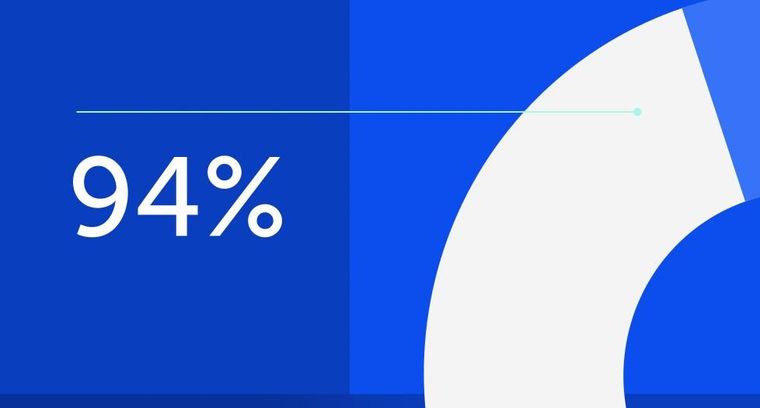
94% of researchers rate our articles as excellent or good
Learn more about the work of our research integrity team to safeguard the quality of each article we publish.
Find out more
ORIGINAL RESEARCH article
Front. Neurol., 27 March 2025
Sec. Stroke
Volume 16 - 2025 | https://doi.org/10.3389/fneur.2025.1489714
Background and purpose: Stroke is a leading cause of morbidity and mortality worldwide. Endovascular therapy (EVT) has been established as a gold standard option to treat acute ischemic stroke (AIS) patients with large vessel occlusion (LVO) presenting within 6 h of symptom onset. However, there is a paucity of information regarding patient outcome and mortality in patients presenting in late time window within 6 to 24 h. In this study, we aimed to assess for predictors of outcomes in late window stroke patients following EVT.
Methods: We analyzed data from 202 patients treated with EVT from four comprehensive stroke centers. All patients were above 18 years of age and had symptoms onset of 6–24 h. mRS of 0–2 after three months was defined as favorable outcome.
Results: Patients with favorable outcome had lower median age (p = 0.003), lower pre-EVT National Institute of Health Stroke Scale (NIHSS) score (p = 0.000), lower diabetes mellitus (p = 0.041), stroke history (p = 0.041), parenchymal hematoma (PH) (p = 0.000) and fewer attempts to achieve successful recanalization (p = 0.001). Multivariate regression analysis found age (p = 0.007), diabetes mellitus (p = 0.022), successful recanalization (mTICI≥2b) (p = 0.006), NIHSS at onset (p = 0.000), and PH1 + PH2 Heidelberg bleeding classification (p = 0.009) as predictors of functional outcome.
Conclusion: Age, diabetes mellitus history, baseline NIHSS score, successful recanalization, and PH are predictors of 90-day functional outcome of late-window ischemic stroke patients undergoing EVT.
Stroke is a major cause of disability worldwide with estimated 12.2 million annual incident cases and the second leading cause of death resulting in 6.55 million annual mortalities (1). Several landmark randomized clinical trial publications revealed endovascular therapy (EVT) as a safe and effective treatment which can be offered as a gold-standard for suitable acute ischemic stroke (AIS) patients with large vessel occlusion (LVO) presenting within 6 h of symptoms onset (2, 3). Significant randomized clinical trials, DEFUSE-3 and DAWN, published in 2018 which demonstrated that EVT is also highly effective in patients presented in late time window between 6 to 24 h after symptomatic onset (4, 5). Therefore, it is believed that EVT can be extended as a gold-standard treatment approach for patients presenting between 6 and 24 h as well. Recently, predicting the outcome of patients in stroke medicine has gained momentum to establish predictive models to facilitate the provision of personalized medicine (6, 7).
In this study, we aimed to predict the outcome and mortality of ischemic stroke patients presenting in late time window (6–24 h) who underwent EVT.
In this retrospective study, we enrolled prospectively collected data of consecutive AIS patients with LVO who underwent EVT at four comprehensive stroke centers in China. We derived our data from the Big Data Observatory Platform for stroke in China in addition to individual hospital data platforms.
Inclusion criteria for this study were as follows as established previously (8): (1) modified Rankin Scale (mRS) score before onset: 0–2; (2) interval from onset or the final onset-free time: 6–24 h; (3) age ≥ 18 years old, pre-EVT (National Institute of Health Stroke Scale) NIHSS score ≥ 6; (4) involved with internal carotid artery (ICA), tandem (ICA + M1), M1, M2, or basilar artery occlusion. Patients with missing follow-up and last know well to puncture time ≤ 6 h were excluded.
We collected the following data in our study: age, sex, risk factors of vascular diseases, such as hypertension, diabetes mellitus, coronary artery disease (CAD), atrial fibrillation (AF), dyslipidemia, previous stroke history, initial premorbid mRS, pre-EVT NIHSS, time metrics, site of arterial occlusion, modified thrombolysis in cerebral infarction (mTICI) post thrombectomy. Successful revascularization was defined as TICI 2b to 3 (8) and complete reperfusion was defined as mTICI of 3.
The patients’ outcomes were evaluated by using mRS 3-month after EVT was performed. Favorable outcome was defined as mRS of 0–2 after three months, as explained previously (9, 10).
The non-parametric Mann–Whitney U test was performed using IBM SPSS 26 version (IBM-Armonk, NY) to analyze non-normally distributed continuous data, reported as medians along with the interquartile range (IQR) in our study. Normally distributed data were reported as means with corresponding standard deviations (SD) and compared using the student’s t-test. Results were considered statistically significant if the p-value was less than 0.05.
The Institutional Review Board of all hospitals involved in the study approved the study protocol. All procedures in the study involving human participants were performed in accordance with the ethical standards of the institutional and/or national research committee and with the 1964 Declaration of Helsinki and its later amendments or comparable ethical standards. Written informed consents from the participants’ legal guardian/next of kin were obtained to perform the EVT.
We treated a total of 541 consecutive AIS patients. After applying the inclusion and exclusion criteria, a final cohort of 202 patients were included in the final analysis. In total, 322 patients were excluded due to last known normal-to-puncture-time (LKNPT) of within 6 h. Seven patients had occlusions which did not involve ICA, Tandem (ICA + M1), M1, M2, and basilar artery. Five patients were missed to follow-up and five other patients had pre-EVT NIHSS <6. A summary of patients’ selection criteria is demonstrated in Figure 1.
The baseline characteristics of patients are demonstrated in Table 1. Patients with favorable outcome had 7 years lower median age (p = 0.003) and had a median 4 points lower pre-EVT NIHSS (p = 0.000). In addition, the favorable outcome group had lower diabetes mellitus (p = 0.041), stroke history (p = 0.041), and PH1 + PH2 Heidelberg bleeding classification (p = 0.000). Furthermore, fewer attempts were required to achieve successful recanalization in the favorable outcome patient group (p = 0.001).
There were no statistically significant differences in sex (p = 0.659), hypertension (p = 0.294), coronary artery disease (p = 0.186), dyslipidemia (p = 0.483), pre-treatment Alberta stroke programme early CT score (ASPECTS) (p = 0.075), occlusion site (p = 0.299), intravenous thrombolysis (p = 0.713), LKNPT (p = 0.545) between the unfavorable and favorable outcome groups.
Our multivariate regression analysis showed that age (p = 0.007), diabetes mellitus (p = 0.022), successful recanalization (mTICI≥2b) (p = 0.006), NIHSS at onset (p = 0.000), and PH1 + PH2 Heidelberg bleeding classification (p = 0.009) (Table 2). In contrast, other factors such as atrial fibrillation (p = 0.500), previous stroke history (p = 0.216), initial pre-treatment ASPECTS (p = 0.120) were not associated with favorable outcome.
Despite significant advances in stroke care over the past decades, the prognosis of acute ischemic stroke patients has been a major challenge for clinicians worldwide, therefore establishing methods to predict functional outcome of patients undergoing EVT is of great clinical importance. In the present study, we aimed to predict the outcome and mortality of ischemic stroke patients from four comprehensive stroke centers presenting in late time window (6–24 h) who underwent EVT. Our data revealed that multiple factors, including age, diabetes mellitus history, successful recanalization (mTICI≥2b), NIHSS at onset, and PH1 + PH2 Heidelberg bleeding classification were associated with favorable outcome in patients with late-presentation who underwent EVT. However, atrial fibrillation, previous stroke history, and initial pre-treatment ASPECTS were not associated with favorable outcome.
Conducting multivariate regression analysis showed that only age, diabetes mellitus, successful recanalization (mTICI≥2b), NIHSS at onset, and PH1 + PH2 Heidelberg bleeding classification were factors involved in the prediction of favorable patient outcome. In comparison, our multivariate regression analysis did not show atrial fibrillation, previous stroke history, initial pre-treatment ASPECTS as factors predictive of favorable outcome. It is worth emphasizing that while ASPECTS was not associated with favorable outcome, initial pre-treatment ASPECTS was shown to be an important factor in our multivariate regression analysis.
A large multi-center prospective study of 1708 patients in France reported that patient age was strongly associated with 90-day functional outcome in patients treated with EVT with LKNPT fewer than 8 h, which is consistent with our findings (11). Our data is aligned with other published studies in European centers, disseminating such information from Asian centers can expand and validate the previously published studies.
Diabetes mellitus diagnosis was another factor in our multivariate regression analysis which was crucial for predicting favorable patient outcome. Similar to our results, a large multi-center European study of 695 patients revealed that diabetes mellitus diagnosis was an independent factor to predict poor functional outcome and mortality after 90 days in stroke patients (12). In addition to human investigations, multiple studies on animal have also reported that a larger infarct area was associated with elevated serum glucose level compared to animals with normal blood glucose (13, 14). Furthermore, some studies proposed multiple mechanisms to explain that more severe tissue damage was observed in AIS patients with diabetes mellitus, including intracellular acidosis, accumulation of extracellular glutamate, disruption to the blood–brain barrier, development of cerebral edema, and impairment of plasma fibrinolysis (15, 16).
Consistent with findings in our investigation, another prospective multi-center study also reported baseline NIHSS correlations with unfavorable functional outcomes in AIS patients treated EVT (17). NIHSS is a critical and well-established tool for determining the risk of clinical deterioration to assess the stroke severity to guide treatment decision-making, thereby selecting suitable treatment options, particularly before decision to initiate EVT (17).
Successful recanalization was another predictor of functional outcome revealed in our study. A recent large-scale European study comprised of 5,853 patients with AIS presenting in late time-window also reported that successful vessel recanalization was independently associated with a higher rate of favorable outcomes, defined as a mRS score of 3 or lower in their investigation (18).
Novel methods in future investigations are required to address the existing challenges in stroke treatment. Accurate stroke diagnosis and determining the exact time of onset are crucial components of comprehensive stroke management. However, a major challenge for clinicians remains predicting the outcomes for stroke patients, including radiological outcomes (e.g., hemorrhagic transformations, final infarct volume), morbidity (e.g., stroke-associated pneumonia), mortality, and other functional independence indices (e.g., mRS, cognitive functions, language functions, Barthel Index score) (19). Machine learning, a subfield of artificial intelligence, utilizes computerized algorithms that automatically improve performance through iterative learning or experience gained from data acquisition (19). As technological advances continue to transform medicine, machine learning has proven especially useful in establishing diagnoses, planning treatments, and predicting outcomes in patients with AIS (20–22). These approaches can analyze vast amounts of clinical and laboratory data within short time frames and with high accuracy, providing valuable insights into stroke research and treatment (23). Machine learning has been employed to identify suitable candidates for specific treatments or to predict patients likely to experience poor functional outcomes at 90 days (24, 25). Various machine learning approaches, including support vector machine (SVM), linear discriminant analysis (LDA), k-nearest neighbors (KNN), and decision tree algorithms have recently been employed in clinical research, paving the way for using large-scale data to improve clinical decision-making and patient prognosis (26–30). Therefore, establishment and utilization of a machine learning algorithm in our study can prompt other neurology and neurosurgery centers to adopt similar approaches for EVT.
A major advantage of our study is its multi-center nature across four large comprehensive stroke centers from an under-studied population in China. Our study has some limitations. First, this was a retrospective study with a medium sample size. We emphasize that prospective multi-center studies are required to validate these findings. Second, some factors, including final infarct volume, and arterial re-occlusion rates were not included in our analysis. In addition, predictive factors obtained in the study were not cross-validated, which is an important consideration for future investigations. Furthermore, we were unable to analyze data on Intracranial atherosclerotic disease (ICAD), intracranial stent or angioplasty use, as well as extracranial stent use. It is also worth emphasizing that we used the ASPECTS to analyze mainly, due to the limited data on computed tomographic perfusion (CPT) and magnetic resonance perfusion (MRP) to calculate the exact core volume in our study. A sensitivity analysis should be conducted in future studies by including the study center as a covariate in multivariate model. Our analysis showed no significant inter-center differences after adjusting for key clinical factors. Nevertheless, our results can prompt other stroke centers to investigate the outcome of patients presenting in late-window undergoing EVT.
Age, diabetes mellitus history, baseline NIHSS score, successful recanalization, and PH1 + PH2 Heidelberg bleeding classification are prognostic tools for the prediction of favorable outcomes in AIS patients presenting between 6 and 24 h of symptoms onset undergoing EVT. Future studies in other patient population and geographical areas are required to validate and extend these findings.
The raw data supporting the conclusions of this article will be made available by the authors, without undue reservation.
The studies involving humans were approved by the Institutional Review Board of First People’s Hospital of Foshan, The Affiliated Yuebei People’s Hospital of Shantou University Medical College, Oshan Sanshui District People’s Hospital, First People’s Hospital of Foshan. The studies were conducted in accordance with the local legislation and institutional requirements. The participants provided their written informed consent to participate in this study.
SZ: Data curation, Formal analysis, Investigation, Methodology, Validation, Visualization, Writing – original draft, Writing – review & editing. MM: Data curation, Project administration, Validation, Visualization, Writing – original draft, Writing – review & editing. ZC: Data curation, Formal analysis, Investigation, Validation, Visualization, Writing – review & editing. JT: Data curation, Formal analysis, Investigation, Writing – review & editing. JM: Data curation, Formal analysis, Investigation, Writing – review & editing. ZO: Data curation, Formal analysis, Investigation, Writing – review & editing. JW: Data curation, Formal analysis, Investigation, Writing – review & editing. SL: Data curation, Formal analysis, Investigation, Writing – review & editing. YH: Data curation, Formal analysis, Investigation, Writing – review & editing. ZL: Data curation, Formal analysis, Visualization, Writing – review & editing. YL: Data curation, Formal analysis, Investigation, Writing – review & editing. XL: Conceptualization, Data curation, Formal Analysis, Investigation, Methodology, Resources, Supervision, Validation, Visualization, Writing – original draft.
The author(s) declare that no financial support was received for the research and/or publication of this article.
We would like to thank to all our colleagues for data collection and patients for their contribution.
The authors declare that the research was conducted in the absence of any commercial or financial relationships that could be construed as a potential conflict of interest.
All claims expressed in this article are solely those of the authors and do not necessarily represent those of their affiliated organizations, or those of the publisher, the editors and the reviewers. Any product that may be evaluated in this article, or claim that may be made by its manufacturer, is not guaranteed or endorsed by the publisher.
1. Feigin, VL, Stark, BA, Johnson, CO, Roth, GA, Bisignano, C, Abady, GG, et al. Global, regional, and national burden of stroke and its risk factors, 1990-2019: a systematic analysis for the global burden of disease study 2019. Lancet Neurol. (2021) 20:795–820. doi: 10.1016/S1474-4422(21)00252-0
2. Goyal, M, Menon, BK, van Zwam, WH, Dippel, DW, Mitchell, PJ, Demchuk, AM, et al. Endovascular thrombectomy after large-vessel ischaemic stroke: a meta-analysis of individual patient data from five randomised trials. Lancet. (2016) 387:1723–31. doi: 10.1016/S0140-6736(16)00163-X
3. Kobeissi, H, Ghozy, S, Adusumilli, G, Kadirvel, R, Brinjikji, W, Rabinstein, AA, et al. Endovascular therapy for stroke presenting beyond 24 hours: a systematic review and Meta-analysis. JAMA Netw Open. (2023) 6:e2311768. doi: 10.1001/jamanetworkopen.2023.11768
4. Albers, GW, Marks, MP, Kemp, S, Christensen, S, Tsai, JP, Ortega-Gutierrez, S, et al. Thrombectomy for stroke at 6 to 16 hours with selection by perfusion imaging. N Engl J Med. (2018) 378:708–18. doi: 10.1056/NEJMoa1713973
5. Nogueira, RG, Jadhav, AP, Haussen, DC, Bonafe, A, Budzik, RF, Bhuva, P, et al. Thrombectomy 6 to 24 hours after stroke with a mismatch between deficit and infarct. N Engl J Med. (2018) 378:11–21. doi: 10.1056/NEJMoa1706442
6. Rajashekar, D, Hill, MD, Demchuk, AM, Goyal, M, Fiehler, J, and Forkert, ND. Prediction of clinical outcomes in acute Ischaemic stroke patients: a comparative study. Front Neurol. (2021) 12:663899. (In eng). doi: 10.3389/fneur.2021.663899
7. Goyal, M, Ospel, JM, Kappelhof, M, and Ganesh, A. Challenges of outcome prediction for acute stroke treatment decisions. Stroke. (2021) 52:1921–8. doi: 10.1161/STROKEAHA.120.033785
8. Chen, Y, Zhou, S, Yang, S, Mofatteh, M, Hu, Y, Wei, H, et al. Developing and predicting of early mortality after endovascular thrombectomy in patients with acute ischemic stroke. Front Neurosci. (2022) 16:1034472. doi: 10.3389/fnins.2022.1034472
9. Lai, Y, Diana, F, Mofatteh, M, Nguyen, TN, Jou, E, Zhou, S, et al. Predictors of failure of early neurological improvement in early time window following endovascular thrombectomy: a multi-center study. Front Neurol. (2023) 14:1227825. doi: 10.3389/fneur.2023.1227825
10. Ma, J, Chervak, LM, Siegler, JE, Li, Z, Mofatteh, M, Galecio-Castillo, M, et al. Postinterventional petechial hemorrhage associated with poor functional outcome after successful recanalization following endovascular therapy. Neurosurgery. (2024) 96:438–46. doi: 10.1227/neu.0000000000003098
11. Finitsis, S, Epstein, J, Richard, S, Bourcier, R, Sibon, I, Dargazanli, C, et al. Age and outcome after endovascular treatment in anterior circulation large-vessel occlusion stroke: ETIS registry results. Cerebrovasc Dis. (2020) 50:68–77. doi: 10.1159/000512203
12. Kalmar, PJ, Tarkanyi, G, Karadi, ZN, Szapary, L, and Bosnyak, E. The impact of diabetes mellitus and admission hyperglycemia on clinical outcomes after recanalization therapies for acute ischemic stroke: Stay Alive National Prospective Registry. Life. (2022) 12:632. doi: 10.3390/life12050632
13. Kawai, N, Keep, RF, Betz, AL, and Nagao, S. Hyperglycemia induces progressive changes in the cerebral microvasculature and blood-brain barrier transport during focal cerebral ischemia. Acta Neurochir Suppl. (1998) 71:219–21. doi: 10.1007/978-3-7091-6475-4_63
14. Quast, MJ, Wei, J, Huang, NC, Brunder, DG, Sell, SL, Gonzalez, JM, et al. Perfusion deficit parallels exacerbation of cerebral ischemia/reperfusion injury in hyperglycemic rats. J Cereb Blood Flow Metab. (1997) 17:553–9. doi: 10.1097/00004647-199705000-00009
15. Ferrari, F, Moretti, A, and Villa, RF. Hyperglycemia in acute ischemic stroke: physiopathological and therapeutic complexity. Neural Regen Res. (2022) 17:292–9. doi: 10.4103/1673-5374.317959
16. Kruyt, ND, Biessels, GJ, Devries, JH, and Roos, YB. Hyperglycemia in acute ischemic stroke: pathophysiology and clinical management. Nat Rev Neurol. (2010) 6:145–55. doi: 10.1038/nrneurol.2009.231
17. Zhang, L, Ma, J, Wang, M, Zhang, L, Sun, W, Ji, H, et al. The association between National Institutes of Health stroke scale score and clinical outcome in patients with large Core infarctions undergoing endovascular treatment. Neurol Ther. (2024) 13:563–81. doi: 10.1007/s40120-024-00588-8
18. Broocks, G, Hanning, U, Bechstein, M, Elsayed, S, Faizy, TD, Brekenfeld, C, et al. Association of Thrombectomy with Functional Outcome for patients with ischemic stroke who presented in the extended time window with extensive signs of infarction. JAMA Netw Open. (2022) 5:e2235733. doi: 10.1001/jamanetworkopen.2022.35733
19. Mainali, S, Darsie, ME, and Smetana, KS. Machine learning in action: stroke diagnosis and outcome prediction. Front Neurol. (2021) 12:734345. doi: 10.3389/fneur.2021.734345
20. Chen, SD, You, J, Yang, XM, Gu, HQ, Huang, XY, Liu, H, et al. Machine learning is an effective method to predict the 90-day prognosis of patients with transient ischemic attack and minor stroke. BMC Med Res Methodol. (2022) 22:195. doi: 10.1186/s12874-022-01672-z
21. Abujaber, AA, Alkhawaldeh, IM, Imam, Y, Nashwan, AJ, Akhtar, N, Own, A, et al. Predicting 90-day prognosis for patients with stroke: a machine learning approach. Front Neurol. (2023) 14:1270767. doi: 10.3389/fneur.2023.1270767
22. Teo, YH, Lim, I, Tseng, FS, Teo, YN, Kow, CS, Ng, ZHC, et al. Predicting clinical outcomes in acute ischemic stroke patients undergoing endovascular Thrombectomy with machine learning: a systematic review and Meta-analysis. Clin Neuroradiol. (2021) 31:1121–30. doi: 10.1007/s00062-020-00990-3
23. Hassan, A, Gulzar Ahmad, S, Ullah Munir, E, Ali Khan, I, and Ramzan, N. Predictive modelling and identification of key risk factors for stroke using machine learning. Sci Rep. (2024) 14:11498. doi: 10.1038/s41598-024-61665-4
24. Quandt, F, Flottmann, F, Madai, VI, Alegiani, A, Küpper, C, Kellert, L, et al. Machine learning-based identification of target groups for Thrombectomy in acute stroke. Transl Stroke Res. (2023) 14:311–21. doi: 10.1007/s12975-022-01040-5
25. Chiu, IM, Zeng, WH, Cheng, CY, Chen, SH, and Lin, CR. Using a multiclass machine learning model to predict the outcome of acute ischemic stroke requiring reperfusion therapy. Diagnostics. (2021) 11:80. doi: 10.3390/diagnostics11010080
26. Cheng, WC, Chen, LH, Jiang, CR, Deng, YM, Wang, DW, Lin, CH, et al. Sensible functional linear discriminant analysis effectively discriminates enhanced Raman spectra of Mycobacterium species. Anal Chem. (2021) 93:2785–92. doi: 10.1021/acs.analchem.0c03681
27. Huang, S, Cai, N, Pacheco, PP, Narrandes, S, Wang, Y, and Xu, W. Applications of support vector machine (SVM) learning in Cancer genomics. Cancer Genomics Proteomics. (2018) 15:41–51. doi: 10.21873/cgp.20063
28. Saçlı, B, Aydınalp, C, Cansız, G, Joof, S, Yilmaz, T, Çayören, M, et al. Microwave dielectric property based classification of renal calculi: application of a kNN algorithm. Comput Biol Med. (2019) 112:103366. doi: 10.1016/j.compbiomed.2019.103366
29. Mofatteh, M. Neurosurgery and artificial intelligence. AIMS Neurosci. (2021) 8:477–95. doi: 10.3934/Neuroscience.2021025
Keywords: ischemic stroke, large vessel occlusion, endovascular therapy, thrombectomy, patient outcome, late time window used machine learning
Citation: Zhou S, Mofatteh M, Chen Z, Tang J, Ma J, Ouyang Z, Wang J, Luo S, He Y, Li Z, Lai Y and Liao X (2025) Predictive outcome of late window ischemic stroke patients following endovascular therapy: a multi-center study. Front. Neurol. 16:1489714. doi: 10.3389/fneur.2025.1489714
Received: 01 September 2024; Accepted: 12 March 2025;
Published: 27 March 2025.
Edited by:
Luis Rafael Moscote-Salazar, Colombian Clinical Research Group in Neurocritical Care, ColombiaReviewed by:
Luis Alberto Camputaro, Specialized Institute “Hospital El Salvador”, El SalvadorCopyright © 2025 Zhou, Mofatteh, Chen, Tang, Ma, Ouyang, Wang, Luo, He, Li, Lai and Liao. This is an open-access article distributed under the terms of the Creative Commons Attribution License (CC BY). The use, distribution or reproduction in other forums is permitted, provided the original author(s) and the copyright owner(s) are credited and that the original publication in this journal is cited, in accordance with accepted academic practice. No use, distribution or reproduction is permitted which does not comply with these terms.
*Correspondence: Xuxing Liao, ZHJsaWFvMjEwNDA5QDE2My5jb20=
†These authors have contributed equally to this work
Disclaimer: All claims expressed in this article are solely those of the authors and do not necessarily represent those of their affiliated organizations, or those of the publisher, the editors and the reviewers. Any product that may be evaluated in this article or claim that may be made by its manufacturer is not guaranteed or endorsed by the publisher.
Research integrity at Frontiers
Learn more about the work of our research integrity team to safeguard the quality of each article we publish.