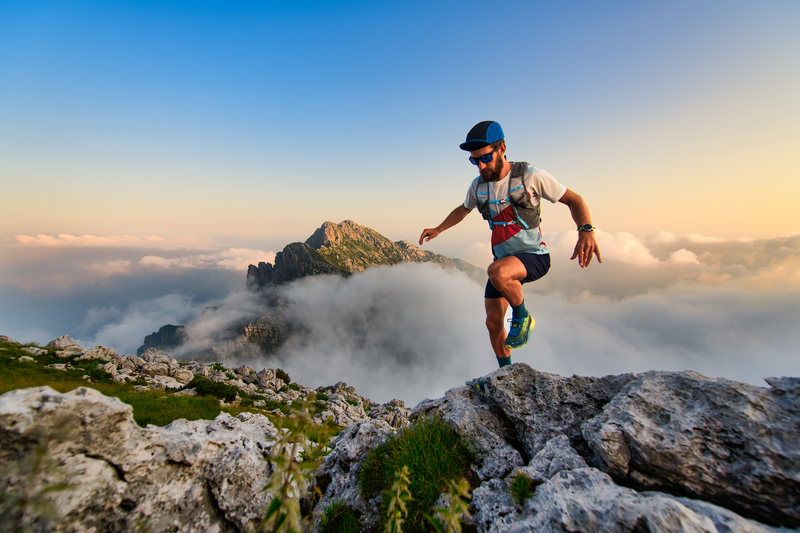
95% of researchers rate our articles as excellent or good
Learn more about the work of our research integrity team to safeguard the quality of each article we publish.
Find out more
ORIGINAL RESEARCH article
Front. Neurol.
Sec. Neurotrauma
Volume 16 - 2025 | doi: 10.3389/fneur.2025.1525416
This article is part of the Research Topic Frontier Research on Artificial Intelligence and Radiomics in Neurodegenerative Diseases View all 12 articles
The final, formatted version of the article will be published soon.
You have multiple emails registered with Frontiers:
Please enter your email address:
If you already have an account, please login
You don't have a Frontiers account ? You can register here
Spinal cord injury (SCI) represents a severe disorder of the central nervous system that causes sensory and motor dysfunction. As an essential trace element in living organisms, copper plays a vital role in life activities. Cuproptosis is a novel metal ion-induced cell death distinct from ferroptosis and closely related to mitochondrial metabolism. In this study, we conducted a comprehensive bioinformatics analysis of the SCI (GSE151371) dataset to explore the variations in expression and correlations of Cuproptosis-related genes (CRGs) between SCI and non-SCI and used the ssGSEA algorithm for immune infiltration analysis. In addition, unsupervised clustering was performed based on CRGs, and WGCNA, GO, and KEGG enrichment analyses were performed based on the clustering results. Then, three machine learning models were constructed, the best candidate genes were selected, and finally, the nomogram model and qRT-PCR experiments were used for verification. The results showed that there were 7 CRGs differentially expressed, and there were significant differences in immune cells such as Activated.dendritic.cell and Macrophage. Cluster C1 and Cluster C2 were obtained by unsupervised clustering, and three key module genes between clusters were obtained by WGCNA involving related pathways such as Ribosome and HIF-1 signaling pathway. Four candidate genes (SLC31A1, DBT, DLST, LIAS) were obtained from RF, Lasso, and SVM models, of which three were down-regulated, and one was up-regulated, and the SLC31A1 performance was the best (AUC = 0.958). The Nomogram model, ROC curve, and experimental results proved that the four candidate genes were highly reliable. Therefore, this study explored the association between SCI and cuproptosis based on the GEO dataset and obtained highly reliable prediction models and genes through a variety of machine learning methods, which is expected to provide new clinical evidence and support for potential therapeutic targets in SCI.
Keywords: spinal cord injury, cuproptosis, machine learning, predictive models, unsupervised clustering
Received: 28 Nov 2024; Accepted: 21 Mar 2025.
Copyright: © 2025 Zhou, Li, Wang, Ng, Rong, Liu, Liu, Fan, Mu and Zhou. This is an open-access article distributed under the terms of the Creative Commons Attribution License (CC BY). The use, distribution or reproduction in other forums is permitted, provided the original author(s) or licensor are credited and that the original publication in this journal is cited, in accordance with accepted academic practice. No use, distribution or reproduction is permitted which does not comply with these terms.
* Correspondence:
Yu Zhou, Postdoctoral research workstation, Chongqing Orthopedic Hospital of Traditional Chinese Medicine, Chongqing, China
Disclaimer: All claims expressed in this article are solely those of the authors and do not necessarily represent those of their affiliated organizations, or those of the publisher, the editors and the reviewers. Any product that may be evaluated in this article or claim that may be made by its manufacturer is not guaranteed or endorsed by the publisher.
Research integrity at Frontiers
Learn more about the work of our research integrity team to safeguard the quality of each article we publish.