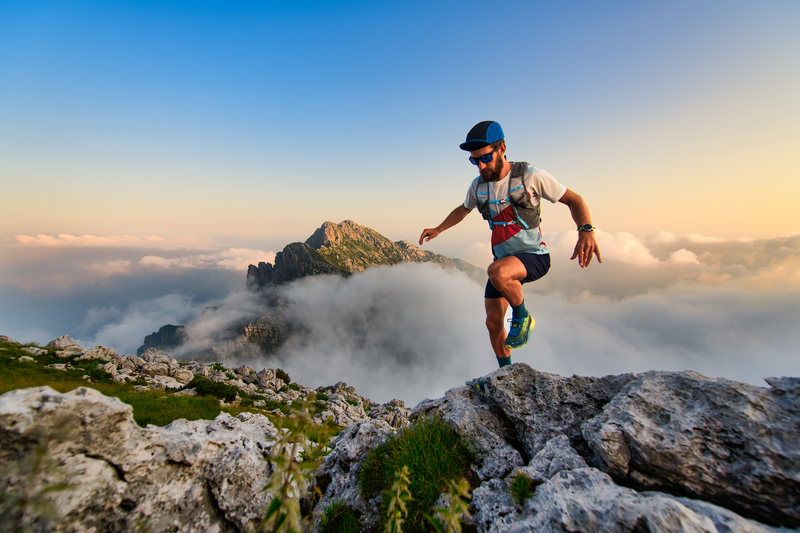
95% of researchers rate our articles as excellent or good
Learn more about the work of our research integrity team to safeguard the quality of each article we publish.
Find out more
ORIGINAL RESEARCH article
Front. Neurol.
Sec. Pediatric Neurology
Volume 16 - 2025 | doi: 10.3389/fneur.2025.1573060
This article is part of the Research Topic Recent Advances in Pediatric Neuroradiology View all 9 articles
The final, formatted version of the article will be published soon.
You have multiple emails registered with Frontiers:
Please enter your email address:
If you already have an account, please login
You don't have a Frontiers account ? You can register here
Objective Our research aims to develop an automated method for segmenting brain CT images in healthy 2-year-old children using the ResU-Net deep learning model. Building on this model, we aim to quantify the volumes of specific brain regions and establish a normative reference database for clinical and research applications. Methods In this retrospective study, we included 1,487 head CT scans of 2-year-old children showing normal radiological findings, which were divided into training (n=1041) and testing (n=446) sets. We preprocessed the Brain CT images by resampling, intensity normalization, and skull stripping. Then, we trained the ResU-Net model on the training set and validated it on the validation set. In addition, we compared the performance of the ResU-Net model with different kernel sizes (3×3×3 and 1×3×3 convolution kernels) against the baseline model, which was the standard 3D U-Net. The performance of the model was evaluated using the Dice similarity score. Once the segmentation model was established, we derived the regional volume parameters. We then conducted statistical analyses to evaluate differences in brain volumes by sex and hemisphere, and performed a Spearman correlation analysis to assess the relationship between brain volume and age. Results
Keywords: brain volume, deep learning, segmentation, CT, Children
Received: 08 Feb 2025; Accepted: 03 Apr 2025.
Copyright: © 2025 Xi, Tu, Zhou, Zhou, Ma and Peng. This is an open-access article distributed under the terms of the Creative Commons Attribution License (CC BY). The use, distribution or reproduction in other forums is permitted, provided the original author(s) or licensor are credited and that the original publication in this journal is cited, in accordance with accepted academic practice. No use, distribution or reproduction is permitted which does not comply with these terms.
* Correspondence:
Jun Ma, Department of Radiology, Beijing Tiantan Hospital, Capital Medical University, Beijing, China
Yun Peng, Imaging Center, Beijing Children’s Hospital, Capital Medical University, National Center for Children’s Health, Beijing, China
Disclaimer: All claims expressed in this article are solely those of the authors and do not necessarily represent those of their affiliated organizations, or those of the publisher, the editors and the reviewers. Any product that may be evaluated in this article or claim that may be made by its manufacturer is not guaranteed or endorsed by the publisher.
Research integrity at Frontiers
Learn more about the work of our research integrity team to safeguard the quality of each article we publish.