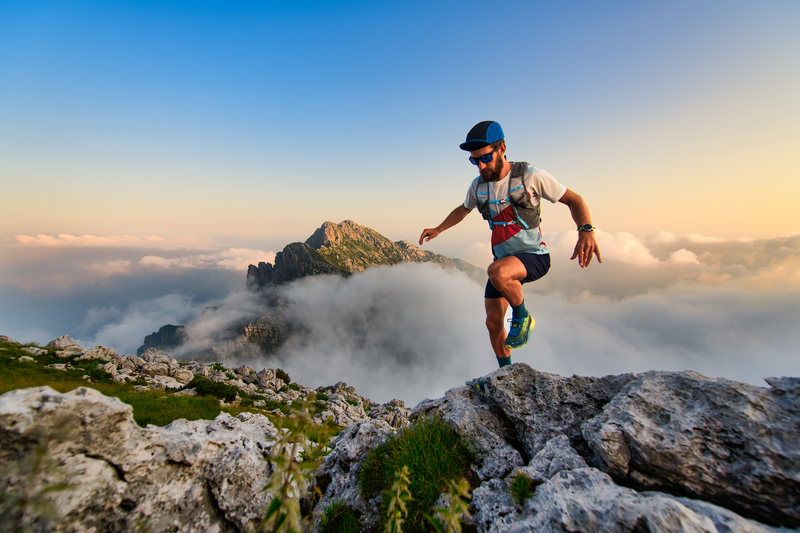
94% of researchers rate our articles as excellent or good
Learn more about the work of our research integrity team to safeguard the quality of each article we publish.
Find out more
ORIGINAL RESEARCH article
Front. Neurol.
Sec. Movement Disorders
Volume 16 - 2025 | doi: 10.3389/fneur.2025.1533942
The final, formatted version of the article will be published soon.
You have multiple emails registered with Frontiers:
Please enter your email address:
If you already have an account, please login
You don't have a Frontiers account ? You can register here
We aimed to clarify the influence of facial expressions on providing early recognition and diagnosis of Parkinson's disease (PD).We included 18 people with PD and 18 controls. The participants were asked to perform 12 monosyllabic tests, 8 disyllabic tests, and 6 multisyllabic tests and the whole process were recorded.Then 26 video clips recorded were used to decipher the facial muscle movements and face expression via Noldus FaceReader 7.0 software. 16 suitable variables were selected to construct a Bayesian network model.The area under the curve of the unsegmented-syllabic, monosyllabic, dissyllabic, and multisyllabic training models was 0.960, 0.958, and 0.962, respectively, with no significant difference between the models. Based on the Bayesian network models, we found that except for valence in the disyllabic model, all positive facial expressions in the four models are negatively associated with the probability of PD. Moreover, negative facial expressions, including sadness, anger, scared, and disgust in the unsegmented-syllabic, monosyllabic, and multisyllabic models, as well as anger in the disyllabic model, are positively correlated to the probability of PD. Sadness, scare and disgust in disyllabic model are negatively associated with the probability of PD.Conclusions: Except for sad, scared, and disgusted generated by reading disyllables, negative expressions generated by reading other syllables were positively associated with the probability of PD.In addition, scared expressions produced during monosyllabic reading had the greatest effect on the probability of PD, and disgusted expressions produced during multisyllabic reading had the least effect.
Keywords: Parkinson's disease, facial expressions, Bayesian network, tree-augmented network, Prediction model, Noldus FaceReader
Received: 25 Nov 2024; Accepted: 10 Mar 2025.
Copyright: © 2025 Mouse, Gong, Liu, Xu, Zou, Huang and Yang. This is an open-access article distributed under the terms of the Creative Commons Attribution License (CC BY). The use, distribution or reproduction in other forums is permitted, provided the original author(s) or licensor are credited and that the original publication in this journal is cited, in accordance with accepted academic practice. No use, distribution or reproduction is permitted which does not comply with these terms.
* Correspondence:
Xi Yang, Chengdu Medical College, Chengdu, 610500, Sichuan, China
Disclaimer: All claims expressed in this article are solely those of the authors and do not necessarily represent those of their affiliated organizations, or those of the publisher, the editors and the reviewers. Any product that may be evaluated in this article or claim that may be made by its manufacturer is not guaranteed or endorsed by the publisher.
Research integrity at Frontiers
Learn more about the work of our research integrity team to safeguard the quality of each article we publish.