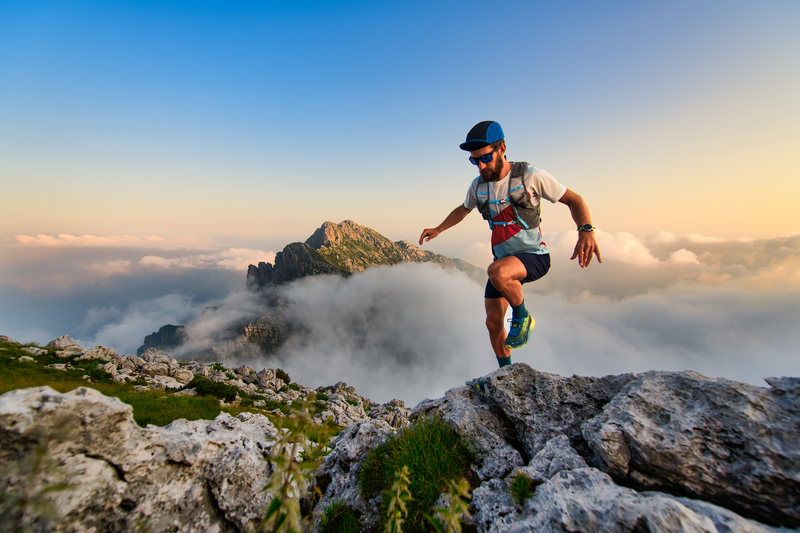
94% of researchers rate our articles as excellent or good
Learn more about the work of our research integrity team to safeguard the quality of each article we publish.
Find out more
ORIGINAL RESEARCH article
Front. Neurol.
Sec. Movement Disorders
Volume 16 - 2025 | doi: 10.3389/fneur.2025.1544453
The final, formatted version of the article will be published soon.
You have multiple emails registered with Frontiers:
Please enter your email address:
If you already have an account, please login
You don't have a Frontiers account ? You can register here
Introduction: Gait assessments in a clinical setting may not accurately reflect mobility in everyday life. To better understand gait during daily life, we compared measures that discriminated Friedreich ataxia (FRDA) from healthy control (HC) subjects in prescribed clinic tests and free, daily-life monitoring. Methods: We recruited 9 people with FRDA (median age: 20, IQR [12,48] years). A comparative healthy control (HC) subject cohort of 9 was sampled using propensity matching on age (median age: 18 [13,22] years). Subjects wore 3 inertial sensors (one each foot and lower back) in the laboratory during a 2-minute walk at a natural pace, followed by 7 days of daily life. For daily life analysis, a total of 99,216 strides across 1,008 hours of recording were included. Mann-Whitney U test and area under the curve (AUC) compared gait differences between FRDA and HC when assessed in the laboratory and daily life. Pairwise Wilcoxon tests also compared if participants exhibited different metric values between the two environments. Results: The FRDA group exhibited lower levels of daily activity. Measures that best discriminated gait characteristics of FRDA from HC differed between environments. Variation in elevation of the feet at midswing best discriminated in-clinic (Clinic AUC = 1, Home AUC = 0.69), whereas slow gait speed performed best in daily life (Home AUC = 1, Clinic AUC = 0.64). Of the 17 measures tested, 11 had an AUC > 0.8 in-clinic and 8 had an AUC >0.8 at home. Variability of swing time (Clinic AUC = 0.97, Home AUC = 0.94) and double-support time (Clinic AUC = 0.94, Home AUC = 0.94) were the most sensitive and specific for FRDA in both environments. Conclusions: Digital gait characteristics from inertial sensors are sensitive and specific for FRDA in both environments. However, different gait measures were more sensitive and specific during free-living versus prescribed gait, suggesting that in-clinic gait does not reflect daily life gait.
Keywords: Gait, free-living, Friedreich's Ataxia, Wearable inertial sensors, Digital biomarker, Clinical Trials
Received: 12 Dec 2024; Accepted: 03 Mar 2025.
Copyright: © 2025 Casey, Shah, Muzyka, McNames, El-Gohary, Sowalsky, Safarpour, Carlson-Kuhta, Rummey, Horak and Gomez. This is an open-access article distributed under the terms of the Creative Commons Attribution License (CC BY). The use, distribution or reproduction in other forums is permitted, provided the original author(s) or licensor are credited and that the original publication in this journal is cited, in accordance with accepted academic practice. No use, distribution or reproduction is permitted which does not comply with these terms.
* Correspondence:
Hannah L. Casey, Department of Neurology, Division of Biological Sciences, The University of Chicago, Chicago, 60637-1470, Illinois, United States
Disclaimer: All claims expressed in this article are solely those of the authors and do not necessarily represent those of their affiliated organizations, or those of the publisher, the editors and the reviewers. Any product that may be evaluated in this article or claim that may be made by its manufacturer is not guaranteed or endorsed by the publisher.
Research integrity at Frontiers
Learn more about the work of our research integrity team to safeguard the quality of each article we publish.