E., and Cross, E. S. (2016a). The impact of experience on affective responses during action observation. PLoS ONE 11, e0154681. doi: 10.1371/journal.pone.0154681
Kirsch, L. P., Urgesi, C., and Cross, E. S. (2016b). Shaping and reshaping the aesthetic brain: Emerging perspectives on the neurobiology of embodied aesthetics. Neurosci. Biobehav. Rev. 62, 56–68. doi: 10.1016/j.neubiorev.2015.12.005
Kirschner, S., and Tomasello, M. (2009). Joint drumming: social context facilitates synchronization in preschool children. J. Exp. Child Psychol. 102, 299–314. doi: 10.1016/j.jecp.2008.07.005
Klimesch, W. (2018). The frequency architecture of brain and brain body oscillations: an analysis. Euro. J. Neurosci. 48, 2431–2453. doi: 10.1111/ejn.14192
Knight, R. T., and Nakada, T. (1998). Cortico-limbic circuits and novelty: a review of EEG and blood flow data. Rev. Neurosci. 9, 57–70. doi: 10.1515/REVNEURO.1998.9.1.57
Koban, L., Ramamoorthy, A., and Konvalinka, I. (2019). Why do we fall into sync with others? Interpersonal synchronization and the brain's optimization principle. Soc. Neurosci. 14, 1–9. doi: 10.1080/17470919.2017.1400463
Kopp, S. (2010). Social resonance and embodied coordination in face-to-face conversation with artificial interlocutors. Speech Commun. 52, 587–597. doi: 10.1016/j.specom.2010.02.007
Kozima, H., Michalowski, M. P., and Nakagawa, C. (2009). Keepon. Int. J. Soc. Robot. 1, 3–18. doi: 10.1007/s12369-008-0009-8
Kühne, V., Rosenthal-von der Pütten, A. M., and Krämer, N. C. (2013). “Using linguistic alignment to enhance learning experience with pedagogical agents: the special case of dialect,” in International Workshop on Intelligent Virtual Agents, eds. R. Aylett, B. Krenn, C. Pelachaud, and H. Shimodaira (Berlin: Springer), 149–158.
Kumagai, Y., Matsui, R., and Tanaka, T. (2018). Music familiarity affects EEG entrainment when little attention is paid. Front. Hum. Neurosci. 12, 444. doi: 10.3389/fnhum.2018.00444
Lakatos, P., Joachim, G., and Gregor, T. (2019). A new unifying account of the roles of neuronal entrainment. Curr. Biol. 29, R890–R905. doi: 10.1016/j.cub.2019.07.075
Lakoff, G., and Johnson, M. (1980). The metaphorical structure of the human conceptual system. Cogn. Sci. 4, 195–208. doi: 10.1207/s15516709cog0402_4
Lampl, I., and Yarom, Y. (1997). Subthreshold oscillations and resonant behavior: two manifestations of the same mechanism. Neuroscience 78, 325–341. doi: 10.1016/S0306-4522(96)00588-X
Large, E. W. (2010). “Neurodynamics of music,” in Music Perception, eds. M. R. Jones, R. R. Fay, A. N. Popper (New York, NY: Springer), 201–231.
Large, E. W., and Snyder, J. S. (2009). Pulse and meter as neural resonance. Ann. N. Y. Acad. Sci. 1169, 46–57. doi: 10.1111/j.1749-6632.2009.04550.x
Larsson, M., Richter, J., and Ravignani, A. (2019). Bipedal steps in the development of rhythmic behavior in humans. Music Sci. 2, 2059204319892617. doi: 10.1177/2059204319892617
Lassiter, J. B., Sobhani, H., Fan, J. A., Kundu, J., Capasso, F., Nordlander, P., et al. (2010). Fano resonances in plasmonic nanoclusters: geometrical and chemical tunability. Nano Lett. 10, 3184–3189. doi: 10.1021/nl102108u
Lee, C.-C., Black, M., Katsamanis, A., Lammert, A., Baucom, B., Christensen, A., et al. (2010). Quantification of prosodic entrainment in affective spontaneous spoken interactions in married couples, in Proceedings of InterSpeech (Makuhari: International Speech Communication Association), 793–796.
Lee, C. S., Kao, Y. F., Kuo, Y. H., and Wang, M. H. (2007). Automated ontology construction for unstructured text documents. Data Knowl. Eng. 60, 547–566. doi: 10.1016/j.datak.2006.04.001
Lee, M. K., Forlizzi, J., Rybski, P. E., Crabbe, F., Chung, W., Finkle, J., Glaser, E., and Kiesler, S. (2009). “The snackbot: documenting the design of a robot for long-term human-robot interaction,” in Proceedings of the 4th ACM/IEEE International Conference on Human Robot Interaction (La Jolla, CA), 7–14.
Lehar, S. (2003). Harmonic resonance theory: an alternative to the “Neuron Doctrine” paradigm of neurocomputation to address gestalt properties of perception. Behav. Brain Sci. 26, 15. doi: 10.1017/S0140525X03000098
Levantino, V. (2018). BAO-ME: design of a huggable device to improve mental well-being through haptic interactions (Keio University Master's Thesis). Minato, Tokyo, Japan: Sugiura, Kazunori杉浦,一Publisher塾大学大学院メディアデザイン研究科.
Li, J., Liu, Q., Zhai, Y., Molinas, M., and Wu, M. (2020). Analysis of harmonic resonance for locomotive and traction network interacted system considering the frequency-domain passivity properties of the digitally controlled converter. Front. Energy Res. 276, 1–16. doi: 10.3389/fenrg.2020.523333
Lim, V., Rooksby, M., and Cross, E. S. (2021). Social robots on a global stage: establishing a role for culture during human-robot interaction. Int. J. Soc. Robot. 13, 1307–1333. doi: 10.1007/s12369-020-00710-4
Lin, A., and Lomas, J. D. (2022). “Enigma of mind: a theory of evolution and conscious experience,” in Enigma: Darwin College Lecture Series, eds. E. Ward and J. Gibson (Cambridge: Cambridge University Press).
Lo, S. Y., and Huang, H. P. (2016). Realization of sign language motion using a dual-arm/hand humanoid robot. Intelligent Service Robot. 9, 333–345. doi: 10.1007/s11370-016-0203-8
Lobis, S. (2015). The Virtue of Sympathy: Magic, Philosophy, and Literature in Seventeenth-Century England (New Haven, CT: Yale University Press).
Lolo Nate (2022). 17 Weird to Wonderful Long Distance Relationship Gadgets. Available online at: https://lastingthedistance.com/long-distance-relationship-gadgets/
Lomas, J. D., Forlizzi, J., Poonwala, N., Patel, N., Shodhan, S., Patel, K., et al. (2016). “Interface design optimization as a multi-armed bandit problem,” in Proceedings of the 2016 CHI Conference on Human Factors in Computing Systems (San Jose, CA), 4142–4153.
Lomas, J. D., Karac, M., and Gielen, M. (2021). Design space cards: using a card deck to navigate the design space of interactive play. Proc. ACM Human Computer Interact. 5, 227. doi: 10.1145/3474654
Lomas, J. D., and Xue, H. (2022). Harmony in design: A synthesis of literature from classical philosophy, the sciences, economics, and design. She Ji: The Journal of Design, Economics, and Innovation 8, 5–64.
Lorenz, T., Weiss, A., and Hirche, S. (2016). Synchrony and reciprocity: key mechanisms for social companion robots in therapy and care. Int. J. Soc. Robot. 8, 125–143. doi: 10.1007/s12369-015-0325-8
Lupetti, M. L., and Van Mechelen, M. (2022). “Promoting children's critical thinking towards robotics through robot deception,” in Proceedings of the 2022 ACM/IEEE International Conference on Human-Robot Interaction (Sapporo, Hokkaido, Japan), 588–597.
MacDougall, H. G., and Moore, S. T. (2005). Marching to the beat of the same drummer: the spontaneous tempo of human locomotion. J. Appl. Physiol. 99, 1164–1173. doi: 10.1152/japplphysiol.00138.2005
Macik, M., Prazakova, K., Kutikova, A., Mikovec, Z., Adolf, J., Havlik, J., et al. (2017). “Breathing friend: tackling stress through portable tangible breathing artifact,” in IFIP Conference on Human-Computer Interaction (Cham: Springer), 106–115.
Madsen, J., Margulis, E. H., Simchy-Gross, R., and Parra, L. C. (2019). Music synchronizes brainwaves across listeners with strong effects of repetition, familiarity and training. Sci. Rep. 9, 1–8. doi: 10.1038/s41598-019-40254-w
Matheny, T., Poe, P., Fisher, M., and Warren, S. (2018). Chaos and stability in Donald Trump's acceptance speech. Media Watch 9, 260–266. doi: 10.15655/mw/2018/v9i3/49499
Mayo, O., and Gordon, I. (2020). In and out of synchrony-Behavioral and physiological dynamics of dyadic interpersonal coordination. Psychophysiology 57, e13574. doi: 10.1111/psyp.13574
McDonnell, T. E., Bail, C. A., and Tavory, I. (2017). A theory of resonance. Sociol. Theory 35, 1–14. doi: 10.1177/0735275117692837
McGarva, A. R., and Warner, R. M. (2003). Attraction and social coordination: mutual entrainment of vocal activity rhythms. J. Psycholinguist. Res. 32, 335–354. doi: 10.1023/A:1023547703110
McMillan, W. L., and Anderson, P. W. (1966). Theory of geometrical resonances in the tunneling characteristics of thick films of superconductors. Phys. Rev. Lett. 16, 85. doi: 10.1103/PhysRevLett.16.85
McQuarrie, E. F., and Mick, D. G. (1992). On resonance: a critical pluralistic inquiry into advertising rhetoric. J. Consumer Res. 19, 180–197. doi: 10.1086/209295
Melnyk, A., and Hénaff, P. (2019). Physical analysis of handshaking between humans: mutual synchronisation and social context. Int. J. Soc. Robot. 11, 541–554. doi: 10.1007/s12369-019-00525-y
Metzler, T. A., Lewis, L. M., and Pope, L. C. (2016). Could robots become authentic companions in nursing care?. Nurs. Philos. 17, 36–48. doi: 10.1111/nup.12101
Mintzberg, H., Quinn, J. B., and Voyer, J. (1995). The Strategy Process. Collegiate edition. Englewood Cliffs, NJ: Prentice-Hall.
Mohammadi-Khanaman, S., and Lundström, C. (2019). Somnox Care Robot: Development of a Soft Robot for Improved Sleep. Chalmers University of Technology Repository. Available online at: https://hdl.handle.net/20.500.12380/300447
Molkov, Y. I., Zoccal, D. B., Baekey, D. M., Abdala, A. P., Machado, B. H., Dick, T. E., et al. (2014). Physiological and pathophysiological interactions between the respiratory central pattern generator and the sympathetic nervous system. Prog. Brain Res. 212, 1–23. doi: 10.1016/B978-0-444-63488-7.00001-X
Moran, N., Pellegrino, D., Slingerland, J. K., and Kells, G. (2017). Parafermionic clock models and quantum resonance. Phys. Rev. B 95, 235127. doi: 10.1103/PhysRevB.95.235127
Moreau, Q., and Dumas, G. (2021). Beyond “correlation vs. causation”: multi-brain neuroscience needs explanation. Trends Cogn. Sci. 25, 542–543. doi: 10.1016/j.tics.2021.02.011
Morgan, R., Fischer, R., and Bulbulia, J. A. (2017). To be in synchrony or not? A meta-analysis of synchrony's effects on behavior, perception, cognition and affect. J. Exp. Soc. Psychol. 72, 13–20. doi: 10.1016/j.jesp.2017.03.009
Mutlu, B., Roy, N., and Šabanović, S. (2016). “Cognitive human-robot interaction,” in Springer Handbook of Robotics, eds. B. Siciliano and O. Khatib (Würzburg, Germany: Springer), 1907–1934.
New York Times (2021 December 9) Will Smith is Done Trying to Be Perfect. Available online at: https://www.nytimes.com/2021/12/09/magazine/will-smith-interview.html.
Northoff, G., Wainio-Theberge, S., and Evers, K. (2020). Is temporo-spatial dynamics the “common currency” of brain and mind? In Quest of “Spatiotemporal Neuroscience”. Phys. Life Rev. 33, 34–54. doi: 10.1016/j.plrev.2019.05.002
Oldenburg, S. J., Averitt, R. D., Westcott, S. L., and Halas, N. J. (1998). Nanoengineering of optical resonances. Chem. Phys. Lett. 288, 243–247. doi: 10.1016/S0009-2614(98)00277-2
O'Loughlin, H. (2018). Effect of a heart beating breathing doll on infant sleep (Master's Thesis). University of Canterbury. Available online at: http://hdl.handle.net/10092/15580
Orgs, G., Dombrowski, J., Heil, M., and Jansen-Osmann. (2008). Expertise in dance modulates alpha/beta event-related desynchronization during action observation. Euro. J. Neurosci. 27, 3380–3384. doi: 10.1111/j.1460-9568.2008.06271.x
Paladino, M.-P., Mazzurega, M., Pavani, F., and Schubert, T. W. (2010). Synchronous multisensory stimulation blurs self-other boundaries. Psychol. Sci. 21, 1202–1207. doi: 10.1177/0956797610379234
Pandey, P., Ahmad, N., Miyapuram, K. P., and Lomas, D. (2021). “Predicting dominant beat frequency from brain responses while listening to music,” in 2021 IEEE International Conference on Bioinformatics and Biomedicine (BIBM) (Houston, TX: IEEE), 3058–3064.
Patel, A., Iversen, J., Bregman, M., and Schulz, I. (2009). Studying synchronization to a musical beat in nonhuman animals. Ann. N. Y. Acad. Sci. 1169, 459–469. doi: 10.1111/j.1749-6632.2009.04581.x
Patel, A. D., and Iversen, J. R. (2014). The evolutionary neuroscience of musical beat perception: the Action Simulation for Auditory Prediction (ASAP) hypothesis. Front. Syst. Neurosci. 8, 57. doi: 10.3389/fnsys.2014.00057
Pérez, P., Madsen, J., Banellis, L., Türker, B., Raimondo, F., Perlbarg, V., et al. (2021). Conscious processing of narrative stimuli synchronizes heart rate between individuals. Cell Rep. 36, 109692. doi: 10.1016/j.celrep.2021.109692
Pikovsky, A., Rosenblum, M., and Kurths, J. (2001). Synchronization: A Universal Concept in Nonlinear Sciences. Cambridge, UK: Cambridge University Press.
Porter, M. E. (1991). Towards a dynamic theory of strategy. Strategic Manage. J. 12, 95–117. doi: 10.1002/smj.4250121008
Poshakinskiy, A. V., and Astakhov, G. V. (2019). Optically detected spin-mechanical resonance in silicon carbide membranes. Phys. Rev. B 100, 094104. doi: 10.1103/PhysRevB.100.094104
Prasad, L. (2019). Ethical resonance: the concept, the practice, and the narration. J. Religious Ethics 47, 394–415. doi: 10.1111/jore.12261
Preston, S. D., and De Waal, F. B. (2002). Empathy: Its ultimate and proximate bases. Behav. Brain Sci. 25, 1–20. doi: 10.1017/S0140525X02000018
Prochazkova, E., Sjak-Shie, E., Behrens, F., Lindh, D., and Kret, M. E. (2022). Physiological synchrony is associated with attraction in a blind date setting. Nat. Human Behav. 6, 269–278. doi: 10.1038/s41562-021-01197-3
Pulvermüller, F. (2018). Neural reuse of action perception circuits for language, concepts and communication. Progress Neurobiol. 160, 1–44. doi: 10.1016/j.pneurobio.2017.07.001
Quach, J. Q. (2016). Spin gravitational resonance and graviton detection. Phys. Rev. D 93, 104048. doi: 10.1103/PhysRevD.93.104048
Raja, V. (2018). A story of resonance (Doctoral Dissertation). Available online at: https://etd.ohiolink.edu/!etd.send_file?accession=ucin1530272507861696&disposition=inline
Raja, V. (2020). Resonance and radical embodiment. Synthese. 199 1–29. doi: 10.1007/s11229-020-02610-6
Rajasekar, S., and Sanjuan, M. A. (2016). Nonlinear Resonances. Switzerland: Springer International Publishing.
Reinero, D. A., Dikker, S., and Van Bavel, J. J. (2021). Inter-brain synchrony in teams predicts collective performance. Soc. Cogn. Affect. Neurosci. 16, 43–57. doi: 10.1093/scan/nsaa135
Reniers, R. L., Corcoran, R., Drake, R., Shryane, N. M., and Völlm, B. A. (2011). The QCAE: a questionnaire of cognitive and affective empathy. J. Pers. Assess. 93, 84–95. doi: 10.1080/00223891.2010.528484
Repp, B. H., and Su, Y. H. (2013). Sensorimotor synchronization: a review of recent research (2006-2012). Psychon. Bull. Rev. 20, 403–452. doi: 10.3758/s13423-012-0371-2
Roger, P. (2020). Toward a theory of resonance in cinema-On Claudia von Alemann's Le Voyage à Lyon. Écrans 2019, 65–78. doi: 10.15122/isbn.978-2-406-10499-5.p.0065
Roll, G., and Schweiger, G. (2000). Geometrical optics model of Mie resonances. JOSA A 17, 1301–1311. doi: 10.1364/JOSAA.17.001301
Ronsse, R., Vitiello, N., Lenzi, T., Van Den Kieboom, J., Carrozza, M. C., and Ijspeert, A. J. (2010). Human-robot synchrony: flexible assistance using adaptive oscillators. IEEE Trans. Biomed. Eng. 58, 1001–1012. doi: 10.1109/TBME.2010.2089629
Rosa, H. (2018). The idea of resonance as a sociological concept. Global Dial. 8, 41–44. Available online at: https://globaldialogue.isa-sociology.org/articles/the-idea-of-resonance-as-a-sociological-concept (accessed April 6, 2022)
Rosa, H. (2019). Resonance: A Sociology of Our Relationship to the World. Cambridge, UK: John Wiley & Sons.
Ruthven, I. (2021). Resonance and the experience of relevance. J. Assoc. Information Sci. Technol. 72, 554–569. doi: 10.1002/asi.24424
Sadler, P., Ethier, N., Gunn, G. R., Duong, D., and Woody, E. (2009). Are we on the same wavelength? Interpersonal complementarity as shared cyclical patterns during interactions. J. Personal. Soc. Psychol. 97, 1005. doi: 10.1037/a0016232
Safron, A. (2016). What is orgasm? A model of sexual trance and climax via rhythmic entrainment. Socioaffective Neurosci. Psychol. 6, 31763. doi: 10.3402/snp.v6.31763
Safron, A. (2020). An Integrated World Modeling Theory (IWMT) of consciousness: combining integrated information and global neuronal workspace theories with the free energy principle and active inference framework; toward solving the hard problem and characterizing agentic causation. Front. Artificial Intell. 3, 30. doi: 10.3389/frai.2020.00030
Santos, M., and Egerstedt, M. (2021). From motions to emotions: can the fundamental emotions be expressed in a robot swarm?. Int. J. Soc. Robot. 13, 751–764. doi: 10.1007/s12369-020-00665-6
Sarma, G. P., Hay, N. J., and Safron, A. (2018). “AI safety and reproducibility: establishing robust foundations for the neuropsychology of human values, in International Conference on Computer Safety, Reliability, and Security (Cham: Springer), 507–512.
Sato, T. G., and Moriya, T. (2019). “Respiration rate change induced by controlling the phasic relationship between melodic sound and respiration,” in 2019 41st Annual International Conference of the IEEE Engineering in Medicine and Biology Society (EMBC) (Berlin, Germany: IEEE), 3123–3126.
Savage, P. E., Loui, P., Tarr, B., Schachner, A., Glowacki, L., Mithen, S., et al. (2021). Music as a coevolved system for social bonding. Behav. Brain Sci. 44, 1–36. doi: 10.1017/S0140525X20000333
Schachner, A., Brady, T. F., Pepperberg, I. M., and Hauser, M. D. (2009). Spontaneous motor entrainment to music in multiple vocal mimicking species. Curr. Biol. 19, 831–836. doi: 10.1016/j.cub.2009.03.061
Schemer, C., Wirth, W., and Matthes, J. (2012). Value resonance and value framing effects on voting intentions in direct-democratic campaigns. Am. Behav. Scientist 56, 334–352. doi: 10.1177/0002764211426329
Schrock, D., Holden, D., and Reid, L. (2004). Creating emotional resonance: interpersonal emotion work and motivational framing in a transgender community. Soc. Probl. 51, 61–81. doi: 10.1525/sp.2004.51.1.61
Schubert, E., Vincs, K., and Stevens, C. J. (2013). Identifying regions of good agreement among responders in engagement with a piece of live dance. Empirical Stud. Arts 31, 1–20. doi: 10.2190/EM.31.1.a
Schütz-Bosbach, S., and Prinz, W. (2007). Perceptual resonance: action-induced modulation of perception. Trends Cogn. Sci. 11, 349–355. doi: 10.1016/j.tics.2007.06.005
Sciutti, A., Bisio, A., Nori, F., Metta, G., Fadiga, L., Pozzo, T., and Sandini, G. (2012). Measuring human-robot interaction through motor resonance. Int. J. Soc. Robot. 4, 223–234.
Sciutti, A., and Sandini, G. (2017). Interacting with robots to investigate the bases of social interaction. IEEE Trans. Neural Syst. Rehabil. Eng. 25, 2295–2304. doi: 10.1109/TNSRE.2017.2753879
Shang, S. S., Wu, Y. L., and Sie, Y. J. (2017). Generating consumer resonance for purchase intention on social network sites. Comput. Human Behav. 69, 18–28. doi: 10.1016/j.chb.2016.12.014
Sharp, H., Preece, J., and Rogers, Y. (2019). Interaction Design: Beyond Human Computer Interaction, 5th Edn. Chichester: Wiley.
Sheldrake, R. (2011). The Presence of the Past: Morphic Resonance and the Habits of Nature. London, UK: Icon Books Ltd.
Shim, S. B., Imboden, M., and Mohanty, P. (2007). Synchronized oscillation in coupled nanomechanical oscillators. Science 316, 95–99. doi: 10.1126/science.1137307
Siegel, I. R. (2013). Therapist as a container for spiritual resonance and client transformation in transpersonal psychotherapy: an exploratory heuristic study. J. Transpersonal Psychol. 45, 49–74.
Sinclair, A. T. (1975). The orbital resonance amongst the Galilean satellites of Jupiter. Mon. Not. R. Astron. Soc. 171, 59–72. doi: 10.1093/mnras/171.1.59
Slichter, C. P. (2013). Principles of Magnetic Resonance, Vol. 1. London, UK: Springer Science & Business Media.
Slow Mo Guys (2021, July 15). Shattering a wine glass with sound at 187,500FPS [Video]. Youtube. Available online at: https://youtu.be/X3uKmTdgmQg?t=339
Smith, A. (1759). Theory of Moral Sentiments, eds A. Kincaid and J. Bell (Edinburgh, UK: Strand Publishing).
Snow, D. A., and Benford, R. D. (1988). Ideology, frame resonance, and participant mobilization. Int. Soc. Movement Res. 1, 197–217.
Stern, D. N., Hofer, L., Haft, W., and Dore, J. (1985). “Affect attunement: the sharing of feeling states between mother and infant by means of inter-modal fluency,” in Social Perception in Infants, eds. T. Field and N. A. Fox (New York, NK: Ablex Publishing Corporation), 249–268.
Stiefel, K. M., and Ermentrout, G. B. (2016). Neurons as oscillators. J. Neurophysiol. 116, 2950–2960. doi: 10.1152/jn.00525.2015
Stray, J., Vendrov, I., Nixon, J., Adler, S., and Hadfield-Menell, D. (2021). What are you optimizing for aligning recommender systems with human values. arXiv preprint arXiv:2107.10939. doi: 10.48550/arXiv.2107.10939
Strogatz, S. H., Abrams, D. M., McRobie, A., Eckhardt, B., and Ott, E. (2005). Crowd synchrony on the millennium bridge. Nature 438, 43–44. doi: 10.1038/438043a
Strydom, P. (2003). “Resonance: triggering a dormant dimension of the public sphere,” in European Social Theory: Sources and Challenges, eds V. Kultygin and V. Zhukov (European Social Theory, Moscow: European Sociological Association), 83–99.
Sundfors, R. K., Bolef, D. I., and Fedders, P. A. (1983). Nuclear acoustic resonance in metals and alloys: a review. Hyperfine Interact. 14, 271–313. doi: 10.1007/BF02043303
Suzuki, N., and Katagiri, Y. (2007). Prosodic alignment in human-computer interaction. Conn. Sci. 19, 131–141. doi: 10.1080/09540090701369125
Swann, W. B. Jr., Jetten, J., Gómez, Á., Whitehouse, H., and Bastian, B. (2012). When group membership gets personal: a theory of identity fusion. Psychol. Rev. 119, 441. doi: 10.1037/a0028589
Takeya, R., Kameda, M., Patel, A. D., and Tanaka, M. (2017). Predictive and tempo-flexible synchronization to a visual metronome in monkeys. Sci. Rep. 7, 1–12. doi: 10.1038/s41598-017-06417-3
Tegnér, J., Compte, A., and Wang, X. J. (2002). The dynamical stability of reverberatory neural circuits. Biol. Cybern. 87, 471–481. doi: 10.1007/s00422-002-0363-9
Titchener, E. B. (1909). Lectures on the Experimental Psychology of the Thought Processes. New York, NY: The MacMillan Company.
Tojo, S., Taguchi, Y., Masuyama, Y., Hayashi, T., Saito, H., and Hirano, T. (2010). Controlling phase separation of binary Bose-Einstein condensates via mixed-spin-channel Feshbach resonance. Phys. Rev. A 82, 033609. doi: 10.1103/PhysRevA.82.033609
Tonks, L. (1931). Plasma-electron resonance, plasma resonance and plasma shape. Phys. Rev. 38, 1219. doi: 10.1103/PhysRev.38.1219
Treal, T., Jackson, P. L., Jeuvrey, J., Vignais, N., and Meugnot, A. (2021). Natural human postural oscillations enhance the empathic response to a facial pain expression in a virtual character. Sci. Rep. 11, 1–10. doi: 10.1038/s41598-021-91710-5
Trehub, S. E., and Trainor, L. (1998). Singing to infants: Lullabies and play songs. Adv. Infancy Res. 12, 43–78.
Trost, W. J., Labbé, C., and Grandjean, D. (2017). Rhythmic entrainment as a musical affect induction mechanism. Neuropsychologia 96, 96–110. doi: 10.1016/j.neuropsychologia.2017.01.004
Uithol, S., van Rooij, I., Bekkering, H., and Haselager, P. (2011). Understanding motor resonance. Soc. Neurosci. 6, 388–397. doi: 10.1080/17470919.2011.559129
Valencia, A. L., and Froese, T. (2020). What binds us? Inter-brain neural synchronization and its implications for theories of human consciousness. Neurosci. Consciousness 2020, niaa010. doi: 10.1093/nc/niaa010
van Werven, R., Bouwmeester, O., and Cornelissen, J. P. (2019). Pitching a business idea to investors: how new venture founders use micro-level rhetoric to achieve narrative plausibility and resonance. Int. Small Business J. 37, 193–214. doi: 10.1177/0266242618818249
Varga, S., and Heck, D. H. (2017). Rhythms of the body, rhythms of the brain: respiration, neural oscillations, and embodied cognition. Conscious. Cogn. 56, 77–90. doi: 10.1016/j.concog.2017.09.008
Vilibić, I. (2008). Numerical simulations of the Proudman resonance. Cont. Shelf Res. 28, 574–581. doi: 10.1016/j.csr.2007.11.005
Vincent, U. E., McClintock, P. V., Khovanov, I. A., and Rajasekar, S. (2021). Vibrational and stochastic resonances in driven nonlinear systems. Philos. Trans. R. Soc. A 379, 20200226. doi: 10.1098/rsta.2020.0226
Vlemincx, E., Abelson, J. L., Lehrer, P. M., Davenport, P. W., Van Diest, I., and Van den Bergh, O. (2013). Respiratory variability and sighing: a psychophysiological reset model. Biol Psychol. 93, 24–32.
Wang, S., Lin, D. N. C., Zheng, X., and Ji, J. (2021). Departure from the exact location of mean motion resonances induced by the gas disk in systems observed by Kepler. Astron. J. 161, 77. doi: 10.3847/1538-3881/abcfb9
Wang, X. J. (2001). Synaptic reverberation underlying mnemonic persistent activity. Trends Neurosci. 24, 455–463. doi: 10.1016/S0166-2236(00)01868-3
Warren, L. (2004). “Negotiating entrepreneurial identity: communities of practice and changing discourses.” Int. J. Entrepreneurship Innov. 5, 25–35. doi: 10.5367/000000004772913764
Weber, R., and Fisher, J. T. (2020). “Advancing the synchronization theory of flow experiences,” in The Handbook of Communication Science and Biology, eds. K. Floyd and R. Weber (London, UK: Routledge), 157–176.
Weber, R., Tamborini, R., Westcott-Baker, A., and Kantor, B. (2009). Theorizing flow and media enjoyment as cognitive synchronization of attentional and reward networks. Commun. Theory 19, 397–422. doi: 10.1111/j.1468-2885.2009.01352.x
Wertz, J. (2012). Electron Spin Resonance: Elementary Theory and Practical Applications. Berlin, Germany: Springer Science & Business Media.
Wheatley, T., and Sievers, B. (2016). Toward a neuroscience of social resonance. Positive Neurosci. 37, 37–54. doi: 10.1093/acprof:oso/9780199977925.003.0004
Wilfinger, R. J., Bardell, P. H., and Chhabra, D. S. (1968). The resonistor: a frequency selective device utilizing the mechanical resonance of a silicon substrate. IBM J. Res. Dev. 12, 113–118. doi: 10.1147/rd.121.0113
Williams, E. R. (1992). The Schumann resonance: a global tropical thermometer. Science 256, 1184–1187. doi: 10.1126/science.256.5060.1184
Wilson, M., and Cook, P. F. (2016). Rhythmic entrainment: why humans want to, fireflies can't help it, pet birds try, and sea lions have to be bribed. Psychon. Bull. Rev. 23, 1647–1659. doi: 10.3758/s13423-016-1013-x
Wilson, M., and Wilson, T. P. (2005). An oscillator model of the timing of turn-taking. Psychon. Bull. Rev. 12, 957–968. doi: 10.3758/BF03206432
Wiltermuth, S. (2012a). Synchrony and destructive obedience. Soc. Influence 7, 78–89. doi: 10.1080/15534510.2012.658653
Wiltermuth, S. (2012b). Synchronous activity boosts compliance with requests to aggress. J. Exp. Soc. Psychol. 48, 453–456. doi: 10.1016/j.jesp.2011.10.007
Winters, R. M., Walker, B. N., and Leslie, G. (2021). “Can you hear my heartbeat?: hearing an expressive biosignal elicits empathy,” in Proceedings of the 2021 CHI Conference on Human Factors in Computing Systems (Yokohama, Japan), 1–11.
Witek, M. A. (2019). “Feeling at one: socio-affective distribution, vibe, and dance-music consciousness,” in Music and Consciousness 2: Worlds, Practices, Modalities, eds R. Herbert, E. Clarke, and D. Clarke (New York, NY: Oxford University Press), 93–112.
Wohltjen, S., and Wheatley, T. (2021). Eye contact marks the rise and fall of shared attention in conversation. Proc. Nat. Acad. Sci. U.S.A. 118, 1–11. doi: 10.1073/pnas.2106645118
Wolf, E. (2010). Statistical similarity as a unifying concept of the theories of coherence and polarization of light. Opt. Commun. 283, 4427–4429. doi: 10.1016/j.optcom.2010.04.091
Wood, A., Rychlowska, M., Korb, S., and Niedenthal, P. (2016). Fashioning the face: sensorimotor simulation contributes to facial expression recognition. Trends Cogn. Sci. 20, 227–240. doi: 10.1016/j.tics.2015.12.010
Wood, C., Caldwell-Harris, C., and Stopa, A. (2018). The rhythms of discontent: synchrony impedes performance and group functioning in an interdependent coordination task. J. Cogn. Cult. 18, 154–179. doi: 10.1163/15685373-12340028
Xie, F., Zuo, Q., Chen, Q., Fang, H., He, K., Du, R., et al. (2021). Designs of the biomimetic robotic fishes performing body and/or caudal fin (BCF) swimming locomotion: a review. J. Intelligent Robotic Syst. 102, 1–19. doi: 10.1007/s10846-021-01379-1
Yang, J. (2014). The role of the right hemisphere in metaphor comprehension: a meta-analysis of functional magnetic resonance imaging studies. Hum. Brain Mapp. 35, 107–122. doi: 10.1002/hbm.22160
Yang, T., Chen, J., Huang, L., Wang, T., Xiao, C., Sun, Z., et al. (2015). Extremely short-lived reaction resonances in Cl+ HD (v= 1) → DCl+ H due to chemical bond softening. Science 347, 60–63. doi: 10.1126/science.1260527
Yaniv, A. U., Salomon, R., Waidergoren, S., Shimon-Raz, O., Djalovski, A., and Feldman, R. (2021). Synchronous caregiving from birth to adulthood tunes humans' social brain. Proc. Nat. Acad. Sci. U.S.A. 118. doi: 10.1073/pnas.2012900118
Zhang, D., Wu, J., Zhang, R., Zhang, H., and He, Z. (2013). High precision micro-impulse measurements for micro-thrusters based on torsional pendulum and sympathetic resonance techniques. Rev. Sci. Instruments 84, 125113. doi: 10.1063/1.4850615
Ziada, S., and Lafon, P. (2014). Flow-excited acoustic resonance excitation mechanism, design guidelines, and counter measures. Appl. Mech. Rev. 66, 1–22. doi: 10.1115/1.4025788
Keywords: resonance, entrainment, synchronization, metaphor, design space, social robotics, AI for wellbeing, human-media interaction
Citation: Lomas JD, Lin A, Dikker S, Forster D, Lupetti ML, Huisman G, Habekost J, Beardow C, Pandey P, Ahmad N, Miyapuram K, Mullen T, Cooper P, van der Maden W and Cross ES (2022) Resonance as a Design Strategy for AI and Social Robots. Front. Neurorobot. 16:850489. doi: 10.3389/fnbot.2022.850489
Received: 07 January 2022; Accepted: 23 March 2022;
Published: 27 April 2022.
Edited by:
Adam Safron, Johns Hopkins Medicine, United StatesReviewed by:
Guillaume Dumas, Université de Montréal, CanadaVicente Raja, Western University, Canada
Copyright © 2022 Lomas, Lin, Dikker, Forster, Lupetti, Huisman, Habekost, Beardow, Pandey, Ahmad, Miyapuram, Mullen, Cooper, van der Maden and Cross. This is an open-access article distributed under the terms of the Creative Commons Attribution License (CC BY). The use, distribution or reproduction in other forums is permitted, provided the original author(s) and the copyright owner(s) are credited and that the original publication in this journal is cited, in accordance with accepted academic practice. No use, distribution or reproduction is permitted which does not comply with these terms.
*Correspondence: James Derek Lomas, ai5kLmxvbWFzQHR1ZGVsZnQubmw=