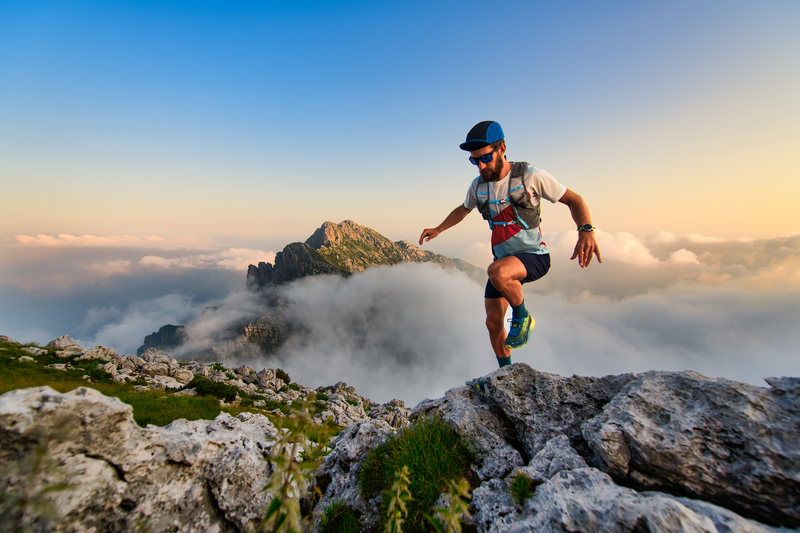
94% of researchers rate our articles as excellent or good
Learn more about the work of our research integrity team to safeguard the quality of each article we publish.
Find out more
ORIGINAL RESEARCH article
Front. Neurol.
Sec. Stroke
Volume 16 - 2025 | doi: 10.3389/fneur.2025.1534961
The final, formatted version of the article will be published soon.
You have multiple emails registered with Frontiers:
Please enter your email address:
If you already have an account, please login
You don't have a Frontiers account ? You can register here
Background Stroke is a leading cause of mortality and disability globally. Among ischemic stroke patients, those with moderate to severe consciousness disorders constitute a particularly high-risk subgroup. Accurate predictive models are essential for guiding clinical decisions in this population.This study aimed to develop and validate an automated scoring system using machine learning algorithms for predicting short-term (3-and 7-day) and relatively long-term (30-and 90-day) mortality in this population.Methods This retrospective observational study utilized data from the MIMIC-IV database, including 648 ischemic stroke patients with Glasgow Coma Scale (GCS) scores ≤12, admitted to the ICU between 2008 and 2019. Patients with GCS scores indicating speech dysfunction but clear consciousness were excluded. A total of 47 candidate variables were evaluated, and the top six predictors for each mortality model were identified using the AutoScore framework. Model performance was assessed using the area under the curve (AUC) from receiver operating characteristic (ROC) analyses.The median age of the cohort was 76.8 years (IQR, 64.97-86.34), with mortality rates of 8.02% at 3 days, 18.67% at 7 days, 33.49% at 30 days, and 38.89% at 90 days. The AUCs for the test cohort's 3-, 7-, 30-, and 90-day mortality prediction models were 0.698, 0.678, 0.724, and 0.730, respectively.We developed and validated a novel machine learning-based scoring tool that effectively predicts both short-term and relatively long-term mortality in ischemic stroke patients with moderate to severe consciousness disorders. This tool has the potential to enhance clinical decision-making and resource allocation for these patients in the ICU.
Keywords: ischemic stroke, Consciousness Disorders, Scoring model, machine learning, Mortality Ischemic stroke, Mortality
Received: 27 Nov 2024; Accepted: 04 Mar 2025.
Copyright: © 2025 Zhou, Chen, Mei, Chen, Cao, Xu, Wang, Ye and Cheng. This is an open-access article distributed under the terms of the Creative Commons Attribution License (CC BY). The use, distribution or reproduction in other forums is permitted, provided the original author(s) or licensor are credited and that the original publication in this journal is cited, in accordance with accepted academic practice. No use, distribution or reproduction is permitted which does not comply with these terms.
* Correspondence:
Lei Ye, Department of Neurosurgery, First Affiliated Hospital of Anhui Medical University, Hefei, Anhui Province, China
Hongwei Cheng, Department of Neurosurgery, First Affiliated Hospital of Anhui Medical University, Hefei, Anhui Province, China
Disclaimer: All claims expressed in this article are solely those of the authors and do not necessarily represent those of their affiliated organizations, or those of the publisher, the editors and the reviewers. Any product that may be evaluated in this article or claim that may be made by its manufacturer is not guaranteed or endorsed by the publisher.
Research integrity at Frontiers
Learn more about the work of our research integrity team to safeguard the quality of each article we publish.