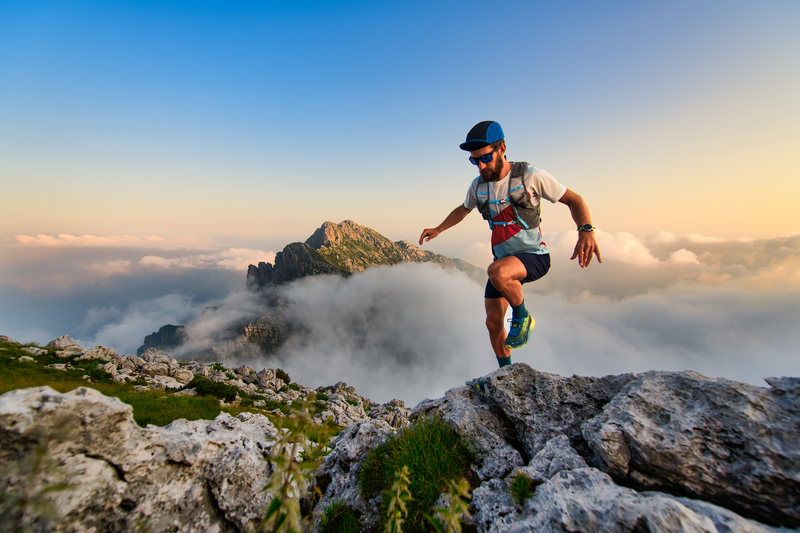
94% of researchers rate our articles as excellent or good
Learn more about the work of our research integrity team to safeguard the quality of each article we publish.
Find out more
ORIGINAL RESEARCH article
Front. Neurol.
Sec. Neuroepidemiology
Volume 16 - 2025 | doi: 10.3389/fneur.2025.1548064
The final, formatted version of the article will be published soon.
You have multiple emails registered with Frontiers:
Please enter your email address:
If you already have an account, please login
You don't have a Frontiers account ? You can register here
Aim: This study aims to investigated the associations between estimated plasma volume status (ePVS) and 30-day and 1-year mortality in intracerebral hemorrhage (ICH) patients, providing insights into the management in ICH. Methods: Data of adult ICH patients were extracted from both the Medical Information Mart for Intensive Care IV (MIMIC-IV) database and the Hospital Information System (HIS) in this retrospective cohort study. Univariate and multivariate Cox regression analyses , and restricted cubic spline plots (RCS) were conducted to explore the associations between ePVS levels and both 30-day and 1-year mortality, with hazard ratios (HR) and 95% confidence intervals (CI) used for evaluation. Subgroup analyses were performed to further investigate these associations. Results: Among 2,512 eligible patients from the MIMIC-IV database, 655 (26.07%) died within 30 days, with 1,254 (49.92%) had died by the 1-year follow-up. After adjusting for covariates, elevated ePVS was independently associated with both 30-day mortality (HR=1.05, 95%CI: 1.01-1.09) and 1-year mortality (HR=1.09, 95% CI: 1.06-1.13). Compared to patients with ePVS levels of [4.63-5.79), those with ePVS levels ≥5.79 had a higher risk of 30-day mortality (HR: 1.36, 95%CI: 1.12-1.64) and 1-year mortality (HR=1.24, 95% CI: 1.08-1.42).Among 515 eligible patients from the HIS, 132 (25.60%) died within 30 days, with 288 (55.90%) mortality observed at 1-year follow-up. After adjusting for covariates, elevated ePVS was independently associated with both 30-day mortality (HR=1.33, 95%CI: 1.23-1.43) and 1-year mortality (HR=1.26, 95% CI: 1.18-1.35). Comparing to patients with ePVS levels of [4.63-5.79), those with ePVS levels of ≥5.79 had a higher risk of 30-day mortality (HR:2.21, 95%CI: 1.48-3.30) and and 1-year mortality (HR=2.75, 95% CI: 2.04-3.72). Additionally, subgroup analyses demonstrated that ePVS was significantly associated with 30-day mortality or 1-year mortality derived from MIMIC-IV and HIS in most subgroups (P<0.05). And RCS analysis indicates that, whether using MIMIC-IV or HIS data, ePVS was linearly associated with 30-day or 1-year mortality.Higher ePVS levels may be a potential risk factor for 30-day and 1-year mortality in ICH patients, suggesting that timely monitoring and stabilization of ePVS could improve prognosis in this population. However, further studies are needed to validate these fingings.
Keywords: ePVS, intracerebral hemorrhage, 30-day mortality, 1-year mortality, MIMIC-IV database
Received: 19 Dec 2024; Accepted: 04 Mar 2025.
Copyright: © 2025 Shen, Shen, Wang, Hu, Xu, Feng, Yang, Zhang and Liang. This is an open-access article distributed under the terms of the Creative Commons Attribution License (CC BY). The use, distribution or reproduction in other forums is permitted, provided the original author(s) or licensor are credited and that the original publication in this journal is cited, in accordance with accepted academic practice. No use, distribution or reproduction is permitted which does not comply with these terms.
* Correspondence:
Yongze Shen, First Affiliated Hospital of Harbin Medical University, Harbin, China
Disclaimer: All claims expressed in this article are solely those of the authors and do not necessarily represent those of their affiliated organizations, or those of the publisher, the editors and the reviewers. Any product that may be evaluated in this article or claim that may be made by its manufacturer is not guaranteed or endorsed by the publisher.
Research integrity at Frontiers
Learn more about the work of our research integrity team to safeguard the quality of each article we publish.