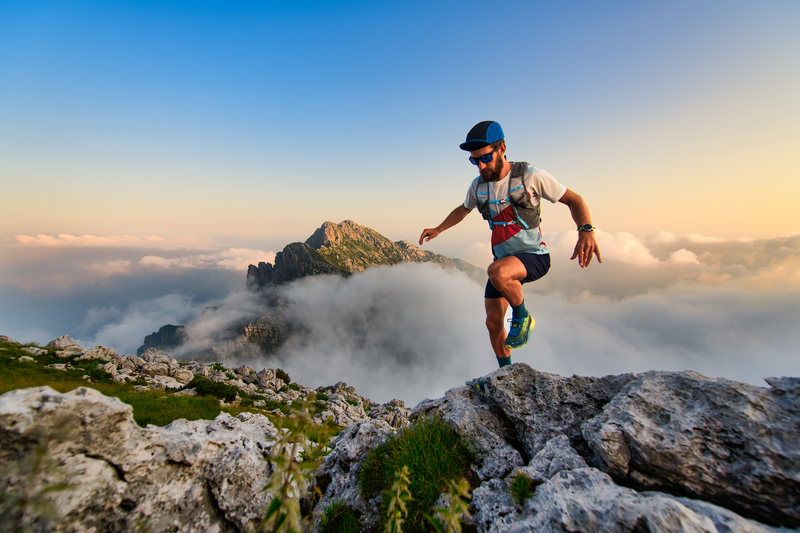
95% of researchers rate our articles as excellent or good
Learn more about the work of our research integrity team to safeguard the quality of each article we publish.
Find out more
ORIGINAL RESEARCH article
Front. Neurol.
Sec. Cognitive and Behavioral Neurology
Volume 16 - 2025 | doi: 10.3389/fneur.2025.1550789
The final, formatted version of the article will be published soon.
You have multiple emails registered with Frontiers:
Please enter your email address:
If you already have an account, please login
You don't have a Frontiers account ? You can register here
Parkinson's Disease (PD) is a neurodegenerative disorder with significant variability in disease progression. Identifying clinical and environmental risk factors associated with severe progression is essential for early diagnosis and personalized treatment. This study evaluates the performance of Random Forest (RF) and Logistic Regression (LR) models in forecasting the major risk factors associated with severe Parkinson's diseasePD progression.We performed a retrospective analysis of 378 PD patients (aged 40-75 years) with at least two years of follow-up. The dataset included patient demographics, clinical features, medication history, comorbidities, and environmental exposures. The data were randomly split into a training group (70%) and a validation group (30%). Both the RF and LR models were trained on the training set, and performance was assessed through accuracy, sensitivity, specificity, and the Area Under the Curve (AUC) derived from ROC analysis.Both models identified similar risk factors for severe PD progression, including older age, tremor-dominant motor subtype, long-term levodopa use, comorbid depression, and occupational pesticide exposure. The RF model outperformed the LR model, achieving an AUC of 0.85, accuracy of 82%, sensitivity of 79%, and specificity of 85%. In comparison, the LR model had an AUC of 0.78, accuracy of 76%, sensitivity of 74%, and specificity of 79%. ROC analysis showed that while both models could distinguish between slow and rapid disease progression, the RF model had stronger discriminatory power, particularly for identifying high-risk patients.The RF model provides better predictive accuracy and discriminatory power compared to Logistic Regression in identifying risk factors for severe PD progression. This study highlights the potential of machine learning techniques like Random Forest for early risk stratification and personalized management of PD.
Keywords: Parkinson's disease, random forest, Logistic regression, Risk factors, disease progression
Received: 24 Dec 2024; Accepted: 18 Mar 2025.
Copyright: © 2025 Zhang, Tan, Huang, Hao and Wan. This is an open-access article distributed under the terms of the Creative Commons Attribution License (CC BY). The use, distribution or reproduction in other forums is permitted, provided the original author(s) or licensor are credited and that the original publication in this journal is cited, in accordance with accepted academic practice. No use, distribution or reproduction is permitted which does not comply with these terms.
* Correspondence:
Qian Zhang, Hubei Provincial Third People's Hospital (Zhongshan Hospital), Wuhan, China
Disclaimer: All claims expressed in this article are solely those of the authors and do not necessarily represent those of their affiliated organizations, or those of the publisher, the editors and the reviewers. Any product that may be evaluated in this article or claim that may be made by its manufacturer is not guaranteed or endorsed by the publisher.
Research integrity at Frontiers
Learn more about the work of our research integrity team to safeguard the quality of each article we publish.