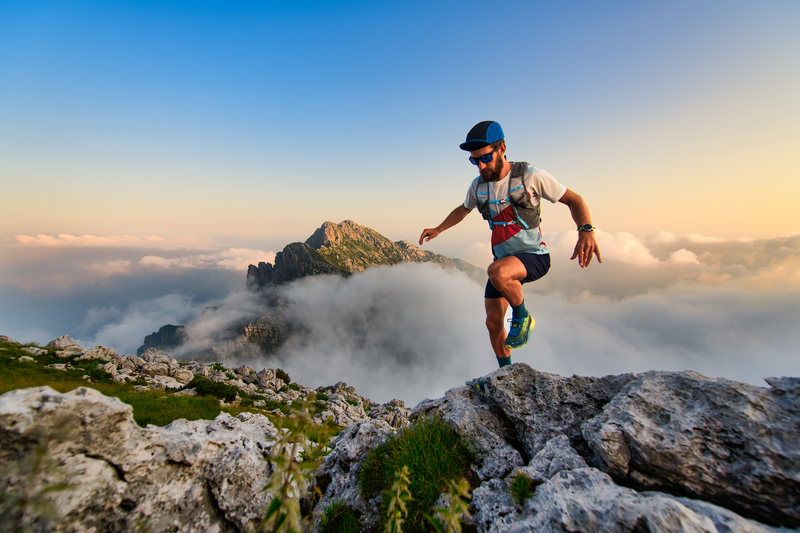
95% of researchers rate our articles as excellent or good
Learn more about the work of our research integrity team to safeguard the quality of each article we publish.
Find out more
ORIGINAL RESEARCH article
Front. Neurol.
Sec. Neurological Biomarkers
Volume 16 - 2025 | doi: 10.3389/fneur.2025.1562247
This article is part of the Research Topic Evaluation of Fitness in Stroke Survivors View all 8 articles
The final, formatted version of the article will be published soon.
You have multiple emails registered with Frontiers:
Please enter your email address:
If you already have an account, please login
You don't have a Frontiers account ? You can register here
Background: Our study aims to utilize unsupervised machine learning methods to perform inflammation clustering on stroke patients via novel CBC-derived inflammatory indicators (NLR, PLR, NPAR, SII, SIRI, and AISI), evaluate the mortality risk among these different clusters and construct prognostic models to provide reference for clinical management.A cross-sectional analysis was conducted using data from stroke participants in the U.S. NHANES 1999-2018. Weighted multivariate logistic regression was used to construct different models; consensus clustering methods were employed to subtype stroke patients based on inflammatory marker levels; LASSO regression analysis was used to construct an inflammatory risk score model to analyze the survival risks of different inflammatory subtypes; WQS regression, Cox regression, as well as XGBoost, random forest, and SVMRFE machine learning methods were used to screen hub markers which affected stroke prognosis; finally, a prognostic nomogram model based on hub inflammatory markers was constructed and evaluated using calibration and DCA curves.Results: A total of 918 stroke patients with a median follow-up of 79 months and 369 deaths. Weighted multivariate logistic regression analysis revealed that high SIRI and NPAR levels were significantly positively correlated with increased all-cause mortality risk in stroke patients (P<0.001), independent of potential confounders; Consensus clustering divided patients into two inflammatory subgroups via SIRI and NPAR, with subgroup 2 having significantly higher markers and mortality risks than subgroup 1 (P<0.001); LASSO regression analysis showed subgroup 2 had higher risk scores and shorter overall survival than subgroup 1 (HR, 1.99 (1.61-2.45), P<0.001); WQS regression, Cox regression, and machine learning methods identified NPAR and SIRI as hub prognostic inflammatory markers; The nomogram prognostic model with NPAR and SIRI demonstrated the best net benefit for predicting 1, 3, 5 and 10-year overall survival in stroke patients.This study shows NPAR and SIRI were key prognostic inflammatory markers and positively correlated with mortality risk (P<0.001) for stroke patients. Patients would been divided into 2 inflammatory subtypes via them, with subtype 2 having higher values and mortality risks (P<0.001). It suggests that enhanced monitoring and management for patients with high SIRI and NPAR levels to improve survival outcomes.
Keywords: Stroke, machine learning, consensus cluster, Inflammation subtype, neutrophil-percentage-to-albumin ratio, systemic inflammatory response index
Received: 17 Jan 2025; Accepted: 27 Mar 2025.
Copyright: © 2025 Zhang, Wang, Zhou and Ma. This is an open-access article distributed under the terms of the Creative Commons Attribution License (CC BY). The use, distribution or reproduction in other forums is permitted, provided the original author(s) or licensor are credited and that the original publication in this journal is cited, in accordance with accepted academic practice. No use, distribution or reproduction is permitted which does not comply with these terms.
* Correspondence:
Chunjuan Zhang, Haiyan People's Hospital, Zhejiang, China
Xiaodong Ma, Haiyan People's Hospital, Zhejiang, China
Disclaimer: All claims expressed in this article are solely those of the authors and do not necessarily represent those of their affiliated organizations, or those of the publisher, the editors and the reviewers. Any product that may be evaluated in this article or claim that may be made by its manufacturer is not guaranteed or endorsed by the publisher.
Research integrity at Frontiers
Learn more about the work of our research integrity team to safeguard the quality of each article we publish.