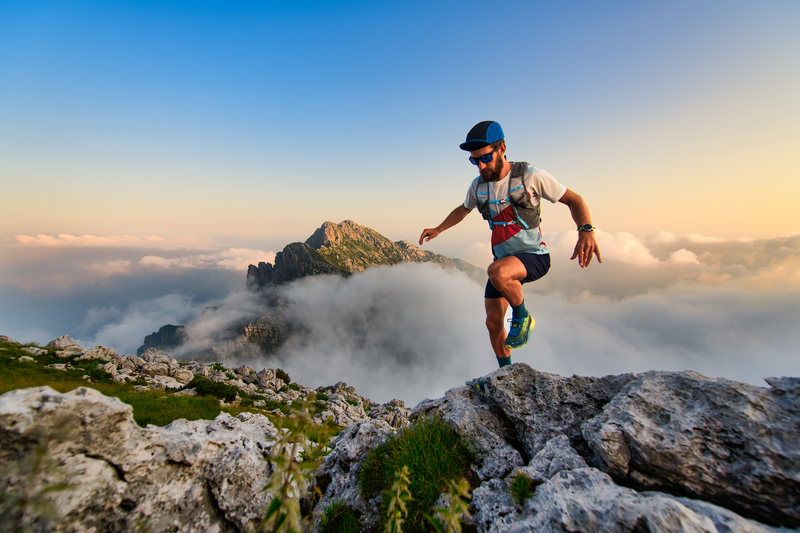
94% of researchers rate our articles as excellent or good
Learn more about the work of our research integrity team to safeguard the quality of each article we publish.
Find out more
EDITORIAL article
Front. Neurol.
Sec. Neurotechnology
Volume 16 - 2025 | doi: 10.3389/fneur.2025.1582142
This article is part of the Research Topic Artificial Intelligence and telemedicine: applications to vascular neurology, neuro-ophthalmology, neuro-otology, and epilepsy View all 5 articles
The final, formatted version of the article will be published soon.
You have multiple emails registered with Frontiers:
Please enter your email address:
If you already have an account, please login
You don't have a Frontiers account ? You can register here
Eye movements are another key area of neurological biomarkers (4), and AI has the potential to support critical medical decision-making (5,6). Duvieusart et al. explored how AI-assisted eye movement analysis can improve diagnostic accuracy, finding that machine learning models trained on gaze test data were able to successfully classify acute vestibular syndrome (AVS) cases as either central (e.g., stroke-related) or peripheral (e.g., vestibular neuritis, benign paroxysmal positional vertical, etc.) (7). As a common neurology consult to the emergency department, AVS evaluation must be timely and accurate (8). Further complicating the matter, in remote settings there may not be specialists present to make an accurate in-person assessment (9,10,11). AIdriven digital biomarkers continue to be refined and will bridge the diagnostic gap for non-specialist clinicians and support accurate triage in the emergency setting. This can be easily paired with telemedicine as a quick, cost-effective method to facilitate a collaborative diagnostic process.Automated nystagmus tracking similarly is essential in neuro-vestibular and neuroophthalmology disorders (12,13,14). Cho et al. presented a deep learning model capable of detecting and tracking nystagmus in real time using object segmentation algorithms to isolate and analyze eye movements (15). This streamlined AI-driven approach achieved accuracy with object segmentation as well as predicting nystagmus direction. In a practical sense, this could help reduce reliance on unnecessary neuroimaging in the setting of clear peripheral vertigo conditions while facilitating prompt intervention. Tracking nystagmus patterns over time can also provide valuable insight into disease progression and treatment efficacy, as mentioned before for pupillometry. Brief clinical observations may not capture episodic eye movement patterns while AI-based tracking can be used for real-time long-term monitoring.This research topic highlights the expanding role of AI in augmenting teleneurology. AIdriven technologies are redefining conventional biomarkers-including pupillometry, ocular motor function, vestibular assessments, and retinal imaging-enhancing clinical practice and deepening our understanding of neurological diseases (5,6). The four studies reviewed here highlight the growing role of AI in improving real-time diagnostics, streamlining clinical workflows, and expanding access to remote healthcare services (1,2,7,15).While these studies focus mainly on ocular digital biomarkers, there is still ongoing potential for AI to expand in other areas of neurology such as stroke, epilepsy, neuromuscular, movement disorders, etc. For example, AI has utility with analysis of subtle abnormal human kinematics (e.g., limb movements or gait), as well as with interpreting complicated electrographic patterns on EEG. The synergistic potential of clinicians and researchers with this technology will enable better patient outcomes. Ongoing challenges include validation, regulatory and ethical considerations, and integration into clinical workflow. Continued interdisciplinary collaboration between neurologists, engineers, and AI specialists is crucial for realizing the potential of AI within neurology. There is a need for a unified seamless platform that can incorporate multi-modal tracking which can revolutionize detection and monitoring, especially considering the expanding spectrum of common and rare neurological diseases.
Keywords: digital biomarkers, precision neurology, artificial intelligence, Telemedicine, neuro-ophthalmology
Received: 24 Feb 2025; Accepted: 03 Mar 2025.
Copyright: © 2025 Browne and Green. This is an open-access article distributed under the terms of the Creative Commons Attribution License (CC BY). The use, distribution or reproduction in other forums is permitted, provided the original author(s) or licensor are credited and that the original publication in this journal is cited, in accordance with accepted academic practice. No use, distribution or reproduction is permitted which does not comply with these terms.
* Correspondence:
Jonathan D. Browne, Department of Neurology, Johns Hopkins University, Baltimore, United States
Disclaimer: All claims expressed in this article are solely those of the authors and do not necessarily represent those of their affiliated organizations, or those of the publisher, the editors and the reviewers. Any product that may be evaluated in this article or claim that may be made by its manufacturer is not guaranteed or endorsed by the publisher.
Research integrity at Frontiers
Learn more about the work of our research integrity team to safeguard the quality of each article we publish.