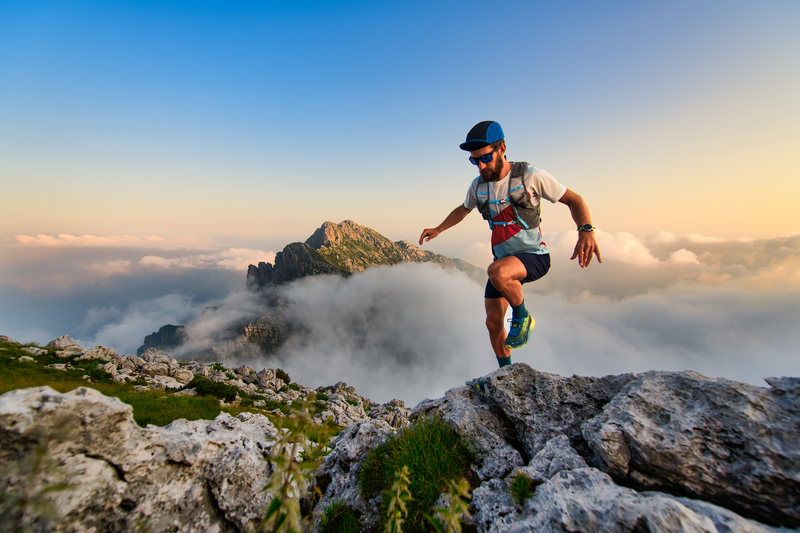
95% of researchers rate our articles as excellent or good
Learn more about the work of our research integrity team to safeguard the quality of each article we publish.
Find out more
ORIGINAL RESEARCH article
Front. Neurol.
Sec. Artificial Intelligence in Neurology
Volume 16 - 2025 | doi: 10.3389/fneur.2025.1562785
This article is part of the Research Topic Modeling Physical Activities, Behavioral Patterns, and Symptoms in Aging and Neurological Disorders via Novel Sensing and AI Techniques View all 3 articles
The final, formatted version of the article will be published soon.
You have multiple emails registered with Frontiers:
Please enter your email address:
If you already have an account, please login
You don't have a Frontiers account ? You can register here
Introduction: Digital technologies have significantly advanced the detection of emotional disorders (ED) in clinical settings. However, their adoption for long-term monitoring remains limited due to reliance on fixed testing formats and active user participation. This study introduces a novel approach utilizing common ball game videos-table tennis-to implicitly capture eye movement trajectories and identify ED through natural viewing behavior.Methods: An eye movement data collection system was developed using VR glasses to display sports videos while recording participants' eye movements. Based on prior research and collected data, four primary eye movement behaviors were identified, along with 14 associated features.Statistical significance was assessed using t-tests and U-tests, and machine learning models were employed for classification (SVM for single-feature analysis and a decision tree for significant features) with k-fold validation. The reliability of the proposed paradigm and extracted features was evaluated using intraclass correlation coefficient (ICC) analysis.Results: Significance tests revealed 11 significant features in table tennis videos, encompassing exploration, fixation, and saccade behaviors, while only 3 features in tennis videos, which served as a supplemental stimulus, were salient in the re-testing. GazeEntropy emerged as the most predictive feature, achieving an accuracy of 0.88 with a significance p-value of 0.0002. A decision tree model trained on all significant features achieved 0.92 accuracy, 0.80 precision, and an AUC of 0.94. ICC analysis further confirmed the high reliability and significance of key features, including GazeEntropy and fixation metrics (average, maximum, and standard deviation).Discussion: This study highlights the potential of ball game video viewing as a natural and effective paradigm for ED identification, particularly focusing on two key characteristics of ED:1 Sample et al.curiosity exploration and psychomotor function. Additionally, participant preferences for video content significantly influenced diagnostic performance. We propose that future in-home, longterm monitoring of psychological conditions can leverage interactions with daily digital devices, integrating behavioral analysis seamlessly into everyday life.
Keywords: Emotional disorder, diagnosis, machine learning, EYE MOVEMENT, Sports Watching
Received: 18 Jan 2025; Accepted: 31 Mar 2025.
Copyright: © 2025 Qiang, Yang, Zhang, Liu, Wang, Zhang, Long, Xu and Sun. This is an open-access article distributed under the terms of the Creative Commons Attribution License (CC BY). The use, distribution or reproduction in other forums is permitted, provided the original author(s) or licensor are credited and that the original publication in this journal is cited, in accordance with accepted academic practice. No use, distribution or reproduction is permitted which does not comply with these terms.
* Correspondence:
Weiwei Xu, The First hospital of Hebei Medical University, Shijiazhuang, 050000, Hebei Province, China
Wei Sun, Institute of Software, Chinese Academy of Sciences (CAS), Beijing, 100190, Beijing Municipality, China
Disclaimer: All claims expressed in this article are solely those of the authors and do not necessarily represent those of their affiliated organizations, or those of the publisher, the editors and the reviewers. Any product that may be evaluated in this article or claim that may be made by its manufacturer is not guaranteed or endorsed by the publisher.
Research integrity at Frontiers
Learn more about the work of our research integrity team to safeguard the quality of each article we publish.