- Division of Plastic Surgery, Department of Surgery, University of Wisconsin–Madison, Madison, WI, United States
Over the last few decades there has been a push to enhance the use of advanced prosthetics within the fields of biomedical engineering, neuroscience, and surgery. Through the development of peripheral neural interfaces and invasive electrodes, an individual's own nervous system can be used to control a prosthesis. With novel improvements in neural recording and signal decoding, this intimate communication has paved the way for bidirectional and intuitive control of prostheses. While various collaborations between engineers and surgeons have led to considerable success with motor control and pain management, it has been significantly more challenging to restore sensation. Many of the existing peripheral neural interfaces have demonstrated success in one of these modalities; however, none are currently able to fully restore limb function. Though this is in part due to the complexity of the human somatosensory system and stability of bioelectronics, the fragmentary and as-yet uncoordinated nature of the neuroprosthetic industry further complicates this advancement. In this review, we provide a comprehensive overview of the current field of neuroprosthetics and explore potential strategies to address its unique challenges. These include exploration of electrodes, surgical techniques, control methods, and prosthetic technology. Additionally, we propose a new approach to optimizing prosthetic limb function and facilitating clinical application by capitalizing on available resources. It is incumbent upon academia and industry to encourage collaboration and utilization of different peripheral neural interfaces in combination with each other to create versatile limbs that not only improve function but quality of life. Despite the rapidly evolving technology, if the field continues to work in divided “silos,” we will delay achieving the critical, valuable outcome: creating a prosthetic limb that is right for the patient and positively affects their life.
Introduction
Limb amputation is a transformative, debilitating life event that has the ability to drastically impair one's quality of life. Consequential psychosocial stressors associated with limb amputation can affect careers, personal relationships, and threaten an individual's sense of self (Resnik et al., 2011; Sinha et al., 2011). There are ~2.2 million people living with limb loss in the United States (Ziegler-Graham et al., 2008). As that number continues to climb, efforts to improve amputation-related morbidity have led to collaborations between experts in the fields of medicine and engineering and the development of innovative rehabilitation strategies. Advancements in biomechanics and prosthetic technology have focused on improving functionality and restoring a sense of embodiment in those who have undergone amputations. Similarly, new surgical techniques have been created to further optimize prosthetic use and alleviate chronic postamputation pain (Mioton and Dumanian, 2018). In contrast with these rapid developments, the surgical approach to limb amputations has evolved at a much slower pace. Traditionally amputation surgery was viewed as a limb salvaging procedure focused on adequate soft tissue coverage and limb preservation strategies (Markatos et al., 2019). This perspective transformed into a reconstructive approach with the production of bionic limbs and the possibility of high-fidelity control capabilities (Clites et al., 2019). A recent review by Herr et al. describes this new approach with the intention of advocating for optimization of the residual limb and reconstructive techniques (Herr et al., 2020). Focus has shifted from preservation of maximal limb length to preservation of adequate limb length for prosthetic control (Sanders and Fatone, 2011). It is now recognized that maximal limb length does not necessarily equate to maximal function and in some instances, a shorter residual limb length may be preferable for superior prosthetic design. The reconstructive approach prioritizes the care of peripheral nerves, soft tissue, and residual muscles in an effort to improve pain control and provide a foundation for neural communication in a prosthetic limb (Brown et al., 2014). The design and implementation of this technology is dependent on the execution of the amputation procedure and subsequent limb reconstruction.
While considerable progress has been made in improving prosthetic technology and motor control, incorporation of sensory feedback is lacking. Sensation is responsible for enhancing motor control and restoring a sense of embodiment that contributes to overall function and quality of life in amputees (Witteveen et al., 2012; Tan et al., 2015; Tyler, 2015; Dornfeld et al., 2016). Without sensation, individuals are unable to recognize the position of their limbs in space and thus must rely on visual cues as their only source of feedback. Prior to the development of neural interfaces, non-invasive substitution methods such as electro-tactile or vibro-tactile feedback were created as a means of restoring sensation. Though these techniques have demonstrated some capability of sensory stimulation, they are not able to offer the level of selectivity necessary for clinical application. As a result, more invasive methods with implantable nerve electrodes and neural interfaces have been pursued (Ghafoor et al., 2017). The direct neural contact allows for more selective and specific stimulation; however, this stimulation comes at the expense of stability and overall durability (Navarro et al., 2005; Wurth et al., 2017). Long term implementation of these devices is dependent on establishing a balance between the conflicting forces of specificity and stability. This requires a multidisciplinary approach that integrates the fields of biomedical engineering, surgery, and prosthetic device design to address the challenges posed by these interfaces and propose more innovative ways to interact with the peripheral nervous system.
The ability for human and machine to communicate directly via the nervous system became a reality when peripheral neural interfaces (PNI) emerged. The interface design uses an individual's own peripheral nerve as a channel for signal relay between the brain and an external device. Each peripheral nerve is made up of both afferent and efferent fibers that allow for bidirectional communication. The afferent fibers transmit information to the nervous system and the efferent fibers transmit information away from the nervous system (Tyler et al., 2015). An electrode(s) is used to harness the electrical energy produced via these pathways for the stimulation or recording of signals required for bidirectional communication and control of a prosthesis.
Electrical signals generated by the peripheral nervous system can be used to power a prosthesis both directly and indirectly. Myoelectric systems record Electromyography (EMG) signals indirectly through surface electrodes to activate motor commands, whereas more invasive PNIs use direct contact with the nervous system to record these signals (Kung et al., 2013). Though these systems have significantly improved prosthetic control, they are far more limited in their ability to incorporate sensory feedback, a key component of bidirectional signaling required for closed loop control (Micera and Navarro, 2009; Carey et al., 2015).
The substantial progress that has been made in the field of neuroprosthetics and the formation of silos is reflected in the robust body of literature published on these topics. Many of the reviews focus on a specific area of the field such as electrodes (Rijnbeek et al., 2018; Russell et al., 2019; Raspopovic et al., 2020); interface design (Larson and Meng, 2020), or surgical techniques like Targeted Muscle Reinnervation (TMR) (Mioton and Dumanian, 2018; Oh and Carlsen, 2019; Peters et al., 2020); and Regenerative Peripheral Nerve Interface (RPNI) (Santosa et al., 2020). Others explore algorithmic control systems (Wolf et al., 2020); prosthetic device designs (Naufel et al., 2020); and specific outcomes such as sensation or myoelectric control (Tyler, 2015; Geethanjali, 2016; Ghafoor et al., 2017; Sensinger and Dosen, 2020; Raspopovic et al., 2021). The former comprehensive reviews are outdated and do not cover newer developments such as the Agonist-antagonist Myoneural Interface (AMI) or Osseointegration (OI) (Navarro et al., 2005; Kung et al., 2013; Bates et al., 2020; Vu et al., 2020a; Yildiz et al., 2020). Additionally, we see each of these reviews as important focal points highlighting gaps and advances within individual domains, without necessarily considering how these domains integrate with each other to form a cohesive solution or standard of practice.
In this review, we will discuss a global overview of the current state of peripheral nerve interfaces including nerve electrodes, surgical innovations, and available prosthetic technology. We explore the approach to amputations and the barriers that prevent clinical use of advanced prosthetics. The main goal of this review is to identify the strengths and weaknesses of various peripheral nerve interfaces in an effort to advocate a more collaborative approach that supports a combination of different electrical and surgical interventions to optimize utilization of the bionic limb.
Electrodes
Electrodes interact with the peripheral nervous system myoelectrically or through nerve electrodes with varying degrees of invasiveness (Woo et al., 2016). This section of the review will focus on the electrodes that have direct contact with nerves. The reliability and longevity of a peripheral nerve interface depends on a number of electrode characteristics and requirements that contribute to the overall design. This includes tissue interaction, stimulation and recording capability, host immune response, and biocompatibility (Raspopovic et al., 2020). The area of greatest concern is electrode-tissue interaction leading to mechanical mismatch and a biological tissue response that results in device failure. Over time, micromotion within the tissue can lead to degradation of signal and potential neuronal damage (Woeppel et al., 2017). Similarly, the implanted foreign materials may trigger an inflammatory reaction that also contributes to such damage (Grill et al., 2009). Strategies to mitigate these challenges include reducing size of implant, constructing soft and flexible electrodes, and use of bioactive or conducting polymer coatings (Diment et al., 2018; Wellman et al., 2018).
The main goal of creating a successful, implantable electrode is establishing high quality signal transmission that is able to endure a surrounding harsh environment without significant tissue disruption. Ideally, this would allow electrodes to record motor potentials and evoke sensory signals with high selectivity and longevity. Selectivity is defined as “the ability to activate one population of neurons without concomitant activation of another” (Grill et al., 2009). This precise activation of different fascicles leads to the production of refined motor movements and natural discrimination of sensory precepts. Longevity refers to the stability—or activation of the same population of axons over time—and is critical for survival of the electrode and production of naturalistic sensation (Ghafoor et al., 2017). Selectivity and stability largely depend on invasiveness of electrodes and unfortunately act in opposition to each other. Selectivity tends to increase with invasiveness whereas stability tends to decrease. In addition, selectivity and stability are influenced by the number and configuration of electrodes (Lee et al., 2017; Charkhkar et al., 2018); biocompatibility (Woeppel et al., 2017); and pattern recognition algorithms (Hargrove et al., 2017). These properties significantly impact the stimulating and recording capabilities of electrodes and their ability to restore function (Merrill et al., 2005; Cogan, 2008). The fundamental electrochemical properties that dictate the actions of stimulating and recording electrodes are beyond the scope of this review.
The peripheral nerve electrodes can be separated into three different categories: extraneural electrodes, intraneural electrodes, and regenerative electrodes.
Extraneural Electrodes
Extraneural electrodes, sometimes referred to as “epineural” electrodes, encircle the outside of the nerve and do not penetrate the epineurium. They are the least invasive nerve electrodes and are therefore less susceptible to damage (Micera et al., 2008). The cuff electrode has an insulated sheath with electrode contacts on the surface of the epineurium for recording and stimulation of large neural fibers (Loeb and Peck, 1996). There are several derivations of the cuff electrode that have been designed to improve selectivity and spatial resolution. These variations involve refining geometry (Dweiri et al., 2016); increasing electrode contact density (Polasek et al., 2009); and reducing nerve compression (Lee et al., 2017) to avoid subsequent damage. The flat interface nerve electrode (FINE) increases surface area, bringing axons to the surface and creating additional contact sites for selective stimulation (Tyler and Durand, 2002). Though this was an improvement from the traditional cuff electrode design, the FINE still requires high stimulation currents which may evoke unnatural feelings or paresthesias (Leventhal and Durand, 2003). The composite flat interface nerve electrode (c-FINE) design further reshaped the cuff electrode with areas of alternating flexibility and stiffness, creating an adaptable electrode with high contact density sites (Freeberg et al., 2017).
Extraneural electrodes have demonstrated chronic stability with recording and stimulating in humans for up to 11 years (Fisher et al., 2009; Tan et al., 2014; Christie et al., 2017; Charkhkar et al., 2018). Selectivity is expected to be limited due to lack of invasiveness and activation of larger afferent populations, creating cross talk from EMG activity of surrounding muscles and undifferentiated neural activity from neighboring fascicles. The recorded electroneurography (ENG) signals have lower amplitudes with poor signal to noise ratio (SNR) compared with EMG signals (Micera et al., 2010). Different strategies using machine learning algorithms and spatial filtering for separation of individual signals have been devised to reduce noise and optimize recording with extraneural electrodes (Wodlinger and Durand, 2011; Tang and Durand, 2014; Dweiri et al., 2016; Aristovich et al., 2018). Additionally, electrode configurations (Howell et al., 2015) and varied stimulation parameters (Cogan et al., 2016) have been designed to improve stimulation specificity. Tan et al. demonstrated that sophisticated patterns of stimulation can produce highly localized sensory precepts of several different qualities. These electrodes were the first to achieve restoration of meaningful sensation with long term stability and have the greatest potential for highly selective stimulation (Tan et al., 2014). Although these methods offer improvements in electrical communication, extraneural electrodes have not been able to provide complex control.
In addition to controlling movement and eliciting sensation, extraneural electrodes have also been investigated for use in pain management in amputees. Stimulation with the cuff electrode eliminated chronic phantom pain within 6 months with pain relief continuing even in the absence of stimulation. Similarly, the FINE electrode demonstrated elimination of phantom limb pain (PLP) after 9 months (Tan et al., 2015). More quantitative and comparative studies are needed to draw conclusions regarding the effectiveness of electrodes and reduction in PLP.
Intraneural Electrodes
Intraneural electrodes penetrate the epineurium and directly communicate with nerve fascicles. There are three main intraneural electrodes: the longitudinal intra-fascicular electrode (LIFE), the transverse intra-fascicular multichannel electrode (TIME), and the Utah Slanted Electrode Array (USEA) (Jung et al., 2018). The original LIFE design places a stiff wire longitudinally along a set of fascicles; one wire is required for each electrode (Zheng et al., 2003). Similar to the early cuff electrodes, the LIFE's limited geometric flexibility and increased risk of damage led to the development of more advanced versions (Lawrence et al., 2004; Lago et al., 2007). The upgraded thin-film LIFE (tf-LIFE) uses flexible polyimide material and a higher density of electrode contacts compared to the original design. The additional sites increase activation of a specific set of fascicles; however, the longitudinal arrangement prevents access to different populations of fascicles (Kundu et al., 2014) and thus may limit selectivity compared with other intraneural electrodes. The TIME was instead purposefully designed in a transverse orientation and therefore can provide multiple contacts for different sets of axons at the same time (Boretius et al., 2010). The most invasive intraneural electrode, the USEA, is a penetrating microelectrode array containing 100 contact sites that is implanted transversely through the nerve to enhance selectivity and spatial resolution (Davis et al., 2016). The vast number of contact sites within different populations of fascicles offers the possibility of precise control of fine motor movements.
While the intraneural electrodes' intimate contact permits high selectivity with stimulation and increased signal amplitude with recording, it puts the nerve at risk for damage (Vu et al., 2020b). The main limiting factor of these electrodes is stability. Over time, the SNR decreases, and higher levels of stimulation are required (Raspopovic et al., 2020). LIFEs have had short term success with providing sensory feedback (Horch et al., 2011) and decoding grasp signals (Micera et al., 2011). A tf-LIFE implanted in a human amputee recorded stable motor signals throughout a 4-week trial, but after 10 days, sensory stimulation had diminished (Rossini et al., 2010). More recently, this stability has increased to 11 weeks with closed loop control of slippage and grasping (Zollo et al., 2019). The TIME has shown more promise, with numerous studies demonstrating its ability to provide selective stimulation and sensory feedback (Raspopovic et al., 2014; Oddo et al., 2016; D'Anna et al., 2019). The TIME's longevity is superior to the LIFE with demonstration of stability of stimulation signals for 6 months in three transradial amputees (Petrini et al., 2019b). Futhermore, the TIME has also been used in lower limb amputees, with findings showing increased mobility and improved confidence when sensory feedback is supplied (Valle et al., 2021). This type of closed loop control is especially important in lower limb amputees who lack balance and native gait cues.
Among the intraneural electrodes, the USEA has the greatest potential for achieving high selectivity. Two transradial amputees with implanted USEAs were able to control individual finger movements as well as elicit multimodality sensory percepts (Davis et al., 2016). USEA implanted in two other transradial amputees achieved independent control of five degrees of freedom (DoF) and perceived as many as 131 sensory percepts. EMG signals in addition to the ENG signals were required to achieve this level of control (Wendelken et al., 2017). Though signals tend to degrade over time, performance of USEAs continue to improve with recent evidence of stability and functionality after 14 months (George et al., 2020). These electrodes are capable of recording and stimulating individual neural fascicles and thus have the potential to provide complex control of a prosthesis. They must demonstrate long-term stability of signals before they will be accepted for chronic implementation in humans.
Similar to the extraneural electrodes, the intraneural electrodes have demonstrated success in mitigating postamputation pain. Using LIFEs for sensory feedback resulted in resolution of symptoms due to phantom-limb syndrome (Rossini et al., 2010) and neural stimulation of TIME resulted in decreased phantom limb pain (Petrini et al., 2019a). This was also seen long term with USEA in a transradial amputation with demonstration of phantom pain reduction and prosthesis embodiment (Page et al., 2018). This was achieved with open loop motor control and open loop sensory control idependently.
Regenerative Electrodes
Regenerative electrodes are considered the most invasive PNIs with the largest number of axon contacts. They are classically defined as requiring transection of the nerve so it can regenerate around or through an electrode (Lago et al., 2005). However, this definition is a byproduct of the experimental methodology, which is based on the standard neurorrhaphy model in an intact limb, measuring return of functions (Vela et al., 2020). In the amputation setting, the invasive transection is typically the result of the traumatic event, or an operative requirement of the amputation. Furthermore, treatment for post-amputation neuroma typically involves surgical dissection and excision of the neuroma (Souza et al., 2014; Woo et al., 2016; Israel et al., 2018) before the final surgical intervention to prevent its return. In the amputation setting, the application of regenerative electrodes to a transected nerve end adds little to no invasiveness to the amputation procedure itself (Millevolte et al., 2021). There are various design types including sieve electrodes, regenerative multi-electrode arrays, and scaffolding electrodes. These electrodes have the potential to provide the highest level of selectivity with low stimulation thresholds and further improve prosthetic control (Grill et al., 2009; Cutrone et al., 2015; Ghafoor et al., 2017; Coker et al., 2019). However, there are a number of challenges that make implementation of regenerative electrodes difficult. The transected nerve fibers must regenerate appropriately and successfully integrate with the electrodes. Regenerative electrodes have demonstrated evidence of stable recording and stimulation of signals in animals (MacEwan et al., 2016), though the concern for damage has prevented their use in humans.
Surgical Techniques
Peripheral nerve interfaces offer promising potential for the future of advanced prosthetics; however, the majority remain experimental, facing obstacles they must first overcome to achieve full clinical translation. The surgical application of PNIs is one such obstacle, particularly in relation to the application of more invasive PNIs available. As a result, unique surgical techniques have been developed to address these limitations and enhance functionality of the interface. They are designed to create high fidelity signals capable of simultaneous control with multiple degrees of freedom (Kung et al., 2013). Increased opportunity for complex movements motivated the prosthetic industry to maximize range of motion (ROM) and revolutionize device attachment.
These procedures not only provide alternative methods of prosthetic control, but can also prevent and treat painful postamputation neuromas (Santosa et al., 2020). After transection, a peripheral nerve works to regenerate until it finds an end organ to innervate. In the setting of amputations, the nerve undergoes axonal sprouting and regeneration without available target sites, and thus there is formation of an unpredictable, excitable mass of fibers (Stokvis et al., 2010). Chronic neuroma pain is a debilitating sequela of amputation that often precludes amputees from using a prosthetic device and significantly contributes to loss of function. Some studies suggest that at least 25% of amputees experience painful neuromas (Sehirlioglu et al., 2009; Bowen et al., 2017). Prior to these innovative surgical techniques, over 100 different treatments were unsuccessful in establishing a standard solution for relief of symptoms, with at least 20-30% of cases being refractory to treatment (Poppler et al., 2018).
In addition, these surgical techniques have encouraged conversations about amputations and have helped shape the way we think about them in the traditional landscape (Herr et al., 2020). There is a fundamental need to redefine the amputation surgery that places an emphasis on reconstruction as well as on incorporation of neural interfaces for prostheses.
Targeted Muscle Reinnervation (TMR)
TMR was one of the first surgical techniques established and was originally designed to be an improvement of the myoelectric systems already used for prosthetic control. These traditional methods typically only allow for control of one DoF at a time and do not provide any sensory feedback or treatment of neuropathic pain (Kilgore et al., 2008). TMR reroutes residual nerves from the amputated limb to different, denervated target muscles that are functionally not required or functionally redundant. The native nerve is transected near the donor muscle and then coapted to the donor nerve at the muscle entry point (Figure 1). The denervated target muscles lose their native function and are instead transformed into biological amplifiers that allow for simultaneous motor control of multiple DoF (Kuiken et al., 2004, 2009). TMR can even provide prosthetic movements from muscles that are no longer present.
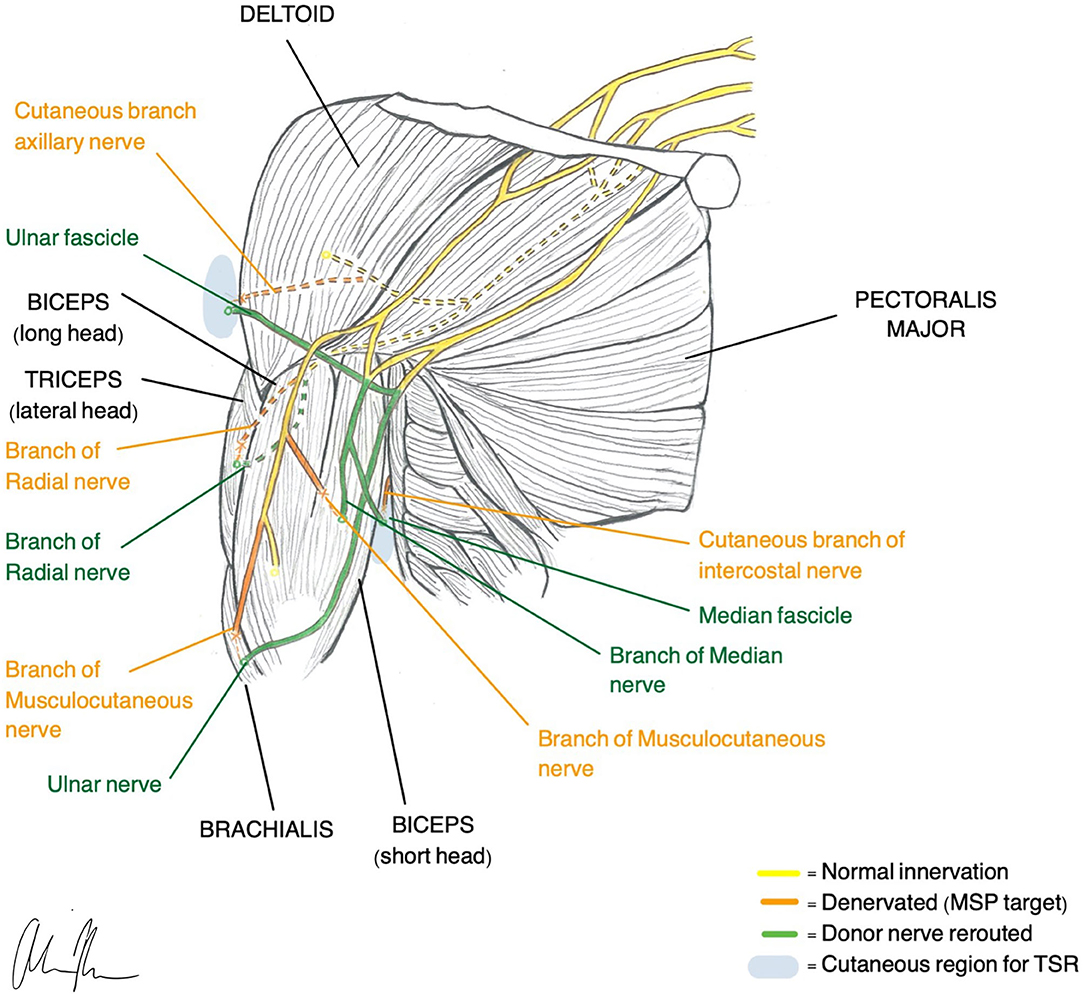
Figure 1. Illustration of TMR (Gart et al., 2015) and TSR (Hebert et al., 2014) construction in a transhumeral amputee.
Motor Control
Unlike the other myoelectric systems, TMR provides more natural control without unnatural code switching or requirement of intact distal muscles for activation of movement (Kuiken et al., 2007). Attempted movements produce EMG signals in the reinnervated muscles that are measured by surface electrodes and used to power a prosthesis. Although effective in recording EMG activity, there are several problems associated with surface electrodes. EMG signals from nearby muscles create noisy cross talk and subsequent difficulty with signal extraction. In addition, there is limited available surface area and any skin disturbances may cause the electrodes to shift (Young et al., 2011; Kuiken et al., 2016). Despite these challenges, surface electrodes provide a method of EMG signaling that is non-invasive and free of percutaneous wiring.
Transhumeral amputees and individuals with shoulder disarticulations are the most common recipients of TMR, although recently there has been more work looking at transradial amputees (Pierrie et al., 2018). In general, TMR in the upper extremity offers four different control sites that are each reinnervated by one of the major peripheral nerves. For example, in transhumeral amputees, native innervation of the long head of the biceps and triceps remains intact while the short head of the biceps is reinnervated by the median nerve and the lateral head of the triceps is reinnervated by the distal radial nerve. Native innervation controls elbow flexion/extension and the new reinnervations control hand open/closure (Cheesborough et al., 2015). If enough residual limb is available, it is possible to re-route part of the ulnar nerve to the brachialis so wrist control can be achieved.
Patterns for reinnervation are determined by the availability of musculature and donor nerves (Gart et al., 2015). The number of control sites is limited by the number of available, denervated target muscles. Since nerve transfer involves reimplantation of a whole nerve only 4–5 functions are possible and thus complex control with intrinsic hand muscles is difficult to achieve (Smith et al., 2015). To obtain direct control with a naturalistic, intuitive feel, one EMG control must correlate with one DoF. Decreased spatial resolution and subsequent EMG cross talk makes this challenging (Farina et al., 2014). In an effort to increase the number of control possibilities and execute functions, pattern recognition algorithms have been applied to enhance signal interpretation (Zhou et al., 2007; Kuiken et al., 2009, 2016). A recent at-home trial with TMR patients demonstrated superior performance with pattern recognition compared with direct control (Hargrove et al., 2017). Although pattern recognition addresses some of the shortcomings of surface EMG, it does not provide the same natural feel as does direct control. Instead, movements must be carried out sequentially in specific patterns, and the lack of simultaneous and proportional control feels slow and unusual (Farina et al., 2014).
Sensory Feedback
To further improve motor control and close the loop in a bidirectional prosthesis, sensation must be restored (Tyler, 2015; Markovic et al., 2018; Petrini et al., 2019a). An analogous sensory version of TMR, called Targeted Sensory Reinnervation (TSR), has been presented as a potential solution to restoring sensation. Similar to TMR, this method transfers transected sensory nerves to the motor entry points so it can then grow into denervated residual skin (Kuiken et al., 2007). This allows an amputee to experience referred sensation from the amputated limb when the corresponding reinnervated area of skin is activated. TSR has demonstrated its ability to reinnervate skin and produce tactile feedback in several studies (Kuiken et al., 2007; Kim and Colgate, 2012; Hebert et al., 2014). However, results with TSR have been variable, and several limitations have prevented full adoption of the method (Hebert et al., 2016). The reinnervated skin overlies muscles that produce additional EMG signals, creating noise and making independent signal extraction challenging. The need for both touch feedback tactors and EMG electrodes requires a large amount of skin surface area (Schofield et al., 2020). Additionally, motor efferent signals predominate over afferent and thus simultaneous control of movement and sensation is not possible (Kim and Colgate, 2012). When tactile feedback is produced, it may feel unnatural since the individual must acknowledge that this sensation is coming from a different part of the body. Studies of upper limb cortical maps in individuals with TSR indicate that integration of sensory information does not occur with visual bodily clues (Serino et al., 2017). Despite the ability of TSR to elicit sensory precepts, cortex integration requires an interpretation of sensation that is otherwise unnatural.
Management of Symptomatic Neuromas
Although TMR has not demonstrated significant success with restoring sensation, it is one of the most effective methods of managing symptomatic neuromas in amputees. The denervated target muscle provides a destination for a regenerating nerve to naturally grow into without formation of aberrant axons and subsequent pain (Mioton and Dumanian, 2018). These findings have translated clinically and patients with neuroma pain who underwent TMR experienced resolution or reduction in their pain postoperatively (Souza et al., 2014; Bowen et al., 2019). TMR is not limited to secondary intervention and can also be performed preemptively at the time of amputation. Studies assessing TMR as a primary intervention have demonstrated reduced phantom limb pain, improved outcomes related to pain quality, and lower rates of opioid use (Valerio et al., 2020; O'Brien et al., 2021). Similarly, a study of primary TMR in below knee amputations (BKA) did not find that any patients developed symptomatic neuromas during the follow up period. Additionally, early phantom limb pain was significantly reduced by 3 months and resolution was seen by 6 months (Bowen et al., 2019). Further investigation with a multi-institutional randomized clinical trial revealed that primary TMR improved phantom limb pain and trended toward reduced residual limb pain compared to standard neurectomy (Dumanian et al., 2019).
Surgical and Prosthetic Applications
Neuroma treatment and prevention with TMR has been used in both upper and lower extremity amputations. In terms of prosthetic control, TMR has only been used in individuals with upper extremity amputations (Oh and Carlsen, 2019; Peters et al., 2020). However, anatomic studies have identified transfer patterns and potential motor targets in BKAs (Fracol et al., 2018) and transfemoral amputations (TFA) (Agnew et al., 2012). For either application TMR can be performed as a primary surgery at the time of amputation or as a secondary surgery post amputation.
Unlike many PNI surgical techniques, TMR utilizes non-invasive electrical connections via surface electrodes, permitting take-home use and participation in clinical studies (Schofield et al., 2020). With new technology it may soon possible to replace these surface electrodes with implantable myoelectric sensors for enhancement of motor control and improvement of sensory feedback (Lowery et al., 2006; Miller et al., 2008). A recent prospective study of three transhumeral amputees with chronically implanted myoelectric sensors (IMES) demonstrated substantially improved functional outcomes compared with standard surface electrodes (Salminger et al., 2019). This provides high quality signals and improved performance in direct control without the need for pattern recognition. IMES represents a significant achievement for PNIs using intramuscular electrodes, thought it does not come without limitations. The current design is not compatible with osseointegration or shoulder disarticulations and is restricted to 6 total sensors, or a maximum of 3 DoFs (Salminger et al., 2019). TMR may be used in conjunction with other invasive nerve electrodes or even osseointegration for implementation of a fully implantable system.
Regenerative Peripheral Neural Interface (RPNI)
RPNI is based on the same neurobiological foundations as TMR in that it uses a transected peripheral nerve or fascicle and implants it into an autologous muscle graft (Figure 2) to serve as a biological amplifier of efferent signals (Santosa et al., 2020). The novel aspects of the RPNI are that it utilizes free muscle grafts that contain integrated electrodes to improve specificity and reduce crosstalk, thereby producing high quality, isolated EMG signals (Urbanchek et al., 2012, 2016; Kung et al., 2014; Woo et al., 2014; Irwin et al., 2016; Ursu et al., 2016). Furthermore, RPNI does not require denervation of existing muscles and is therefore not restricted to a limited number of control sites. Its ability to interface with multiple fascicles gives RPNI the potential to control for many DoFs. RPNI, like TMR, was initially developed as a means of advancing prosthetic function and was later discovered to serve as a viable treatment for symptomatic neuromas. Substantial preclinical evidence demonstrating RPNI's ability to produce stable efferent signals has resulted in clinical translation of the RPNI to treat amputation neuroma and prosthetic control in humans.
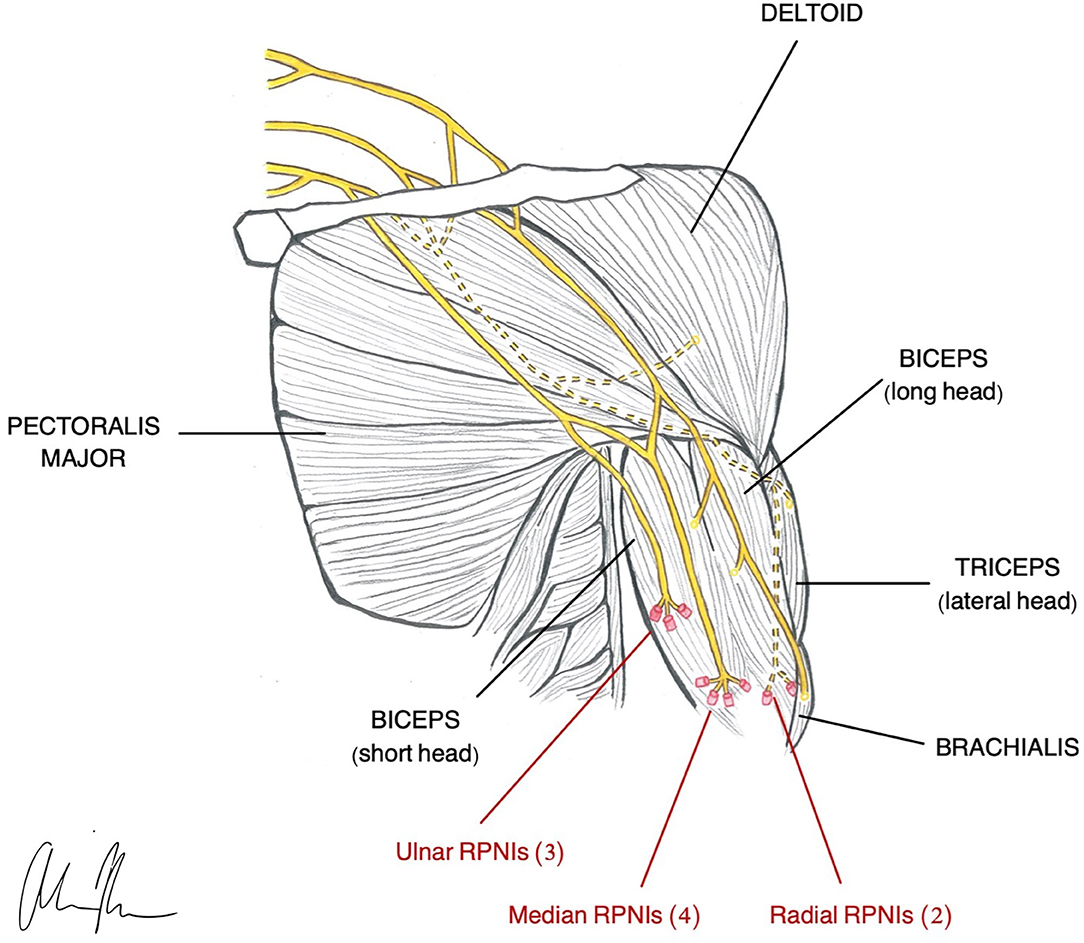
Figure 2. Illustration of RPNI construction in a transhumeral amputee (Vu et al., 2020a).
Motor Control
The RPNI was created with the purpose of expanding myoelectric interface capabilities and achieving greater signal specificity with chronic stability. The RPNI is designed to provide complex intuitive movement by maximizing number of control sites and increasing DoF. In a pilot study by Vu et al., RPNIs in upper limb amputees generated large amplitude EMG signals with high SNR and chronic stability over 10 months. This allowed for real-time continuous and simultaneous control of one DoF finger and two DoF thumb movements, as well as control of missing intrinsic hand muscles (Vu et al., 2020b), making RPNI the first PNI in which control of individual finger movements could be demonstrated.
This type of selectivity is possible with RPNI because of the way it utilizes free muscle grafts and is not limited by availability of residual native muscles. In addition, implantable muscular electrodes provide specific EMG signals with minimal cross talk, enhancing selectivity (Farina et al., 2014). To further maximize prosthetic function, electrodes may be implanted in residual muscles for additional control sites and improvement of signal specificity (Vu et al., 2020b). Similarly, application of control algorithms used in other PNI designs may help enhance interpretation of fine movement.
Sensory Feedback
There is currently no published data available regarding RPNIs and restoration of sensation.
Management of Symptomatic Neuromas
In addition to providing prosthetic control, RPNI is a novel surgical technique capable of preventing and treating symptomatic neuromas. Similar to TMR, it provides denervated target muscles for regenerating axons to grow into, thereby reducing the number of available axons for neuroma formation (Kung et al., 2014).
The first human study of RPNI for neuroma relief was a retrospective case series of 16 amputees. Findings revealed a 71% and 53% reduction in neuroma pain and phantom pain, respectively (Woo et al., 2016). Patients also reported decreased pain interference, reduction in opioid use, and improved prosthetic use (Woo et al., 2016). A more recent retrospective study investigated RPNI as a prophylactic intervention for the prevention of neuromas. The study compared postamputation pain outcomes between individuals who underwent prophylactic RPNI at the time of amputation to individuals who underwent amputation without RPNI and thus served as controls. The patients with RPNI showed 0% incidence of symptomatic neuroma formation (13% in control group) as well as a significant reduction in phantom limb pain compared with the control group (Kubiak et al., 2018). The results of these initial studies suggest that RPNI is efficacious in both the prevention and treatment of symptomatic neuromas and phantom pain.
Surgical Application and Prosthetic Compatibility
RPNIs can be implemented at the time of amputation or at a later date with a second operation. Although it may be more beneficial to do prophylactically for neuroma prevention, electrode implantation may dictate the timing of the surgery. A recent study by Srinivasan et al. hypothesized that electrical stimulation would expedite the process of revascularization and regeneration in free muscle grafts; however, the group instead discovered that stimulation actually interferes with these processes (Srinivasan et al., 2019). Future work will be needed to determine optimal timing for surgery based on these results.
RPNI can be used in upper and lower extremity amputations for symptomatic neuroma management (Woo et al., 2016; Kubiak et al., 2019), but in terms of prosthetic control, current studies are limited to upper extremity amputations. The devascularized free muscle grafts used for each RPNI do not require denervation of any native muscles nor depend on long nerve transfers (Woo et al., 2014), and thus could theoretically be applied to any amputation level in any limb. Previous studies have used the vastus lateralis as the autologous donor muscle (Vu et al., 2020b), though future work may explore other options. Smaller grafts have demonstrated better signal production and tissue viability compared with larger grafts in rat models (Hu et al., 2021). Current research is underway to determine optimal size, location, and number of RPNIs that can be used.
Relative to other PNI designs, surgical implantation of RPNI is relatively straightforward. It does not require microsurgery techniques and is likely translatable across multiple surgical subspecialties (Kubiak et al., 2018). This should help expedite the adoption of RPNI as an established neuroma treatment. By contrast, there are barriers that preclude current clinical use of RPNIs for prosthetic control. For instance, the use of implantable electrodes requires percutaneous wiring that presents a risk for infection, breakage, and disrupted connection (Ortiz-Catalan et al., 2014). For take-home use, it will be essential that RPNIs operate via surface electrodes, wireless IMES, or in combination with osseointegration. Lastly, it will be necessary for RPNIs to incorporate sensory feedback for complete bidirectional control. The current design does not have a specified method of providing this feedback and therefore will likely require use in conjunction with other PNIs to restore sensation.
Agonist-Antagonist Myoneural Interface (AMI)
The AMI is the newest PNI surgical innovation represented in the literature. It is an amputation model designed to incorporate native residual muscles and corresponding neural signals for supplementation of proprioceptive feedback and joint control (Clites et al., 2017). Agonist-antagonist muscle pairs in the residual limb are surgically coapted in series so that agonist contraction results in antagonist stretch (Figure 3). Activation of mechanoreceptors in these muscles generates proprioceptive signals that provide the CNS with information related to joint movement. One AMI construct correlates with one DoF and thus control of a prosthesis with two joints requires two AMIs (Clites et al., 2018). Preservation and utilization of the native muscle relationships makes the AMI a unique and promising design for bidirectional communication in a prosthesis.
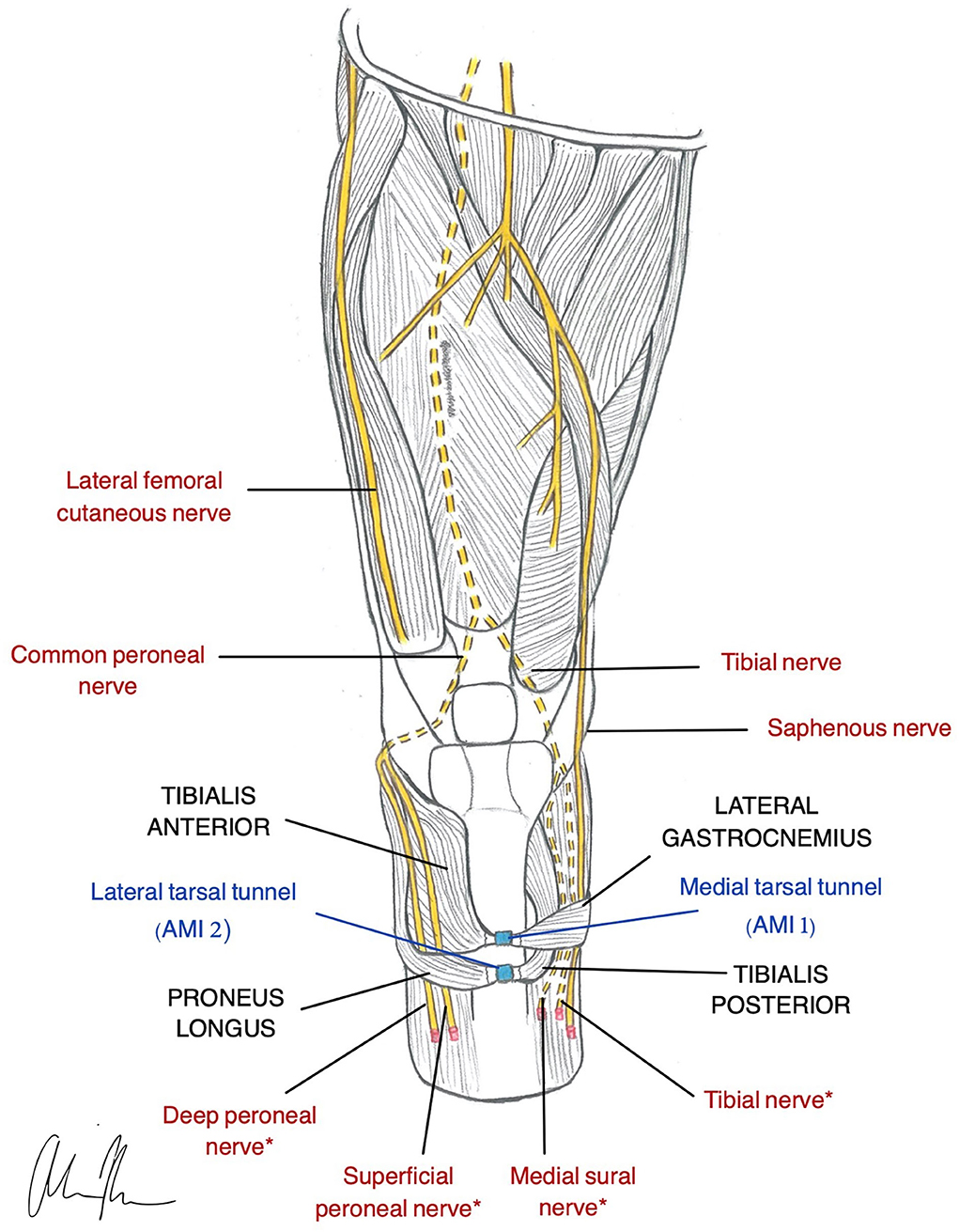
Figure 3. Illustration of AMI construction in a transtibial amputee (Herr et al., 2020). Native relationship between the Lateral Gastrocnemius and Tibialis Anterior is restored using the medial tarsal tunnel (AMI 1). The Tibialis Posterior and Peroneus Longus native relationship is restored using the lateral tarsal tunnel (AMI 2). *nerves are used for RPNI for motor control.
There are a number of different ways an AMI and its corresponding prosthetic joint can be controlled. This is largely dependent on the viability of residual limb. Herr et al. outlines the recommended surgical approaches for BKAs based on availability of distal tissues. If these tissues are fully intact, the AMIs can be controlled through the native neural pathways provided by the agonist-antagonists. If tissue availability is limited, it may be necessary to use alternative approaches such as TMR or RPNI in combination with the AMI for control (Herr et al., 2020). Lastly, if there is no residual tissue remaining, TMR, RPNIs, or 2-stage AMI is used (Srinivasan et al., 2019). If accessible, the AMI muscle pairs are mechanically linked using the tarsal tunnels from the amputated limb. Otherwise, they may be created artificially. Additionally, in any of these scenarios, neurovascular island flaps may be created to improve sensation and fit within a socket prosthetic (Herr et al., 2020). These complex surgical techniques incorporate many of the PNI designs already discussed in this review.
Motor Control
The AMI utilizes native spindle fibers and Golgi tendon organs in paired muscles to restore both motor control and proprioceptive feedback. Although studies are limited, early data suggests that the AMI is capable of generating high quality efferent signals, integrating reflex arcs, and preventing disuse atrophy (Clites et al., 2018; Srinivasan et al., 2019). When compared with four traditional amputees, an AMI patient exhibited natural reflexive behaviors and improved prosthetic control (Clites et al., 2019). This suggests that proprioceptive feedback not only restores sensation, but also enhances joint control and prosthetic function.
Sensory Feedback
The most promising application of the AMI is the restoration of proprioception. While other interfaces such as the cuff electrode have provided some sensory capabilities, they have not successfully incorporated natural proprioceptive feedback (Tan et al., 2015). This is largely due to the complex interplay between mechanoreceptors in the skin, muscles, and joints that make proprioception difficult to achieve (Weber et al., 2012). Proprioception is a unique sensation of which humans are not consciously aware. It allows us to know where our body is in time and space without visual input. Though its mechanisms are not well-understood, the significance of proprioception in motor control and joint stability is well-established (Proske and Gandevia, 2012). This capacity allows us to adapt to changes in the external environment and assists us in the planning of motor commands (Riemann and Lephart, 2002). Furthermore, it contributes to the sense of embodiment necessary for our limbs to feel like they are a part of “us.”
The AMI takes advantage of the body's natural proprioceptive pathways by coupling agonist and antagonist muscle pairs to control and translate feedback from a prosthetic joint. This was first demonstrated in animal models with evidence of graded afferent signals in multiple studies (Clites et al., 2017, 2018; Srinivasan et al., 2019). In humans, the AMI has demonstrated closed-loop joint torque control (Clites et al., 2018), and functional neuroimaging has revealed proprioceptive activity similar to that of individuals without an amputation (Srinivasan et al., 2020). Though the AMI does not provoke natural sensory precepts such as touch, the ability to restore proprioception is undoubtedly just as important a capability.
Management of Symptomatic Neuromas
There is currently minimal published literature discussing the AMI in terms of symptomatic neuromas and postamputation pain. Unlike TMR and RPNI, the AMI was not designed based on amputation neuroma. In the first pilot study (Clites et al., 2018) none of the three patients reported phantom sensations or cutaneous pain post-operatively. However, the authors attribute this to the RPNIs that were created for transected nerves. Although the AMI may not directly prevent or treat symptomatic neuromas, it is conceivable that preservation of native neuromuscular relationships may contribute to a reduction in phantom pain (Karl et al., 2001; Grüsser et al., 2004; MacIver et al., 2008). Future studies should further explore the role of AMI in the management of postamputation pain.
Surgical Application and Prosthetic Compatibility
Of all the proposed surgical techniques, AMI is the most complex and technically challenging approach. It requires an extensive operation (or several), longer hospital stay and recovery time, and a surgeon capable of performing such an advanced procedure (Herr et al., 2020). Thus, far, the AMI has only been used in humans requiring a primary BKA. Recently, Srinivasan et al. explored the possibility of implementing AMI as a secondary, revision surgery in patients who already underwent amputation. This dual staged operation is even more technically difficult than the original AMI approach. Regenerative AMIs are created by identifying and securing nerve fascicles in muscle grafts during the first operation. During the second stage, the appropriate flexor and extensor grafts are then coupled together for functional use (Srinivasan et al., 2019). This revision model provides evidence of viability, graded efferent and afferent signaling, and mechanical stability that is comparable to the original single stage approach (Clites et al., 2017). Surgical models for using AMI in AKAs have been created in animals and have the potential to be translatable in humans (Clites et al., 2019).
In terms of using AMI in Upper extremity amputations, no data is currently published. Given the complexity of upper extremity anatomy and fine motor control, it is likely that the AMI would need to be used in conjunction with TMR and/or RPNI for control of multiple DoF.
The AMI procedure offers an “alternative form of limb reconstruction” designed to augment the residual limb in preparation for a prosthesis. Current studies have used temporary fine wire electrodes for electrical connectivity (Herr et al., 2020). These are not applicable outside the lab and thus surface electrodes or implantable muscle electrodes (without external wiring) would be necessary for clinical use. Nonetheless, the AMI is the first technique designed to mimic the natural gait cycle which relies heavily on proprioception and coordination of fine muscle movements.
Osseointegration (OI)/Osseointegrated Neural Interface (ONI)
Osseointegration is the direct skeletal anchorage of a metal implant to bone. Developed as a method of improving mechanical stability between an bone/implant interface, OI initially found success in the field of dentistry with tooth implants (Jacobs et al., 2000; Brånemark et al., 2001). Its application was eventually extended to extremity prosthetics in response to the challenges and complications associated with traditional socket prostheses. Unlike the socket-based prostheses, OI provides reliable mechanical stability, physiological load bearing, and increased range of motion (Al Muderis et al., 2018; Brånemark et al., 2019; Ackerley et al., 2020; Hagberg et al., 2020). This facilitates ease of use and promotes continued prosthetic use.
The principles of OI, combined with concepts of nerve regeneration, led to the creation of a novel peripheral nerve interface design, the Osseointegrated Neural interface (Figure 4). Dingle et al. built this interface based on the idea that the intramedullary canal can provide a stable and protective environment for nerve regeneration (Dingle et al., 2020b). Although transposing nerves into bone was established as a treatment for symptomatic neuromas almost eight decades ago (Boldrey, 1943), the ONI takes the application one step further, demonstrating the intramedullary environment's ability to provide the stability required for the implementation of more selective, invasive electrodes (Dingle et al., 2020b). Like TMR and RPNI, the ONI is rooted in the treatment of symptomatic neuromas, while its application for prosthetic control remains experimental.
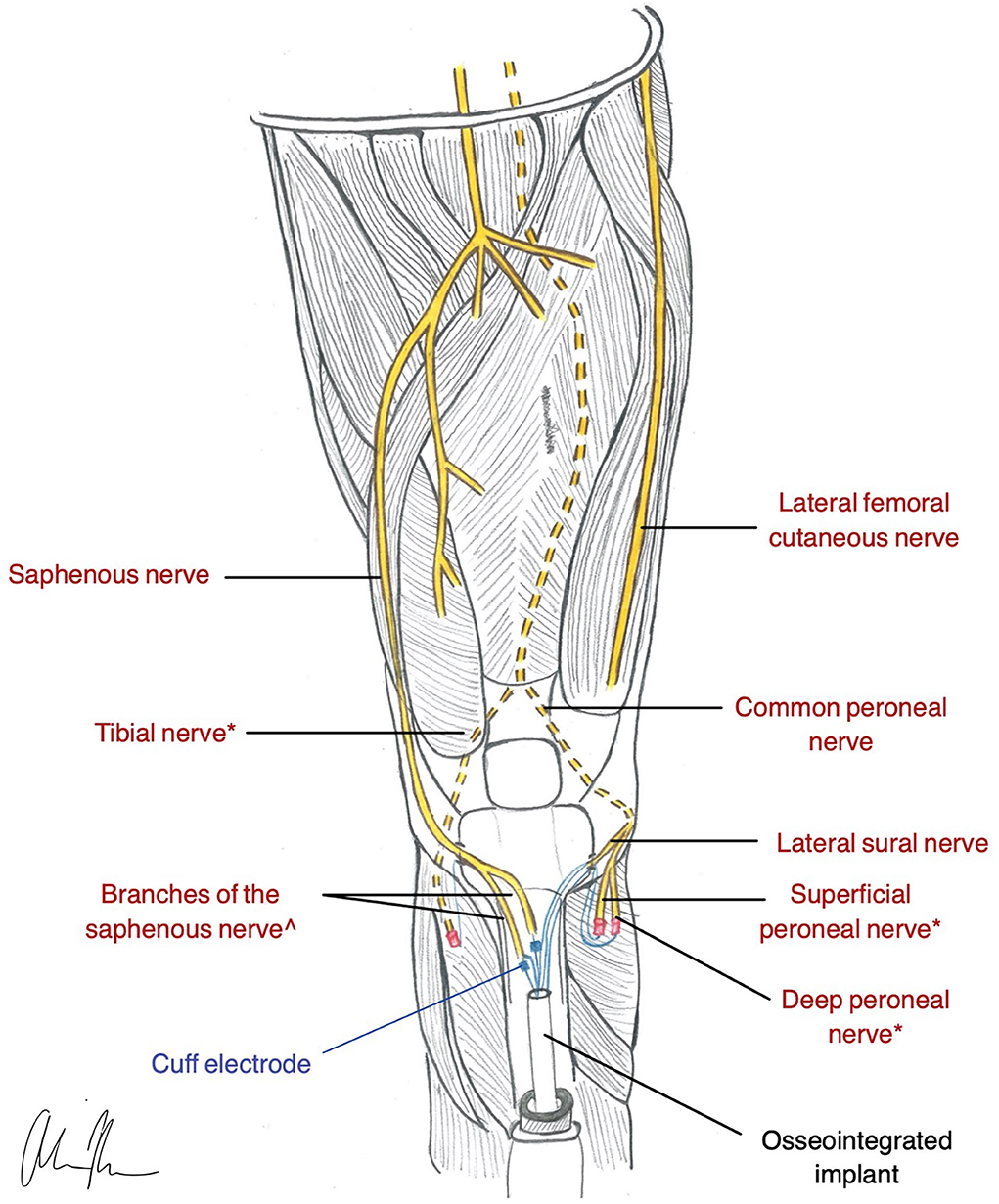
Figure 4. Illustration of ONI construction in a transtibial amputee (Dingle et al., 2020b). * nerves are used for RPNI for motor control. ^nerves are used with a cuff electrode for sensation and are inserted into medullary canal via corticotomy in the Tibia.
Motor Control
The ONI has not yet been evaluated in humans, but animal studies have provided promising results. A rabbit model with an intramedullary cuff electrode demonstrated evidence of nerve regeneration with the ability to produce efferent and afferent signals over 3 months (Dingle et al., 2020a). If this result can be translated to even more invasive electrodes such as intraneural or regenerative electrodes, the ONI may be capable of providing bi-directional signaling with high fidelity control. Additionally, the increased range of motion provided by OI could further supplement functionality.
Sensory Feedback
Osseointegration is capable of producing sensation on its own through the mechanical stimulation generated from a bone anchored prosthesis. This has been termed osseoperception or osseoproprioception and is thought to be an important factor in prosthetic usability (Jacobs et al., 2000; Klineberg, 2005). The underlying mechanisms that contribute to osseoperception are not well-understood and remain a subject of debate (Mohan Bhatnagar et al., 2015). Despite this uncertainty, there is evidence in the literature demonstrating the benefits of osseoperception. Subjectively, osseoperception has been identified as an improved perception of the environment with an increased awareness of a prosthesis (Jacobs et al., 2000). When compared with individuals with socket prostheses, those with an osseointegrated prosthesis experience an increased sensitivity to vibration (Häggström et al., 2013; Clemente et al., 2017). Furthermore, numerous studies have shown that osseointegration is associated with improved patient reported outcomes, better prosthesis-associated quality of life, and increased mobility (Brånemark et al., 2019; Hagberg et al., 2020; Pospiech et al., 2020).
Although osseoperception improves vibratory sensation and prosthetic embodiment compared with traditional socket users, it does not provide sufficient sensory feedback for restoring grasp behavior and improving motor coordination (Mastinu et al., 2019). The single abutment point in OI implants limits the available stimulation points for sensory feedback. However, Mayer et al. demonstrated that supplementary tactile feedback can be transmitted simultaneously through a single feedback channel to achieve adequate performance in these tasks (Mayer et al., 2020). This addresses the challenges of conveying information through one channel by improving its efficiency. Additionally, hearing has been shown to play a role in the improved sensation experienced by those with osseointegration, compared with those with a socket prosthesis (Clemente et al., 2017). Combing tactile feedback with hearing creates an additive effect that further improves prosthetic performance.
In relation to the ONI, there is additional potential for restoring sensation through the nerve electrodes housed in the intramedullary canal. As mentioned above, the ONI rabbit model was capable of both efferent and afferent signaling, and thus sensation could potentially be restored in this manner (Dingle et al., 2020b). The more invasive the electrodes are, the greater potential there is for establishing highly selective stimulation.
Management of Symptomatic Neuromas
The original design of the ONI was created on the basis of using the intramedullary canal of long bones to prevent symptomatic neuromas. This method was first established many years ago (Boldrey, 1943) and is based on the premise that the medullary cavity provides an insulated environment that can prevent a regenerating nerve from erroneously innervating muscles and skin. This prevents neuropathic pain by protecting the exposed nerve from external stimuli (Israel et al., 2018). The ONI not only takes advantage of neuronal regeneration for electrical signaling purposes but also for the prevention of symptomatic neuromas. Pain is prevented by placing axons in an environment that restricts neuronal regeneration and innervation to electrode sites rather than problematic areas such as skin.
Surgical Application and Prosthetic Compatibility
Osseointegration can be performed as a single stage operation (Al Muderis et al., 2017) or through a dual-staged approach. There is currently no standardized protocol for either strategy. Instead, the various surgical techniques tend be institution-based or implant dependent. Overall, the concept of OI is designed to be applied to amputations of any level. Although more attention has been given to the lower extremity amputations, it has been successfully used in upper extremity amputations as well (Jönsson et al., 2011). OI has been used throughout Europe and Australia for the last few decades; however, it was only recently approved in the United States by the Food and Drug Administration (FDA) via a humanitarian use device exemption. There are currently five implant options being used worldwide (Zaid et al., 2019).
As outlined above, individuals with OI experience superior outcomes compared to those with socket prostheses. However, there are challenges and potential issues related to OI that should be considered. The biggest concern with an OI implant is infection. Fortunately, the majority of OI- related infections are superficial and are successfully treated with outpatient antibiotics (Overmann et al., 2020). The risk of osteomyelitis is reported to be around 10% (Jacobs et al., 2000; Tillander et al., 2017). Nevertheless, management of the soft tissue interface is critical to preventing a deep infection and subsequent implant failure. Strategies for infection prevention involve preserving tissue adherence and tissue perfusion to optimize survival (Souza et al., 2020). Additional mechanical complications that may contribute to implant failure include loosening or failure of fixation and peri-implant fracture. A prospective study of 51 patients with TFA revealed a 5-year cumulative fixture survival rate of 92% a revision-free survival rate of 45%. Eleven patients experienced mechanical complications, with 3 implants requiring removal secondary to loose fixation (Brånemark et al., 2019).
Overall, the ONI has the potential be a revisionist surgery capable of improving functionality and maximizing usability through integration of a peripheral nerve interface and an osseointegrated implant for intuitive prosthetic control and prevention of neuropathic pain all in one procedure.
Control Methods
Myoelectric Control Options
Creating a system that is capable of naturalistic and intuitive prosthetic control is dependent on more than just interface design. It requires control methods that employ algorithms to interpret extracted EMG signals for the prediction of intended postures and movements. These non-invasive strategies can provide sophisticated control when there is poor selectivity of signals due to cross-talk from surface electrodes or if there is a lack of stability with invasive electrodes.
Conventional Control Methods
The most simple and common control method utilized in myoelectric devices is conventional or direct control. This uses two EMG signals from one muscle pair to control one DoF. Control of more than one DoF requires a mode switch with co-contraction of the muscle pair. This switching is cumbersome and can be cognitively challenging with increasing complexity (Young et al., 2014). Furthermore, direct control typically uses surface EMG electrodes, limiting the overall efficacy due to interfering signals from EMG crosstalk. In order to achieve simultaneous control of more than one DoF there must be at least four control sites available with minimal EMG cross talk (Farina et al., 2014). This is not possible in many upper limb amputees unless they have additional control sites through TMR or RPNI. As a result, this type of control can feel unnatural and is often restricted to simple movements (Hargrove et al., 2017).
Pattern Recognition Algorithms
In an effort to improve direct control, pattern recognition algorithms have been developed. This method identifies a pattern of signals provided by multiple electrodes and interprets it as a pre-defined movement or posture. This is particularly important for restoring function in higher level amputees as it has the ability to increase DoF despite missing residual joints. Although this provides intuitive control, it does not allow for simultaneous control of multiple motions. Instead, they must occur in sequence, limiting the ability to restore natural feel (Li et al., 2010). Furthermore, pattern recognition requires intensive training sessions and is cognitively demanding. It. depends on repetition of same the movements and thus any changes in the EMG patterns may lead to worsening performance.
Despite these limitations, there has been success with pattern recognition in the virtual and off-line setting (Hargrove et al., 2018), but very limited data to support its use in the clinical setting. The translation of off-line performance to online performance with real time feedback is uncertain (Farina et al., 2014; Hargrove et al., 2017). The potential deterioration of performance in an at-home setting poses a dangerous risk to both the individual and the integrity of the prosthetic device. However, Hargrove et al. recently demonstrated improved performance with pattern recognition compared to direct control in transhumeral amputees in the very first take-home trial (Hargrove et al., 2018). There are supplementary control strategies that can help address the shortcomings of pattern recognition. Collecting data on joint position (Adewuyi et al., 2017) and reducing classification errors made by variations in muscle contraction and mobility (Samuel et al., 2019) can help improve performance. Though these additional control strategies can provide simultaneous control and enhance overall use (Young et al., 2013, 2014; Ortiz-Catalan et al., 2014), they require complex algorithms and rigorous training for high performance achievement.
Regression Based Algorithms
A potential solution for achieving simultaneous and proportional is regression-based control. This algorithm allows for classification of multiple movements at one time through estimation of different EMG signals. This provides intuitive control of multiple DoFs at the same time (Smith et al., 2015; Wendelken et al., 2017; Hahne et al., 2018). For example, a person could extend their elbow and rotate their wrist simultaneously. In comparison to conventional control methods or pattern recognition, regression-based methods have demonstrated improved performance with independent control of movement velocity and a more natural feeling (Kuiken et al., 2016; Hahne et al., 2017, 2018). Although this type of control has promising potential, similar to pattern recognition, the differences between the training environment and real-life make it challenging for use outside the laboratory setting (Jiang et al., 2009). Progress is slowly being made with an 8-week at home trial demonstrating successful application in everyday life with regression performance exceeding conventional control (Hahne et al., 2020). More longitudinal studies are needed to demonstrate stability of performance over time.
ENG Signal Enhancement and Processing
Similar to EMG based control methods, ENG signals can also be analyzed for the application of neurprostheses. These methods address the challenges posed by EMG control including muscle fatigue, stimulation of surrounding tissues, and desensitization of cells. Direct interfacing with neural electrodes can help with specificity of signal but face their own obstacles with biocompatibility and tissue damage related to their level invasiveness. The best physical interface will balance the electrical properties of the system to isolate different sensory precepts, while maintaining a safe and stable environment. Techniques have been employed to improve usability of neural electrodes through changes in electrode configuration, electrical connectors, biocompatibility, and surgical techniques to not only enhance longevity but also facilitate signal extraction and processing.
Recording
ENG signals are recorded from peripheral nerves differently depending on the invasiveness of the electrode that is used. Extraneural electrodes, such as the cuff, record a population of signals with information from the overall nerve, whereas intraneural electrodes penetrate the nerve and record spike activity from individual axons (Hong et al., 2018). The raw ENG signals have low signal to noise ratio and are impacted by interference from surrounding muscles, micromotion, and neighboring axons (Tam et al., 2019). Thus, pre-processing with wavelet denoising and different types of filters are necessary prior to channel selection (Micera et al., 2011). This step in processing is complex and expensive, requiring dimensionality reduction algorithms or component analysis with significant computational power.
When population activity recordings are obtained, as in the case of extraneural electrodes, both motor and sensory activity is included and thus algorithms must be used to separate the sources so different features can be extracted. In the case of intraneural electrodes neural spikes are recorded and can be processed using spike sorting algorithms (Cracchiolo et al., 2020). Different axons will generate unique spike activity that is associated with a distinct motor activity. Once the sorted spike signals and population activity features are extracted, they can be used with classification algorithms for prediction of movement intention (Raspopovic et al., 2014). The details of these classification algorithms are beyond the scope of this review.
In humans there have been several successful demonstrations of ENG signal decoding and classification of hand movements. The spike denoising and sorting algorithms as described above have been used with tLIFEs (Micera and Navarro, 2009; Micera et al., 2011) and USEAs (Davis et al., 2016). More recently multi-class neural motor decoding with TIME allowed for velocity and force predictions for 11 different grasping positions (Cracchiolo et al., 2021).
Though ENG recording and processing can improve the specificity of signals, there are inherent limitations. The immune response to the implanted electrodes promotes fibrosis over time, eventually leading to electrical impedance and difficulty in recording signals (Raspopovic et al., 2020). In terms of stimulation this can be overcome with increased injection of charge (de la Oliva et al., 2018), however, this does not improve the ability to record motor signals in the peripheral nerves.
Stimulation
Restoration of sensory feedback and stimulation of peripheral nerves is inherently challenging given the complexity of the somatosensory system. Sensory axons outnumber motor axons by at least 10:1. Unlike with motor control where a constant firing can produce a movement, stimulation for sensation requires an interpretation of signals originating from a multitude of different receptors. It is the differential firing between these fibers that elicits the sensation of natural touch. A constant firing instead will generate a very unnatural sensation (Dhillon and Horch, 2005). In terms of translating natural sensation in a prosthetic device, the sensations stimulated must resemble the spatial and temporal characteristics of an intact limb (Horch et al., 2011; Raspopovic et al., 2014; Tan et al., 2015). Neural modulation using pulse shape and frequency can determine quality and intensity of the sensation that is being perceived, respectively (Tyler, 2015; Gracyzk et al., 2016; Valle et al., 2018). Biomemetic encoding strategies can generate optimal stimulation patterns that can effectively produce natural sensation, reduce phantom limb pain, and improve prosthetic embodiment (Raspopovic et al., 2021).
Although signal processing may be easier with stimulation, compared to motor recording, there are concerns regarding safety of electrical stimulation. This involves biostability and consideration of tissue response, as well as identification of acceptable stimulation parameters. Specifically with stimulation of sensation there are numerous different peripheral nerve fibers each with a unique ability to withstand electrical stimulation (Johansson and Flanagan, 2009). Extraneural electrodes currently demonstrate superior stability compared to intraneural electrodes given the level of invasiveness (Günter et al., 2019). However, more work must be done to classify neuronal damage and identify the limits of stimulation parameters to ensure long term safety.
Electrodes must be designed in a way that takes advantage of electrical characteristics using computational modeling for optimization of contacts and subsequent isolation of specific sensory qualities without damaging the surrounding tissue. This allows for processing and interpretation of sensory information in a way that makes the sensation feel like an intact limb. Furthermore, this sensory information closes the loop and provides accurate output information that can be used for advancing motor decoding algorithms.
Prosthetic Technology
All of the electrodes, surgical techniques, and control methods discussed thus far have been designed to create an interface capable of high-fidelity prosthetic control. These methods have focused on manufacturing a biocompatible, closed looped system with the ability to transmit bidirectional signals for prosthetic control. While these developments have improved functionality, they do not address all of the problems associated with usability. This is influenced by the prosthetic device itself. Device characteristics such as availability, capability, fit of the socket, durability, and cost are all instrumental in implementing daily use of an advanced prosthesis (Samuel et al., 2019). The following section investigates the barriers to clinical translation and subsequent take-home use of the different interfaces.
Fully Implantable System
In order to implement this type of prosthetic system, it must be fully implantable. In other words, there can be no external wires protruding through the skin for electrical connections. This type of wiring is prone to breakage, infection, and thus is limited to the lab setting. While more invasive electrodes can improve signal transmission, it requires percutaneous wired electrodes. Solutions to combatting this problem include wireless signal transmission or use of an osseointegrated implant. Creating a reliable, wireless communication system with preserved signal strength is a challenging endeavor. It requires the use of appropriately packaged electronics capable of efficient and safe power management in a way that does not require larger or more implantable hardware (Borton et al., 2013; Seo et al., 2016). The most successful wireless design thus far is the IMES system, which uses implantable sensors within the residual limb for prosthetic control (Weir et al., 2009). It is currently being used in clinical trials and has demonstrated effective and reliable signaling in TMR patients (Merrill et al., 2011; Pasquina et al., 2015). Other potential designs explore the use of magnetic beads (Herr et al., 2020) and implantable capsules. In rodents, implantable capsules have demonstrated reliable and robust signal transmission using small electronics and inductive battery power (Deshmukh et al., 2020).
Osseointegration offers a solution for the external, percutaneous wiring that does not require wireless communication. The implant uses an abutment fixated within the bone that can be used to house the wired electrical connectors (Ortiz-Catalan et al., 2014). Although the OI implant is percutaneous, the electrical wiring housed within is protected from the problems associated with the external environment.
Successfully integrating a fully implantable system is the first step in creating a device that is ready for home and clinical use. For this to be applicable for long-term use, other considerations must be taken into account such as the feasibility of hardware implantation and/or removal (if necessary), as well as the long-term safety of bioelectronics.
Real Time Performance vs. Offline Performance
In addition to physical connections and environmental interference, there are various requirements for signal processing that complicate use of myoelectric prostheses outside of the lab. The laboratory environment represents a controlled, predictable setting that is far different from the dynamic home environment. Conditions within the lab do not simulate real life situations and cannot account for unpredictable, changing movements associated with activities of daily living. The lack of dependability and the potential for unwanted movements creates a dangerous situation that may harm the individual or damage the prosthetic device. While performance in the offline setting demonstrates successful outcomes, it is measured using passive data collection and classification accuracy– the ability of the algorithm to identify certain movements (Nilsson et al., 2017). Though the classification accuracy may be high, the completion rate of tasks can be up to 30% lower (Li et al., 2010). Thus, classification accuracy does not necessarily correlate with functional outcomes in real time control and is not a sufficient comparison tool for control methods. Instead, it is essential that online performance testing be used for a more accurate assessment (Jiang et al., 2009; Ortiz-Catalan et al., 2014; Hargrove et al., 2018). This involves additional interpretation of data during active movement for improved real-time performance (Woodward and Hargrove, 2019). When practiced over a long period time, the EMG patterns become more easily repeatable and adaptable with sustained high-quality performance (He et al., 2015).
The metrics to evaluate performance are often arbitrary, making it challenging to compare outcomes between studies (Tabor et al., 2018). Without standardized criteria, it is difficult to objectively state how “selective” a particular interface is. In order to draw meaningful conclusions, comparative studies with standardized outcome measurements are needed. This involves a systematic evaluation throughout training—assessing progress, functional outcomes, and quality of life (Balk et al., 2019). Until more standardized assessments are implemented, it will be difficult to accurately predict function outside of the lab.
Training Systems
Despite the increasing sophistication of machine learning algorithms and artificial intelligence, user training remains a critical component of functional success. Standard rehabilitation programs are rigorous and focus on regaining muscle strength and coordination. The tasks are both mentally and physically exhausting, requiring long-term commitments. This process can become especially challenging when an individual no longer has a coach or a therapist to provide motivation (Prahm et al., 2017). Many of the conventional training methods are laboratory-based and lack the mental stimulation required to keep users engaged. To increase motivation and participation, alternative training systems with a game-based model have been developed. These include computer gaming, virtual reality environments, and augmented reality (Resnik et al., 2011; Winslow et al., 2018; Boschmann et al., 2021). Several studies have demonstrated successful prosthesis control with these training systems, in addition to individuals reporting increased usability and motivation (Tabor et al., 2018; Kristoffersen et al., 2021). Not only do these methods improve participant engagement, but they also provide an at-home training system that is more convenient and affordable than daily sessions with a physiotherapist.
Additionally, these training programs help address the remapping of the somatosensory cortex in individuals postamputation. These changes in cortical organization and structural morphology are thought to contribute to phantom limb pain and impact functional ability, hindering prosthetic use (Zhang et al., 2018). While motor cortex may remap, the sensory cortex appears to remain more fixed throughout adulthood with studies showing visuo-tactile mismatches that do not resolve (Ortiz-Catalan et al., 2020). However, numerous studies have demonstrated that prosthetic rehabilitation programs can positively impact neural plasticity with a reduction in phantom limb pain and improved function (Preißler et al., 2017). This has been seen in upper limb amputees with improved grasping and manipulation of objects (Cuberovic et al., 2019) and in lower limb amputees with improved posture control and balance (Dietrich et al., 2018; Bramati et al., 2019).
While these alternative training methods demonstrate functional outcomes like conventional methods, they address a key cause of prosthetic abandonment: lack of motivation and an unwillingness to commit to a rehabilitation program. Despite these challenges, it is necessary to activate mechanisms of the brain that contribute to changes in somatosensory plasticity, a critical component of nerve recovery.
Commercial Devices
While innovative surgical techniques and complex control methods continue to expand possibilities for amputees, function is largely dictated by the actual prosthetic device. The decision to pursue a bionic limb is mainly determined by affordability and availability. Upper limb devices have become increasingly sophisticated with several companies designing different devices and producing prosthetic components. Though these bionic hands have yet to achieve the same dexterity as the natural fingers and thumb, they are capable of completing activities of daily living. The cost of these below the elbow devices range anywhere from 8,000 to 60,000 USD. Above the elbow options are even less affordable, costing a minimum 50,000 to upwards of 100,000 USD. There are less market options for bionic limbs of the lower extremity, however, the devices that are available are similar in cost to those of the upper extremity. Unfortunately, given the limited availability and affordability of many of these devices, individuals may find themselves needing to purchase different components from different companies to complete their prosthesis. Additionally, there is a lack of communication between the stakeholders in device design and prosthesis control. In an effort to mitigate these problems and improve collaboration within the field, the University of Michigan has developed an open-source initiative for bionic limbs (https://opensourceleg.com). A more viable prosthetic solution capable of supplying the market's needs would improve availability and help with cost reduction as competitors adopt it.
Prosthetic Embodiment/Sensation
Overall use of a prosthesis is not only dependent on availability or operability, but most importantly, on an individual's desires and goals. This may be related to occupation or hobbies, or to their level of function before the amputation. It may even be influenced by a patient's motivation and dedication to the training required to use one of the advanced prosthesis (Kerver et al., 2020). Furthermore, co-morbidities and health status may limit an individual's ability to explore more invasive options. Whatever the case, it is likely that the primary objective will vary between individuals. Thus, the conversation regarding goals is an essential one that must take place early so that expectations can be established and prosthesis use can be optimized.
While comparative studies and long-term effectiveness of prostheses remain limited, there is research exploring prosthesis abandonment and the qualities that are most important to the individuals who are using them. A survey study by Zheng et al. found that improved dexterity, durability, and sensation were the most important qualities for amputees (Zheng et al., 2019). Similarly, a case series with 3 female amputees found advanced prostheses to be desirable if they increased abilities and if device support was available (Resnik et al., 2019). The most common concerns regarding these devices include cost, durability, invasiveness of surgery, and ease of use (Engdahl et al., 2015, 2017; Resnik et al., 2017). Though many of these studies demonstrate shared desires, they do not necessarily indicate shared experiences or lifestyles.
Improvement of sensation is a critical area of ongoing research in the field of prosthetics. Numerous studies have demonstrated the value of sensation in improving control, adaptability, and embodiment (Sinha et al., 2011; Schiefer et al., 2016, 2018). Prosthesis abandonment rates range anywhere from 24 to 44%, with lack of sensation being a significant contributing factor (Biddiss and Chau, 2007; Salminger et al., 2019). When an individual is unable to feel their prosthetic limb, it does not feel like a part of their body or self and discourages overall use. This lack of sensation can put both the user and the device at risk for harm and damage. Additionally, when sensation is restored, there are a number of psychosocial factors that improve including social interaction, sense of self, and overall quality of life (Graczyk et al., 2018; Page et al., 2018; Petrini et al., 2019a; Middleton and Ortiz-Catalan, 2020).
After a type of prosthesis is decided upon, rehabilitation and incorporation of the device must occur. This process involves a large multidisciplinary team that extends beyond the surgeons and engineers who helped create the device. Wound care specialists and medical management are important for ensuring sufficient recovery and appropriate fitting of a prosthesis. Occupational therapists, physical therapists, and prosthetists help prepare an individual for prosthesis training (Cancio et al., 2019). Early rehabilitation and training are not only necessary for maximizing functionality, but are also associated with improved patient satisfaction (Resnik et al., 2020). This training can occur long before an individual is even fitted for a prosthesis.
Discussion
During the last few decades, considerable strides have been made in the field of neuroprosthetics. With advanced robotic technology and revolutionary surgical techniques, the use of a fully integrated, bidirectional prosthesis is now within the realm of possibility for amputees. Though the technology is available to make these improvements, there is division within the industry that makes it challenging to take technical ideas and translate them into the commercial world. One of the most significant limiting factors for this translation is the inability for one PNI to carry out all the functions required to fully operate an advanced neuroprosthetic. Individually, the existing interfaces have significantly advanced or improved a particular aspect of the bidirectional system (Table 1). For example, extraneural electrodes have demonstrated chronic long-term stability, while intraneural electrodes offer a promising solution for high-fidelity signaling. Additionally, neural electrodes have shown their ability to restore sensory feedback and subsequently enhance motor control with improved object manipulation and force control (Tan et al., 2014; Oddo et al., 2016). This allows for precise stimulation capable of eliciting natural-like sensations while balancing specificity and cognitive load. Repetitive motor input with visual feedback alone is not capable of adjusting sensory cortical maps (Ortiz-Catalan et al., 2020). Although direct stimulation of the nervous system can successfully restore tactile sensations, proprioceptive precepts are much more challenging.
While many existing surgical techniques have not been as successful in providing sensory feedback, they have demonstrated sophisticated motor capabilities. TMR provides movement for missing muscles with multiple DoF, whereas RPNI has demonstrated motor control selective enough for movement of intrinsic hand muscles. Osseointegration allows for better mechanical stability and increased ROM, and ONI has the potential to provide high fidelity signaling through the use of regenerative electrodes within the medullary canal. Additionally, all of these surgical techniques are a treatment option for postamputation pain and symptomatic neuromas. Although the AMI does not directly improve motor control or treat neuromas, it is the first PNI to restore proprioceptive feedback. Summation of the advantages and disadvantages of the electrodes and surgical techniques are outlined in Table 2.
Despite all the progress that has occurred in specific areas of the field, no single PNI can perform the needed functions all on its own. The path forward requires a comprehensive approach with collaboration between all stakeholders—surgeons, biomedical engineers, prosthetists, device companies, and most importantly, the patient. Different technologies with unique capabilities should be combined to best suit the patient's needs and optimize quality of life. Until recently, most innovation within the prosthetic field has been created in silos. Although this work has led to substantial progress, without coordination, it can be redundant and inefficient, and only further perpetuates the gap between academia and clinical utility. A collaborative approach encourages facilitation of standardized performance metrics and promotes translation of advanced prosthetics into the commercial, and thus practical world. The technology is available; what is needed is a way to synthesize and coordinate the diverse work.
Fortunately, the field has already begun to see some joint efforts come to fruition. For example, the use of TMR with neural and muscular electrodes in upper extremity amputees allows for bidirectional communication and improved somatosensory control (OrtizCatalan et al., 2020). In addition, use of osseointegration further increases range of motion and prosthetic embodiment (Vincitorio et al., 2020). Combination of TMR and RPNI capitalizes on the advantages of each individual design to optimize efficacy (Valerio et al., 2020). Even new surgical constructs such as the AMI rely on the integration of other PNI designs to provide more comprehensive functions (Herr et al., 2020). Though AMI can restore proprioception, it depends on concurrent use of TMR and/or RPNI for motor control and neuroma treatment. Similarly, the ONI takes advantage of neuronal regeneration and the medullary canal environment to optimize use of regenerative nerve electrodes (Millevolte et al., 2021). Potential combination of different PNIs is presented in Table 3. There are many variations listed, but this table is not exhaustive. While these synergistic combinations demonstrate the advantages of collaboration, they are only the beginning. Currently none of these technologies are suited to meet the broader amputee community's needs. As these technologies extend into clinical trials, as TMR and RPNI have (at least for treating neuroma), they are then going to have to meet all of the regulatory requirements of each country/region if they are to be broadly accepted and clinically applied. As with any medical device, each implantable device required for prosthetic control is going to need to be classified for regulatory purposes, which in the US comes under the jurisdiction of the Food and Drug Administration, as stated in the Code of Federal Regulations for medical devices (Title 21, 800 series).
Incorporation of this technology will be dependent on the patient, technological availability, and surgical implementation. The potential need for secondary surgery is not yet clear given the infancy of these devices. In the majority of cases, these devices have been implemented in persons already living with amputation, who may be returning to have neuropathic/phantom pain managed, at which point an interface is implanted as part of an already necessary secondary procedure (Di Pino et al., 2014). However, use of the surgical techniques such as TMR and RPNI, have been performed at the time of amputation to prophylactically prevent neuropathic/phantom pain (Santosa et al., 2020). For many involved in prosthetic control studies, patients have been heavily vetted to meet long-term study requirements. As evidenced by the increasing uptake of osseointegration, constant refinements have enabled what is more commonly a two-step surgical procedure to be reduced to a single surgical procedure as the technologies and techniques have become more refined with practice (Hoellwarth et al., 2020). The decision by patients over what is more suitable and more widely accepted is going to be heavily based on what the technology can achieve relative to what the individual desires. This is highlighted by the increased uptake of osseointegration by patients of standard socket prosthesis, given that it is a highly invasive, secondary procedure, increasing numbers of patients are seeking OI globally for its improved functionality and the subsequent improvements in quality of life (Souza et al., 2020). From the perspective of sensory restoration, there is yet to be a technology that does not require implantation of an electrode to provide chronic restoration of sensation.
Given the current state of technology and adaptive surgical strategies, there are theoretically a multitude of unique PNI combinations that can be tailored to an individual's needs in order to maximize function and improve quality of life. In the case of a lower extremity amputee with the desire to return to his love of hiking, TMR or RPNI, AMI, OI, and a FINE electrode could be used to provide sophisticated motor skills, proprioception, increased ROM, and tactile sensory feedback, respectively. If an amputee with a socket prosthesis already underwent TMR surgery but is seeking further functional improvement, the ONI can be performed as a revisionist procedure with installation of an osseointegrated implant for improved usability and use of neural electrodes for sensory feedback. If the primary goal is optimizing prosthetic embodiment, either the AMI or ONI should be used for restoration of proprioception or osseoperception, respectively. When an individual is not interested in using a prosthesis, but is seeking optimal pain control, TMR, RPNI, and ONI are all viable options for prevention or treatment of postamputation pain. These surgical approaches alone provide a wide range of application to all of the currently available interfaces, including those considered most invasive. Different combinations will be more suitable for certain individuals than others. While an ultimate decision relies on availability of technology and surgical accessibility, it is still largely dependent on a patient's desires. Even if there were a single PNI that could “do it all,” it likely would not be suitable for every patient. Thus, the goal should not be directed at developing a universal prosthesis but should instead be aimed at employing creative strategies to encourage collaboration and provide unique options that can be tailored to each individual.
Conclusion
In this review, we summarize the current landscape of Peripheral Neural Interfaces and the progress that has been made to advance the field of prosthetics. While the technological advancements in prosthetic devices have been remarkable, their use is severely limited by market availability, take home ability, and lack of sensation. Moreover, progress in the field has not been coordinated and this has limited the ability to serve patients' needs and optimize their quality of life. In this comprehensive investigation, we argue for the importance of collaboration and the use of interfaces in combination with each other to offer each patient the best quality of life.
Author Contributions
AK developed the concept and idea with support and help from other contributing authors, and took the lead in manuscript writing. AD provided support in concept development, manuscript writing, and provided critical feedback in manuscript revision. SP offered support in concept development, manuscript writing, and provided critical feedback in manuscript revision. All authors contributed to the article and approved the submitted version.
Conflict of Interest
The authors declare that the research was conducted in the absence of any commercial or financial relationships that could be construed as a potential conflict of interest.
References
Ackerley, R., Wasling, H. B., Ortiz-catalan, M., Brånemark, R. P., Wessberg, J., Al Muderis, M. M., et al. (2020). Invasive neural interfaces: the perspective of the surgeon. J. Neuroeng. Rehabil. 6, 1–12.
Adewuyi, A. A., Hargrove, L. J., and Kuiken, T. A. (2017). Resolving the effect of wrist position on myoelectric pattern recognition control. J. Neuroeng. Rehabil. 14, 1–11. doi: 10.1186/s12984-017-0246-x
Agnew, S. P., Schultz, A. E., Dumanian, G. A., and Kuiken, T. A. (2012). Targeted reinnervation in the transfemoral amputee: a preliminary study of surgical technique. Plast. Reconstr. Surg. 129, 187–194. doi: 10.1097/PRS.0b013e3182268d0d
Al Muderis, M., Lu, W., Tetsworth, K., Bosley, B., and Li, J. J. (2017). Single-stage osseointegrated reconstruction and rehabilitation of lower limb amputees: the Osseointegration Group of Australia Accelerated Protocol-2 (OGAAP-2) for a prospective cohort study. BMJ Open 7, 1–4. doi: 10.1136/bmjopen-2016-013508
Al Muderis, M. M., Lu, W. Y., Li, J. J., Kaufman, K., Orendurff, M., Highsmith, M. J., et al. (2018). Clinically relevant outcome measures following limb osseointegration; systematic review of the literature. J. Orthop. Trauma 32, e64–e75. doi: 10.1097/BOT.0000000000001031
Aristovich, K., Donegá, M., Blochet, C., Avery, J., Hannan, S., Chew, D. J., et al. (2018). Imaging fast neural traffic at fascicular level with electrical impedance tomography: Proof of principle in rat sciatic nerve. J. Neural Eng. 15:056025. doi: 10.1088/1741-2552/aad78e
Balk, E. M., Gazula, A., Markozannes, G., Kimmel, H. J., Saldanha, I. J., Trikalinos, T. A., et al. (2019). Psychometric properties of functional, ambulatory, and quality of life instruments in lower limb amputees: a systematic review. Arch. Phys. Med. Rehabil. 100, 2354–2370. doi: 10.1016/j.apmr.2019.02.015
Bates, T. J., Fergason, J. R., and Pierrie, S. N. (2020). Technological advances in prosthesis design and rehabilitation following upper extremity limb loss. Curr. Rev. Musculoskelet. Med. 13, 485–493. doi: 10.1007/s12178-020-09656-6
Biddiss, E., and Chau, T. (2007). Upper limb prosthesis use and abandonment: a survey of the last 25 years. Prosthet. Orthot. Int. 31, 236–257. doi: 10.1080/03093640600994581
Boldrey, E. (1943). Amputation neuroma in nerves implanted in bone. Ann. Surg. 118, 1052–1057. doi: 10.1097/00000658-194312000-00011
Boretius, T., Badia, J., Pascual-Font, A., Schuettler, M., Navarro, X., Yoshida, K., et al. (2010). A transverse intrafascicular multichannel electrode (TIME) to interface with the peripheral nerve. Biosens. Bioelectron. 26, 62–69. doi: 10.1016/j.bios.2010.05.010
Borton, D. A., Yin, M., Aceros, J., and Nurmikko, A. (2013). An implantable wireless neural interface for recording cortical circuit dynamics in moving primates. J. Neural Eng. 10:026010. doi: 10.1088/1741-2560/10/2/026010.An
Boschmann, A., Neuhaus, D., Vogt, S., Kaltschmidt, C., Platzner, M., and Dosen, S. (2021). Immersive augmented reality system for the training of pattern classification control with a myoelectric prosthesis. J. Neuroeng. Rehabil. 18, 1–15. doi: 10.1186/s12984-021-00822-6
Bowen, J. B., Ruter, D., Wee, C., West, J., and Valerio, I. L. (2019). Targeted muscle reinnervation technique in below-knee amputation. Plast. Reconstr. Surg. 143, 309–312. doi: 10.1097/PRS.0000000000005133
Bowen, J. B., Wee, C. E., Kalik, J., and Valerio, I. L. (2017). Targeted muscle reinnervation to improve pain, prosthetic tolerance, and bioprosthetic outcomes in the amputee. Adv. Wound Care 6, 261–267. doi: 10.1089/wound.2016.0717
Brånemark, R., Brånemark, P. I., Rydevik, B., and Myers, R. R. (2001). Osseointegration in skeletal reconstruction and rehabilitation: a review. J. Rehabil. Res. Dev. 38, 175–181.
Brånemark, R. P., Hagberg, K., Kulbacka-Ortiz, K., Berlin, Ö., and Rydevik, B. (2019). Osseointegrated percutaneous prosthetic system for the treatment of patients with transfemoral amputation: a prospective five-year follow-up of patient-reported outcomes and complications. J. Am. Acad. Orthop. Surg. 27, E743–E751. doi: 10.5435/JAAOS-D-17-00621
Bramati, I. E., Rodrigues, E. C., Simões, E. L., Melo, B., Höfle, S., Moll, J., et al. (2019). Lower limb amputees undergo long-distance plasticity in sensorimotor functional connectivity. Sci. Rep. 9, 1–10. doi: 10.1038/s41598-019-39696-z
Brown, B. J., Iorio, M. L., Klement, M., Conti Mica, M. R., El-Amraoui, A., O'Halloran, P., et al. (2014). Outcomes after 294 transtibial amputations with the posterior myocutaneous flap. Int. J. Low. Extrem. Wounds 13, 33–40. doi: 10.1177/1534734614520706
Cancio, J. M., Ikeda, A. J., Barnicott, S. L., Childers, W. L., Alderete, J. F., and Goff, B. J. (2019). Upper extremity amputation and prosthetics care across the active duty military and veteran populations. Phys. Med. Rehabil. Clin. N. Am. 30, 73–87. doi: 10.1016/j.pmr.2018.08.011
Carey, S., Lura, D., and Highsmith, M. J. (2015). Differences in myoelectric and body-powered upper-limb prostheses: systematic literature review. J. Prosthetics Orthot. 52, 247–262. doi: 10.1097/JPO.0000000000000159
Charkhkar, H., Shell, C. E., Marasco, P. D., Pinault, G. J., Tyler, D. J., and Triolo, R. J. (2018). High-density peripheral nerve cuffs restore natural sensation to individuals with lower-limb amputations. J. Neural Eng. 15:2552. doi: 10.1088/1741-2552/aac964
Cheesborough, J. E., Smith, L. H., Kuiken, T. A., and Dumanian, G. A. (2015). Targeted muscle reinnervation and advanced prosthetic arms. Semin. Plast. Surg. 29, 62–72. doi: 10.1055/s-0035-1544166
Christie, B. P., Freeberg, M., Memberg, W. D., Pinault, G. J. C., Hoyen, H. A., Tyler, D. J., et al. (2017). “Long-term stability of stimulating spiral nerve cuff electrodes on human peripheral nerves.” J. Neuroeng. Rehabil. 14, 1–12. doi: 10.1186/s12984-017-0285-3
Clemente, F., Håkansson, B., Cipriani, C., Wessberg, J., Kulbacka-ortiz, K., Brånemark, R., et al. (2017). Touch and hearing mediate osseoperception. Sci. Rep. 7, 1–11. doi: 10.1038/srep45363
Clites, T. R., Carty, M. J., Srinivasan, S., Zorzos, A. N., and Herr, H. M. (2017). A murine model of a novel surgical architecture for proprioceptive muscle feedback and its potential application to control of advanced limb prostheses. J. Neural Eng. 14:036002. doi: 10.1088/1741-2552/aa614b
Clites, T. R., Carty, M. J., Srinivasan, S. S., Talbot, S. G., Brånemark, R., and Herr, H. M. (2019). Caprine models of the agonist-antagonist myoneural interface implemented at the above- and below-knee amputation levels. Plast. Reconstr. Surg. 144, 218e−229e. doi: 10.1097/PRS.0000000000005864
Clites, T. R., Herr, H. M., Srinivasan, S. S., Zorzos, A. N., and Carty, M. J. (2018). The Ewing amputation: The first human implementation of the agonist-antagonist myoneural interface. Plast. Reconstr. Surg. - Glob. Open 6, 1–8. doi: 10.1097/GOX.0000000000001997
Cogan, S. F. (2008). Neural stimulation and recording electrodes. Annu. Rev. Biomed. Eng. 10, 275–309. doi: 10.1146/annurev.bioeng.10.061807.160518
Cogan, S. F., Ludwig, K. A., Welle, C. G., and Takmakov, P. (2016). Tissue damage thresholds during therapeutic electrical stimulation. J. Neural Eng. 13:021001. doi: 10.1088/1741-2560/13/2/021001.Tissue
Coker, R. A., Zellmer, E. R., and Moran, D. W. (2019). Micro-channel sieve electrode for concurrent bidirectional peripheral nerve interface. Part A: Recording. J. Neural Eng. 16. doi: 10.1088/1741-2552/aaefcf
Cracchiolo, M., Panarese, A., Valle, G., Strauss, I., Granata, G., Iorio, R., et al. (2021). Computational approaches to decode grasping force and velocity level in upper-limb amputee from intraneural peripheral signals. J. Neural Eng. 18, 1–17. doi: 10.1088/1741-2552/abef3a
Cracchiolo, M., Valle, G., Petrini, F., Strauss, I., Granata, G., Stieglitz, T., et al. (2020). Decoding of grasping tasks from intraneural recordings in trans-radial amputee. J. Neural Eng. 17, 1–12. doi: 10.1088/1741-2552/ab8277
Cuberovic, I., Gill, A., Resnik, L. J., Tyler, D. J., and Graczyk, E. L. (2019). Learning of artificial sensation through long-term home use of a sensory-enabled prosthesis. Front. Neurosci. 13, 1–24. doi: 10.3389/fnins.2019.00853
Cutrone, A., Del Valle, J., Santos, D., Badia, J., Filippeschi, C., Micera, S., et al. (2015). A three-dimensional self-opening intraneural peripheral interface (SELINE). J. Neural Eng. 12:016016. doi: 10.1088/1741-2560/12/1/016016
D'Anna, E., Valle, G., Mazzoni, A., Strauss, I., Iberite, F., Patton, J., et al. (2019). A closed-loop hand prosthesis with simultaneous intraneural tactile and position feedback. Sci. Robot. 4:8892. doi: 10.1126/scirobotics.aau8892
Davis, T. S., Wark, H. A. C., Hutchinson, D. T., Warren, D. J., O'Neill, K., Scheinblum, T., et al. (2016). Restoring motor control and sensory feedback in people with upper extremity amputations using arrays of 96 microelectrodes implanted in the median and ulnar nerves. J. Neural Eng. 13:36001. doi: 10.1088/1741-2560/13/3/036001
de la Oliva, N., Navarro, X., and del Valle, J. (2018). Time course study of long-term biocompatibility and foreign body reaction to intraneural polyimide-based implants. J. Biomed. Mater. Res. - Part A 106, 746–757. doi: 10.1002/jbm.a.36274
Deshmukh, A., Brown, L., Barbe, M. F., Braverman, A. S., Tiwari, E., Hobson, L., et al. (2020). Fully implantable neural recording and stimulation interfaces: peripheral nerve interface applications. J. Neurosci. Methods 333:108562. doi: 10.1016/j.jneumeth.2019.108562
Dhillon, G. S., and Horch, K. W. (2005). Direct neural sensory feedback and control of a prosthetic arm. IEEE Trans. Neural Syst. Rehabil. Eng. 13, 468–472. doi: 10.1109/TNSRE.2005.856072
Di Pino, G., Denaro, L., Vadal,à, G., Marinozzi, A., Tombini, M., Ferreri, F., et al. (2014). Invasive neural interfaces: the perspective of the surgeon. J. Surg. Res. 188, 77–87. doi: 10.1016/j.jss.2013.12.014
Dietrich, C., Nehrdich, S., Seifert, S., Blume, K. R., Miltner, W. H. R., Hofmann, G. O., et al. (2018). Leg prosthesis with somatosensory feedback reduces phantom limb pain and increases functionality. Front. Neurol. 9:270. doi: 10.3389/fneur.2018.00270
Diment, L. E., Thompson, M. S., and Bergmann, J. H. M. (2018). Three-dimensional printed upper-limb prostheses lack randomised controlled trials: a systematic review. Prosthet. Orthot. Int. 42, 7–13. doi: 10.1177/0309364617704803
Dingle, A. M., Ness, J. P., Novello, J., Israel, J. S., Sanchez, R., Millevolte, A. X. T., et al. (2020a). Methodology for creating a chronic osseointegrated neural interface for prosthetic control in rabbits. J. Neurosci. Methods 331:108504. doi: 10.1016/j.jneumeth.2019.108504
Dingle, A. M., Ness, J. P., Novello, J., Millevolte, A. X. T., Zeng, W., Sanchez, R., et al. (2020b). Experimental basis for creating an osseointegrated neural interface for prosthetic control: a pilot study in rabbits. Mil. Med. 185, 462–469. doi: 10.1093/milmed/usz246
Dornfeld, C., Swanston, M., Cassella, J., Beasley, C., Green, J., Moshayev, Y., et al. (2016). Is the prosthetic homologue necessary for embodiment? Front. Neurorobot. 10:21. doi: 10.3389/fnbot.2016.00021
Dumanian, G. A., Potter, B. K., Mioton, L. M., Ko, J. H., Cheesborough, J. E., Souza, J. M., et al. (2019). Targeted muscle reinnervation treats neuroma and phantom pain in major limb amputees: a randomized clinical trial. Ann. Surg. 270, 238–246. doi: 10.1097/SLA.0000000000003088
Dweiri, Y. M., Stone, M. A., Tyler, D. J., McCallum, G. A., and Durand, D. M. (2016). Fabrication of high contact-density, flat-interface nerve electrodes for recording and stimulation applications. J. Vis. Exp. 2016, 1–11. doi: 10.3791/54388
Engdahl, S. M., Chestek, C. A., Kelly, B., Davis, A., and Gates, D. H. (2017). Factors associated with interest in novel interfaces for upper limb prosthesis control. PLoS One 12, 1–17. doi: 10.1371/journal.pone.0182482
Engdahl, S. M., Christie, B. P., Kelly, B., Davis, A., Chestek, C. A., and Gates, D. H. (2015). Surveying the interest of individuals with upper limb loss in novel prosthetic control techniques. J. Neuroeng. Rehabil. 12, 1–11. doi: 10.1186/s12984-015-0044-2
Farina, D., Jiang, N., Rehbaum, H., Holobar, A., Graimann, B., Dietl, H., et al. (2014). The extraction of neural information from the surface EMG for the control of upper-limb prostheses: emerging avenues and challenges. IEEE Trans. Neural Syst. Rehabil. Eng. 22, 797–809. doi: 10.1109/TNSRE.2014.2305111
Fisher, L. E., Tyler, D. J., Anderson, J. S., and Triolo, R. J. (2009). Chronic Stability and selectivity of four-contact spiral nerve-cuff electrodes in stimulating the human femoral nerve. J. Neural Eng. 6:371. doi: 10.1088/1741-2560/6/4/046010
Fracol, M. E., Janes, L. E., Ko, J. H., and Dumanian, G. A. (2018). Targeted muscle reinnervation in the lower leg: an anatomical study. Plast. Reconstr. Surg. 142, 541E-550E. doi: 10.1097/PRS.0000000000004773
Freeberg, M. J., Stone, M. A., Triolo, R. J., and Tyler, D. J. (2017). The design of and chronic tissue response to a composite nerve electrode with patterned stiffness. J. Neural Eng. 14:40. doi: 10.1016/j.physbeh.2017.03.040
Gart, M. S., Souza, J. M., and Dumanian, G. A. (2015). Targeted muscle reinnervation in the upper extremity amputee: a technical roadmap. J. Hand Surg. Am. 40, 1877–1888. doi: 10.1016/j.jhsa.2015.06.119
Geethanjali, P. (2016). Myoelectric control of prosthetic hands: state-of-the-art review. Med. Devices Evid. Res. 9, 247–255. doi: 10.2147/MDER.S91102
George, J. A., Page, D. M., Davis, T. S., Duncan, C. C., Hutchinson, D. T., Rieth, L. W., et al. (2020). Long-term performance of Utah Slanted Electrode Arrays and intramuscular electromyographic leads implanted chronically in human arm nerves and muscles. J. Neural Eng. 17, 1–20. doi: 10.1088/1741-2552/abc025
Ghafoor, U., Kim, S., and Hong, K. S. (2017). Selectivity and longevity of peripheral-nerve and machine interfaces: a review. Front. Neurorobot. 11, 1–21. doi: 10.3389/fnbot.2017.00059
Gracyzk, E. L., Schiefer, M. A., Saal, H. P., Delhaye, B. P., Bensmaia, S. J., and Tyler, D. J. (2016). The neural basis of perceived intensity in natural and artificial touch. Sci. Transl. Med. 8:362ra142. doi: 10.1126/scitranslmed.aaf5187
Graczyk, E. L., Resnik, L., Schiefer, M. A., Schmitt, M. S., and Tyler, D. J. (2018). Home use of a neural-connected sensory prosthesis provides the functional and psychosocial experience of having a hand again. Sci. Rep. 8, 1–17. doi: 10.1038/s41598-018-26952-x
Grill, W. M., Norman, S. E., and Bellamkonda, R. V. (2009). Implanted neural interfaces: biochallenges and engineered solutions. Annu. Rev. Biomed. Eng. 11, 1–24. doi: 10.1146/annurev-bioeng-061008-124927
Grüsser, S. M., Mühlnickel, W., Schaefer, M., Villringer, K., Christmann, C., Koeppe, C., et al. (2004). Remote activation of referred phantom sensation and cortical reorganization in human upper extremity amputees. Exp. Brain Res. 154, 97–102. doi: 10.1007/s00221-003-1649-4
Günter, C., Delbeke, J., and Ortiz-Catalan, M. (2019). Safety of long-term electrical peripheral nerve stimulation: review of the state of the art. J. Neuroeng. Rehabil. 16, 1–16. doi: 10.1186/s12984-018-0474-8
Hagberg, K., Ghassemi Jahani, S. A., Kulbacka-Ortiz, K., Thomsen, P., Malchau, H., and Reinholdt, C. (2020). A 15-year follow-up of transfemoral amputees with bone-anchored transcutaneous prostheses : MECHANICAL COMPLICATIONS and PATIENT-REPORTED OUTCOMES. Bone Jt. J. 102 B, 55–63. doi: 10.1302/0301-620X.102B1.BJJ-2019-0611.R1
Häggström, E., Hagberg, K., Rydevik, B., and Brånemark, R. (2013). Vibrotactile evaluation: osseointegrated versus socket-suspended transfemoral prostheses. J. Rehabil. Res. Dev. 50, 1423–1434. doi: 10.1682/JRRD.2012.08.0135
Hahne, J. M., Markovic, M., and Farina, D. (2017). User adaptation in Myoelectric Man-Machine Interfaces. Sci. Rep. 7, 1–10. doi: 10.1038/s41598-017-04255-x
Hahne, J. M., Schweisfurth, M. A., Koppe, M., and Farina, D. (2018). Simultaneous control of multiple functions of bionic hand prostheses: performance and robustness in end users. Sci. Robot. 3:3630. doi: 10.1126/scirobotics.aat3630
Hahne, J. M., Wilke, M. A., Koppe, M., Farina, D., and Schilling, A. F. (2020). Longitudinal case study of regression-based hand prosthesis control in daily life. Front. Neurosci. 14, 1–8. doi: 10.3389/fnins.2020.00600
Hargrove, L., Miller, L., Turner, K., and Kuiken, T. (2018). Control within a virtual environment is correlated to functional outcomes when using a physical prosthesis. J. Neuroeng. Rehabil. 15:60. doi: 10.1186/s12984-018-0402-y
Hargrove, L. J., Miller, L. A., Turner, K., and Kuiken, T. A. (2017). Myoelectric pattern recognition outperforms direct control for transhumeral amputees with targeted muscle reinnervation: a randomized clinical trial. Sci. Rep. 7, 1–9. doi: 10.1038/s41598-017-14386-w
He, J., Zhang, D., Jiang, N., Sheng, X., Farina, D., and Zhu, X. (2015). User adaptation in long-term, open-loop myoelectric training: implications for EMG pattern recognition in prosthesis control. J. Neural Eng. 12:046005. doi: 10.1088/1741-2560/12/4/046005
Hebert, J. S., Chan, K. M., and Dawson, M. R. (2016). Cutaneous sensory outcomes from three transhumeral targeted reinnervation cases. Prosthet. Orthot. Int. 40, 303–310. doi: 10.1177/0309364616633919
Hebert, J. S., Olson, J. L., Morhart, M. J., Dawson, M. R., Marasco, P. D., Kuiken, T. A., et al. (2014). Novel targeted sensory reinnervation technique to restore functional hand sensation after transhumeral amputation. IEEE Trans. Neural Syst. Rehabil. Eng. 22, 765–773. doi: 10.1109/TNSRE.2013.2294907
Herr, H. M., Clites, T. R., Srinivasan, S., Talbot, S. G., Dumanian, G. A., Cederna, P. S., et al. (2020). Reinventing extremity amputation in the era of functional limb restoration. Ann. Surg. 273, 269–279. doi: 10.1097/sla.0000000000003895
Hoellwarth, J. S., Tetsworth, K., Rozbruch, S. R., Handal, M. B., Coughlan, A., and Al Muderis, M. (2020). Osseointegration for Amputees. JBJS Rev. 8:e0043. doi: 10.2106/jbjs.rvw.19.00043
Hong, K. S., Aziz, N., and Ghafoor, U. (2018). Motor-commands decoding using peripheral nerve signals: a review. J. Neural Eng. 15:383. doi: 10.1088/1741-2552/aab383
Horch, K., Meek, S., Taylor, T. G., and Hutchinson, D. T. (2011). Object discrimination with an artificial hand using electrical stimulation of peripheral tactile and proprioceptive pathways with intrafascicular electrodes. IEEE Trans. Neural Syst. Rehabil. Eng. 19, 483–489. doi: 10.1109/TNSRE.2011.2162635
Howell, B., Huynh, B., and Grill, W. M. (2015). Design and in vivo evaluation of more efficient and selective deep brain stimulation electrodes. J. Neural Eng. 12, 1–34. doi: 10.1088/1741-2560/12/4/046030
Hu, Y., Ursu, D. C., Sohasky, R. A., Sando, I. C., Ambani, S. L. W., French, Z. P., et al. (2021). Regenerative peripheral nerve interface free muscle graft mass and function. Muscle Nerve 63, 421–429. doi: 10.1002/mus.27138
Irwin, Z. T., Schroeder, K. E., Vu, P. P., Tat, D. M., Bullard, A. J., Woo, S. L., et al. (2016). Chronic recording of hand prosthesis control signals via a regenerative peripheral nerve interface in a rhesus macaque. J. Neural Eng. 13:6007. doi: 10.1088/1741-2560/13/4/046007
Israel, J. S., Dingle, A. M., Sanchez, R. J., Kapur, S. K., Brodnick, S., Richner, T. J., et al. (2018). Neuroma implantation into long bones: clinical foundation for a novel osseointegrated peripheral nerve interface. Plast. Reconstr. Surg. - Glob. Open 6, 1–4. doi: 10.1097/GOX.0000000000001788
Jacobs, R., Brånemark, R., Olmarker, K., Rydevik, B., Van Steenberghe, D., and Brånemark, P. I. (2000). Evaluation of the psychophysical detection threshold level for vibrotactile and pressure stimulation of prosthetic limbs using bone anchorage or soft tissue support. Prosthet. Orthot. Int. 24, 133–142. doi: 10.1080/03093640008726536
Jiang, N., Englehart, K. B., and Parker, P. A. (2009). Extracting simultaneous and proportional neural control information for multiple-dof prostheses from the surface electromyographic signal. IEEE Trans. Biomed. Eng. 56, 1070–1080. doi: 10.1109/TBME.2008.2007967
Johansson, R. S., and Flanagan, J. R. (2009). Coding and use of tactile signals from the fingertips in object manipulation tasks. Nat. Rev. Neurosci. 10, 345–359. doi: 10.1038/nrn2621
Jönsson, S., Caine-Winterberger, K., and Brånemark, R. (2011). Osseointegration amputation prostheses on the upper limbs: methods, prosthetics and rehabilitation. Prosthet. Orthot. Int. 35, 190–200. doi: 10.1177/0309364611409003
Jung, R., Abbas, J. J., Kuntaegowdanahalli, S., and Thota, A. K. (2018). Bionic intrafascicular interfaces for recording and stimulating peripheral nerve fibers. Bioelectron. Med. 1, 55–69. doi: 10.2217/bem-2017-0009
Karl, A., Birbaumer, N., Lutzenberger, W., Cohen, L. G., and Flor, H. (2001). Reorganization of motor and somatosensory cortex in upper extremity amputees with phantom limb pain. J. Neurosci. 21, 3609–3618. doi: 10.1523/jneurosci.21-10-03609.2001
Kerver, N., Twillert, S., Van, M.aas, B., and Van Der Sluis, C. K. (2020). User-relevant factors determining prosthesis choice in persons with major unilateral upper limb defects: a meta-synthesis of qualitative literature and focus group results. PLoS ONE 15, 1–25. doi: 10.1371/journal.pone.0234342
Kilgore, K. L., Hoyen, H. A., Bryden, A. M., Hart, R. L., Keith, M. W., and Peckham, P. H. (2008). An implanted upper-extremity neuroprosthesis using myoelectric control. J. Hand Surg. Am. 33, 539–550. doi: 10.1016/j.jhsa.2008.01.007
Kim, K., and Colgate, J. E. (2012). Haptic feedback enhances grip force control of sEMG-controlled prosthetic hands in targeted reinnervation amputees. IEEE Trans. Neural Syst. Rehabil. Eng. 20, 798–805. doi: 10.1109/TNSRE.2012.2206080
Klineberg, I. (2005). Introduction: from osseointegration to osseoperception. The functional translation. Clin. Exp. Pharmacol. Physiol. 32, 97–99. doi: 10.1111/j.1440-1681.2005.04135.x
Kristoffersen, M. B., Franzke, A. W., Bongers, R. M., Wand, M., Murgia, A., and van der Sluis, C. K. (2021). User training for machine learning controlled upper limb prostheses: a serious game approach. J. Neuroeng. Rehabil. 18, 1–15. doi: 10.1186/s12984-021-00831-5
Kubiak, C. A., Kemp, S. W. P., and Cederna, P. S. (2018). Regenerative peripheral nerve interface for management of postamputation neuroma. JAMA Surg. 153, 681–682. doi: 10.1001/jamasurg.2018.0864
Kubiak, C. A., Kemp, S. W. P., Cederna, P. S., and Kung, T. A. (2019). Prophylactic regenerative peripheral nerve interfaces to prevent postamputation pain. Plast. Reconstr. Surg. 144, 421e-430e. doi: 10.1097/PRS.0000000000005922
Kuiken, T. A., Dumanian, G. A., Lipschutz, R. D., Miller, L. A., and Stubblefield, K. A. (2004). The use of targeted muscle reinnervation for improved myoelectric prosthesis control in a bilateral shoulder disarticulation amputee. Prosthet. Orthot. Int. 28, 245–253. doi: 10.3109/03093640409167756
Kuiken, T. A., Li, G., Lock, B. A., Lipschutz, R. D., Miller, L. A., Stubblefield, K. A., et al. (2009). Targeted muscle reinnervation for real-time myoelectric control of multifunction artificial arms. JAMA - J. Am. Med. Assoc. 301, 619–628. doi: 10.1001/jama.2009.116
Kuiken, T. A., Marasco, P. D., Lock, B. A., Harden, R. N., and Dewald, J. P. A. (2007). Redirection of cutaneous sensation from the hand to the chest skin of human amputees with targeted reinnervation. Proc. Natl. Acad. Sci. U.S.A. 104, 20061–20066. doi: 10.1073/pnas.0706525104
Kuiken, T. A., Miller, L. A., Turner, K., and Hargrove, L. J. (2016). A comparison of pattern recognition control and direct control of a multiple degree-of-freedom transradial prosthesis. IEEE J. Transl. Eng. Heal. Med. 4:123. doi: 10.1109/JTEHM.2016.2616123
Kundu, A., Harreby, K. R., Yoshida, K., Boretius, T., Stieglitz, T., and Jensen, W. (2014). Stimulation selectivity of the “thin-film longitudinal intrafascicular electrode” (tfLIFE) and the “transverse intrafascicular multi-channel electrode” (time) in the large nerve animal model. IEEE Trans. Neural Syst. Rehabil. Eng. 22, 400–410. doi: 10.1109/TNSRE.2013.2267936
Kung, T. A., Bueno, R. A., Alkhalefah, G. K., Langhals, N. B., Urbanchek, M. G., and Cederna, P. S. (2013). Innovations in prosthetic interfaces for the upper extremity. Plast. Reconstr. Surg. 132, 1515–1523. doi: 10.1097/PRS.0b013e3182a97e5f
Kung, T. A., Langhals, N. B., Martin, D. C., Johnson, P. J., Cederna, P. S., and Urbanchek, M. G. (2014). Regenerative peripheral nerve interface viability and signal transduction with an implanted electrode. Plast. Reconstr. Surg. 133, 1380–1394. doi: 10.1097/PRS.0000000000000168
Lago, N., Ceballos, D, J., Rodríguez, F., Stieglitz, T., and Navarro, X. (2005). Long term assessment of axonal regeneration through polyimide regenerative electrodes to interface the peripheral nerve. Biomaterials 26, 2021–2031. doi: 10.1016/j.biomaterials.2004.06.025
Lago, N., Yoshida, K., Koch, K. P., and Navarro, X. (2007). Assessment of biocompatibility of chronically implanted polyimide and platinum intrafascicular electrodes. IEEE Trans. Biomed. Eng. 54, 281–290. doi: 10.1109/TBME.2006.886617
Larson, C. E., and Meng, E. (2020). A review for the peripheral nerve interface designer. J. Neurosci. Methods 332:108523. doi: 10.1016/j.jneumeth.2019.108523
Lawrence, S. M., Dhillon, G. S., Jensen, W., Yoshida, K., and Horch, K. W. (2004). Acute peripheral nerve recording characteristics of polymer-based longitudinal intrafascicular electrodes. IEEE Trans. Neural Syst. Rehabil. Eng. 12, 345–348. doi: 10.1109/TNSRE.2004.831491
Lee, S., Sheshadri, S., Xiang, Z., Delgado-Martinez, I., Xue, N., Sun, T., et al. (2017). Selective stimulation and neural recording on peripheral nerves using flexible split ring electrodes. Sensors Actuators, B Chem. 242, 1165–1170. doi: 10.1016/j.snb.2016.09.127
Leventhal, D. K., and Durand, D. M. (2003). Subfascicle stimulation selectivity with the flat interface nerve electrode. Ann. Biomed. Eng. 31, 643–652. doi: 10.1114/1.1569266
Li, G., Schultz, A. E., and Kuiken, T. A. (2010). Quantifying pattern recognition- based myoelectric control of multifunctional transradial prostheses. IEEE Trans. Neural Syst. Rehabil. Eng. 18, 185–192. doi: 10.1109/TNSRE.2009.2039619
Loeb, G. E., and Peck, R. A. (1996). Cuff electrodes for chronic stimulation and recording of peripheral nerve activity. J. Neurosci. Methods 64, 95–103. doi: 10.1016/0165-0270(95)00123-9
Lowery, M. M., Weir, R. F. F., and Kuiken, T. A. (2006). Simulation of intramuscular EMG signals detected using implantable myoelectric sensors (IMES). IEEE Trans. Biomed. Eng. 53, 1926–1933. doi: 10.1109/TBME.2006.881774
MacEwan, M. R., Zellmer, E. R., Wheeler, J. J., Burton, H., and Moran, D. W. (2016). Regenerated sciatic nerve axons stimulated through a chronically implanted macro-sieve electrode. Front. Neurosci. 10, 1–12. doi: 10.3389/fnins.2016.00557
MacIver, K., Lloyd, D. M., Kelly, S., Roberts, N., and Nurmikko, T. (2008). Phantom limb pain, cortical reorganization and the therapeutic effect of mental imagery. Brain 131, 2181–2191. doi: 10.1093/brain/awn124
Markatos, K., Karamanou, M., Saranteas, T., and Mavrogenis, A. F. (2019). Hallmarks of amputation surgery. Int. Orthop. 43, 493–499. doi: 10.1007/s00264-018-4024-6
Markovic, M., Schweisfurth, M. A., Engels, L. F., Farina, D., and Dosen, S. (2018). Myocontrol is closed-loop control: incidental feedback is sufficient for scaling the prosthesis force in routine grasping. J. Neuroeng. Rehabil. 15, 1–11. doi: 10.1186/s12984-018-0422-7
Mastinu, E., Clemente, F., Sassu, P., Aszmann, O., Brånemark, R., Håkansson, B., et al. (2019). Grip control and motor coordination with implanted and surface electrodes while grasping with an osseointegrated prosthetic hand. J. Neuroeng. Rehabil. 16, 1–10. doi: 10.1186/s12984-019-0511-2
Mayer, R. M., Garcia-Rosas, R., Mohammadi, A., Tan, Y., Alici, G., Choong, P., et al. (2020). Tactile feedback in closed-loop control of myoelectric hand grasping: conveying information of multiple sensors simultaneously via a single feedback channel. Front. Neurosci. 14, 1–12. doi: 10.3389/fnins.2020.00348
Merrill, D. R., Bikson, M., and Jefferys, J. G. R. (2005). Electrical stimulation of excitable tissue: design of efficacious and safe protocols. J. Neurosci. Methods 141, 171–198. doi: 10.1016/j.jneumeth.2004.10.020
Merrill, D. R., Lockhart, J., Troyk, P. R., Weir, R. F., and Hankin, D. L. (2011). Development of an implantable myoelectric sensor for advanced prosthesis control. Artif. Organs 35, 249–252. doi: 10.1111/j.1525-1594.2011.01219.x
Micera, S., Carpaneto, J., and Raspopovic, S. (2010). Control of hand prostheses using peripheral information. IEEE Rev. Biomed. Eng. 3, 48–68. doi: 10.1109/RBME.2010.2085429
Micera, S., and Navarro, X. (2009). Chapter 2 Bidirectional Interfaces With the Peripheral Nervous System. Int. Rev. Neurobiol. 86, 23–38. doi: 10.1016/S0074-7742(09)86002-9
Micera, S., Navarro, X., Carpaneto, J., Citi, L., Tonet, O., Rossini, P. M., et al. (2008). On the use of longitudinal intrafascicular peripheral interfaces for the control of cybernetic hand prostheses in amputees. IEEE Trans. Neural Syst. Rehabil. Eng. 16, 453–472. doi: 10.1109/TNSRE.2008.2006207
Micera, S., Rossini, P. M., Rigosa, J., Citi, L., Carpaneto, J., Raspopovic, S., et al. (2011). Decoding of grasping information from neural signals recorded using peripheral intrafascicular interfaces. J. Neuroeng. Rehabil. 8:53. doi: 10.1186/1743-0003-8-53
Middleton, A., and Ortiz-Catalan, M. (2020). Neuromusculoskeletal arm prostheses: personal and social implications of living with an intimately integrated bionic Arm. Front. Neurorobot. 14, 1–18. doi: 10.3389/fnbot.2020.00039
Miller, L. A., Stubblefield, K. A., Lipschutz, R. D., Lock, B. A., and Kuiken, T. A. (2008). Improved myoelectric prosthesis control using targeted reinnervation surgery: a case series. IEEE Trans. Neural Syst. Rehabil. Eng. 16, 46–50. doi: 10.1109/TNSRE.2007.911817
Millevolte, A. X. T., Dingle, A. M., Ness, J. P., Novello, J., Zeng, W., Lu, Y., et al. (2021). Improving the selectivity of an osseointegrated neural interface: proof of concept for housing sieve electrode arrays in the medullary canal of long bones. Front. Neurosci. 15, 1–9. doi: 10.3389/fnins.2021.613844
Mioton, L. M., and Dumanian, G. A. (2018). Targeted muscle reinnervation and prosthetic rehabilitation after limb loss. J. Surg. Oncol. 118, 807–814. doi: 10.1002/jso.25256
Mohan Bhatnagar, V., Karani, J. T., Khanna, A., Badwaik, P., and Pai, A. (2015). Osseoperception: an implant mediated sensory motor control- A review. J. Clin. Diagnostic Res. 9, ZE18–ZE20. doi: 10.7860/JCDR/2015/14349.6532
Naufel, S., Knaack, G. L., Miranda, R., Best, T. K., Fitzpatrick, K., Emondi, A. A., et al. (2020). DARPA investment in peripheral nerve interfaces for prosthetics, prescriptions, and plasticity. J. Neurosci. Methods 332:108539. doi: 10.1016/j.jneumeth.2019.108539
Navarro, X., Krueger, T. B., Lago, N., Micera, S., Stieglitz, T., and Dario, P. (2005). A critical review of interfaces with the peripheral nervous system for the control of neuroprostheses and hybrid bionic systems. J. Peripher. Nerv. Syst. 10, 229–258. doi: 10.1111/j.1085-9489.2005.10303.x
Nilsson, N., Håkansson, B., and Ortiz-Catalan, M. (2017). Classification complexity in myoelectric pattern recognition. J. Neuroeng. Rehabil. 14, 1–18. doi: 10.1186/s12984-017-0283-5
O'Brien, A. L., Jordan, S. W., West, J. M., Mioton, L. M., Dumanian, G. A., and Valerio, I. L. (2021). Targeted muscle reinnervation at the time of upper-extremity amputation for the treatment of pain severity and symptoms. J. Hand Surg. Am. 46, 72.e1-72.e10. doi: 10.1016/j.jhsa.2020.08.014
Oddo, C. M., Raspopovic, S., Artoni, F., Mazzoni, A., Spigler, G., Petrini, F., et al. (2016). Intraneural stimulation elicits discrimination of textural features by artificial fingertip in intact and amputee humans. Elife 5, 1–27. doi: 10.7554/eLife.09148
Oh, C., and Carlsen, B. T. (2019). New innovations in targeted muscle reinnervation: a critical analysis review. JBJS Rev. 7, 1–8. doi: 10.2106/JBJS.RVW.18.00138
Ortiz-Catalan, M., Håkansson, B., and Brånemark, R. (2014). Real-time and simultaneous control of artificial limbs based on pattern recognition algorithms. IEEE Trans. Neural Syst. Rehabil. Eng. 22, 756–764. doi: 10.1109/TNSRE.2014.2305097
Ortiz-Catalan, M., Mastinu, E., Greenspon, C. M., and Bensmaia, S. J. (2020). Chronic use of a sensitized bionic hand does not remap the sense of touch. Cell Rep. 33:8539. doi: 10.1016/j.celrep.2020.108539
OrtizCatalan, M., Mastinu, E., Sassu, P., Aszmann, O., and Brånemark, R. (2020). Self-contained neuromusculoskeletal arm prostheses. N. Engl. J. Med. 382, 1732–1738. doi: 10.1056/NEJMoa1917537
Overmann, A. L., Aparicio, C., Richards, J. T., Mutreja, I., Fischer, N. G., Wade, S. M., et al. (2020). Orthopaedic osseointegration: implantology and future directions. J. Orthop. Res. 38, 1445–1454. doi: 10.1002/jor.24576
Page, D. M., George, J. A., Kluger, D. T., Duncan, C., Wendelken, S., Davis, T., et al. (2018). Motor control and sensory feedback enhance prosthesis embodiment and reduce phantom pain after long-term hand amputation. Front. Hum. Neurosci. 12, 1–16. doi: 10.3389/fnhum.2018.00352
Pasquina, P. F., Evangelista, M., Carvalho, A. J., Lockhart, J., Griffin, S., Nanos, G., et al. (2015). First-in-man demonstration of fully implanted myoelectric sensors for control of an advanced electromechanical arm by transradial amputees. J. Neurosci. Methods 244, 85–93. doi: 10.1016/j.jneumeth.2014.07.016.First-in-Man
Peters, B. R., Russo, S. A., West, J. M., Moore, A. M., and Schulz, S. A. (2020). Targeted muscle reinnervation for the management of pain in the setting of major limb amputation. SAGE Open Med. 8:205031212095918. doi: 10.1177/2050312120959180
Petrini, F. M., Bumbasirevic, M., Valle, G., Ilic, V., Mijovi,ć, P., Cvančara, P., et al. (2019a). Sensory feedback restoration in leg amputees improves walking speed, metabolic cost and phantom pain. Nat. Med. 25, 1356–1363. doi: 10.1038/s41591-019-0567-3
Petrini, F. M., Valle, G., Strauss, I., Granata, G., Di Iorio, R., D'Anna, E., et al. (2019b). Six-month assessment of a hand prosthesis with intraneural tactile feedback. Ann. Neurol. 85, 137–154. doi: 10.1002/ana.25384
Pierrie, S. N., Gaston, R. G., and Loeffler, B. J. (2018). Current concepts in upper-extremity amputations. J. Hand Surg. Am. 43, 657–667. doi: 10.1016/j.jhsa.2018.03.053
Polasek, K. H., Hoyen, H. A., Michael, K. W., Kirsch, R. F., and Tyler, D. J. (2009). Stimulation stability and selectivity of chronically implanted multicontact nerve cuff electrodes in the human upper extremity. IEEE Trans. Neural Syst. Rehabil. Eng. 17, 428–437. doi: 10.1109/TNSRE.2009.2032603
Poppler, L. H., Parikh, R. P., Bichanich, M. J., Rebehn, K., Bettlach, C. R., Mackinnon, S. E., et al. (2018). Surgical interventions for the treatment of painful neuroma: a comparative meta-analysis. Pain 159, 214–223. doi: 10.1097/j.pain.0000000000001101.Surgical
Pospiech, P. T., Wendlandt, R., Aschoff, H. H., Ziegert, S., and Schulz, A. P. (2020). Quality of life of persons with transfemoral amputation: comparison of socket prostheses and osseointegrated prostheses. Prosthet. Orthot. Int. 45, 20–25. doi: 10.1177/0309364620948649
Prahm, C., Vujaklija, I., Kayali, F., Purgathofer, P., and Aszmann, O. C. (2017). Game-based rehabilitation for myoelectric prosthesis control. JMIR Serious Games 5:6026. doi: 10.2196/games.6026
Preißler, S., Thielemann, D., Dietrich, C., Hofmann, G. O., Miltner, W. H. R., and Weiss, T. (2017). Preliminary evidence for training-induced changes of morphology and phantom limb pain. Front. Hum. Neurosci. 11, 1–12. doi: 10.3389/fnhum.2017.00319
Proske, U., and Gandevia, S. C. (2012). The proprioceptive senses: their roles in signaling body shape, body position and movement, and muscle force. Physiol. Rev. 92, 1651–1697. doi: 10.1152/physrev.00048.2011
Raspopovic, S., Capogrosso, M., Petrini, F. M., Bonizzato, M., Rigosa, J., Pino, G., et al. (2014). Bioengineering: restoring natural sensory feedback in real-time bidirectional hand prostheses. Sci. Transl. Med. 6, 1–12. doi: 10.1126/scitranslmed.3006820
Raspopovic, S., Cimolato, A., Panarese, A., Vallone, F., del Valle, J., Micera, S., et al. (2020). Neural signal recording and processing in somatic neuroprosthetic applications. A review. J. Neurosci. Methods 337:108653. doi: 10.1016/j.jneumeth.2020.108653
Raspopovic, S., Valle, G., and Petrini, F. M. (2021). Sensory feedback for limb prostheses in amputees. Nat. Mater. 20, 925–939. doi: 10.1038/s41563-021-00966-9
Resnik, L., Borgia, M., Heinemann, A. W., and Clark, M. A. (2020). Prosthesis satisfaction in a national sample of Veterans with upper limb amputation. Prosthet. Orthot. Int. 44, 81–91. doi: 10.1177/0309364619895201
Resnik, L., Borgia, M., Silver, B., and Cancio, J. (2017). Systematic review of measures of impairment and activity limitation for persons with upper limb trauma and amputation. Arch. Phys. Med. Rehabil. 98, 1863–1892.e14. doi: 10.1016/j.apmr.2017.01.015
Resnik, L., Etter, K., Klinger, S. L., and Kambe, C. (2011). Using virtual reality environment to facilitate training with advanced upper-limb prosthesis. J. Rehabil. Res. Dev. 48, 707–718. doi: 10.1682/JRRD.2010.07.0127
Resnik, L., Klinger, S., Gill, A., and Ekerholm Biester, S. (2019). Feminine identity and functional benefits are key factors in women's decision making about upper limb prostheses: a case series. Disabil. Rehabil. Assist. Technol. 14, 194–208. doi: 10.1080/17483107.2018.1467973
Riemann, B. L., and Lephart, S. M. (2002). The sensorimotor system, Part II: The role of proprioception in motor control and functional joint stability. J. Athl. Train. 37, 80–84. doi: 10.1016/j.jconhyd.2010.08.009
Rijnbeek, E. H., Eleveld, N., and Olthuis, W. (2018). Update on peripheral nerve electrodes for closed-loop neuroprosthetics. Front. Neurosci. 12, 1–9. doi: 10.3389/fnins.2018.00350
Rossini, P. M., Micera, S., Benvenuto, A., Carpaneto, J., Cavallo, G., Citi, L., et al. (2010). Double nerve intraneural interface implant on a human amputee for robotic hand control. Clin. Neurophysiol. 121, 777–783. doi: 10.1016/j.clinph.2010.01.001
Russell, C., Roche, A. D., and Chakrabarty, S. (2019). Peripheral nerve bionic interface: a review of electrodes. Int. J. Intell. Robot. Appl. 3, 11–18. doi: 10.1007/s41315-019-00086-3
Salminger, S., Sturma, A., Hofer, C., Evangelista, M., Perrin, M., Bergmeister, K. D., et al. (2019). Long-term implant of intramuscular sensors and nerve transfers for wireless control of robotic arms in above-elbow amputees. Sci. Robot. 4:6306. doi: 10.1126/scirobotics.aaw6306
Samuel, O. W., Asogbon, M. G., Geng, Y., Al-Timemy, A. H., Pirbhulal, S., Ji, N., et al. (2019). Intelligent EMG pattern recognition control method for upper-limb multifunctional prostheses: advances, current challenges, and future prospects. IEEE Access 7, 10150–10165. doi: 10.1109/ACCESS.2019.2891350
Sanders, J. E., and Fatone, S. (2011). Residual Limb Volume Change: Systematic Review of Measurement and Management. J. Rehabil. Res. Dev. 48, 949–986. doi: 10.1682/JRRD.2010.09.0189
Santosa, K. B., Oliver, J. D., Cederna, P. S., and Kung, T. A. (2020). Regenerative peripheral nerve interfaces for prevention and management of neuromas. Clin. Plast. Surg. 47, 311–321. doi: 10.1016/j.cps.2020.01.004
Schiefer, M., Tan, D., Sidek, S. M., and Tyler, D. J. (2016). Sensory feedback by peripheral nerve stimulation improves task performance in individuals with upper limb loss using a myoelectric prosthesis. J. Neural Eng. 13, 1–25. doi: 10.1088/1741-2560/13/1/016001.Sensory
Schiefer, M. A., Graczyk, E. L., Sidik, S. M., Tan, D. W., and Tyler, D. J. (2018). Artificial tactile and proprioceptive feedback improves performance and confidence on object identification tasks. PLoS ONE 13, 1–18. doi: 10.1371/journal.pone.0207659
Schofield, J. S., Shell, C. E., Beckler, D. T., Thumser, Z. C., and Marasco, P. D. (2020). Long-term home-use of sensory-motor-integrated bidirectional bionic prosthetic arms promotes functional, perceptual, and cognitive changes. Front. Neurosci. 14, 1–20. doi: 10.3389/fnins.2020.00120
Sehirlioglu, A., Ozturk, C., Yazicioglu, K., Tugcu, I., Yilmaz, B., and Goktepe, A. S. (2009). Painful neuroma requiring surgical excision after lower limb amputation caused by landmine explosions. Int. Orthop. 33, 533–536. doi: 10.1007/s00264-007-0466-y
Sensinger, J. W., and Dosen, S. (2020). A review of sensory feedback in upper-limb prostheses from the perspective of human motor control. Front. Neurosci. 14, 1–24. doi: 10.3389/fnins.2020.00345
Seo, D., Neely, R. M., Shen, K., Singhal, U., Alon, E., Rabaey, J. M., et al. (2016). Wireless recording in the peripheral nervous system with ultrasonic neural dust. Neuron 91, 529–539. doi: 10.1016/j.neuron.2016.06.034
Serino, A., Akselrod, M., Salomon, R., Martuzzi, R., Blefari, M. L., Canzoneri, E., et al. (2017). Upper limb cortical maps in amputees with targeted muscle and sensory reinnervation. Brain 140, 2993–3011. doi: 10.1093/brain/awx242
Sinha, R., Van Den Heuvel, W. J. A., and Arokiasamy, P. (2011). Factors affecting quality of life in lower limb amputees. Prosthet. Orthot. Int. 35, 90–96. doi: 10.1177/0309364610397087
Smith, L. H., Kuiken, T. A., and Hargrove, L. J. (2015). Real-time simultaneous and proportional myoelectric control using intramuscular EMG. 11, 1–27. doi: 10.1088/1741-2560/11/6/066013.Real-time
Souza, J. M., Cheesborough, J. E., Ko, J. H., Cho, M. S., Kuiken, T. A., and Dumanian, G. A. (2014). Targeted muscle reinnervation: a novel approach to postamputation neuroma pain. Clin. Orthop. Relat. Res. 472, 2984–2990. doi: 10.1007/s11999-014-3528-7
Souza, J. M., Mioton, L. M., Harrington, C. J., Potter, B. K., and Forsberg, J. A. (2020). Osseointegration of extremity prostheses: a primer for the plastic surgeon. Plast. Reconstr. Surg., 1394–1403. doi: 10.1097/PRS.0000000000007364
Srinivasan, S. S., Diaz, M., Carty, M., and Herr, H. M. (2019). Towards functional restoration for persons with limb amputation: a dual-stage implementation of regenerative agonist-antagonist myoneural interfaces. Sci. Rep. 9, 1–10. doi: 10.1038/s41598-018-38096-z
Srinivasan, S. S., Tuckute, G., Zou, J., Gutierrez-Arango, S., Song, H., Barry, R. L., et al. (2020). Agonist-antagonist myoneural interface amputation preserves proprioceptive sensorimotor neurophysiology in lower limbs. Sci. Transl. Med. 12:5926. doi: 10.1126/scitranslmed.abc5926
Stokvis, A., Van Der Avoort, D. J. J. C., Van Neck, J. W., Hovius, S. E. R., and Coert, J. H. (2010). Surgical management of neuroma pain: a prospective follow-up study. Pain 151, 862–869. doi: 10.1016/j.pain.2010.09.032
Tabor, A., Bateman, S., and Scheme, E. (2018). Evaluation of myoelectric control learning using multi-session game-based training. IEEE Trans. Neural Syst. Rehabil. Eng. 26, 1680–1689. doi: 10.1109/TNSRE.2018.2855561
Tam, W., Wu, T., Zhao, Q., Keefer, E., and Yang, Z. (2019). Human motor decoding from neural signals: a review. BMC Biomed. Eng. 1, 1–22. doi: 10.1186/s42490-019-0022-z
Tan, D. W., Schiefer, M. A., Keith, M. W., Anderson, R. J., and Tyler, D. J. (2015). U. S. Department of Veterans Affairs for sensory stimulation in human amputees. J. Neural Eng. 12, 1–19. doi: 10.1088/1741-2560/12/2/026002.Stability
Tan, D. W., Schiefer, M. A., Keith, M. W., Robert, J., Tyler, J., and Tyler, D. J. (2014). U. S. Department of Veterans Affairs perception. Sci. Transl. Med. 6, 1–25. doi: 10.1126/scitranslmed.3008669.A
Tang, Y., and Durand, D. M. (2014). Bayesian spatial filters for the extraction of source signals, a study in the peripheral nerve. IEEE Trans. Neural Syst. Rehabil. Eng. 22, 302–311. doi: 10.1109/TNSRE.2014.2303472.Bayesian
Tillander, J., Hagberg, K., Berlin, Ö., Hagberg, L., and Brånemark, R. (2017). Osteomyelitis risk in patients with transfemoral amputations treated with osseointegration prostheses. Clin. Orthop. Relat. Res. 475, 3100–3108. doi: 10.1007/s11999-017-5507-2
Tyler, D. J. (2015). Neural interfaces for somatosensory feedback: bringing life to a prosthesis. Curr. Opin. Neurol. 28, 574–581. doi: 10.1097/WCO.0000000000000266
Tyler, D. J., and Durand, D. M. (2002). Functionally selective peripheral nerve stimulation with a flat interface nerve electrode. IEEE Trans. Neural Syst. Rehabil. Eng. 10, 294–303. doi: 10.1109/TNSRE.2002.806840
Tyler, D. J., Polasek, K. H., and Schiefer, M. A. (2015). “Peripheral nerve interfaces,” in Nerves and Nerve Injuries, 1033–1054. doi: 10.1016/B978-0-12-802653-3.00112-3
Urbanchek, M. G., Baghmanli, Z., Moon, J. D., Sugg, K. B., Langhals, N. B., and Cederna, P. S. (2012). Quantification of regenerative peripheral nerve interface signal transmission. Biomed Res. Int. 130, 55–56. doi: 10.1109/EMBC.2014.6944595
Urbanchek, M. G., Kung, T. A., Frost, C. M., Martin, D. C., Larkin, L. M., Wollstein, A., et al. (2016). Development of a regenerative peripheral nerve interface for control of a neuroprosthetic limb. Biomed Res. Int. 2016:730. doi: 10.1155/2016/5726730
Ursu, D. C., Urbanchek, M. G., Nedic, A., Cederna, P. S., and Gillespie, R. B. (2016). In vivo characterization of regenerative peripheral nerve interface function. J. Neural Eng. 13. doi: 10.1088/1741-2560/13/2/026012
Valerio, I., Schulz, S. A., West, J., Westenberg, R. F., and Eberlin, K. R. (2020). Targeted muscle reinnervation combined with a vascularized pedicled regenerative peripheral nerve interface. Plast. Reconstr. Surg. - Glob. Open 8:e2689. doi: 10.1097/gox.0000000000002689
Valle, G., Mazzoni, A., Iberite, F., D'Anna, E., Strauss, I., Granata, G., et al. (2018). Biomimetic intraneural sensory feedback enhances sensation naturalness, tactile sensitivity, and manual dexterity in a bidirectional prosthesis. Neuron 100, 37–45.e7. doi: 10.1016/j.neuron.2018.08.033
Valle, G., Saliji, A., Fogle, E., Cimolato, A., Petrini, F. M., and Raspopovic, S. (2021). Mechanisms of neuro-robotic prosthesis operation in leg amputees. Sci. Adv. 7:8354. doi: 10.1126/sciadv.abd8354
Vela, F., Martínez-Chacón, G., Ballestín, A., Campos, J., Sánchez-Margallo, F., and Abellán, E. (2020). Animal models used to study direct peripheral nerve repair: a systematic review. Neural Regen. Res. 15, 491–502. doi: 10.4103/1673-5374.266068
Vincitorio, F., Staffa, G., Aszmann, O. C., Fontana, M., Brånemark, R., Randi, P., et al. (2020). Targeted muscle reinnervation and osseointegration for pain relief and prosthetic arm control in a woman with bilateral proximal upper limb amputation. World Neurosurg. 143, 365–373. doi: 10.1016/j.wneu.2020.08.047
Vu, P. P., Chestek, C. A., Nason, S. R., Kung, T. A., Kemp, S. W. P., and Cederna, P. S. (2020a). The future of upper extremity rehabilitation robotics: research and practice. Muscle Nerve 61, 708–718. doi: 10.1002/mus.26860
Vu, P. P., Vaskov, A. K., Irwin, Z. T., Henning, P. T., Lueders, D. R., Laidlaw, A. T., et al. (2020b). A regenerative peripheral nerve interface allows real-time control of an artificial hand in upper limb amputees. Sci. Transl. Med. 12, 1–12. doi: 10.1126/scitranslmed.aay2857
Weber, D. J., Friesen, R., and Miller, L. E. (2012). Interfacing the somatosensory system to restore touch and Proprioception: essential considerations. J. Mot. Behav. 44, 403–418. doi: 10.1080/00222895.2012.735283
Weir, R. F., Troyk, P. R., DeMichele, G. A., Kerns, D. A., Schorsch, J. F., and Maas, H. (2009). Implantable myoelectric sensors (IMESs) for intramuscular electromyogram recording. IEEE Trans. Biomed. Eng. 56, 159–171. doi: 10.1109/TBME.2008.2005942
Wellman, S. M., Eles, J. R., Ludwig, K. A., Seymour, J. P., Michelson, N. J., McFadden, W. E., et al. (2018). A materials roadmap to functional neural interface design. Adv Funct Mater 28, 1–77. doi: 10.1002/adfm.201701269.A
Wendelken, S., Page, D. M., Davis, T., Wark, H. A. C., Kluger, D. T., Duncan, C., et al. (2017). Restoration of motor control and proprioceptive and cutaneous sensation in humans with prior upper-limb amputation via multiple Utah Slanted Electrode Arrays (USEAs) implanted in residual peripheral arm nerves. J. Neuroeng. Rehabil. 14, 1–17. doi: 10.1186/s12984-017-0320-4
Winslow, B. D., Ruble, M., and Huber, Z. (2018). Mobile, game-based training for myoelectric prosthesis control. Front. Bioeng. Biotechnol. 6, 1–8. doi: 10.3389/fbioe.2018.00094
Witteveen, H. J. B., Droog, E. A., Rietman, J. S., and Veltink, P. H. (2012). Vibro- and electrotactile user feedback on hand opening for myoelectric forearm prostheses. IEEE Trans. Biomed. Eng. 59, 2219–2226. doi: 10.1109/TBME.2012.2200678
Wodlinger, B., and Durand, D. (2011). Selective recovery of fascicular peripheral nerves. J. Neural Eng. 8, 1–13. doi: 10.1088/1741-2560/8/5/056005
Woeppel, K., Yang, Q., and Cui, X. T. (2017). Recent advances in neural electrode-tissue interfaces. Curr. Opin. Biomed. Eng. 176, 21–31. doi: 10.1016/j.cobme.2017.09.003.Recent
Wolf, E. J., Cruz, T. H., Emondi, A. A., Langhals, N. B., Naufel, S., Peng, G. C. Y., et al. (2020). Advanced technologies for intuitive control and sensation of prosthetics. Biomed. Eng. Lett. 10, 119–128. doi: 10.1007/s13534-019-00127-7
Woo, S. L., Kung, T. A., Brown, D. L., Leonard, J. A., Kelly, B. M., and Cederna, P. S. (2016). Regenerative peripheral nerve interfaces for the treatment of postamputation neuroma pain: a pilot study. Plast. Reconstr. Surg. - Glob. Open 4, 1–8. doi: 10.1097/GOX.0000000000001038
Woo, S. L., Urbanchek, M. G., Cederna, P. S., and Langhals, N. B. (2014). Revisiting nonvascularized partial muscle grafts: a novel use for prosthetic control. Plast. Reconstr. Surg. 134, 344–346. doi: 10.1097/PRS.0000000000000317
Woodward, R. B., and Hargrove, L. J. (2019). Adapting myoelectric control in real-time using a virtual environment. J. Neuroeng. Rehabil. 16, 1–12. doi: 10.1186/s12984-019-0480-5
Wurth, S., Capogrosso, M., Raspopovic, S., Gandar, J., Federici, G., Kinany, N., et al. (2017). Long-term usability and bio-integration of polyimide-based intra-neural stimulating electrodes. Biomaterials 122, 114–129. doi: 10.1016/j.biomaterials.2017.01.014
Yildiz, K. A., Shin, A. Y., and Kaufman, K. R. (2020). Interfaces with the peripheral nervous system for the control of a neuroprosthetic limb: a review. J. Neuroeng. Rehabil. 17, 1–19. doi: 10.1186/s12984-020-00667-5
Young, A. J., Hargrove, L. J., and Kuiken, T. A. (2011). The effects of electrode size and orientation on the sensitivity of myoelectric pattern recognition systems to electrode shift. IEEE Trans. Biomed. Eng. 58, 2537–2544. doi: 10.1109/TBME.2011.2159216
Young, A. J., Smith, L. H., Rouse, E. J., and Hargrove, L. J. (2013). Classification of simultaneous movements using surface EMG pattern recognition. IEEE Trans. Biomed. Eng. 60, 1250–1258. doi: 10.1109/TBME.2012.2232293.Classification
Young, A. J., Smith, L. H., Rouse, E. J., and Hargrove, L. J. (2014). A comparison of the real-time controllability of pattern recognition to conventional myoelectric control for discrete and simultaneous movements. J. Neuroeng. Rehabil. 11, 1–10. doi: 10.1186/1743-0003-11-5
Zaid, M. B., O'Donnell, R. J., Potter, B. K., and Forsberg, J. A. (2019). Orthopaedic osseointegration: state of the art. J. Am. Acad. Orthop. Surg. 27, E977–E985. doi: 10.5435/JAAOS-D-19-00016
Zhang, J., Zhang, Y., Wang, L., Sang, L., Li, L., Li, P., et al. (2018). Brain functional connectivity plasticity within and beyond the sensorimotor network in lower-limb amputees. Front. Hum. Neurosci. 12, 1–11. doi: 10.3389/fnhum.2018.00403
Zheng, J. Y., Kalpakjian, C., Larrága-Martínez, M., Chestek, C. A., and Gates, D. H. (2019). Priorities for the design and control of upper limb prostheses: a focus group study. Disabil. Health J. 12, 706–711. doi: 10.1016/j.dhjo.2019.03.009
Zheng, X., Zhang, J., Chen, T., and Chen, Z. (2003). Longitudinally implanted intrafascicular electrodes for stimulating and recording fascicular physioelectrical signals in the sciatic nerve of rabbits. Microsurgery 23, 268–273. doi: 10.1002/micr.10116
Zhou, P., Lowery, M. M., Englehart, K. B., Huang, H., Li, G., Hargrove, L., et al. (2007). Decoding a new neural-machine interface for control of artificial limbs. J. Neurophysiol. 98, 2974–2982. doi: 10.1152/jn.00178.2007
Ziegler-Graham, K., MacKenzie, E. J., Ephraim, P. L., Travison, T. G., and Brookmeyer, R. (2008). Estimating the prevalence of limb loss in the United States: 2005 to 2050. Arch. Phys. Med. Rehabil. 89, 422–429. doi: 10.1016/j.apmr.2007.11.005
Keywords: neuroprosthetic, amputation, prosthesis, peripheral nerve interface, neuroprosthetic interfacing, sensory motor function, clinical translation, human machine collaboration
Citation: Karczewski AM, Dingle AM and Poore SO (2021) The Need to Work Arm in Arm: Calling for Collaboration in Delivering Neuroprosthetic Limb Replacements. Front. Neurorobot. 15:711028. doi: 10.3389/fnbot.2021.711028
Received: 17 May 2021; Accepted: 22 June 2021;
Published: 21 July 2021.
Edited by:
Calogero Maria Oddo, Sant'Anna School of Advanced Studies, ItalyReviewed by:
Peng Zhang, Huazhong University of Science and Technology, ChinaGiacomo Valle, ETH Zürich, Switzerland
Copyright © 2021 Karczewski, Dingle and Poore. This is an open-access article distributed under the terms of the Creative Commons Attribution License (CC BY). The use, distribution or reproduction in other forums is permitted, provided the original author(s) and the copyright owner(s) are credited and that the original publication in this journal is cited, in accordance with accepted academic practice. No use, distribution or reproduction is permitted which does not comply with these terms.
*Correspondence: Aaron M. Dingle, ZGluZ2xlJiN4MDAwNDA7c3VyZ2VyeS53aXNjLmVkdQ==