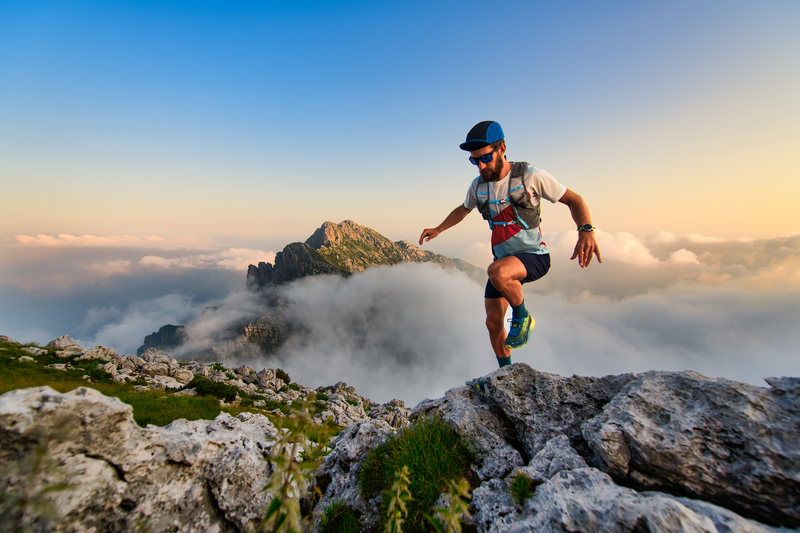
94% of researchers rate our articles as excellent or good
Learn more about the work of our research integrity team to safeguard the quality of each article we publish.
Find out more
ORIGINAL RESEARCH article
Front. Neurol.
Sec. Neuroepidemiology
Volume 16 - 2025 | doi: 10.3389/fneur.2025.1499564
This article is part of the Research Topic Quality of Stroke Care: What Could Be Improved, and How? - Volume II View all 9 articles
The final, formatted version of the article will be published soon.
You have multiple emails registered with Frontiers:
Please enter your email address:
If you already have an account, please login
You don't have a Frontiers account ? You can register here
[Abstract] Objective To investigate independent risk factors for unplanned readmission of ischemic stroke patients within 31 days in Wenzhou, and establish a nomogram model for risk prediction . Methods A total of 3035 patients with ischemic stroke were randomly grouped(in an 8:2 ratio) into 2428 training set and 607validation set. Independent sample t-test,pearson chisquare test ,fisher’s exact and multivariate logistic regression analysis were used to determine the factors associated with 31-day unplanned readmission in ischemic stroke,and the nomogram was established and validated. Results 669 patients (22.04%) had unplanned readmission. Atrial fibrillation , smoking,education of junior highschool and below , length of stay>16days, Hcy,and UA were independent risk factors for 31-day unplanned readmission in patients with ischemic stroke. The training set (AUC=0.883 (95%CI =0.867-0.899)) and validation set (AUC=0.817(95%CI=0.777-0.858)), and the calibration curve closely resembled the ideal curve, demonstrating good agreement between the predicted and actual values, it shows that the prediction model has a good degree of differentiation and calibration. At the same time, the decision curve shows that the model has a high clinical net benefit rate. Conclusion The nomograph model establishmented in this study to predict the risk of unplanned readmission of ischemic stroke patients within 31 days has good prediction ability.
Keywords: Unplanned readmission within 31 days, ischemic stroke, Risk model, Nomograph, Pediatrics
Received: 03 Oct 2024; Accepted: 11 Mar 2025.
Copyright: © 2025 Jin, Ma, Xianqiong and Ye. This is an open-access article distributed under the terms of the Creative Commons Attribution License (CC BY). The use, distribution or reproduction in other forums is permitted, provided the original author(s) or licensor are credited and that the original publication in this journal is cited, in accordance with accepted academic practice. No use, distribution or reproduction is permitted which does not comply with these terms.
* Correspondence:
Zhili Jin, Wenzhou, Wenzhou TCM Hosptial of Zhejiang Chinese Medical University, China
Disclaimer: All claims expressed in this article are solely those of the authors and do not necessarily represent those of their affiliated organizations, or those of the publisher, the editors and the reviewers. Any product that may be evaluated in this article or claim that may be made by its manufacturer is not guaranteed or endorsed by the publisher.
Research integrity at Frontiers
Learn more about the work of our research integrity team to safeguard the quality of each article we publish.