- 1Combined Studies Plan in Medicine (PECEM), Universidad Nacional Autónoma de México (UNAM), Mexico City, Mexico
- 2Faculty of Medicine, Universidad Nacional Autónoma de México (UNAM), Mexico City, Mexico
- 3Faculty of Superior Studies (FES) Iztacala, Universidad Nacional Autónoma de México (UNAM), Mexico City, Mexico
- 4Fundación Gimnasio Moderno, Bogota, Colombia
- 5Neuro-Oncology Unit, Instituto Nacional de Cancerología, Mexico City, Mexico
- 6Precision Medicine Laboratory, Sub-Direction of Research Unit, INCan, Mexico City, Mexico
- 7Neuro-Oncology, Department of Neurology and Neurosurgery, UC Davis Comprehensive Cancer Center, Sacramento, CA, United States
Background: This systematic review and meta-analysis investigated the relationship between somatic TP53 oncogenic variants and prognosis, specifically with overall survival (OS) and progression-free survival (PFS) in patients diagnosed with supratentorial glioblastoma.
Methods: We included longitudinal studies and clinical trials involving a minimum of 40 adult participants diagnosed with supratentorial glioblastoma, wherein the status of TP53 variants was assessed. We conducted searches in multiple databases. We assessed bias risk using a modified version of the Quality in Prognosis Studies tool, and the certainty of evidence was evaluated following the principles of the GRADE approach.
Results and conclusion: This study encompassed 23 papers involving 2,555 patients, out of which 716 had reported oncogenic variants. TP53 oncogenic variants were associated with a reduced likelihood of 1-year survival (OR 0.52, 95% CI 0.29–0.94). However, our analysis did not reveal any significant impact of TP53 variants on overall survival, progression-free survival, or 2-year survival. Therefore, this comprehensive analysis demonstrates that the presence of genetic variants in TP53 does not provide useful information for the prognosis of glioblastoma.
Systematic review registration: https://www.crd.york.ac.uk/prospero/, identifier CRD42021289496.
1 Introduction
Glioblastoma stands as the most prevalent and aggressive primary malignant tumor of the central nervous system, representing a formidable clinical challenge (1). Despite comprehensive treatment strategies encompassing surgery and chemoradiation, patients diagnosed with glioblastoma face a daunting prognosis, marked by a median overall survival (OS) of merely 15.6 months (2).
The diagnostic landscape of central nervous system tumors underwent a significant transformation with the advent of the World Health Organization (WHO) 2016 classification of Tumors of the Central Nervous System (TCNS). This revision incorporated diagnostic molecular factors into the standard histopathological evaluation. Among these pivotal biomarkers, IDH1/2 somatic variants are critical in confirming the diagnosis of glial tumors, including glioblastoma, and distinguishing them from lower-grade counterparts (3). Furthermore, the WHO published a new TCNS classification in 2021, focusing more on tumors’ genetic landscape. This update presents new tumor types and subtypes and includes a reclassification of specific tumors based on their genetic profile and a reviewed tumor taxonomy (4).
TP53 variants exhibit a dual presence within glioblastoma patients’ germline and somatic lineages (5). Germline pathogenic variants in TP53 are associated with Li-Fraumeni syndrome, a hereditary cancer predisposition syndrome (6). On the other hand, somatic TP53 oncogenic variants manifest at a prevalence ranging from 15 to 36% among glioblastoma cases (7, 8). These variants typically occur within exons 5–8, predominantly clustering in hotspots intricately linked to the DNA-binding domain of the TP53 protein (9).
While TP53 oncogenic variants have earned notoriety for their adverse prognostic implications in various cancer types, including breast cancer, esophageal carcinoma, and leukemia, their precise role in dictating outcomes for glioblastoma patients remains an enigma (10, 11). Despite their relatively common occurrence, a definitive association between TP53 status and prognosis in glioblastoma remains elusive.
Therefore, this systematic review and meta-analysis were undertaken to elucidate the intricate relationship between somatic TP53 oncogenic variants and the prognosis of individuals grappling with glioblastoma. We define our inquiry utilizing the PICOTS framework, delineating the population, index prognostic factor, comparator prognostic factor, outcome(s), timing, and setting.
2 Methods
We followed the Preferred Reporting Items for Systematic Reviews and Meta-Analysis (PRISMA) Statement. We registered our systematic review in PROSPERO (registry number: CRD42021289496).
2.1 Eligible studies
Eligible studies: reports with publication dates after 2008, data from humans, a minimum sample size of 40 eligible individuals whose oncogenic variants status had been evaluated by DNA sequencing or PCR, longitudinal studies, and clinical trials. The minimum number of participants was 40 to avoid or minimize the risk of small-study effects and exclude case series.
Eligible participants: individuals with a pathologic confirmed diagnosis of glioblastoma, aged ≥18 years. If an article reported summary data from a mixed pediatric and adult cohort, we pondered its inclusion only if the pediatric component was <10%. Tumors from eligible participants had to be localized in the intracranial supratentorial compartment of the brain. If an article provided specific information about anatomical localization, it was considered for inclusion only if the aggregated fraction of infratentorial and spinal tumors was less than 10%. We generally chose a 10% margin for pediatric patients and infratentorial tumors to avoid discarding studies with more prominent participants. Furthermore, individual patient data (IPD) were reported in most cases. Studies that included participants that did not meet all the inclusion criteria were included only if an out-lined sub-analysis of eligible participants was performed or if individual participant data (IPD) was reported.
In this work, we avoided the use of the word “mutation”; while this term is widely used to describe changes in the nucleotide sequence, it is no longer recommended; instead, we used the term “genetic variant” as proposed by the Joint recommendations of Clinical Genome Resource (ClinGen), Cancer Genomics Consortium (CGC), and Variant Interpretation for Cancer Consortium (VICC). Further classification of somatic genetic variants in the context of cancer is possible with the following five categories: oncogenic (O), likely oncogenic (LO), a variant of uncertain significance (VUS), likely benign, and benign (12).
2.2 Search strategy and screening process
Our systematic search, conducted until October 1st, 2022, encompassed several critical databases: PubMed, Web of Science, Scopus, Biblioteca Virtual en Salud (a search engine aggregating 53 databases, excluding MEDLINE), and OpenGrey. We confined our search to English, Spanish, or Portuguese manuscripts.
To ensure rigor and impartiality, two authors conducted the initial screening of articles independently, employing the search algorithm detailed in Supplementary material 1. When disagreements arose concerning the inclusion or exclusion of specific articles, a constructive dialogue between the two investigators ensued. In cases where a unanimous decision remained elusive, a third author intervened as a tiebreaker to facilitate consensus.
2.3 Data extraction
Data from the included studies were extracted and collected in spreadsheets individually by five authors: DE, KDG, KGRC, JACE, and BCD—another corroborated data extracted by one author. Time-to-event data comparing individuals grouped by TP53 for overall survival (OS) and progression-free survival (PFS) outcomes were extracted as hazard ratios (HRs). In contrast, survival data presented as dichotomous (i.e., 1-year or 2-year survival) was recorded as odd ratios (ORs). Adjusted effect measurements were preferred over unadjusted, particularly when adjusting included the following molecular and clinical variables: IDH1 or IDH2 oncogenic variant status, age, sex, the extent of resection, chemotherapy, radiotherapy, and functional status. The 95% confidence intervals (95% CI) and p values were also extracted for all effect measurements. If the information of interest was not directly provided in the article but IPD was available, we calculated HRs and ORs, adjusting for relevant variables reported in the IPD. Without clearly reported HRs or individual patients’ data, HRs were calculated from Kaplan–Meier curves if image resolution was adequate. Heterogeneity was evaluated with the Cochran Q test and the Higgins I2 statistics.
2.4 Assessment of risk of bias
The risk of bias was assessed with a modified version of the Quality in Prognosis Studies (QUIPS) tool (Supplementary material 2) (13). This modified QUIPS tool was based on the version used by McAleenan et al. (14).
2.5 Data synthesis
We used a random effects model and evaluated the risk of publication bias in each meta-analysis if it included ten or more studies. We expected to synthesize time-to-event survival data through HRs; therefore, we used a modified version of Peter’s test, proposed by Debray, Moons, and Riley (15). For meta-analyses pooling odds ratio, we used Egger’s test. Contour-enhanced funnel plots were created with the “funnel” command from the package metaphor for R (v. 3.8-1). Finally, the certainty of evidence evaluation was carried out according to the principles proposed by the GRADE approach (16).
3 Results
3.1 Study selection
Our initial database search yielded a substantial pool of 14,820 manuscripts. After rigorous screening and assessment, a total of 23 manuscripts were deemed eligible for inclusion in our analysis, as illustrated in Figure 1 and Table 1 (7, 8, 17–37). Notably, it’s worth mentioning that the publication authored by Yang et al. (37) encompassed two distinct studies. It’s also important to note that three of the initially considered reports, specifically those authored by Felsberg et al. (7), Jesionek-Kupnicka et al. (23), and Motomura et al. (26), lacked the essential quantitative data required for inclusion in the quantitative synthesis. Therefore, they were only summarized in the Systematic Review. The studies included in our systematic review are summarized in Table 2.
3.2 The impact of TP53 oncogenic variants on OS
Fifteen reports comprising 16 studies evaluated the effect of TP53 oncogenic variants on OS, and only 13 (14 studies) provided enough quantitative data for the meta-analysis. The 14 studies reported 1,306 participants. After quantitative pooling, the occurrence of TP53 variants was not significantly associated with OS (HR: 1.00, 95% CI: 0.76–1.19, p = 0.98), as observed in Figure 2. There was evidence of moderate heterogeneity in the meta-analysis (Cochran’s Q test p-value: 0.07), with an I2 of 40%. The heterogeneity found in the meta-analysis came from the studies reported by Wang et al. (33) and Parsons et al. (27). When both studies were removed from the analysis, we calculated an I2 of 0%. One of the possible explanations is that the patients reported in these two studies were the youngest among all the reviewed manuscripts. In addition, the study by Wang et al. (33) was designed as a clinical trial.
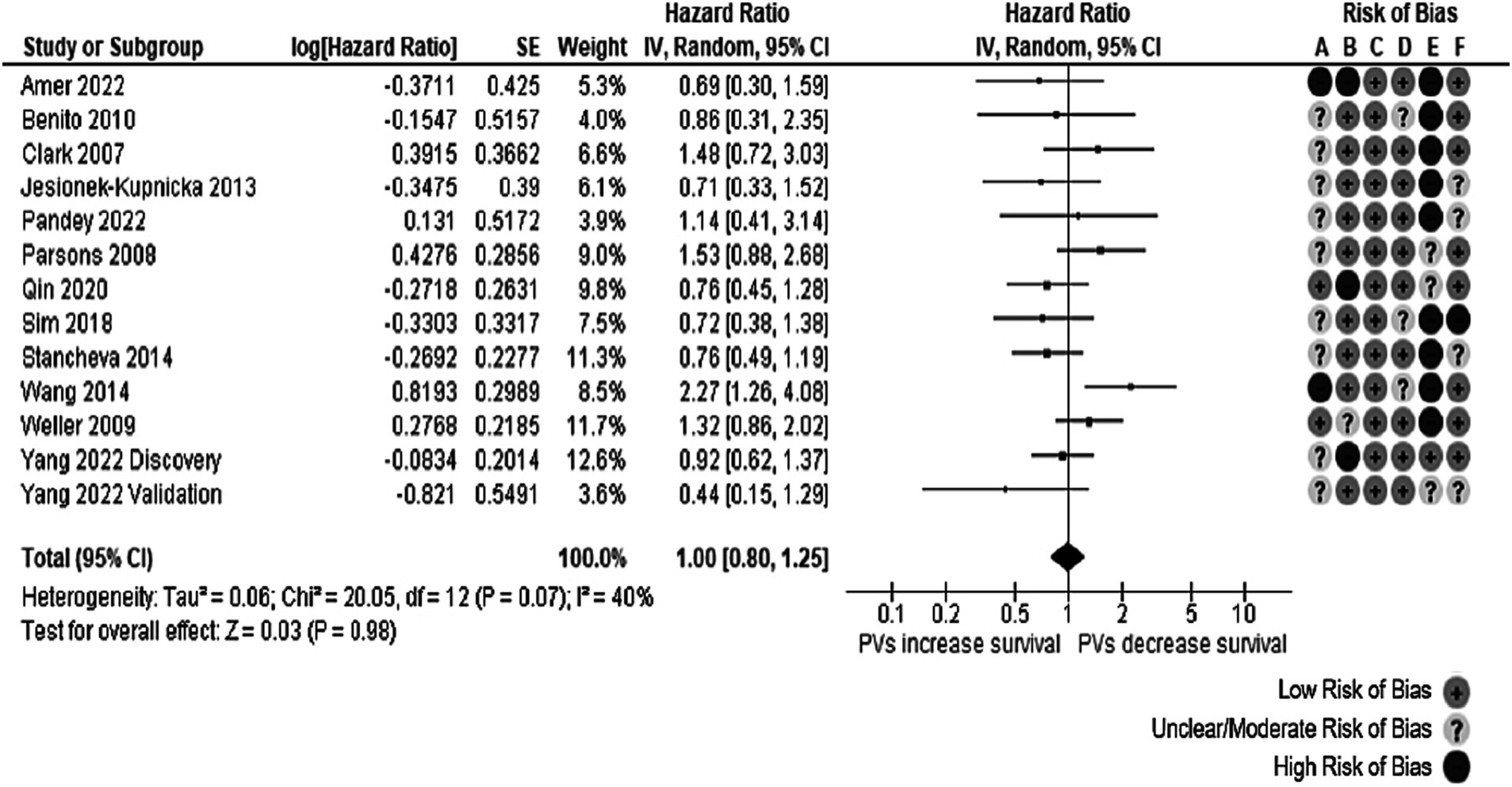
Figure 2. Forest plot of the meta-analysis evaluating the impact of TP53 oncogenic variants on OS, with a summary of the risk of bias assessment through the QUIPS tool. Notice that all studies had at least one domain of the QUIPS tool rated with unclear, moderate, or high risk of bias, particularly the study confounding domain, which was only considered low risk in (36) discovery cohort.
A post hoc subgroup analysis separated reports into those with selected exons (exons 4 or 5 through 8) and those where all exons were sequenced. This subgroup analysis failed to explain the heterogeneity found; the I2 in the “selected exons” subgroup was 55%, and in the “all exons” subgroup, the I2 was 10% (Supplementary Figure S1). No gross asymmetry was observed in the funnel plot (Supplementary Figure S2).
We performed an additional analysis to evaluate the OS in a subgroup of patients with IDH-wt GB (Figure 3). The information from 8 studies with complete details (n = 1,125) found no effect on OS (HR: 0.96, 95% CI: 0.71–1.32, p = 0.82). Significant heterogeneity was uncovered for a Cochran’s Q test p-value of 0.06 and an I2 of 49%. Subgroup analyses for age or sex could not be accomplished due to insufficient information. Most of the heterogeneity in this meta-analysis came from the validation cohort (36) reported. A significant asymmetry was observed in the contour-enhanced funnel plot from this meta-analysis; all the studies fell in the white (non-significant) region, similar to where their missing counterparts would have been plotted, without mainly modifying the total effect size (Supplementary Figure S3).
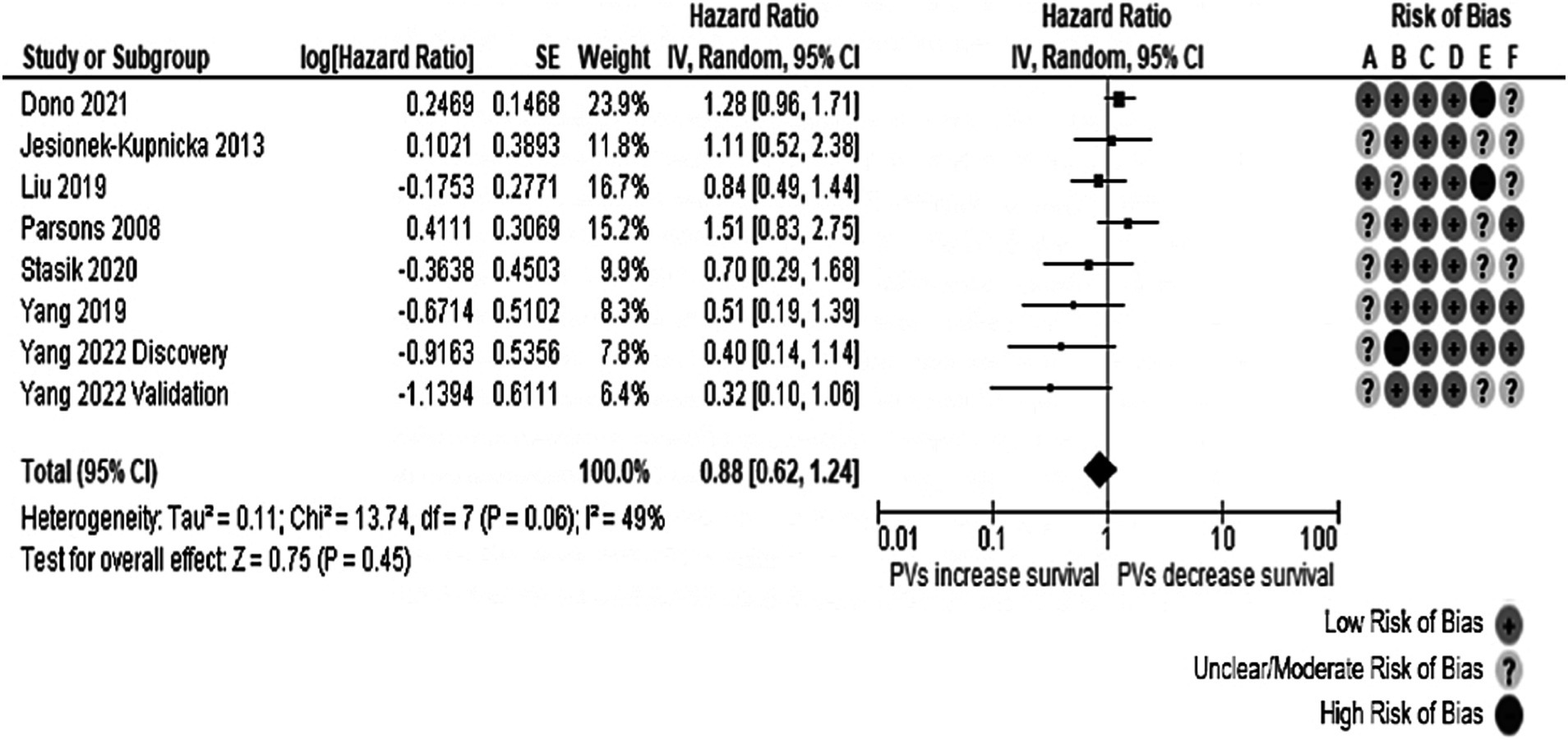
Figure 3. Forest plot of the meta-analysis evaluating the impact of TP53 oncogenic variants on OS in individuals with IDH-wt glioblastomas, with a summary of the risk of bias assessment through the QUIPS tool. All studies had suboptimal ratings according to the QUIPS tool. Risk of Bias domains: A, Study participation; B, Study attrition; C, Prognostic factor measurement; D, Outcome measurement; E, Study confounding; F, Statistical analysis and reporting. 95% CI, 95% Confidence interval; HR, Hazard ratio; IV, Inverse variance; SE, Standard error.
3.3 The impact of TP53 oncogenic variants on PFS
Five studies (four reports) reporting the information on 690 patients were considered to measure the association between TP53 and PFS. The overall effect was insignificant (HR: 0.90, 95% CI: 0.79–1.39, p = 0.55), as observed in Figure 4. Moderate heterogeneity (Cochran’s Q test p-value: 0.20, I2 = 34%) was found. Removing the studies published by Amer et al. (17) and the validation cohort from Yang et al. (37), the I2 value was <20%. Notably, the population in the Amer et al. (17) study reported only gliosarcoma, an uncommon GB variant.
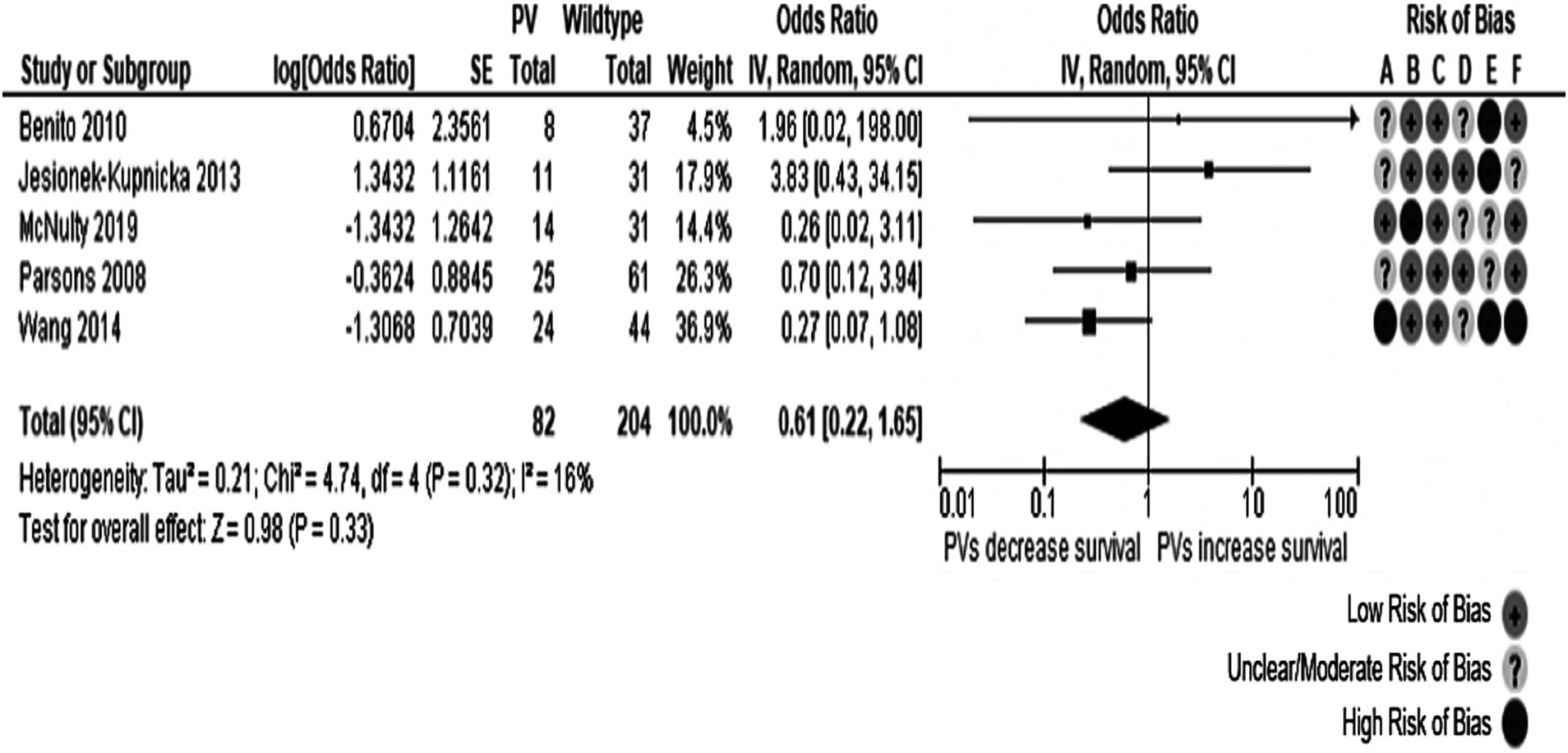
Figure 4. Forest plot of the meta-analysis evaluating the impact of TP53 O/LO genetic variants on PFS in all patients with GB, with a summary of the risk of bias assessment through the QUIPS tool. All studies had suboptimal ratings in at least one domain according to the QUIPS tool. Risk of Bias domains: A, Study participation; B, Study attrition; C, Prognostic factor measurement; D, Outcome measurement; E, Study confounding; F, Statistical analysis and reporting. 95% CI, 95% Confidence interval; HR, Hazard ratio; IV, Inverse variance; SE, Standard error.
In the meta-analysis carried out in individuals with IDH-wt tumors, described in Figure 5, four studies from three different manuscripts were pooled, reporting information from 592 patients. The total effect was non-significant (HR: 0.95, 95% CI: 0.73–1.25, p-value: 0.72). No evidence of heterogeneity was present (Cochran’s Q test p-value: 0.42, I2 = 0%). In the same way, as other meta-analyses in the review, all studies had domains not rated as presenting with a low risk of bias. The Yang et al. (37) validation cohort was responsible for the funnel plot’s notorious asymmetry in both meta-analyses. Further exploration of the reasons behind the imprecision in this study was impossible due to inadequate reporting of the recruitment process and the clinical and demographical characteristics of the cohort.
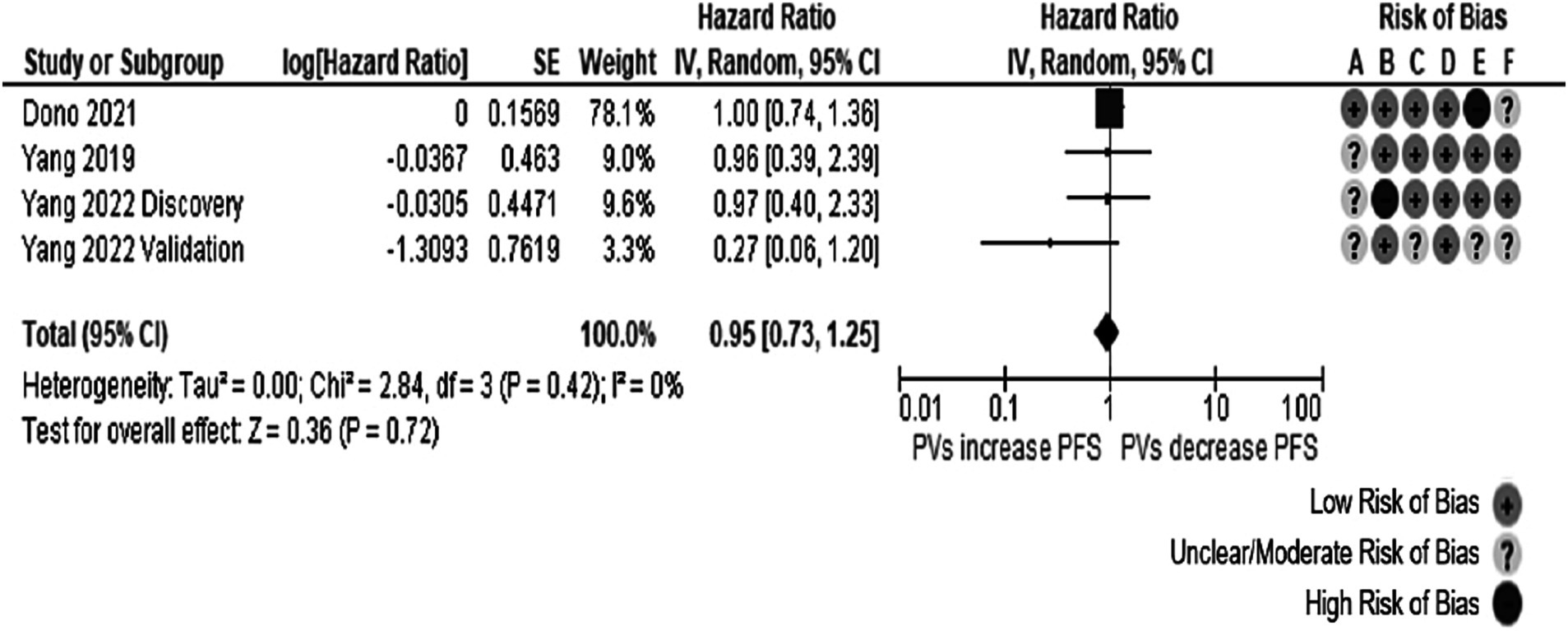
Figure 5. Forest plot of the meta-analysis evaluating the impact of TP53 oncogenic variants on PFS in patients with IDH-wt glioblastomas, with a summary of the risk of bias assessment. The pooled effect was not statistically significant. All studies had suboptimal ratings in at least one domain according to the QUIPS tool. Risk of Bias domains: A, Study participation; B, Study attrition; C, Prognostic factor measurement; D, Outcome measurement; E, Study confounding; F, Statistical analysis and reporting. 95% CI, 95% Confidence interval; HR, Hazard ratio; IV, Inverse variance; SE, Standard error.
3.4 TP53 prognostic effect on dichotomous outcomes
Four meta-analyses evaluating the influence of TP53 oncogenic variants on dichotomous outcomes for 1-year survival and 2-year survival were done. Each of them analyzed separately all glioblastoma patients and those with IDH-wt tumors. Six studies were pooled with information from 350 patients. TP53 somatic oncogenic variants were associated with a lower possibility of 1-year survival in all patients with GB (OR: 0.52, 95% CI: 0.29–0.94, p-value: 0.03). This meta-analysis found no heterogeneity (Cochran’s Q test p-value: 0.44, I2 = 0%). However, all the included studies had two or three domains affected by moderate/unclear or high risk of bias, particularly the study confounding domain, as it was not rated with a low risk of bias. The corresponding forest plot is represented in Figure 6. In the contour-enhanced funnel plot, there was asymmetry in one study in the plot’s p < 0.01 area (gray-shaded). In contrast, the remaining four studies fell in the white area, suggesting a low likelihood of publication bias.
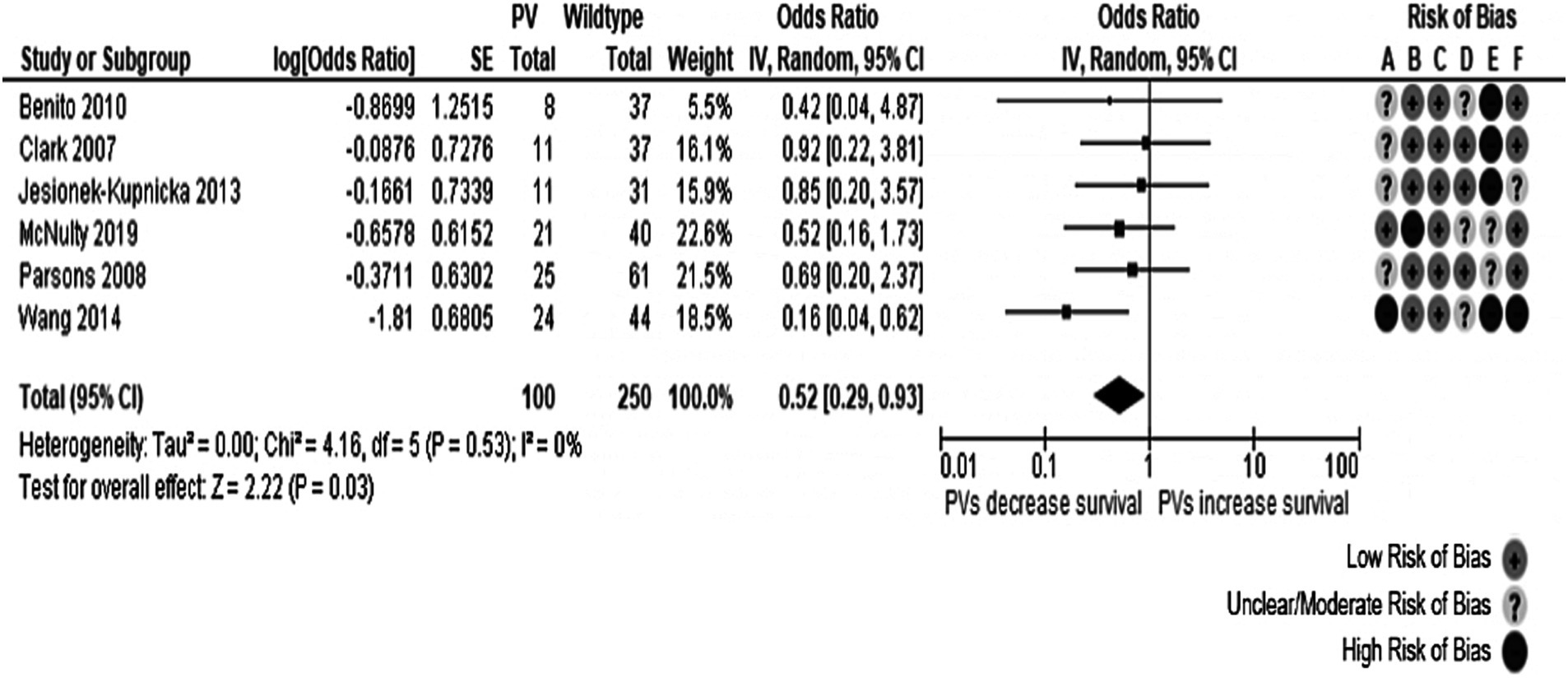
Figure 6. Forest plot of the meta-analysis evaluating the impact of TP53 oncogenic variants on 1-year survival in all patients with glioblastoma, with a summary of the risk of bias assessment. Overall effect size showed a decreased 1-year survival in individuals with TP53 genetic variants. Risk of Bias domains: A, Study participation; B, Study attrition; C, Prognostic factor measurement; D, Outcome measurement; E, Study confounding; F, Statistical analysis and reporting. 95% CI, 95% Confidence interval; HR, Hazard ratio; IV, Inverse variance; SE, Standard error.
No significant effect was observed for any of the other meta-analyses. The meta-analyses for the 1-year survival among individuals with IDH-wt tumors and for the 2-year survival among all individuals with glioblastoma showed a trend toward increased mortality in individuals with oncogenic variants of TP53 without statistical significance. Details about the three remaining meta-analyses are presented in Table 3, and their forest plots can be found in Supplementary Figures S1–S4. Asymmetries in the 1-year survival of patients with IDH-wt glioblastoma and the 2-year survival of all patients funnel plots were caused by Tabone et al. (32) and Wang et al. (33), respectively, with both having several domains in their risk of bias assessment rated as having unclear/moderate or high risk of bias.
3.5 Summary of findings
Table 4 presents the outline of the results from our systematic review.
4 Discussion
In this systematic review and meta-analysis, we analyzed the impact of somatic TP53 oncogenic variants on OS, PFS, and 1-year or 2-year survival in patients with glioblastoma. The presence of TP53 oncogenic variants was associated with a lower 1-year survival possibility (OR: 0.52, 95% CI: 0.29–0.94) but was not related to other outcome measures, namely OS, 2-year mortality, and PFS. However, due to the nature of the meta-analysis and the studies included, it remains essential to question the integrity of the effect seen at the 1-year mark.
We performed four meta-analyses with data obtained from longitudinal studies and clinical trials reporting a minimum of 40 adult participants diagnosed with supratentorial glioblastoma, including cases of IDH-wt glioblastoma, which is a common finding (1). All included reports were from individuals of European or East Asian descent, while individuals of other ethnic backgrounds were underrepresented, which could bias the study. We selected only reports that included the status of somatic TP53 oncogenic variants. However, future studies on TP53 variants should ideally include comparison between different variants, e.g., loss versus gain of function; DNA-binding domain (exons 5–11) versus transactivation domain of the p53 protein; as well as modifier genes and/or interaction with other genes, among other considerations.
Considerable heterogeneity was observed in several of the meta-analyses performed for this review, and subgroup analysis was impossible due to incomplete clinical and demographic characteristics reporting. The heterogeneity might be attributed to three main factors. First, there was insufficient statistical adjustment since only two reports in all the systematic reviews were rated with a low risk of bias in the study confounding domain. Second, differences in medical treatments may be an important source of heterogeneity, but it was not possible to record them since only a few studies described pharmacological or radiotherapeutic regimens. Third, population differences were exemplified by the disappearance of heterogeneity after eliminating studies with younger patients from the OS meta-analysis in all individuals with glioblastoma. Other reports with an important difference in their study population were those of Amer et al. (17) and Yang et al. (37), who only included patients with gliosarcoma. The heterogeneity and asymmetry likely observed in the funnel and forest plots that included these studies derive, at least in part, from the inclusion of gliosarcoma.
The TP53 gene is the most mutated in human cancers. Its protein, p53, acts as a transcription factor that regulates critical processes such as cell cycle control, senescence, apoptosis, DNA repair, and genomic stability, thereby protecting against cancer development. Additionally, p53 influences cellular metabolism, immune responses, ferroptosis, autophagy, and the tumor microenvironment. The activity of p53 is tightly regulated by various proteins, most notably MDM2 and MDMX. In turn, p53 directly interacts with or modulates the expression of multiple genes involved in these pathways, such as XPC, GADD45, CDKN1A, Cyclin B, Bax, Bak, Fas/FasL, SCO2, and G6PDH, among others. However, genetic variants in TP53 not only impair these protective roles but can also endow mutant p53 with oncogenic properties, driving cancer progression, metastasis, and resistance to therapies. Given the complexity of TP53 interactions and actions, it is necessary that future research should include not only the study of TP53 but also its multiple interactions (38, 39). TP53 variants can be classified based on their functional effects: loss of function (LOF), resulting in haploinsufficiency; gain of function (GOF); and a dominant-negative effect (DNE), which impairs transactivation (6). Specific TP53 variants have been shown to influence treatment responses and survival outcomes. For example, mutations at codon 273 in astrocytoma are associated with significantly longer overall survival (OS) and increased chemosensitivity compared to wild-type TP53, whereas in oligodendroglioma, TP53 mutations correlate with shorter OS (40). Additionally, in vitro studies have demonstrated that GOF mutations at codons 237 and 273 are linked to resistance to temozolomide treatment (41). Mutated TP53 protein, particularly within the DNA-binding domain, often forms aggregates with other proteins, including wild-type p53, leading to its inactivation. These aggregates further exacerbate the loss of normal tumor-suppressor activity (42). TP53 variants not only cause misfolding of the protein but also promote the formation of biomolecular condensates and aggregates with amyloid-like properties, particularly in the form of amyloid oligomers located in the nucleus, participating in cancer progression through loss-of-function, negative dominance, and gain-of-function pathways (43). Studies have shown the presence of amyloid oligomers of TP53 in various tumor tissues. Notably, glioblastoma cells resistant to TMZ chemotherapy exhibited significantly higher levels of these amyloid oligomers of mutated TP53 compared to glioblastoma cells with wild-type TP53 or hotspot TP53 mutations not associated with chemoresistance (44). This finding suggests a role for amyloid-like TP53 oligomers in the chemoresistance phenotype of malignant and invasive brain tumors. To study the mechanism by which mutated TP53 forms these amyloid-like aggregates, Petronilho et al. demonstrated that during the aggregation process, the DNA binding domain of TP53 undergoes phase separation before aggregation. Moreover, mutant proteins such as p.Met237Ile and p.Arg249Ser, undergo solid phase transition faster than the WT protein (45).
Importantly, this knowledge about the formation of aggregates by mutated TP53 has enabled the development of therapeutic strategies targeting the phase transitions to solid-like, amorphous, and amyloid-like states (43) or the phase separation process of TP53 (45). While our study aimed to correlate the presence of TP53 variants with prognosis, it is now clear that future research should also consider the influence of TP53 mutations on treatment response.
Several in vitro studies have also investigated targeting TP53 and MDM2 in glioblastoma, emphasizing the necessity of wild-type TP53 for therapeutic efficacy, as certain TP53 variants may accelerate tumor progression or alter treatment responses. There is no strong evidence linking TP53 mutational status directly to standard therapy response in glioblastoma (46, 47).
Despite these insights, our review could not perform a meta-analysis of the impact of TP53 variants on survival or treatment response due to insufficient data on chemotherapy protocols and the lack of individual patient data. As a result, there is currently no robust evidence to definitively establish the clinical relevance of these variants in this context.
The limitations include that some studies presented underpowered multivariate analyses, resulting in wide confidence intervals. According to the QUIPS tool, most studies had at least one domain with a suboptimal rating of risk of bias. Also, we could not evaluate the effect of TP53 oncogenic variants in patients with IDH-mutant tumors due to the low number of patients reported. Another significant limitation is that this is an analysis of the impact of only TP53 without the interaction of this gene with other genes since few studies reported this gene–gene interaction. Despite these shortcomings, the findings of the present systematic review were consistent with the selected population and the measurements done.
A systematic review has certain limitations, particularly the lack of control over key aspects of the included studies, such as the number of individuals analyzed, the data reported in each manuscript, and the follow-up periods. In the case of glioblastoma patients with a median survival of approximately 15 months, studies with follow-up periods exceeding 2 years are rare. Regarding sample size, we carefully selected studies that included a minimum of 40 patients, and although different techniques were used in the selected papers to identify TP53 gene variants (PCR-SSCP, Sanger sequencing, NGS, and WES), each of these methods is widely accepted and validated for this purpose due to their high sensitivity and specificity. Furthermore, we found a 28% prevalence of TP53 variants (716 out of 2,555 individuals), which is consistent with rates reported for glioblastoma (46). Concerning treatment regimens, glioblastoma, being relatively rare and associated with limited life expectancy, has few well-established protocols, resulting in variability in therapeutic approaches based on individual patient factors. The standard treatment remains the STUPP protocol, although alternative regimens explored in some countries have not significantly impacted survival, as demonstrated by our group (48). Another limitation of our study is the low prevalence of the disease, which results in a limited number of published cases. However, using meta-analysis allowed us to achieve statistical significance by weighing the articles based on the number of cases, and while a larger number of cases could provide greater statistical power, the current analysis remains robust.
The results gathered in this systematic review and its meta-analysis are evidence for solving the controversy surrounding the prognostic implication of TP53 genetic variants; for now, the evidence does not support the routinary use of TP53 sequencing as a marker of GB prognosis. In particular, this is important for low-income countries, where efforts should focus on the identification of markers that have demonstrated clinical utility for diagnosis, prognosis and/or choice of treatment, such as the identification of genetic variants in IDH1/2 or MGMT promoter methylation should be a priority.
5 Conclusion
Glioblastoma is a highly aggressive disease with a survival rate of about 15 months, making improvements in diagnosis critical. In this systematic review and meta-analysis, TP53 oncogenic somatic variants were associated with decreased 1-year survival rates in glioblastoma patients; however, no significant correlations were found with overall survival, 2-year mortality, or progression-free survival. Based on current evidence, TP53 sequencing does not appear to be routinely necessary for glioblastoma prognosis. This information is especially relevant for institutions and countries with limited resources. Therefore, this comprehensive analysis demonstrates that the presence of genetic variants in TP53 does not provide useful prognostic information for glioblastoma.
Data availability statement
The original contributions presented in the study are included in the article/Supplementary material, further inquiries can be directed to the corresponding author.
Author contributions
DE: Investigation, Methodology, Writing – review & editing. KG: Investigation, Methodology, Writing – review & editing. KR-C: Investigation, Methodology, Writing – review & editing. BC-D: Writing – review & editing. CT-B: Writing – review & editing. LT-C: Writing – review & editing. OA: Writing – review & editing. JC-E: Conceptualization, Writing – original draft, Formal analysis, Methodology. TW-O: Conceptualization, Data curation, Writing – original draft.
Funding
The author(s) declare that no financial support was received for the research, authorship, and/or publication of this article.
Conflict of interest
The authors declare that the research was conducted in the absence of any commercial or financial relationships that could be construed as a potential conflict of interest.
The author(s) declared that they were an editorial board member of Frontiers, at the time of submission. This had no impact on the peer review process and the final decision.
Publisher’s note
All claims expressed in this article are solely those of the authors and do not necessarily represent those of their affiliated organizations, or those of the publisher, the editors and the reviewers. Any product that may be evaluated in this article, or claim that may be made by its manufacturer, is not guaranteed or endorsed by the publisher.
Supplementary material
The Supplementary material for this article can be found online at: https://www.frontiersin.org/articles/10.3389/fneur.2024.1490246/full#supplementary-material
References
1. Ostrom, QT, Price, M, Neff, C, Cioffi, G, Waite, KA, Kruchko, C, et al. CBTRUS statistical report: primary brain and other central nervous system tumors diagnosed in the United States in 2015-2019. Neuro-Oncology. (2022) 24:v1–v95. doi: 10.1093/neuonc/noac202
2. Marenco-Hillembrand, L, Wijesekera, O, Suarez-Meade, P, Mampre, D, Jackson, C, Peterson, J, et al. Trends in glioblastoma: outcomes over time and type of intervention: a systematic evidence-based analysis. J Neuro Oncol. (2020) 147:297–307. doi: 10.1007/s11060-020-03451-6
3. Loui, SDN, Perry, A, Reifenberger, G, von Deimling, A, Figarella-Branger, D, Cavenee, WK, et al. The 2016 World Health Organization classification of tumors of the central nervous system: a summary. Acta Neuropathol. (2016) 131:803–20. doi: 10.1007/s00401-016-1545-1
4. Louis, DN, Perry, A, Wesseling, P, Brat, DJ, Cree, IA, Figarella-Branger, D, et al. The 2021 WHO classification of tumors of the central nervous system: a summary. Neuro Oncol. (2021) 23:1231–51. doi: 10.1093/neuonc/noab106
5. Kastenhuber, ER, and Lowe, SW. Putting p53 in context. Cell. (2017) 170:1062–78. doi: 10.1016/j.cell.2017.08.028
6. de Andrade, KC, Khincha, PP, Hatton, JN, Frone, MN, Wegman-Ostrosky, T, Mai, PL, et al. Cancer incidence, patterns, and genotype–phenotype associations in individuals with pathogenic or likely pathogenic germline TP53 variants: an observational cohort study. Lancet Oncol. (2021) 22:1787–98. doi: 10.1016/S1470-2045(21)00580-5
7. Felsberg, J, Rapp, M, Loeser, S, Fimmers, R, Stummer, W, Goeppert, M, et al. Prognostic significance of molecular markers and extent of resection in primary glioblastoma patients. Clin Cancer Res. (2009) 15:6683–93. doi: 10.1158/1078-0432.CCR-08-2801
8. Pandey, M, Xiu, J, Mittal, S, Zeng, J, Saul, M, Kesari, S, et al. Molecular alterations associated with improved outcome in patients with glioblastoma treated with tumor-treating fields. Neuro Oncol Adv. (2022) 4:1–10. doi: 10.1093/noajnl/vdac096
9. Bouaoun, L, Sonkin, D, Ardin, M, Hollstein, M, Byrnes, G, Zavadil, J, et al. TP53 variations in human cancers: new lessons from the IARC TP53 database and Genomics data. Hum Mutat. (2016) 37:865–76. doi: 10.1002/humu.23035
10. Fisher, OM, Lord, SJ, Falkenback, D, Clemons, NJ, Eslick, GD, and Lord, RV. The prognostic value of TP53 mutations in oesophageal adenocarcinoma: a systematic review and meta-analysis. Gut. (2017) 66:399–410. doi: 10.1136/gutjnl-2015-310888
11. Petitjean, A, Mathe, E, Kato, S, Ishioka, C, Tavtigian, SV, Hainaut, P, et al. Impact of mutant p53 functional properties on TP53 mutation patterns and tumor phenotype: lessons from recent developments in the IARC TP53 database. Hum Mutat. (2007) 28:622–9. doi: 10.1002/humu.20495
12. Horak, P, Griffith, M, Danos, AM, Pitel, BA, Madhavan, S, Liu, X, et al. Standards for the classification of pathogenicity of somatic variants in cancer (oncogenicity): joint recommendations of clinical genome resource (ClinGen), Cancer Genomics consortium (CGC), and variant interpretation for Cancer consortium (VICC). Genet Med. (2022) 24:986–98. doi: 10.1016/j.gim.2022.01.001
13. Hayden, JA, van der Windt, DA, Cartwright, JL, Côté, P, and Bombardier, C. Assessing Bias in studies of prognostic factors. Ann Intern Med. (2013) 158:280–6. doi: 10.7326/0003-4819-158-4-201302190-00009
14. McAleenan, A, Kell, YC, Spiga, F, Kernohan, A, Cheng, HY, Dawson, S, et al. Prognostic value of test(s) for O6-methylguanine-DNA methyltransferase (MGMT) promoter methylation for predicting overall survival in people with glioblastoma treated with temozolomide. Cochrane Database Syst Rev. (2021) 3:CD013316. doi: 10.1002/14651858.CD013316.pub2
15. Debray, TPA, Moons, KGM, and Riley, RD. Detecting small-study effects and funnel plot asymmetry in meta-analysis of survival data: a comparison of new and existing tests. Res Synth Methods. (2018) 9:41–50. doi: 10.1002/jrsm.1266
16. Foroutan, F, Guyatt, G, Zuk, V, Vandvik, PO, Alba, AC, Mustafa, R, et al. GRADE guidelines 28: use of GRADE for the assessment of evidence about prognostic factors: rating certainty in identification of groups of patients with different absolute risks. J Clin Epidemiol. (2020) 121:62–70. doi: 10.1016/j.jclinepi.2019.12.023
17. Amer, A, Khose, S, Alhasan, H, Pokhylevych, H, Fuller, G, Chasen, N, et al. Clinical and survival characteristics of primary and secondary gliosarcoma patients. Clin Neurol Neurosurg. (2022) 214:107146. doi: 10.1016/j.clineuro.2022.107146
18. Benito, R, Gil-Benso, R, Quilis, V, Perez, M, Gregori-Romero, M, Roldan, P, et al. Primary glioblastomas with and without EGFR amplification: relationship to genetic alterations and clinicopathological features. Neuropathology. (2010) 30:392–400. doi: 10.1111/j.1440-1789.2009.01081.x
19. Clark, AJ, Dos Santos, WG, McCready, J, Chen, MY, Van Meter, TE, Ware, JL, et al. Wilms tumor 1 expression in malignant gliomas and correlation of +KTS isoforms with p53 status. J Neurosurg. (2007) 107:586–92. doi: 10.3171/JNS-07/09/0586
20. Dono, A, Ramesh, AV, Wang, E, Shah, M, Tandon, N, Ballester, LY, et al. The role of RB1 alteration and 4q12 amplification in IDH-WT glioblastoma. Neurooncol Adv. (2021) 3:vdab 050. doi: 10.1093/noajnl/vdab050
21. Hartmann, C, Hentschel, B, Simon, M, Westphal, M, Schackert, G, Tonnet, JC, et al. Long-term survival in primary glioblastoma with versus without isocitrate de-hydrogenase mutations. Clin Cancer Res. (2013) 19:5146–57. doi: 10.1158/1078-0432.CCR-13-0017
22. Jesionek-Kupnicka, D, Szybka, M, Potemski, P, Kulczycka-Wojdala, D, Jaskólski, D, Bieńkowski, M, et al. Association of loss of heterozygosity with shorter survival in primary glioblastoma patients. Polish J Pathol. (2013) 4:268–75. doi: 10.5114/PJP.2013.39335
23. Jesionek-Kupnicka, D, Braun, M, Trąbska-Kluch, B, Czech, J, Szybka, M, Szymańska, B, et al. Basic research miR-648 levels inversely correlate with MGMT and TP53 expression in primary glioblastoma patients. Arch Med Sci. (2019) 15:504–12. doi: 10.5114/aoms.2017.69374
24. Liu, YQ, Wu, F, Li, JJ, Li, YF, Liu, X, Wang, Z, et al. Gene expression profiling stratifies IDH-Wildtype glioblastoma with distinct prognoses. Front Oncol. (2019) 9:1433. doi: 10.3389/fonc.2019.01433
25. McNulty, SN, Cottrell, CE, Vigh-Conrad, KA, Carter, JH, Heusel, JW, Ansstas, G, et al. Beyond sequence variation: assessment of copy number variation in adult glioblastoma through targeted tumor somatic profiling. Hum Pathol. (2019) 86:170–81. doi: 10.1016/j.humpath.2018.12.004
26. Motomura, K, Natsume, A, Kishida, Y, Higashi, H, Kondo, Y, Nakasu, Y, et al. Benefits of interferon-β and temozolomide combination therapy for newly diagnosed primary glioblastoma with the unmethylated MGMT promoter. Cancer. (2010) 117:1721–30. doi: 10.1002/cncr.25637
27. Parsons, DW, Jones, S, Zhang, X, Lin, JCH, Leary, RJ, Angenendt, P, et al. An integrated genomic analysis of human glioblastoma multiforme. Science. (2008) 321:1807–12. doi: 10.1126/science.1164382
28. Qin, Z, Zhang, X, Chen, Z, and Liu, N. Establishment and validation of an immune-based prognostic score model in glioblastoma. Int Immunopharmacol. (2020) 85:106636. doi: 10.1016/j.intimp.2020.106636
29. Sim, J, Nam, DH, Kim, Y, Lee, IH, Choi, JW, Sa, JK, et al. Comparison of 1p and 19q status of glioblastoma by whole exome sequencing, array-comparative genomic hybridization, and fluorescence in situ hybridization. Med Oncol. (2018) 35:60. doi: 10.1007/s12032-018-1119-2
30. Stancheva, G, Goranova, T, Laleva, M, Kamenova, M, Mitkova, A, Velinov, N, et al. IDH1/IDH2 but not TP53 mutations predict prognosis in Bulgarian glioblastoma patients. Biomed Res Int. (2014) 2014:654727. doi: 10.1155/2014/654727
31. Stasik, S, Juratli, TA, Petzold, A, Richter, S, Zolal, A, Schackert, G, et al. Exome sequencing identifies frequent genomic loss of TET1 in IDH-wild-type glioblastoma. Neoplasia. (2020) 22:800–8. doi: 10.1016/j.neo.2020.10.010
32. Tabone, T, Abuhusain, HJ, Nowak, AK, Australian Genomics and Clinical Outcome of Glioma (AGOG) NetworkErber, WN, and McDonald, KL. Multigene profiling to identify alternative treatment options for glioblastoma: a pilot study. J Clin Pathol. (2014) 67:550–5. doi: 10.1136/jclinpath-2014-202173
33. Wang, X, Chen, JX, Liu, JP, You, C, Liu, YH, and Mao, Q. Gain of function of mutant TP53 in glioblastoma: prognosis and response to temozolomide. Ann Surg Oncol. (2014) 21:1337–44. doi: 10.1245/s10434-013-3380-0
34. Weller, M, Felsberg, J, Hartmann, C, Berger, H, Steinbach, JP, Schramm, J, et al. Molecular predictors of progression-free and overall survival in patients with newly diagnosed glioblastoma: a prospective translational study of the German glioma network. J Clin Oncol. (2009) 27:5743–50. doi: 10.1200/JCO.2009.23.0805
35. Wong, QHW, Li, KKW, Wang, WW, Malta, TM, Noushmehr, H, Grabovska, Y, et al. Molecular landscape of IDH-mutant primary astrocytoma grade IV/glioblastomas. Mod Pathol. (2021) 34:1245–60. doi: 10.1038/s41379-021-00778-x
36. Yang, K, Jung, SW, Shin, H, Lim, DH, Lee, JI, Kong, DS, et al. Cancer genetic markers according to radiotherapeutic response in patients with primary glioblastoma – Radiogenomic approach for precision medicine. Radiother Oncol. (2019) 131:66–74. doi: 10.1016/j.radonc.2018.11.025
37. Yang, PH, Tao, Y, Luo, J, Paturu, M, Lu, HC, Ramkissoon, S, et al. Multivariate analysis of associations between clinical sequencing and outcome in glioblastoma. Neurooncol Adv. (2022) 4:vdac 002. doi: 10.1093/noajnl/vdac002
38. Amelio, I, and Melino, G. Context is everything: extrinsic signalling and gain-of-function p53 mutants. Cell Death Discov. (2020) 6:16. doi: 10.1038/s41420-020-0251-x
39. Wang, H, Guo, M, Wei, H, and Chen, Y. Targeting p53 pathways: mechanisms, structures, and advances in therapy. Signal Transduct Target Ther. (2023) 8:92. doi: 10.1038/s41392-023-01347-1
40. Noor, H, Briggs, NE, McDonald, KL, Holst, J, and Vittorio, O. TP53 mutation is a prognostic factor in lower grade glioma and may influence chemotherapy efficacy. Cancers. (2021) 13:5362. doi: 10.3390/cancers13215362
41. Wang, X, Chen, JX, Liu, YH, You, C, and Mao, Q. Mutant TP53 enhances the resistance of glioblastoma cells to temozolomide by up-regulating O (6)-methylguanine DNA-methyltransferase. Neurol Sci. (2013) 34:1421–8. doi: 10.1007/s10072-012-1257-9
42. Pradhan, MR, Siau, JW, Kannan, S, Nguyen, MN, Ouaray, Z, Kwoh, CK, et al. Simulations of mutant p53 DNA binding domains reveal a novel druggable pocket. Nucleic Acids Res. (2019) 47:1637–52. doi: 10.1093/nar/gky1314
43. Silva, JL, Foguel, D, Ferreira, VF, Vieira, TCRG, Marques, MA, Ferretti, GDS, et al. Targeting biomolecular condensation and protein aggregation against cancer. Chem Rev. (2023) 123:9094–138. doi: 10.1021/acs.chemrev.3c00131
44. Pedrote, MM, Motta, MF, Ferretti, GDS, Norberto, DR, Spohr, TCLS, Lima, FRS, et al. Oncogenic gain of function in glioblastoma is linked to mutant p53 amyloid oligomers. iScience. (2020) 23:100820. doi: 10.1016/j.isci.2020.100820
45. Petronilho, EC, Pedrote, MM, Marques, MA, Passos, YM, Mota, MF, Jakobus, B, et al. Phase separation of p53 precedes aggregation and is affected by oncogenic mutations and ligands. Chem Sci. (2021) 12:7334–49. doi: 10.1039/d1sc01739j
46. Pellot Ortiz, KI, Rechberger, JS, Nonnenbroich, LF, Daniels, DJ, and Sarkaria, JN. MDM2 inhibition in the treatment of glioblastoma: from concept to clinical investigation. Biomedicines. (2023) 11:1879. doi: 10.3390/biomedicines11071879
47. Phoa, AF, Recasens, A, Gurgis, FMS, Betts, TA, Menezes, SV, Chau, D, et al. MK2 inhibition induces p53-dependent senescence in glioblastoma cells. Cancers (Basel). (2020) 12:654. doi: 10.3390/cancers12030654
Keywords: glioblastoma, TP53 , brain tumors, survival, meta-analysis, prognosis
Citation: Esperante D, Galicia KD, Rivas-Cuervo KG, Cacho-Díaz B, Trejo-Becerril C, Taja-Chayeb L, Aboud O, Carlos-Escalante JA and Wegman-Ostrosky T (2024) TP53 oncogenic variants as prognostic factors in individuals with glioblastoma: a systematic review and meta-analysis. Front. Neurol. 15:1490246. doi: 10.3389/fneur.2024.1490246
Edited by:
Félix Javier Jiménez-Jiménez, Hospital Universitario del Sureste, SpainReviewed by:
Guilherme A. P. de Oliveira, Federal University of Rio de Janeiro, BrazilVigyasa Singh, University of Arizona, United States
Copyright © 2024 Esperante, Galicia, Rivas-Cuervo, Cacho-Díaz, Trejo-Becerril, Taja-Chayeb, Aboud, Carlos-Escalante and Wegman-Ostrosky. This is an open-access article distributed under the terms of the Creative Commons Attribution License (CC BY). The use, distribution or reproduction in other forums is permitted, provided the original author(s) and the copyright owner(s) are credited and that the original publication in this journal is cited, in accordance with accepted academic practice. No use, distribution or reproduction is permitted which does not comply with these terms.
*Correspondence: Talia Wegman-Ostrosky, dGFsaWF3QGdtYWlsLmNvbQ==
†These authors have contributed equally to this work