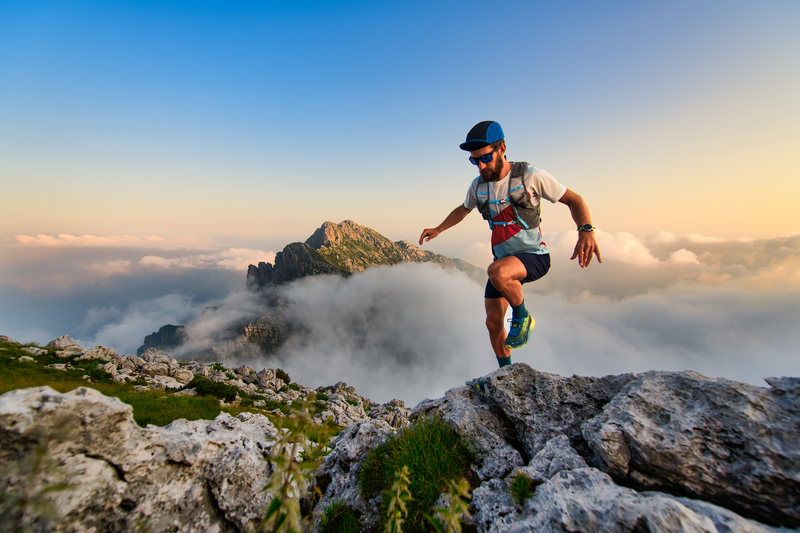
95% of researchers rate our articles as excellent or good
Learn more about the work of our research integrity team to safeguard the quality of each article we publish.
Find out more
ORIGINAL RESEARCH article
Front. Neurol.
Sec. Artificial Intelligence in Neurology
Volume 16 - 2025 | doi: 10.3389/fneur.2025.1555215
This article is part of the Research Topic Modeling Physical Activities, Behavioral Patterns, and Symptoms in Aging and Neurological Disorders via Novel Sensing and AI Techniques View all 4 articles
The final, formatted version of the article will be published soon.
You have multiple emails registered with Frontiers:
Please enter your email address:
If you already have an account, please login
You don't have a Frontiers account ? You can register here
In many countries, patients with headache disorders such as migraine are among the underrecognized and under-diagnosed. Patients who are affected by headache disorders are not generally aware of the seriousness of these headaches since it does not cause death and are not contagious.In many cases, patients with migraine are often misdiagnosed as regular headaches.In this paper, we present the study of migraine, known triggers, different phases, classification of migraine into different types based on clinical studies, and the use of various machine learning algorithms such as logistic regression (LR), support vector machine (SVM), random forest (RF) and artificial neural network (ANN) to learn and classify different migraine types. This paper will only consider using these methods for diagnostic purposes. Models based on these algorithms are then trained using the dataset, a compilation of types of migraine experienced by various patients.These models are then used to classify the types of migraines, and the results are analyzed.The results of the machine learning models trained on the dataset are verified for their performance.The results are further evaluated by selective sampling and tuning, and improved performance is observed. The precision and accuracy obtained by the Support Vector Machine and Artificial Neural Network are 91% compared to Logistic Regression (90%) and Random Forest (87%).These models are run with the dataset without optimal tuning for the overall dataset for different migraine types, which is further improved with selective sampling and optimal tuning. These results indicate that the discussed models are relatively good and can be used with high precision and accuracy for diagnosing different types of migraine.Our study presents our realistic assessment of promising models that are dependable in aiding physicians. The study shows the performance of various models based on the classification metrics computed for each model. It is evident from the results that Artificial Neural Network (ANN) performs better, irrespective of the sampling techniques used. With these machine learning models, types of migraines can be classified with high accuracy and reliability so that physicians can make timely clinical diagnoses of patients.
Keywords: migraine triggers, migraine phases, migraine types, Logistic regression, Support vector machine, random forest, neural networks
Received: 03 Jan 2025; Accepted: 07 Apr 2025.
Copyright: © 2025 Reddy and Reddy. This is an open-access article distributed under the terms of the Creative Commons Attribution License (CC BY). The use, distribution or reproduction in other forums is permitted, provided the original author(s) or licensor are credited and that the original publication in this journal is cited, in accordance with accepted academic practice. No use, distribution or reproduction is permitted which does not comply with these terms.
* Correspondence:
Ajit Reddy, Lockheed Martin (United States), Bethesda, Maryland, United States
Disclaimer: All claims expressed in this article are solely those of the authors and do not necessarily represent those of their affiliated organizations, or those of the publisher, the editors and the reviewers. Any product that may be evaluated in this article or claim that may be made by its manufacturer is not guaranteed or endorsed by the publisher.
Research integrity at Frontiers
Learn more about the work of our research integrity team to safeguard the quality of each article we publish.