- 1Department of Radiology, The Second Affiliated Hospital, Jiangxi Medical College, Nanchang University, Nanchang, China
- 2Jiangxi Provincial Key Laboratory of Intelligent Medical Imaging, Nanchang, China
Background: Alzheimer’s disease (AD) is a common neurodegenerative disorder worldwide and the using of magnetic resonance imaging (MRI) in the management of AD is increasing. The present study aims to summarize MRI in AD researches via bibliometric analysis and predict future research hotspots.
Methods: We searched for records related to MRI studies in AD patients from 2004 to 2023 in the Web of Science Core Collection (WoSCC) database. CiteSpace was applied to analyze institutions, references and keywords. VOSviewer was used for the analysis of countries, authors and journals.
Results: A total of 13,659 articles were obtained in this study. The number of published articles showed overall exponential growth from 2004 to 2023. The top country and institution were the United States and the University of California System, accounting for 40.30% and 9.88% of the total studies, respectively. Jack CR from the United States was the most productive author. The most productive journal was the Journal of Alzheimers Disease. Keyword burst analysis revealed that “machine learning” and “deep learning” were the keywords that frequently appeared in the past 6 years. Timeline views of the references revealed that “#0 tau pathology” and “#1 deep learning” are currently the latest research focuses.
Conclusion: This study provides an in-depth overview of publications on MRI studies in AD. The United States is the leading country in this field with a concentration of highly productive researchers and high-level institutions. The current research hotspot is deep learning, which is being applied to develop noninvasive diagnosis and safer treatment of AD.
1 Introduction
Alzheimer’s disease (AD) is the most common neurodegenerative disease in elderly individuals and can cause progressive memory loss and cognitive impairment (1). It is considered a serious problem for both individual health and government healthcare systems worldwide (2). AD is characterized by the accumulation of β-amyloid (Aβ) plaques and neurofibrillary tangles (NFTs) composed of hyperphosphorylated tau protein in the brain, which impair neuronal function and communication (3, 4). The advent of new anti-amyloid monoclonal antibodies such as aducanemab, lecanemab, and donanemab as treatment for early AD, may slow disease progression but also pose significant risks such as amyloid related imaging abnormalities (ARIA) (5–8). Although many studies have explored the pathogenesis, diagnostics and treatment of AD, the underlying mechanisms are currently not well understood.
In recent years, with the rapid development of medical imaging technologies, magnetic resonance imaging (MRI) has been widely applied to investigate the pathological features of AD, which provides a new perspective for better understanding the pathogenesis of AD. Structural MRI-based measures of medial temporal lobe atrophy are regarded as valid markers in clinical AD diagnosis (9–11). Researchers have used MRI as a unique and noninvasive tool to monitor cortical gray matter loss (12–14), white matter lesions (15), and small vessel abnormalities (16) longitudinally in AD patients. Then multimodal imaging techniques, including MRI and positron emission computed tomography (PET) technology, are also widely used in the early diagnosis and prognosis prediction of AD clinically, and researchers aim to provide important imaging evidence from the aspects of structure (9, 10, 17, 18), function (19–23), metabolism (24), and biomarkers (1, 25–27). Moreover, with rapid advancements in high-field MRI in small animals, many studies have been conducted to explore the underlying mechanisms and drug development of AD in animal models using high-field MRI (22, 28–31). Although these MRI studies have enhanced our understanding of the imaging features and underlying mechanisms of AD, little attention has been given to the current research status, hotspots, and frontier trends in this field (32).
In recent years, bibliometric analysis has been widely used to explore the literature in specific research fields, which can quantitatively analyze and visualize the literature data and measure characteristics through various bibliometric tools (33–35), thus helping researchers quickly and accurately understand the research status, hotspots, and trends of this topic in the field. In this study, we conducted a bibliometric analysis of publications related to MRI studies in AD in the Web of Science Core Collection (WoSCC) between 2004 and 2023. This study aimed to explore the research status, hotspots, and frontier trends of MRI studies in AD over the past 20 decades, which may help new researchers better grasp future research interest.
2 Methods
2.1 Database
The WoSCC database was chosen as the data source. It is the most frequently used and acceptable database for researchers in a variety of fields.
2.2 Search strategy
We searched for publications about MRI in the field of AD on August 26, 2024. The search query string was as follows: TS = (Alzheimer’s disease OR Alzheimer disease OR Alzheimer) AND (magnetic resonance imaging OR MRI OR T1WI OR T2WI OR DWI OR diffusion-weighted imaging OR ESWAN OR enhanced gradient echo T2 star weighted angiography OR SWI OR susceptibility weighted imaging OR MRS OR magnetic resonance spectroscopy OR ASL OR arterial spin labeling OR DCE OR dynamic contrast-enhanced OR PWI OR perfusion weighted imaging OR BOLD-fMRI OR blood oxygenation level-dependent functional magnetic resonance imaging OR DTI OR diffusion tensor imaging OR DKI OR diffusion kurtosis imaging OR IVIM OR intravoxel incoherent motion OR CEST OR chemical exchange saturation transfer OR APT OR amide proton transfer OR MPRAGE OR three-dimensional T1-weighted magnetization-prepared rapid gradient echo OR QSM OR quantitative susceptibility mapping OR rs-fMRI OR resting-state functional MRI OR fMRI OR functional magnetic resonance imaging OR VBM OR voxel-based morphology OR multimodal imaging technique OR volumetric MRI OR structural magnetic resonance imaging) AND (FPY = 2004–2023). The language was limited to English, and the document types were limited to articles and review articles. Information regarding titles, keywords, abstracts, authors, institutions and reference records of the papers was downloaded and saved in plain text format. The study flowchart is shown in Figure 1.
2.3 Data analysis and visualization
Microsoft Excel 2017 (Microsoft, Redmond, WA, United States) was used to display the global trend of publications and analyze the targeted files export statistical charts and tables of the most productive countries/regions, institutions, authors and journals.
VOSviewer (version 1.6.20) and CiteSpace (version 6.3. R1) are two widely used software programs for constructing and visualizing bibliometric networks (33, 36). CiteSpace was applied to analyze institutions, reference clusters and keyword bursts. VOSviewer was used for the analysis of countries/regions, authors and journals. The node in each map represents a country, institution or reference. The size of the node (country, institution or reference) represents the number of publications. The larger the node, the greater is the number of publications. The links between the nodes represent the strength of collaborations.
3 Results
3.1 Global trends in publications
A total of 13,659 articles were included in the subsequent analyzes. The number of articles published each year and the cumulative number of articles published are shown in Figure 2, which shows a consistent overall upward trend in the number of annual publications from 2004 to 2022. The fastest annual growth was in 2020, with an increase of 138 articles. The statistical graph is adjusted to fit a linear curve that follows the following equation: the fitted curve index is y = 700.62x – 2230, with a correlation coefficient of 0.9511. In addition, the number of articles published in 2023 decreased from 1,335 in 2022 to 1,276, which may be caused by research bias in that it takes time for articles to reach a certain number of citations after publication.
3.2 Analysis of countries/regions and institutions
A total of 108 countries/regions published related articles in this field, 57 of which published no fewer than 10 articles. Table 1 shows the top 10 most productive countries/regions. The United States ranked first with 5,504 articles, accounting for 40.30% of the total number of articles published, followed by China (2,150, 15.74%), England (1,782, 13.05%), and Germany (1,192, 8.73%).
The H-index is a mixed index that is used as a significant indicator of the number and level of academic output of a scientific researcher, country, journal, or institution (37). The country with the highest H-index was the United States, followed by England, Germany and the Netherlands. A cooperative network of countries and their collaborations via VOSviewer is shown in Figure 3.
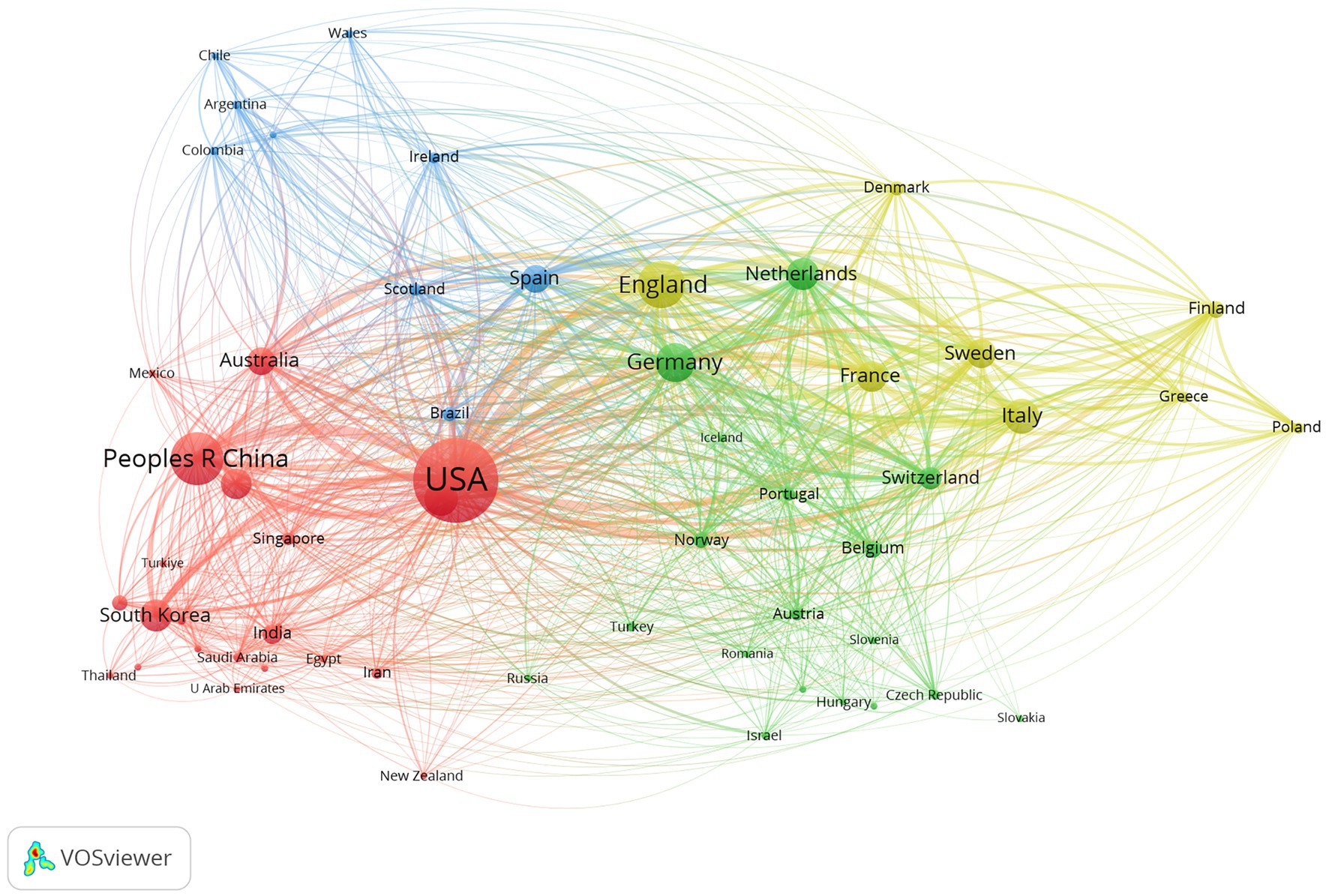
Figure 3. Country/region citation network visualization map generated via VOSviewer. The node represents a country/region, the size of the node represents the publication counts of a country/region, and the lines between nodes represent the strength of collaborations.
In addition, 9,233 institutions published articles in this field, of which 1,491 institutions had no fewer than 5 articles. Table 2 summarizes the top 10 institutions and their partnerships. The University of California System published the most, with 1,350 articles, accounting for 9.88%, followed by the University of London (854, 6.25%), Harvard University (738, 5.40%), and Mayo Clinic (625, 4.58%). The cooperative network of institutions and their collaborations via CiteSpace are shown in Figure 4.
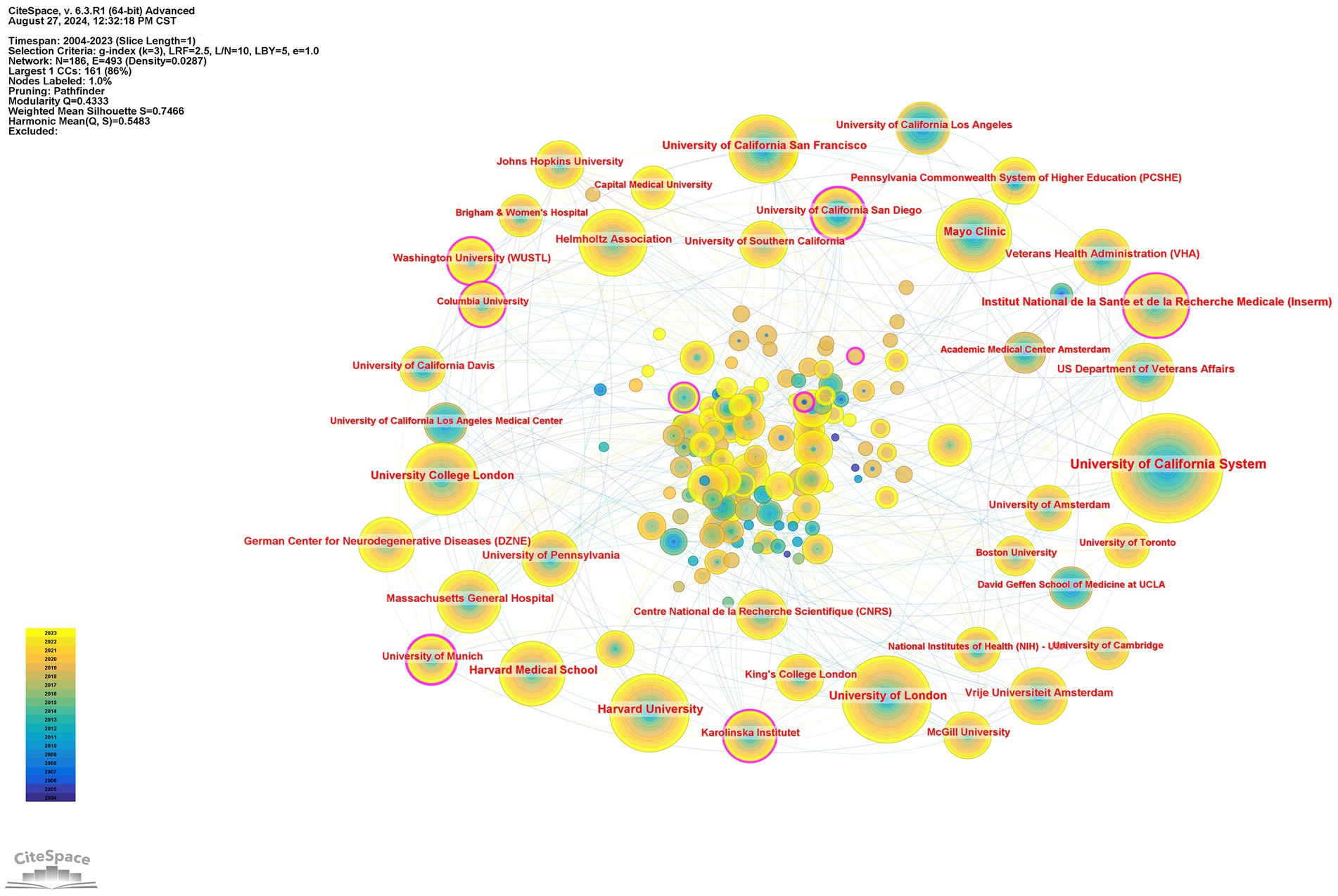
Figure 4. Institutions’ citation network visualization map generated via CiteSpace. The node represents an institution, the size of the node represents the publication counts of an institution, and the lines between nodes represent the strength of collaborations. The color of circles and the links between them reflect the occurrence time. The brighter they are, the more recently they occurred.
3.3 Analysis of authors
A total of 48,736 authors were included in this study, 3,784 of whom published no fewer than 5 articles. Table 3 summarizes the top 10 most productive authors. Half of the authors were from the United States. Among them, Jack CR, Scheltens P, and Petersen RC were the top 3 productive authors, with 413, 240, and 224 articles, respectively.
3.4 Analysis of journals
A total of 1,326 journals published related articles in this field, of which 367 authors published no fewer than 5 articles. The top 15 most prolific journals are listed in Table 4. Among the top 15 journals, one in three were from the United States. In addition, the journal with the highest IF was Brain among the top 15 journals. Journal of Alzheimers Disease [impact factor (IF) 2023: 3.4] published the most (1,175 publications), followed by Neurobiology of Aging (IF 2023: 3.7, 592 publications), and Neuroimage (IF 2023: 4.7, 561 publications). The top 15 journals published 5,460 articles, accounting for 40.12% of the total number of publications. These journals have made great contributions to the development of MRI studies in AD, indicating that more high-quality articles in this field will be published in these journals as a priority in the future.
3.5 Analysis of hotspots
3.5.1 Analysis of keywords
Keywords are the central ideas of an article. The research hotspots in MRI research on AD have been investigated mainly through keyword burst detection. The top 25 keywords according to CiteSpace are presented in Figure 5 and are sorted by the initial year of the burst. As shown in the diagram, “medial temporal lobe,” “entorhinal cortex,” “temporal lobe atrophy,” “gray matter loss,” “white matter lesions” and “hippocampal volume” had the longest burst durations (8–9 years), indicating the importance of these lesion locations for research in this field. “Deep learning,” “gray matter loss,” “entorhinal cortex,” and “vascular dementia” had the highest burst intensities, with burst strengths of 77.32, 75.55, 72.09, and 53.62, respectively. Moreover, “subjective cognitive decline,” “machine learning,” “convolutional neural networks” and “deep learning” appeared most frequently in the past 6 years, and they all lasted to the present, indicating that these are current and future research hotspots.
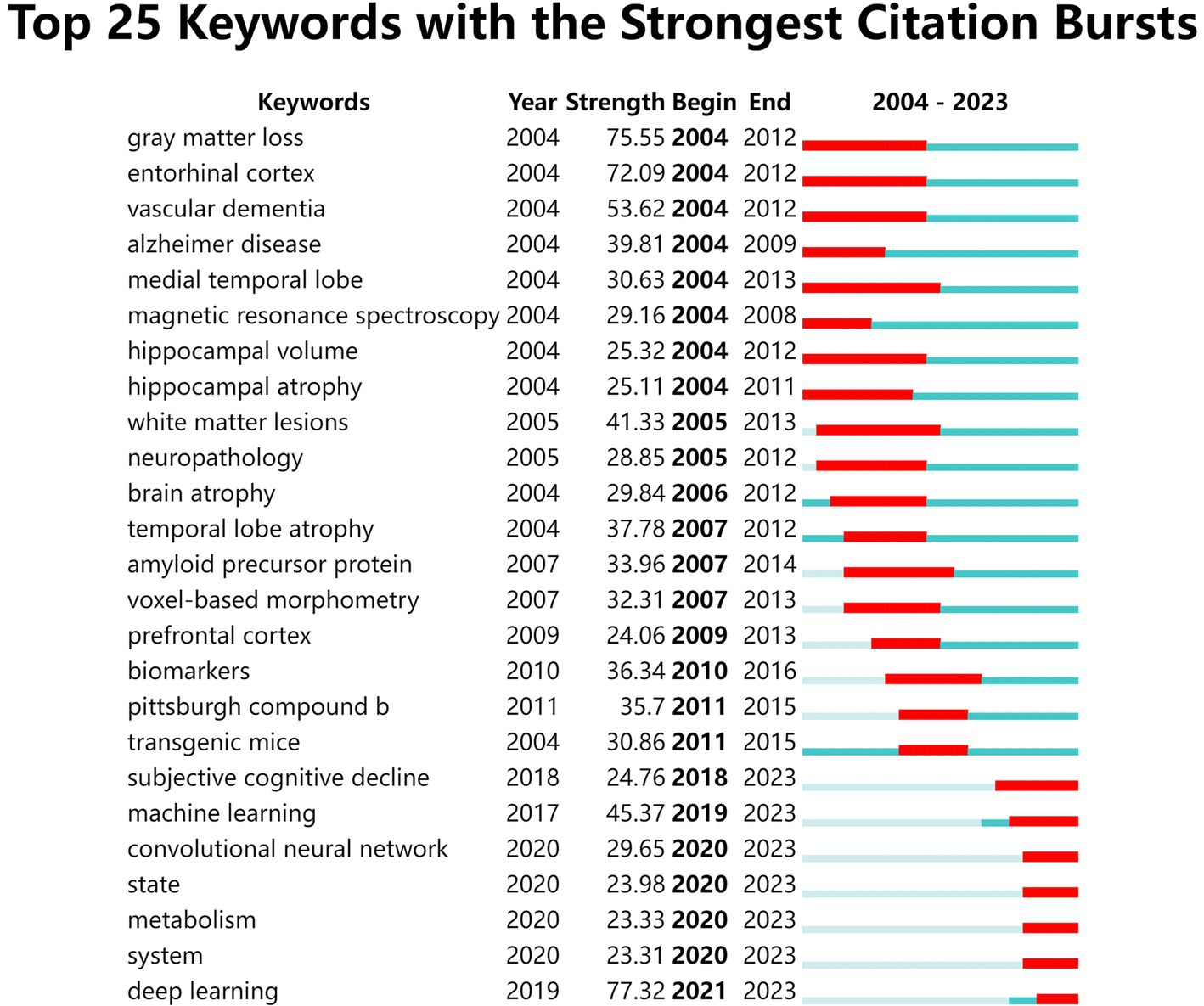
Figure 5. The top 25 keywords with the strongest citation bursts from 2004 to 2023 according to CiteSpace. The blue line represents the time period, and the red line represents the time span of the burst.
3.5.2 Reference analysis
Reference analysis uses reference as the element of analysis to reflect the relationship between the references by analyzing patterns and trends in citations. CiteSpace software was used to build reference clustering. Each cluster was considered to represent a research focus.
From the analysis results, the modularity Q was 0.781, and the mean silhouette S was as high as 0.9202, indicating an excellent clustering effect and good network homogeneity.
Table 5 and Figure 6 present the 12 main clusters and their respective first five feature words. According to the clustering results, there were 12 clusters as follows: “#0 voxel-based morphometry,” “#1 deep learning,” “#2 tau pathology,” “#3 hippocampal atrophy,” “#4 white matter integrity,” “#5 resting state,” “#6 amyloid load,” “#7 arterial spin,” “#8 random forest algorithm,” “#9 hippocampal activation,” “#10 plasma biomarker,” and “#11 white matter hyperintensities.”
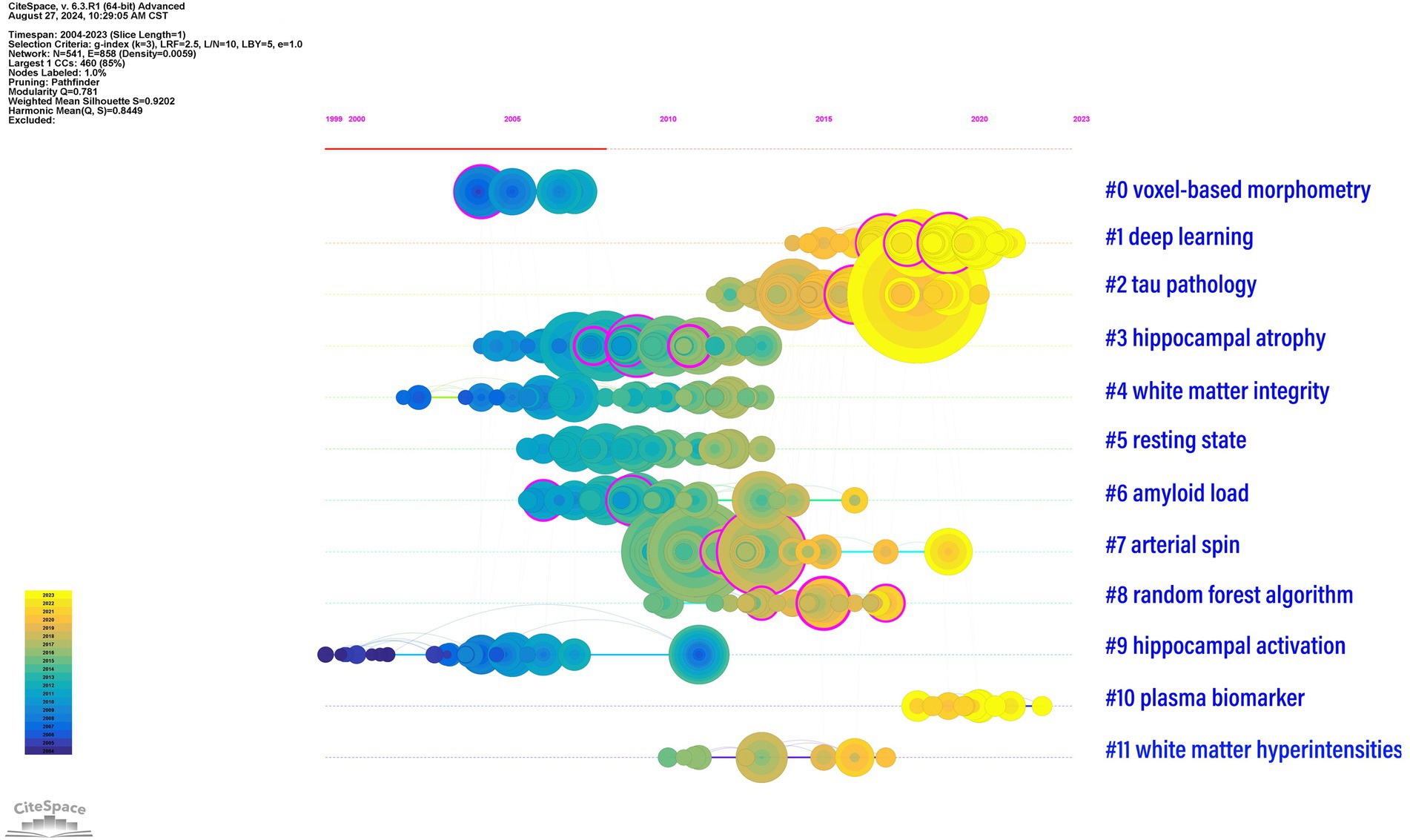
Figure 6. Timeline graph of reference clusters by CiteSpace. The node represents a reference, and the size of each node is associated with the number of references. Each line represents a cluster, and the numbers 0–11 refer to the top 12 clusters which represent 12 different research directions. The emergence time point and time span of all clusters are shown. Colors indicate the occurrence time: the brighter they are, the more recently they occurred.
As shown in Table 5, cluster #0 contained 69 keywords with the first five feature words of voxel-based morphometry, which included voxel-based morphometry, the whole-brain atrophy rate, volumetric MRI, and the cingulate gyrus. Cluster #1 included 60 keywords, and the first five keywords were deep learning, convolutional neural network, artificial intelligence, neural network, and structural MRI. Cluster #2 included 59 keywords, and the first five features were tau pathology, tau PET, tau accumulation, tau deposition, and subjective cognitive decline. Cluster #3 consisted of 59 keywords with the first five feature words of hippocampal atrophy, the ADNI cohort, tensor-based morphometry, multitask learning, and automated 3D mapping. Cluster #4 had 40 keywords, and the first five words were white matter integrity, white matter degeneration, white matter microstructure, uncinate fasciculus, and white matter disruption. Cluster #5 had 32 keywords, and the first five words were resting state, default mode network, default mode network, functional connectivity, and functional connection. Cluster #6 involved 31 keywords, with the first five feature words being amyloid load, 42 measure, transforming cerebrospinal fluid, calculated Pittsburgh compound B unit, and dynamic biomarker. Cluster #7 had 30 keywords, and the first five feature words extracted were arterial spin, diagnostic criteria, cerebrospinal fluid, prospective cohort study, and cerebral blood flow. Cluster #8 contained 30 keywords, with the first five words being the random forest algorithm, feature extraction method, grading biomarker, major brain diseases, and 5-year trend. Cluster #9 had 29 keywords, and the first five words were related to hippocampal activation, functional MRI studies, parietal deactivation, functional alteration, and genetic risk. Cluster #10 included 12 keywords, and the first five features were plasma biomarkers, dementia risk, prognostic capabilities, diagnosing dementia, and tau PET tracers. Cluster #11 involved 9 keywords, with the first five feature words being white matter hyperintensities, white matter lesions, cerebral amyloid angiopathy, age-associated aggregation, and at-risk.
In Figure 6, the above 12 clusters are displayed according to the reference time. Each line represents a cluster, where the nodes represent a reference, and the size of each node is associated with the number of references. Therefore, a timeline view of the references visually presents the phased hotspots of MRI studies in AD from the time dimension. As shown in Figure 6, “#0 voxel-based morphometry” and “#9 hippocampal activation” were the earliest studies in this field. “#0 tau pathology” and “#1 deep learning” are currently the latest research hotspots, suggesting that an increasing number of researchers are paying attention to the application of deep learning and tau pathology in MRI studies of AD.
4 Discussion
This study investigated the global trend of publications by authors from different countries and institutions, as well as the references and keywords in MRI studies on AD through bibliometric analysis from 2004 to 2023. The increasing number of annual publications suggests that an increasing number of researchers are paying attention to this field. It can be expected that the number of publications in this field will continue to remain high over the next few years. The most productive country was the United States. Jack CR from the United States was the most productive author. The most productive journal was the Journal of Alzheimers Disease, and the 15 journal with the highest IF was Brain. According to hotspot analysis, the period of the last 20 years can be roughly divided into 3 stages with different research hotspots, which sequentially evolved from (I) exploring the morphological changes in the AD brain by structural MRI (sMRI) to (II) identifying different biomarkers of AD and exploring the functional changes via functional MRI (fMRI) in AD patients and finally to (III) artificial intelligence (AI) applications.
In the current bibliographic study, CiteSpace software was used to construct a timeline graph of reference clusters and explore keyword bursts. According to the reference clustering results and keyword burst analysis, the evolution of hotspots in MRI studies of AD from 2004 to 2023 can be divided into three stages: stage I (2004–2010), stage II (2010–2018), and stage III (2018–2023).
4.1 Stage I
Stage I focused on the study of morphological changes in the AD brain via sMRI. The main keywords were associated with gray matter loss, the entorhinal cortex, temporal lobe atrophy, hippocampal atrophy, brain atrophy, and white matter lesions.
sMRI is widely used to explore the underlying pathophysiology of AD (38). Brain atrophy detected by sMRI is a valid biomarker of the stage and intensity of AD pathology (9, 10, 39). Structural changes in the brain map accurately upstream to Braak stages of NFT deposition (4, 40, 41) and downstream of neuropsychological deficits (42, 43). Considerable evidence suggests that AD initiates in the entorhinal cortex and hippocampus and spreads thereafter to the rest of the brain. The earliest sites of tau deposition and MRI-based atrophy are located along the perforant (polysynaptic) hippocampal pathway (entorhinal cortex, hippocampus and posterior cingulate cortex), which is consistent with early memory deficits (9, 44, 45). Progressive atrophy in the temporal, parietal and frontal neocortex is closely related to neuronal loss, as well as language, praxic, visuospatial and behavioral impairments (4, 17, 46, 47).
Several different processing methods, such as voxel-based morphometry (VBM) (48), boundary shift integral (BSI) (4, 49), and tensor-based morphometry (TBM) (45, 50), are employed to detect subtle changes and assess atrophy in the brain MR images of the same individual or groupwise comparisons. VBM studies have revealed that regional gray matter atrophy lies mainly in the bilateral mesial temporal lobes, including the hippocampus, amygdala, entorhinal cortex, and posterior cingulum, and extends to the frontal and parietal lobes with the progression of AD (51–53). VBM studies have also revealed white matter abnormalities in patients with AD compared with healthy controls (54–59). Nevertheless, structural neuroimaging studies of white matter volume in AD patients have yielded variable findings owing to heterogeneous subjects or small sample sizes, as well as methodological differences among studies. For example, two studies (56, 57) reported white matter volume reduction only in bilateral temporal structures, whereas other studies (54, 55, 58, 59) reported widespread white matter volume abnormalities beyond the temporal lobe. Li reported that white matter atrophy in AD patients occurred mainly in bilateral structures close to memory formations, such as the hippocampus, amygdala, and entorhinal cortex, through meta-analysis (60).
4.2 Stage II
Stage II focused on the study of biomarkers and fMRI in AD, and the main keywords were biomarkers, transgenic mice, and Pittsburgh compound b.
The major biomarkers of AD that are typically considered for clinical trials and observational studies are cerebrospinal fluid (CSF) Aβ1-42, CSF total-tau (t-tau), fluoro-deoxy-glucose positron emission tomography (FDG–PET), Pittsburgh compound B-PET (PIB–PET), and sMRI. As suggested by Wahlund and Blennow (61, 62), CSF tau, p-tau, and sMRI may reflect the disease stage or intensity of AD, whereas CSF Aβ represents a specific molecular pathway or etiology. These main CSF biomarkers have high diagnostic accuracy for identifying prodromal AD in the mild cognitive impairment (MCI) stage, with a sensitivity and specificity of 85–90% (25, 26). However, variability in measurements between clinical laboratories has hindered the identification of a unified critical value for CSF biomarkers because of differences in analytical procedures for manual ELISA methods between different laboratories, as well as variability in reagent quality and manufacturing procedures resulting in batch-to-batch variations (63). Research based on Alzheimer’s Disease Neuroimaging Initiative (ADNI) data has shown that sMRI is more closely related to cognition than CSF biomarkers are (64, 65).
In earlier stages of AD, such as MCI or preclinical AD, atrophy can be minimal, although metabolic imaging (FDG PET) findings may already be abnormal (66, 67). Although there are many amyloid imaging PET tracers based on 11C and 18F, the most studied tracer in the field of AD is PIB (68). C-pPIB can be used as a powerful biomarker of rCBF to measure neural activity and improve the diagnostic ability of PET for AD in conjunction with [11C]-aPIB (69). Tiepolt (70) reported that early [18F]FBB and [11C]PiB PET brain images are analogous to [18F]FDG PET images of the AD brain and that these tracers could be used as potential biomarkers in place of [18F]FDG. Furthermore, [11C]-PIB R (1) could serve as a complementary biomarker of neuronal activity and neurodegeneration in addition to the Aβ load given by [11C]-PIB BP(ND) (71). These findings suggest that Aβ deposition measured by PIB is an upstream process, whereas neurodegeneration is a downstream process initiated by Aβ deposition and is more closely related to cognitive decline (10, 72). There are two possible reasons why the keyword “PIB (amyloid PET tracer)” appears in the field of MRI studies in AD. One of which is some studies have evaluated AD using both PET-CT and MRI for pairwise comparison (72). Another potential reason is that partial studies have used PET-MR, a novel imaging technique, to evaluate AD (73).
Genetic data from the ADNI database and from transgenic model experiments have been crucial in advancing the understanding of AD pathophysiology. All of these human genetic data can be obtained from apolipoprotein E (APOE) genotyping, genome-wide association studies (GWAS), and whole-exome and whole-genome sequencing. Researchers have strived to identify new genetic susceptibility loci for AD by integrating genetic and imaging data obtained from the ADNI database. The first GWAS of an ADNI quantitative phenotype was published in 2009 (74). Potkin identified a number of loci potentially associated with hippocampal atrophy through an imaging-genetics approach, and progress has been rapid since then. Furney discovered PICALM, a significant gene associated with entorhinal cortical thickness (75). To date, GWASs have identified more than 20 genetic loci associated with the risk of AD (1, 27, 76). It is well known that APOE ε4 is the major genetic risk factor for late-onset or sporadic AD (77), whereas mutations in amyloid precursor protein (APP), presenilin-1 (PSEN1), and presenilin-2 (PSEN2) can cause early-onset or familial AD (78, 79). It is widely reported that APOE e4 allele is the strongest genetic risk factor of ARIA incidence in anti-Aβ monoclonal antibody-treated AD patients (6, 7, 80). Furthermore, transgenic murine model experiments have revealed the underlying mechanisms as well as treatment of AD in animal models using high-field MRI (22, 28–31, 81). Burgess (28) demonstrated that repeated MR imaging-guided focused ultrasound treatments led to spatial memory improvement in a Tg mouse model of AD, which may be mediated by decreased amyloid pathologic abnormalities and increased neuronal plasticity. Snow (22) demonstrated for the first time that diffusion tensor imaging (DTI) abnormalities were present in the gray matter of an AD mouse model in which both pathological hallmarks are present. Shah (31) identified that hypersynchrony of function connection (FC) may be used as a new non-invasive read-out of early AD and can be recovered by anti-Aβ treatment in TG2576 mice, providing an early therapeutic window before amyloid plaque deposition. Overall, MRI is more widely used in clinical settings for assisting in the early diagnosis and prognosis prediction of AD patients, while preclinical research toward precision medicine and pathological mechanisms in small animal models using MRI is relatively less, because of the high requirements for MR equipment, e.g., ultra-high field MRI (often ≥7.0 T) is generally required.
Furthermore, multimodal MRI is widely used for the early diagnosis of AD. In addition to sMRI and PET-MRI, fMRI has been used to measure the activity of neurons and evaluate the functional relationships between brain regions indirectly and noninvasively. First, increased hippocampal activity has been found to be associated with increased risk for AD (82, 83). Studies have revealed reduced left CA2, 3 and dentate gyrus (CA23DG) activity in cognitively intact APOE ε4 carriers, which may suggest that reduced neural activity in hippocampal subregions may underlie the compensatory increase in extrahippocampal activity in people with a genetic risk for AD prior to the onset of cognitive deficits (84). Second, arterial spin labeling (ASL) is an important MR imaging technique used to assess cerebral blood flow (CBF) quantitatively at the tissue level by magnetically labeling inflowing blood (23). AD patients have consistently shown a reduction in CBF in a posterior parietal region, including the posterior cingulate, angular gyrus, precuneus, and superior parietal gyrus (21, 85–88). Chao reported that abnormal perfusion in the precuneus may predict conversion from MCI to AD (89). Collij demonstrated that the combination of CBF and the entorhinal cortex atrophy score can significantly increase the diagnostic ability of AD, with a prediction probability of 95% (90). The ASL pattern is remarkably similar to the pattern of hypometabolism observed with FDG PET, and both modalities have similar diagnostic performance (23, 88, 91). Third, resting-state fMRI (rs-fMRI) has become one of the most widely used neuroimaging techniques for studying brain function correlated with pathological biomarkers of AD (92, 93). Studies have detected decreased FC in the default mode network (DMN), and the posterior cingulate cortex/pecuneus is the most severely affected brain region (20). DMN is one of the most vulnerable brain networks in AD because of the earliest accumulation of Aβ (19, 20, 94, 95). The early deposition of the pathological protein Aβ in the DMN region may be related to the influence of resting-state whole-brain activity on the lymphatic clearance pathway (96), suggesting that rs-fMRI technology has the potential to be used as an imaging indicator for the early identification of AD and for guiding clinical prevention and treatment. Anti-amyloid monoclonal antibodies have raised concerns about adverse effects, particularly ARIA (5, 6, 8). ARIA includes ARIA-E (parenchymal or sulcal hyperintensities on FLAIR indicative of parenchymal edema or sulcal effusions) and ARIA-H (hypointense regions on gradient recalled-echo/T2* indicative of hemosiderin deposition) (97). Timely detection and monitor of ARIA in clinical practice is crucial.
4.3 Stage III
Stage III focused on the study of AI techniques in the MRI analysis of the AD brain. The research focused on “#0 tau pathology” and “#1 deep learning (DL),” and the main keywords used were subjective cognitive decline, machine learning (ML), convolutional neural network (CNN), and DL.
Recently, increasing evidence has shown that hyperphosphorylated tau protein appears earlier than Aβ does and is more closely related to cognitive impairment, which is deemed to be more sensitive for the early detection of AD (98, 99). Both sMRI and FDG-PET are commonly used for measuring tau-mediated neuronal injury. Emerging MRI modalities such as DTI and rs-fMRI have also shown great potential in capturing changes due to tau pathology (100, 101). Visual assessment (102) or quantification of the hippocampus (103) is the most frequently used biomarker for measuring tau-mediated injury in AD and has been confirmed via several autopsy studies (40). In addition, atrophy is closely associated with tau accumulation in certain brain regions and is related to biomarkers of tau accumulation and brain hypometabolism, such as CSF p-tau/t-tau levels, FDG-PET, or PET imaging with tau ligands (104, 105). At present, most of the studies on tau PET have focused on specific regions of interest, including the occipital lobe, parietal lobe, temporal lobe cortex and parietal lobe cortex, in the AD cohort, which may reflect the correlation between tau protein deposition and cognitive decline (106). Ossenkoppele reported that tau PET was a promising prognostic tool for predicting cognitive decline in preclinical and prodromal stages of AD, that was superior to amyloid PET and MRI (107). Although tau PET imaging has attracted much attention compared with Aβ PET imaging, it still needs to be verified on a large scale to become a reliable tool for AD diagnosis.
In the past 6 years, the keywords have gradually evolved to use AI-powered technologies, particularly ML and DL, combined with MRI to achieve early diagnosis and prognosis of AD (108, 109). DL is an important branch of ML that uses neural networks of simple interconnected units to extract patterns from data to solve complex problems (110). A neural network is the basis of the DL method. Common AI algorithms include logistic regression (LR), support vector machines (SVMs), random forests (RFs), CNNs, and nonconvolutional artificial neural networks (NC-ANNs). ML and DL have a wide range of applications for the early differentiation of normal cognition and AD (110–112). To date, the most widely used image analysis model is CNN (113). CNNs have gained popularity quickly in MRI analysis for AD, first in 2017, with promising performance (114). Ashyam applied DeepBrainNet, a recent DL algorithm with transfer learning, to discriminate neurologic diseases on the basis of MRI brain age and achieved 86% accuracy in differentiating AD patients from healthy controls (HCs) (115). The CNN studies that combined T1WI with FDG-PET data yielded considerably different results (98% vs. 90% accuracy for AD patients vs. HCs) (116). Frizzell demonstrated that CNNs have better performance metrics than the other major algorithm types do in the classification of AD, MCI, and normal aging and the prediction of MCI conversion to AD (111). Sima applied an DL-based assistive software that can automatically detect and quantify ARIA on brain MRI scans, which has the potential to be a clinically important tool to improve safety monitoring and management of patients with AD treated with Aβ-directed monoclonal antibody therapies (117). With the development of AI, more noninvasive examinations, minimally invasive diagnoses, and more safer treatment methods have been developed, suggesting that the application of AI in AD has attracted the attention of many researchers and will be a research hotspot in the next few years.
5 Limitation
Some limitations of this study must be highlighted. First, all publications included in this study were downloaded from the WoSCC; therefore, they may not represent the complete research field of MRI in AD. Second, our study included only English literature, which may have led to language bias and the consequent omission of high-quality literature from other languages. Third, the publication number of countries, institutions and authors are based on all co-authors, rather than the institutions and countries of the first author. And some authors not only list the school of medicine as their affiliation but also list the hospital as a second affiliation. This led to overlap on the results, causing a bias of the influence. Finally, it takes time for articles to reach a certain number of citations after publication, which may mean that high-quality articles published in recent years have not yet reached the level of citations commensurate with their quality and may have led to research bias.
6 Conclusion
MRI research on AD is rapidly progressing. Advances in basic science and molecular diagnostics have provided unprecedented possibilities for early diagnosis and prognosis prediction. The United States has always been in a leading position in this field. In addition, notably, the research focus in this field has gradually shifted from invasive diagnosis and treatment to noninvasive diagnosis and more accurate safer treatment through AI-aided technologies such as ML and DL.
Recent advancements in AD treatment have focused on eliminating Aβ plaques, whereas MR imaging abnormalities collectively referred to as ARIA have been reported for several agents, thereby lowering the expectations around the eagerly awaited first-generation monoclonal antibodies. Therefore, application of the AI-aided technology to monitor and predict the efficacy and the probable side effect of new drugs or new therapies on brain MRI scans from AD patients is projected to be the research hotspot in the future.
Data availability statement
The raw data supporting the conclusions of this article will be made available by the authors, without undue reservation.
Author contributions
XS: Methodology, Writing – original draft. JZ: Conceptualization, Investigation, Writing – original draft. RL: Data curation, Writing – original draft. YP: Software, Supervision, Writing – review & editing. LG: Investigation, Supervision, Writing – review & editing.
Funding
The author(s) declare that financial support was received for the research, authorship, and/or publication of this article. This study was supported by the youth research project of Jiangxi science and technology department (20171BAB215049).
Acknowledgments
The authors would like to thank all study participants.
Conflict of interest
The authors declare that the research was conducted in the absence of any commercial or financial relationships that could be construed as a potential conflict of interest.
Generative AI statement
The authors declare that no Generative AI was used in the creation of this manuscript.
Publisher’s note
All claims expressed in this article are solely those of the authors and do not necessarily represent those of their affiliated organizations, or those of the publisher, the editors and the reviewers. Any product that may be evaluated in this article, or claim that may be made by its manufacturer, is not guaranteed or endorsed by the publisher.
References
1. Scheltens, P, Blennow, K, Breteler, MM, de Strooper, B, Frisoni, GB, Salloway, S, et al. Alzheimer's disease. Lancet. (2016) 388:505–17. doi: 10.1016/S0140-6736(15)01124-1
2. Alzheimer’s Association. 2023 Alzheimer's disease facts and figures. Alzheimers Dement. (2023) 19:1598–695. doi: 10.1002/alz.13016
3. Karran, E, Mercken, M, and De Strooper, B. The amyloid cascade hypothesis for Alzheimer's disease: an appraisal for the development of therapeutics. Nat Rev Drug Discov. (2011) 10:698–712. doi: 10.1038/nrd3505
4. Braak, H, and Braak, E. Neuropathological stageing of Alzheimer-related changes. Acta Neuropathol. (1991) 82:239–59. doi: 10.1007/BF00308809
5. Jeong, SY, Suh, CH, Kim, SJ, Lemere, CA, Lim, JS, and Lee, JH. Amyloid-related imaging abnormalities in the era of anti-amyloid Beta monoclonal antibodies for Alzheimer's disease: recent updates on clinical and imaging features and MRI monitoring. Korean J Radiol. (2024) 25:726–41. doi: 10.3348/kjr.2024.0105
6. Sperling, RA, Jack, CR Jr, Black, SE, Frosch, MP, Greenberg, SM, Hyman, BT, et al. Amyloid-related imaging abnormalities in amyloid-modifying therapeutic trials: recommendations from the Alzheimer's Association research roundtable workgroup. Alzheimers Dement. (2011) 7:367–85. doi: 10.1016/j.jalz.2011.05.2351
7. Loomis, SJ, Miller, R, Castrillo-Viguera, C, Umans, K, Cheng, W, O'Gorman, J, et al. Genome-wide association studies of ARIA from the Aducanumab phase 3 ENGAGE and EMERGE studies. Neurology. (2024) 102:e207919. doi: 10.1212/WNL.0000000000207919
8. Filippi, M, Cecchetti, G, Spinelli, EG, Vezzulli, P, Falini, A, and Agosta, F. Amyloid-related imaging abnormalities and beta-amyloid-targeting antibodies: a systematic review. JAMA Neurol. (2022) 79:291–304. doi: 10.1001/jamaneurol.2021.5205
9. Vemuri, P, and Jack, CR Jr. Role of structural MRI in Alzheimer's disease. Alzheimers Res Ther. (2010) 2:23. doi: 10.1186/alzrt47
10. Frisoni, GB, Fox, NC, Jack, CR Jr, Scheltens, P, and Thompson, PM. The clinical use of structural MRI in Alzheimer disease. Nat Rev Neurol. (2010) 6:67–77. doi: 10.1038/nrneurol.2009.215
11. Murray, ME, Kouri, N, Lin, WL, Jack, CR Jr, Dickson, DW, and Vemuri, P. Clinicopathologic assessment and imaging of tauopathies in neurodegenerative dementias. Alzheimers Res Ther. (2014) 6:1. doi: 10.1186/alzrt231
12. Rose, SE, Janke, AL, and Chalk, JB. Gray and white matter changes in Alzheimer's disease: a diffusion tensor imaging study. J Magn Reson Imaging. (2008) 27:20–6. doi: 10.1002/jmri.21231
13. Itkyal, VS, Abrol, A, LaGrow, TJ, Fedorov, A, and Calhoun, VD. Voxel-wise fusion of resting fMRI networks and gray matter volume for Alzheimer's disease classification using deep multimodal learning. Res Sq. (2023):rs.3.rs-3740218. doi: 10.21203/rs.3.rs-3740218/v1
14. Tian, Y, Oh, JH, Rhee, HY, Park, S, Ryu, CW, Cho, AR, et al. Gray-white matter boundary Z-score and volume as imaging biomarkers of Alzheimer's disease. Front Aging Neurosci. (2023) 15:1291376. doi: 10.3389/fnagi.2023.1291376
15. Vermeer, SE, Prins, ND, den Heijer, T, Hofman, A, Koudstaal, PJ, and Breteler, MM. Silent brain infarcts and the risk of dementia and cognitive decline. N Engl J Med. (2003) 348:1215–22. doi: 10.1056/NEJMoa022066
16. Acharya, A, Liang, X, Tian, W, Jiang, C, Han, Y, and Yi, L. White matter Hyperintensities relate to basal ganglia functional connectivity and memory performance in aMCI and SVMCI. Front Neurosci. (2019) 13:1204. doi: 10.3389/fnins.2019.01204
17. McDonald, CR, McEvoy, LK, Gharapetian, L, Fennema-Notestine, C, Hagler, DJ Jr, Holland, D, et al. Regional rates of neocortical atrophy from normal aging to early Alzheimer disease. Neurology. (2009) 73:457–65. doi: 10.1212/WNL.0b013e3181b16431
18. Duara, R, Loewenstein, DA, Potter, E, Appel, J, Greig, MT, Urs, R, et al. Medial temporal lobe atrophy on MRI scans and the diagnosis of Alzheimer disease. Neurology. (2008) 71:1986–92. doi: 10.1212/01.wnl.0000336925.79704.9f
19. Palmqvist, S, Scholl, M, Strandberg, O, Mattsson, N, Stomrud, E, Zetterberg, H, et al. Earliest accumulation of beta-amyloid occurs within the default-mode network and concurrently affects brain connectivity. Nat Commun. (2017) 8:1214. doi: 10.1038/s41467-017-01150-x
20. Ibrahim, B, Suppiah, S, Ibrahim, N, Mohamad, M, Hassan, HA, Nasser, NS, et al. Diagnostic power of resting-state fMRI for detection of network connectivity in Alzheimer's disease and mild cognitive impairment: a systematic review. Hum Brain Mapp. (2021) 42:2941–68. doi: 10.1002/hbm.25369
21. Binnewijzend, MA, Kuijer, JP, Benedictus, MR, van der Flier, WM, Wink, AM, Wattjes, MP, et al. Cerebral blood flow measured with 3D pseudocontinuous arterial spin-labeling MR imaging in Alzheimer disease and mild cognitive impairment: a marker for disease severity. Radiology. (2013) 267:221–30. doi: 10.1148/radiol.12120928
22. Snow, WM, Dale, R, O'Brien-Moran, Z, Buist, R, Peirson, D, Martin, M, et al. In vivo detection of gray matter neuropathology in the 3xTg mouse model of Alzheimer's disease with diffusion tensor imaging. J Alzheimers Dis. (2017) 58:841–53. doi: 10.3233/JAD-170136
23. Haller, S, Zaharchuk, G, Thomas, DL, Lovblad, KO, Barkhof, F, and Golay, X. Arterial spin labeling perfusion of the brain: emerging clinical applications. Radiology. (2016) 281:337–56. doi: 10.1148/radiol.2016150789
24. Voevodskaya, O, Sundgren, PC, Strandberg, O, Zetterberg, H, Minthon, L, Blennow, K, et al. Myo-inositol changes precede amyloid pathology and relate to APOE genotype in Alzheimer disease. Neurology. (2016) 86:1754–61. doi: 10.1212/WNL.0000000000002672
25. Shaw, LM, Vanderstichele, H, Knapik-Czajka, M, Clark, CM, Aisen, PS, Petersen, RC, et al. Cerebrospinal fluid biomarker signature in Alzheimer's disease neuroimaging initiative subjects. Ann Neurol. (2009) 65:403–13. doi: 10.1002/ana.21610
26. Visser, PJ, Verhey, F, Knol, DL, Scheltens, P, Wahlund, LO, Freund-Levi, Y, et al. Prevalence and prognostic value of CSF markers of Alzheimer's disease pathology in patients with subjective cognitive impairment or mild cognitive impairment in the DESCRIPA study: a prospective cohort study. Lancet Neurol. (2009) 8:619–27. doi: 10.1016/S1474-4422(09)70139-5
27. Saykin, AJ, Shen, L, Yao, X, Kim, S, Nho, K, Risacher, SL, et al. Genetic studies of quantitative MCI and AD phenotypes in ADNI: Progress, opportunities, and plans. Alzheimers Dement. (2015) 11:792–814. doi: 10.1016/j.jalz.2015.05.009
28. Burgess, A, Dubey, S, Yeung, S, Hough, O, Eterman, N, Aubert, I, et al. Alzheimer disease in a mouse model: MR imaging-guided focused ultrasound targeted to the hippocampus opens the blood-brain barrier and improves pathologic abnormalities and behavior. Radiology. (2014) 273:736–45. doi: 10.1148/radiol.14140245
29. Grandjean, J, Derungs, R, Kulic, L, Welt, T, Henkelman, M, Nitsch, RM, et al. Complex interplay between brain function and structure during cerebral amyloidosis in APP transgenic mouse strains revealed by multi-parametric MRI comparison. NeuroImage. (2016) 134:1–11. doi: 10.1016/j.neuroimage.2016.03.042
30. Kuhla, A, Ruhlmann, C, Lindner, T, Polei, S, Hadlich, S, Krause, BJ, et al. APPswe/PS1dE9 mice with cortical amyloid pathology show a reduced NAA/Cr ratio without apparent brain atrophy: a MRS and MRI study. Neuroimage Clin. (2017) 15:581–6. doi: 10.1016/j.nicl.2017.06.009
31. Shah, D, Praet, J, Latif Hernandez, A, Hofling, C, Anckaerts, C, Bard, F, et al. Early pathologic amyloid induces hypersynchrony of BOLD resting-state networks in transgenic mice and provides an early therapeutic window before amyloid plaque deposition. Alzheimers Dement. (2016) 12:964–76. doi: 10.1016/j.jalz.2016.03.010
32. Yue, JH, Zhang, QH, Yang, X, Wang, P, Sun, XC, Yan, SY, et al. Magnetic resonance imaging of white matter in Alzheimer's disease: a global bibliometric analysis from 1990 to 2022. Front Neurosci. (2023) 17:1163809. doi: 10.3389/fnins.2023.1163809
33. Chen, C, Dubin, R, and Kim, MC. Emerging trends and new developments in regenerative medicine: a scientometric update (2000 – 2014). Expert Opin Biol Ther. (2014) 14:1295–317. doi: 10.1517/14712598.2014.920813
34. Ma, C, Su, H, and Li, H. Global research trends on prostate diseases and erectile dysfunction: a bibliometric and visualized study. Front Oncol. (2020) 10:627891. doi: 10.3389/fonc.2020.627891
35. Shen, Z, Wu, H, Chen, Z, Hu, J, Pan, J, Kong, J, et al. The global research of artificial intelligence on prostate Cancer: a 22-year bibliometric analysis. Front Oncol. (2022) 12:843735. doi: 10.3389/fonc.2022.843735
36. Yu, Y, Li, Y, Zhang, Z, Gu, Z, Zhong, H, Zha, Q, et al. A bibliometric analysis using VOSviewer of publications on COVID-19. Ann Transl Med. (2020) 8:816. doi: 10.21037/atm-20-4235
37. Ioannidis, JPA, Baas, J, Klavans, R, and Boyack, KW. A standardized citation metrics author database annotated for scientific field. PLoS Biol. (2019) 17:e3000384. doi: 10.1371/journal.pbio.3000384
38. Reijmer, YD, van Veluw, SJ, and Greenberg, SM. Ischemic brain injury in cerebral amyloid angiopathy. J Cereb Blood Flow Metab. (2016) 36:40–54. doi: 10.1038/jcbfm.2015.88
39. Fox, NC, Scahill, RI, Crum, WR, and Rossor, MN. Correlation between rates of brain atrophy and cognitive decline in AD. Neurology. (1999) 52:1687–9. doi: 10.1212/WNL.52.8.1687
40. Whitwell, JL, Josephs, KA, Murray, ME, Kantarci, K, Przybelski, SA, Weigand, SD, et al. MRI correlates of neurofibrillary tangle pathology at autopsy: a voxel-based morphometry study. Neurology. (2008) 71:743–9. doi: 10.1212/01.wnl.0000324924.91351.7d
41. Vemuri, P, Wiste, HJ, Weigand, SD, Shaw, LM, Trojanowski, JQ, Weiner, MW, et al. MRI and CSF biomarkers in normal, MCI, and AD subjects: predicting future clinical change. Neurology. (2009) 73:294–301. doi: 10.1212/WNL.0b013e3181af79fb
42. Thompson, PM, Hayashi, KM, De Zubicaray, GI, Janke, AL, Rose, SE, Semple, J, et al. Mapping hippocampal and ventricular change in Alzheimer disease. NeuroImage. (2004) 22:1754–66. doi: 10.1016/j.neuroimage.2004.03.040
43. Vemuri, P, Whitwell, JL, Kantarci, K, Josephs, KA, Parisi, JE, Shiung, MS, et al. Antemortem MRI based STructural abnormality iNDex (STAND)-scores correlate with postmortem Braak neurofibrillary tangle stage. NeuroImage. (2008) 42:559–67. doi: 10.1016/j.neuroimage.2008.05.012
44. Thompson, PM, Hayashi, KM, de Zubicaray, G, Janke, AL, Rose, SE, Semple, J, et al. Dynamics of gray matter loss in Alzheimer's disease. J Neurosci. (2003) 23:994–1005. doi: 10.1523/JNEUROSCI.23-03-00994.2003
45. Scahill, RI, Schott, JM, Stevens, JM, Rossor, MN, and Fox, NC. Mapping the evolution of regional atrophy in Alzheimer's disease: unbiased analysis of fluid-registered serial MRI. Proc Natl Acad Sci USA. (2002) 99:4703–7. doi: 10.1073/pnas.052587399
46. Delacourte, A, David, JP, Sergeant, N, Buee, L, Wattez, A, Vermersch, P, et al. The biochemical pathway of neurofibrillary degeneration in aging and Alzheimer's disease. Neurology. (1999) 52:1158–65. doi: 10.1212/WNL.52.6.1158
47. Frisoni, GB, Henneman, WJ, Weiner, MW, Scheltens, P, Vellas, B, Reynish, E, et al. The pilot European Alzheimer's disease Neuroimaging initiative of the European Alzheimer's disease consortium. Alzheimers Dement. (2008) 4:255–64. doi: 10.1016/j.jalz.2008.04.009
48. Ashburner, J, and Friston, KJ. Voxel-based morphometry—the methods. NeuroImage. (2000) 11:805–21. doi: 10.1006/nimg.2000.0582
49. Silbert, LC, Quinn, JF, Moore, MM, Corbridge, E, Ball, MJ, Murdoch, G, et al. Changes in premorbid brain volume predict Alzheimer's disease pathology. Neurology. (2003) 61:487–92. doi: 10.1212/01.WNL.0000079053.77227.14
50. Thompson, PM, and Apostolova, LG. Computational anatomical methods as applied to ageing and dementia. Br J Radiol. (2007) 80 Spec No 2:S78–91. doi: 10.1259/BJR/20005470
51. Bozzali, M, Filippi, M, Magnani, G, Cercignani, M, Franceschi, M, Schiatti, E, et al. The contribution of voxel-based morphometry in staging patients with mild cognitive impairment. Neurology. (2006) 67:453–60. doi: 10.1212/01.wnl.0000228243.56665.c2
52. Karas, GB, Burton, EJ, Rombouts, SA, van Schijndel, RA, O'Brien, JT, Scheltens, P, et al. A comprehensive study of gray matter loss in patients with Alzheimer's disease using optimized voxel-based morphometry. NeuroImage. (2003) 18:895–907. doi: 10.1016/S1053-8119(03)00041-7
53. Whitwell, JL, Przybelski, SA, Weigand, SD, Knopman, DS, Boeve, BF, Petersen, RC, et al. 3D maps from multiple MRI illustrate changing atrophy patterns as subjects progress from mild cognitive impairment to Alzheimer's disease. Brain. (2007) 130:1777–86. doi: 10.1093/brain/awm112
54. Balthazar, ML, Yasuda, CL, Pereira, FR, Pedro, T, Damasceno, BP, and Cendes, F. Differences in grey and white matter atrophy in amnestic mild cognitive impairment and mild Alzheimer's disease. Eur J Neurol. (2009) 16:468–74. doi: 10.1111/j.1468-1331.2008.02408.x
55. Baxter, LC, Sparks, DL, Johnson, SC, Lenoski, B, Lopez, JE, Connor, DJ, et al. Relationship of cognitive measures and gray and white matter in Alzheimer's disease. J Alzheimers Dis. (2006) 9:253–60. doi: 10.3233/JAD-2006-9304
56. Honea, RA, Thomas, GP, Harsha, A, Anderson, HS, Donnelly, JE, Brooks, WM, et al. Cardiorespiratory fitness and preserved medial temporal lobe volume in Alzheimer disease. Alzheimer Dis Assoc Disord. (2009) 23:188–97. doi: 10.1097/WAD.0b013e31819cb8a2
57. Serra, L, Cercignani, M, Lenzi, D, Perri, R, Fadda, L, Caltagirone, C, et al. Grey and white matter changes at different stages of Alzheimer's disease. J Alzheimers Dis. (2010) 19:147–59. doi: 10.3233/JAD-2010-1223
58. Villain, N, Desgranges, B, Viader, F, de la Sayette, V, Mezenge, F, Landeau, B, et al. Relationships between hippocampal atrophy, white matter disruption, and gray matter hypometabolism in Alzheimer's disease. J Neurosci. (2008) 28:6174–81. doi: 10.1523/JNEUROSCI.1392-08.2008
59. Yoon, B, Shim, YS, Hong, YJ, Koo, BB, Kim, YD, Lee, KO, et al. Comparison of diffusion tensor imaging and voxel-based morphometry to detect white matter damage in Alzheimer's disease. J Neurol Sci. (2011) 302:89–95. doi: 10.1016/j.jns.2010.11.012
60. Li, J, Pan, P, Huang, R, and Shang, H. A meta-analysis of voxel-based morphometry studies of white matter volume alterations in Alzheimer's disease. Neurosci Biobehav Rev. (2012) 36:757–63. doi: 10.1016/j.neubiorev.2011.12.001
61. Wahlund, LO, and Blennow, K. Cerebrospinal fluid biomarkers for disease stage and intensity in cognitively impaired patients. Neurosci Lett. (2003) 339:99–102. doi: 10.1016/S0304-3940(02)01483-0
62. Blennow, K, Hampel, H, Weiner, M, and Zetterberg, H. Cerebrospinal fluid and plasma biomarkers in Alzheimer disease. Nat Rev Neurol. (2010) 6:131–44. doi: 10.1038/nrneurol.2010.4
63. Mattsson, N, Andreasson, U, Persson, S, Carrillo, MC, Collins, S, Chalbot, S, et al. CSF biomarker variability in the Alzheimer's Association quality control program. Alzheimers Dement. (2013) 9:251–61. doi: 10.1016/j.jalz.2013.01.010
64. Vemuri, P, Wiste, HJ, Weigand, SD, Knopman, DS, Trojanowski, JQ, Shaw, LM, et al. Serial MRI and CSF biomarkers in normal aging, MCI, and AD. Neurology. (2010) 75:143–51. doi: 10.1212/WNL.0b013e3181e7ca82
65. Fjell, AM, Walhovd, KB, Fennema-Notestine, C, McEvoy, LK, Hagler, DJ, Holland, D, et al. CSF biomarkers in prediction of cerebral and clinical change in mild cognitive impairment and Alzheimer's disease. J Neurosci. (2010) 30:2088–101. doi: 10.1523/JNEUROSCI.3785-09.2010
66. Jack, CR Jr, Knopman, DS, Jagust, WJ, Shaw, LM, Aisen, PS, Weiner, MW, et al. Hypothetical model of dynamic biomarkers of the Alzheimer's pathological cascade. Lancet Neurol. (2010) 9:119–28. doi: 10.1016/S1474-4422(09)70299-6
67. Jack, CR Jr, Knopman, DS, Jagust, WJ, Petersen, RC, Weiner, MW, Aisen, PS, et al. Tracking pathophysiological processes in Alzheimer's disease: an updated hypothetical model of dynamic biomarkers. Lancet Neurol. (2013) 12:207–16. doi: 10.1016/S1474-4422(12)70291-0
68. Klunk, WE, Engler, H, Nordberg, A, Wang, Y, Blomqvist, G, Holt, DP, et al. Imaging brain amyloid in Alzheimer's disease with Pittsburgh compound-B. Ann Neurol. (2004) 55:306–19. doi: 10.1002/ana.20009
69. Fu, L, Liu, L, Zhang, J, Xu, B, Fan, Y, and Tian, J. Comparison of dual-biomarker PIB-PET and dual-tracer PET in AD diagnosis. Eur Radiol. (2014) 24:2800–9. doi: 10.1007/s00330-014-3311-x
70. Tiepolt, S, Hesse, S, Patt, M, Luthardt, J, Schroeter, ML, Hoffmann, KT, et al. Early [(18)F]florbetaben and [(11)C]PiB PET images are a surrogate biomarker of neuronal injury in Alzheimer's disease. Eur J Nucl Med Mol Imaging. (2016) 43:1700–9. doi: 10.1007/s00259-016-3353-1
71. Meyer, PT, Hellwig, S, Amtage, F, Rottenburger, C, Sahm, U, Reuland, P, et al. Dual-biomarker imaging of regional cerebral amyloid load and neuronal activity in dementia with PET and 11C-labeled Pittsburgh compound B. J Nucl Med. (2011) 52:393–400. doi: 10.2967/jnumed.110.083683
72. Jack, CR Jr, Lowe, VJ, Weigand, SD, Wiste, HJ, Senjem, ML, Knopman, DS, et al. Serial PIB and MRI in normal, mild cognitive impairment and Alzheimer's disease: implications for sequence of pathological events in Alzheimer's disease. Brain. (2009) 132:1355–65. doi: 10.1093/brain/awp062
73. Sheikh-Bahaei, N, Sajjadi, SA, Manavaki, R, McLean, M, O'Brien, JT, and Gillard, JH. Positron emission tomography-guided magnetic resonance spectroscopy in Alzheimer disease. Ann Neurol. (2018) 83:771–8. doi: 10.1002/ana.25202
74. Potkin, SG, Guffanti, G, Lakatos, A, Turner, JA, Kruggel, F, Fallon, JH, et al. Hippocampal atrophy as a quantitative trait in a genome-wide association study identifying novel susceptibility genes for Alzheimer's disease. PLoS One. (2009) 4:e6501. doi: 10.1371/journal.pone.0006501
75. Furney, SJ, Simmons, A, Breen, G, Pedroso, I, Lunnon, K, Proitsi, P, et al. Genome-wide association with MRI atrophy measures as a quantitative trait locus for Alzheimer's disease. Mol Psychiatry. (2011) 16:1130–8. doi: 10.1038/mp.2010.123
76. Harold, D, Abraham, R, Hollingworth, P, Sims, R, Gerrish, A, Hamshere, ML, et al. Genome-wide association study identifies variants at CLU and PICALM associated with Alzheimer's disease. Nat Genet. (2009) 41:1088–93. doi: 10.1038/ng.440
77. Genin, E, Hannequin, D, Wallon, D, Sleegers, K, Hiltunen, M, Combarros, O, et al. APOE and Alzheimer disease: a major gene with semi-dominant inheritance. Mol Psychiatry. (2011) 16:903–7. doi: 10.1038/mp.2011.52
78. Bateman, RJ, Aisen, PS, De Strooper, B, Fox, NC, Lemere, CA, Ringman, JM, et al. Autosomal-dominant Alzheimer's disease: a review and proposal for the prevention of Alzheimer's disease. Alzheimers Res Ther. (2011) 3:1. doi: 10.1186/alzrt59
79. Karch, CM, and Goate, AM. Alzheimer's disease risk genes and mechanisms of disease pathogenesis. Biol Psychiatry. (2015) 77:43–51. doi: 10.1016/j.biopsych.2014.05.006
80. VandeVrede, L, Gibbs, DM, Koestler, M, La Joie, R, Ljubenkov, PA, Provost, K, et al. Symptomatic amyloid-related imaging abnormalities in an APOE epsilon4/epsilon4 patient treated with aducanumab. Alzheimers Dement (Amst). (2020) 12:e12101. doi: 10.1002/dad2.12101
81. Shu, X, Qin, YY, Zhang, S, Jiang, JJ, Zhang, Y, Zhao, LY, et al. Voxel-based diffusion tensor imaging of an APP/PS1 mouse model of Alzheimer's disease. Mol Neurobiol. (2013) 48:78–83. doi: 10.1007/s12035-013-8418-6
82. Ewers, M, Sperling, RA, Klunk, WE, Weiner, MW, and Hampel, H. Neuroimaging markers for the prediction and early diagnosis of Alzheimer's disease dementia. Trends Neurosci. (2011) 34:430–42. doi: 10.1016/j.tins.2011.05.005
83. Sperling, R. Potential of functional MRI as a biomarker in early Alzheimer's disease. Neurobiol Aging. (2011) 32:S37–43. doi: 10.1016/j.neurobiolaging.2011.09.009
84. Suthana, NA, Krupa, A, Donix, M, Burggren, A, Ekstrom, AD, Jones, M, et al. Reduced hippocampal CA2, CA3, and dentate gyrus activity in asymptomatic people at genetic risk for Alzheimer's disease. NeuroImage. (2010) 53:1077–84. doi: 10.1016/j.neuroimage.2009.12.014
85. Yoshiura, T, Hiwatashi, A, Noguchi, T, Yamashita, K, Ohyagi, Y, Monji, A, et al. Arterial spin labelling at 3-T MR imaging for detection of individuals with Alzheimer's disease. Eur Radiol. (2009) 19:2819–25. doi: 10.1007/s00330-009-1511-6
86. Yoshiura, T, Hiwatashi, A, Yamashita, K, Ohyagi, Y, Monji, A, Takayama, Y, et al. Simultaneous measurement of arterial transit time, arterial blood volume, and cerebral blood flow using arterial spin-labeling in patients with Alzheimer disease. AJNR Am J Neuroradiol. (2009) 30:1388–93. doi: 10.3174/ajnr.A1562
87. Du, AT, Jahng, GH, Hayasaka, S, Kramer, JH, Rosen, HJ, Gorno-Tempini, ML, et al. Hypoperfusion in frontotemporal dementia and Alzheimer disease by arterial spin labeling MRI. Neurology. (2006) 67:1215–20. doi: 10.1212/01.wnl.0000238163.71349.78
88. Musiek, ES, Chen, Y, Korczykowski, M, Saboury, B, Martinez, PM, Reddin, JS, et al. Direct comparison of fluorodeoxyglucose positron emission tomography and arterial spin labeling magnetic resonance imaging in Alzheimer's disease. Alzheimers Dement. (2012) 8:51–9. doi: 10.1016/j.jalz.2011.06.003
89. Chao, LL, Buckley, ST, Kornak, J, Schuff, N, Madison, C, Yaffe, K, et al. ASL perfusion MRI predicts cognitive decline and conversion from MCI to dementia. Alzheimer Dis Assoc Disord. (2010) 24:19–27. doi: 10.1097/WAD.0b013e3181b4f736
90. Collij, LE, Heeman, F, Kuijer, JP, Ossenkoppele, R, Benedictus, MR, Moller, C, et al. Application of machine learning to arterial spin labeling in mild cognitive impairment and Alzheimer disease. Radiology. (2016) 281:865–75. doi: 10.1148/radiol.2016152703
91. Chen, Y, Wolk, DA, Reddin, JS, Korczykowski, M, Martinez, PM, Musiek, ES, et al. Voxel-level comparison of arterial spin-labeled perfusion MRI and FDG-PET in Alzheimer disease. Neurology. (2011) 77:1977–85. doi: 10.1212/WNL.0b013e31823a0ef7
92. Li, S, Daamen, M, Scheef, L, Gaertner, FC, Buchert, R, Buchmann, M, et al. Abnormal regional and global connectivity measures in subjective cognitive decline depending on cerebral amyloid status. J Alzheimers Dis. (2021) 79:493–509. doi: 10.3233/JAD-200472
93. Alorf, A, and Khan, MUG. Multi-label classification of Alzheimer's disease stages from resting-state fMRI-based correlation connectivity data and deep learning. Comput Biol Med. (2022) 151:106240. doi: 10.1016/j.compbiomed.2022.106240
94. Buckner, RL, Sepulcre, J, Talukdar, T, Krienen, FM, Liu, H, Hedden, T, et al. Cortical hubs revealed by intrinsic functional connectivity: mapping, assessment of stability, and relation to Alzheimer's disease. J Neurosci. (2009) 29:1860–73. doi: 10.1523/JNEUROSCI.5062-08.2009
95. Buckner, RL, Snyder, AZ, Shannon, BJ, LaRossa, G, Sachs, R, Fotenos, AF, et al. Molecular, structural, and functional characterization of Alzheimer's disease: evidence for a relationship between default activity, amyloid, and memory. J Neurosci. (2005) 25:7709–17. doi: 10.1523/JNEUROSCI.2177-05.2005
96. Han, F, Liu, X, Mailman, RB, Huang, X, and Liu, X. Resting-state global brain activity affects early beta-amyloid accumulation in default mode network. Nat Commun. (2023) 14:7788. doi: 10.1038/s41467-023-43627-y
97. Barakos, J, Sperling, R, Salloway, S, Jack, C, Gass, A, Fiebach, JB, et al. MR imaging features of amyloid-related imaging abnormalities. AJNR Am J Neuroradiol. (2013) 34:1958–65. doi: 10.3174/ajnr.A3500
98. Bao, W, Jia, H, Finnema, S, Cai, Z, Carson, RE, and Huang, YH. PET imaging for early detection of Alzheimer's disease: from pathologic to physiologic biomarkers. PET Clin. (2017) 12:329–50. doi: 10.1016/j.cpet.2017.03.001
99. Gomez-Isla, T, Hollister, R, West, H, Mui, S, Growdon, JH, Petersen, RC, et al. Neuronal loss correlates with but exceeds neurofibrillary tangles in Alzheimer's disease. Ann Neurol. (1997) 41:17–24. doi: 10.1002/ana.410410106
100. McMillan, CT, Irwin, DJ, Avants, BB, Powers, J, Cook, PA, Toledo, JB, et al. White matter imaging helps dissociate tau from TDP-43 in frontotemporal lobar degeneration. J Neurol Neurosurg Psychiatry. (2013) 84:949–55. doi: 10.1136/jnnp-2012-304418
101. Xiao-Chuan, W, Zheng-Hui, H, Zheng-Yu, F, Yue, F, Yun-Huang, Y, Qun, W, et al. Correlation of Alzheimer-like tau hyperphosphorylation and fMRI bold intensity. Curr Alzheimer Res. (2004) 1:143–8. doi: 10.2174/1567205043332216
102. Scheltens, P, Leys, D, Barkhof, F, Huglo, D, Weinstein, HC, Vermersch, P, et al. Atrophy of medial temporal lobes on MRI in "probable" Alzheimer's disease and normal ageing: diagnostic value and neuropsychological correlates. J Neurol Neurosurg Psychiatry. (1992) 55:967–72. doi: 10.1136/jnnp.55.10.967
103. Jack, CR Jr, Petersen, RC, O'Brien, PC, and Tangalos, EG. MR-based hippocampal volumetry in the diagnosis of Alzheimer's disease. Neurology. (1992) 42:183–8. doi: 10.1212/WNL.42.1.183
104. Krajcovicova, L, Klobusiakova, P, and Rektorova, I. Gray matter changes in Parkinson's and Alzheimer's disease and relation to cognition. Curr Neurol Neurosci Rep. (2019) 19:85. doi: 10.1007/s11910-019-1006-z
105. Ni, R. Magnetic resonance imaging in Tauopathy animal models. Front Aging Neurosci. (2021) 13:791679. doi: 10.3389/fnagi.2021.791679
106. Pontecorvo, MJ, Devous, MD, Kennedy, I, Navitsky, M, Lu, M, Galante, N, et al. A multicentre longitudinal study of flortaucipir (18F) in normal ageing, mild cognitive impairment and Alzheimer's disease dementia. Brain. (2019) 142:1723–35. doi: 10.1093/brain/awz090
107. Ossenkoppele, R, Smith, R, Mattsson-Carlgren, N, Groot, C, Leuzy, A, Strandberg, O, et al. Accuracy of tau positron emission tomography as a prognostic marker in preclinical and prodromal Alzheimer disease: a head-to-head comparison against amyloid positron emission tomography and magnetic resonance imaging. JAMA Neurol. (2021) 78:961–71. doi: 10.1001/jamaneurol.2021.1858
108. Guan, H, Wang, C, Cheng, J, Jing, J, and Liu, T. A parallel attention-augmented bilinear network for early magnetic resonance imaging-based diagnosis of Alzheimer's disease. Hum Brain Mapp. (2022) 43:760–72. doi: 10.1002/hbm.25685
109. Turhan, G, Kucuk, H, and Isik, EO. Spatio-temporal convolution for classification of alzheimer disease and mild cognitive impairment. Comput Methods Prog Biomed. (2022) 221:106825. doi: 10.1016/j.cmpb.2022.106825
110. Warren, SL, and Moustafa, AA. Functional magnetic resonance imaging, deep learning, and Alzheimer's disease: a systematic review. J Neuroimaging. (2023) 33:5–18. doi: 10.1111/jon.13063
111. Frizzell, TO, Glashutter, M, Liu, CC, Zeng, A, Pan, D, Hajra, SG, et al. Artificial intelligence in brain MRI analysis of Alzheimer's disease over the past 12 years: a systematic review. Ageing Res Rev. (2022) 77:101614. doi: 10.1016/j.arr.2022.101614
112. Arya, AD, Verma, SS, Chakarabarti, P, Chakrabarti, T, Elngar, AA, Kamali, AM, et al. A systematic review on machine learning and deep learning techniques in the effective diagnosis of Alzheimer's disease. Brain Inform. (2023) 10:17. doi: 10.1186/s40708-023-00195-7
113. LeCun, Y, Bengio, Y, and Hinton, G. Deep learning. Nature. (2015) 521:436–44. doi: 10.1038/nature14539
114. Ghafoorian, M, Karssemeijer, N, Heskes, T, Bergkamp, M, Wissink, J, Obels, J, et al. Deep multi-scale location-aware 3D convolutional neural networks for automated detection of lacunes of presumed vascular origin. Neuroimage Clin. (2017) 14:391–9. doi: 10.1016/j.nicl.2017.01.033
115. Bashyam, VM, Erus, G, Doshi, J, Habes, M, Nasrallah, I, Truelove-Hill, M, et al. MRI signatures of brain age and disease over the lifespan based on a deep brain network and 14 468 individuals worldwide. Brain. (2020) 143:2312–24. doi: 10.1093/brain/awaa160
116. Huang, Y, Xu, J, Zhou, Y, Tong, T, and Zhuang, XAlzheimer’s Disease Neuroimaging Initiative. Diagnosis of Alzheimer's disease via multi-modality 3D convolutional neural network. Front Neurosci. (2019) 13:509. doi: 10.3389/fnins.2019.00509
Keywords: Alzheimer’s disease, magnetic resonance imaging, bibliometric, VOSviewer, CiteSpace
Citation: Sun X, Zhu J, Li R, Peng Y and Gong L (2025) The global research of magnetic resonance imaging in Alzheimer’s disease: a bibliometric analysis from 2004 to 2023. Front. Neurol. 15:1510522. doi: 10.3389/fneur.2024.1510522
Edited by:
Ruiqing Ni, ETH Zürich, SwitzerlandReviewed by:
Zhiliang Wei, The Johns Hopkins Hospital, United StatesXufeng Yao, Shanghai University of Medicine and Health Sciences, China
Copyright © 2025 Sun, Zhu, Li, Peng and Gong. This is an open-access article distributed under the terms of the Creative Commons Attribution License (CC BY). The use, distribution or reproduction in other forums is permitted, provided the original author(s) and the copyright owner(s) are credited and that the original publication in this journal is cited, in accordance with accepted academic practice. No use, distribution or reproduction is permitted which does not comply with these terms.
*Correspondence: Yun Peng, cGVuZ2hoMTI1ODBAMTYzLmNvbQ==; Lianggeng Gong, Z29uZzExMTk5OUAxMjYuY29t