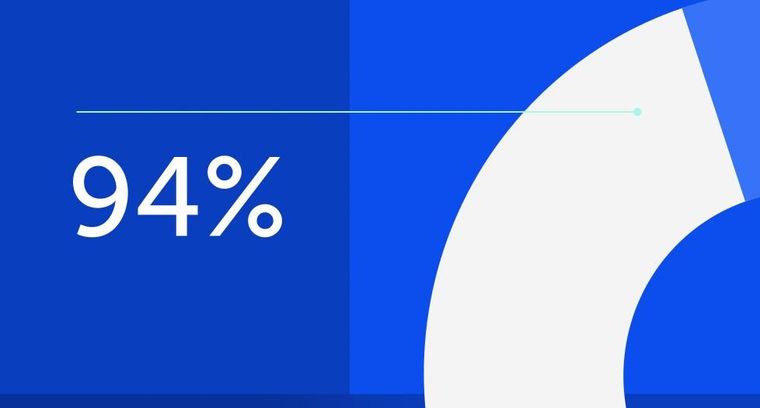
94% of researchers rate our articles as excellent or good
Learn more about the work of our research integrity team to safeguard the quality of each article we publish.
Find out more
SYSTEMATIC REVIEW article
Front. Neurol.
Sec. Artificial Intelligence in Neurology
Volume 16 - 2025 | doi: 10.3389/fneur.2025.1536751
This article is part of the Research TopicAI's Transformative Role in Neuro-Intervention: Enhancing Diagnosis and Treatment StrategiesView all 4 articles
The final, formatted version of the article will be published soon.
Select one of your emails
You have multiple emails registered with Frontiers:
Notify me on publication
Please enter your email address:
If you already have an account, please login
You don't have a Frontiers account ? You can register here
Accurate preoperative grading of meningiomas is crucial for selecting the most suitable treatment strategies and predicting patient outcomes. Traditional MRI-based assessments are often insufficient to distinguish between low-and high-grade meningiomas reliably. Deep learning (DL) models have emerged as promising tools for automated histopathological grading using imaging data. This systematic review and meta-analysis aimed to comprehensively evaluate the diagnostic performance of deep learning (DL) models for meningioma grading.This study was conducted in accordance with the PRISMA-DTA guidelines and was prospectively registered on the Open Science Framework. A systematic search of PubMed, Scopus, and Web of Science was performed up to March 2025. Studies using DL models to classify meningiomas based on imaging data were included. A random-effects meta-analysis was used to pool sensitivity, specificity, accuracy, and area under the receiver operating characteristic curve (AUC). A bivariate randomeffects model was used to fit the summary receiver operating characteristic (SROC) curve. Study quality was assessed using the Newcastle-Ottawa Scale, and publication bias was evaluated using Egger's test.Twenty-seven studies involving 13130 patients were included. The pooled sensitivity was 92.31% (95% CI: 92.1-92.52%), specificity 95.3% (95% CI: 95.11-95.48%), and accuracy 97.97% (95% CI: 97.35-97.98%), with an AUC of 0.97 (95% CI: 0.96-0.98). The bivariate SROC curve demonstrated excellent diagnostic performance, characterized by a relatively narrow 95% confidence interval despite moderate to high heterogeneity (I² = 79.7%, p < 0.001).DL models demonstrate high diagnostic accuracy for automatic meningioma grading and could serve as valuable clinical decision-support tools.
Keywords: Histopathological grading, deep learning, Meningioma, Meta-analysis, AI
Received: 29 Nov 2024; Accepted: 14 Apr 2025.
Copyright: © 2025 Asadi Anar, Noori Mirtaheri, Akhbari, Najafi, Mehrabi, Babapour, Rahimian, Rigi, Rahbarbaghbani, Mobaraki, Masoumi, Nouri, Mirzohreh, Sadat RAfiei, Golkar, Asadollah Salmanpour, Vesali Mahmoud and Gholami Chahkand. This is an open-access article distributed under the terms of the Creative Commons Attribution License (CC BY). The use, distribution or reproduction in other forums is permitted, provided the original author(s) or licensor are credited and that the original publication in this journal is cited, in accordance with accepted academic practice. No use, distribution or reproduction is permitted which does not comply with these terms.
* Correspondence: Mahsa Asadi Anar, College of Medicine, University of Arizona, Tucson, United States
Disclaimer: All claims expressed in this article are solely those of the authors and do not necessarily represent those of their affiliated organizations, or those of the publisher, the editors and the reviewers. Any product that may be evaluated in this article or claim that may be made by its manufacturer is not guaranteed or endorsed by the publisher.
Supplementary Material
Research integrity at Frontiers
Learn more about the work of our research integrity team to safeguard the quality of each article we publish.