- 1Radiography Center - Interventional Catheter Room, Yixing People’s Hospital, Yixing, China
- 2Department of Medicine, Xinglin College, Nantong University, Nantong, China
- 3Nanjing Drum Tower Hospital Clinical College of Nanjing Medical University, Nanjing, China
- 4Department of Emergency Medicine, Yixing People’s Hospital, Yixing, China
Purpose: This research employed Mendelian randomization (MR) methods to explore whether metabolites are causally associated with embolic stroke of undetermined source (ESUS).
Methods: Genome-Wide Association Study (GWAS) data regarding metabolites and ESUS were downloaded from the database. Metabolites were employed as exposure factors, ESUS served as the outcome variable, and single nucleotide polymorphisms (SNPs) exhibiting significant association with ESUS were chosen as instrumental variables. The causal association between exposure factor metabolites and the outcome variable ESUS was assessed using two methods: MR-Egger regression and inverse variance-weighted (IVW) analysis.
Results: A causal relationship was observed between X-11593--O-methylascorbate* and ESUS, indicating a protective factor. Moreover, a causal relationship was identified between cholesterol esters in large very-low-density lipoprotein (VLDL), cholesterol esters in medium low-density lipoprotein (LDL), concentration of medium LDL particles, phospholipids in medium LDL, phenylalanine, total cholesterol in small LDL, total lipids in medium LDL and ESUS, representing risk factor. Funnel plots exhibited a symmetrical distribution of SNPs, while pleiotropic tests (p > 0.05) and leave-one-out tests indicated that the results were relatively stable.
Conclusion: Metabolites are causally associated with ESUS. LDL and VLDL-related metabolites are identified as risk factors for ESUS.
1 Introduction
Stroke is a serious disease marked by high morbidity, recurrence, disability, and mortality rates. It poses significant health risks and economic burdens. The main pathological types are ischemic and hemorrhagic stroke, with embolic stroke of undetermined source (ESUS) accounting for about 17% of ischemic stroke patients (1). ESUS refers to non-lacunar ischemic stroke where intracranial or extracranial vascular stenosis and cardiogenic emboli are excluded (2). Currently, effective treatment options for ESUS remain limited. Therefore, understanding the etiology and risk factors of ESUS and identifying potential diagnostic treatments are crucial for public health (3).
Metabolites are intermediates or end products of metabolic reactions and their levels can be impacted by numerous factors, including diet, genetics, gut microbiota, lifestyle, and disease (4). Through metabolite analysis, the mechanisms of disease development can be understood, targets for disease intervention can be sought, disease risk can be mitigated, and stratified prevention and management can be achieved (5). Currently, the causal function of metabolites in disease etiology has been demonstrated, providing new ideas for diagnosis and treatment. Additionally, many metabolites exhibit high heritability, making human genetics a method for assessing the impact of metabolites on disease outcomes.
Building on this understanding, Mendelian Randomization (MR), a causal inference method, has recently gained widespread usage in the field of epidemiology. This approach relies on genome-wide association study (GWAS) data, utilizing genetic variants as instrumental variables (IVs) in order to examine the impact of exposure on disease outcomes (6). Due to the random assignment of genetic variation at conception, this approach can break the confounding of most risk factors and reduce the tendency of confounding to bias the results, thus achieving the effect of simulating randomized controlled trials. In this research, MR analysis methods utilizing GWAS data were employed for the investigation of the causal association between metabolites and ESUS. The aim was to present novel ideas and directions for the future diagnosis and treatment of ESUS.
2 Methodology
2.1 Data selection
In this study, metabolite-related GWAS data were used as exposure factors. Single nucleotide polymorphisms (SNPs) exhibiting significant association with ESUS were employed as IVs, while ESUS-associated data were used as outcome variables.
2.2 Data sources
Metabolite-related data and GWAS data for ESUS in this study were derived from databases encompassing https://gwas.mrcieu.ac.uk/, https://www.ebi.ac.uk/gwas/, and https://www.finngen.fi/en. Metabolite-related GWAS data utilized in this research mainly included met-a-536, met-c-881, met-c-905, met-c-906, met-c-907, met-c-908, met-c-919, and met-c-924. GWAS data for ESUS was I9_STR_EMBOLIC. As this study relied on publicly available data, ethical approval or consent was not required.
2.3 Relevance analysis
MR Assay meets the following three hypothetical premiums:
① Relevance hypothesis: significant association between IVs and metabolites (exposure factors);
② Independence hypothesis: lack of association between IVs and ESUS (outcome variable) and other confounding factors;
③ Exclusivity hypothesis: IVs can only influence ESUS (outcome variable) by affecting metabolites (exposure factors) and cannot influence ESUS through other pathways (7).
SNPs strongly associated with exposure factors were selected as IVs and filtered at the condition of p < 5e-08.
2.4 Linkage disequilibrium
Linkage disequilibrium (LD) is the phenomenon where genetic variants located close to each other on a chromosome tend to be inherited together more often than would be expected by chance. This means that the likelihood of specific alleles (gene variants) from two or more loci appearing together on a single chromosome is greater than if the alleles were distributed randomly (8). To mitigate potential bias from LD, the screening criteria for single nucleotide polymorphisms (SNPs) are outlined below:
1. Kb > 10,000;
2. r2 < 0.001.
Here, Kb refers to the range of regions in LD. r2 is evaluated between 0 and 1, where r2 = 1 denotes a complete LD relationship between two SNPs, while r2 = 0 suggests a complete linkage equilibrium relationship between two SNPs, indicating the random assignment of these two SNPs (9).
2.5 Removing weak tool variables
To avoid bias for weak IVs, the F-statistic was utilized to determine their strength. An IV with an F-value <10 was defined as weak, while an F-value >10 was considered a non-weak IV (10). The strength of selected individual tool variables was evaluated by formula ( ), with N representing the total sample size of GWAS data exposed, K representing the number of screened IVs, and R2 representing the proportion of exposures explained by IV. R2 is calculated as R2 = 2 × (1-MAF) × MAF × (β/SD)2, where MAF represents the minor allele frequency, β indicates the effect size of the allele, and SD denotes the standard deviation (11).
2.6 Mendelian randomization analysis
MR-Egger regression and inverse variance-weighted (IVW) analysis were employed to reveal the causal association between exposure factor metabolites and outcome variable ESUS. IVW was utilized as the main method to evaluate the reliability of the findings, defining p < 0.05 as a positive result (12). The R package “TwoSampleMR” was utilized for the visualization of MR results, including forest plots, perceptual analysis plots, and scatter plots.
2.7 Sensitivity analyses
IVW and MR-Egger tests were employed to assess the heterogeneity. If the p-value was <0.05, it indicates heterogeneity in the study. IVs are considered pleiotropic if they influence outcome occurrence through factors other than exposure factors (13). Pleiotropy can lead to independence and exclusivity assumptions that do not hold. The MR-Egger intercept test can detect the pleiotropy of the data and assess the robustness of the results (14). Data were considered pleiotropic on p-value <0.05. Sensitivity analysis was conducted using the “leave-one-out method,” a systematic process that involves the step-by-step removal of individual SNP results. This method evaluates the combined effect of the remaining SNPs, aiming to examine whether a single SNP impacts the causal effect. Funnel plots were drawn using R language to observe whether SNPs were symmetrical and thereby determine the reliability of the findings.
2.8 Statistical methods and software
In this study, R 4.3.1 software1 and strawberry-perl 5.32.1.1 software2 were utilized to conduct the relationship analysis of MR. Statistical significance was determined at p < 0.05.
3 Results
3.1 Screening instrumental variables
The selection of SNPs strongly associated with exposure and outcome factors was carried out by utilizing the R packages, including “dplyr,” “gwasglue,” “tidyr,” and “VariantAnnotation”. The filtration condition was set at p < 5e-08. A total of 8 SNP datasets strongly linked with metabolites were identified:
met-a-536 (X-11593--O-methylascorbate*),
met-c-881 (Cholesterol esters in large very-low-density lipoprotein (VLDL)),
met-c-906 (Total lipids in medium LDL),
met-c-907 (Concentration of medium LDL particles),
met-c-908 (Phospholipids in medium LDL),
met-c-919 (Phenylalanine), and.
met-c-924 (Total cholesterol in small LDL) (Figure 1; Table 1).
3.2 Screening instrumental variables
SNPs strongly associated with exposure and outcome factors were selected employing the R packages, such as “dplyr,” “gwasglue,” “tidyr,” and “VariantAnnotation” with filtration condition of p < 5e-08. Among them, 6 SNPs in met-a-536 were strongly associated with ESUS; 25 SNPs in met-c-881 were strongly associated with ESUS; 25 SNPs in met-c-905 were strongly associated with ESUS; 23 SNPs in met-c-906 were strongly associated with ESUS; 23 SNPs in met-c-907 were strongly associated with ESUS; 20 SNPs in met-c-908 were strongly associated with ESUS; 4 SNPs in met-c-919 were strongly associated with ESUS; and 21 SNPs in met-c-924 were strongly associated with ESUS. F-value calculations and removal of confounding factors were performed for the selected SNPs by employing the R package MR. As a result, no weak IVs and confounding factors were identified (Table 2).
3.3 Findings of Mendelian randomization analysis
The causal association between exposure factors and outcomes was assessed by employing MR-Egger regression and IVW analysis. Visualization of MR results was performed by utilizing the R package “TwoSampleMR,” including forest plots and scatter plots. The findings exhibited a causal relationship between X-11593--O-methylascorbate (p = 0.029, OR = 0.222, 95% CI: 0.058 ~ 0.858) and ESUS, indicating a protective factor. Cholesterol esters in large VLDL (p = 0.046, OR = 1.184, 95% CI: 1.003 ~ 1.398), cholesterol esters in medium LDL (p = 0.030, OR = 1.205, 95% CI: 1.018 ~ 1.426), total lipids in medium LDL (p = 0.041, OR = 1.204, 95% CI: 1.008 ~ 1.438), the concentration of medium LDL particles (p = 0.042, OR = 1.203, 95% CI: 1.006 ~ 1.437), phospholipids in medium LDL (p = 0.022, OR = 1.239, 95% CI: 1.031 ~ 1.489), phenylalanine (p = 0.046, OR = 1.749, 95% CI: 1.009 ~ 3.032), and total cholesterol in small LDL (p = 0.024, OR = 1.225, 95% CI: 1.028 ~ 1.461) were causally related to ESUS and were identified as risk factors (Figures 2A–G).
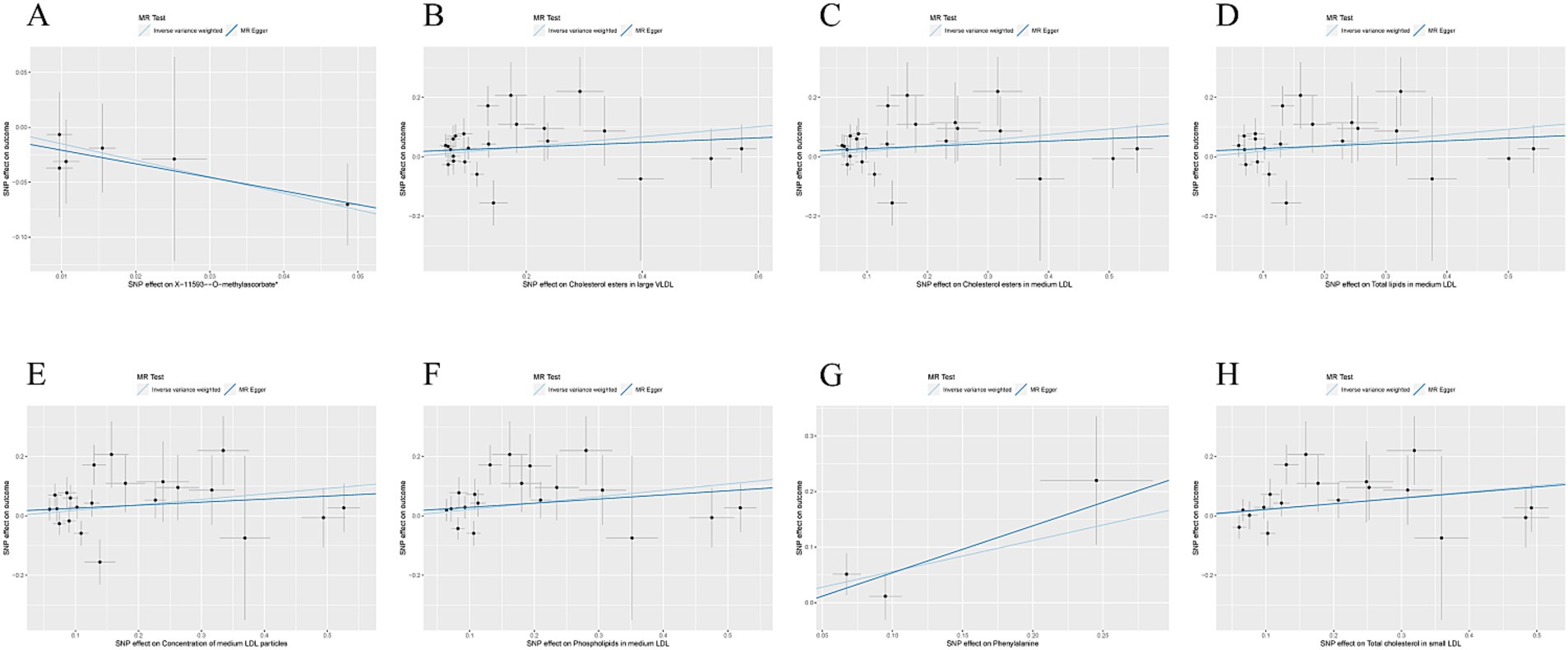
Figure 2. (A) Scatter plot of the causal relationship between X-11593--O-methylascorbate* and ESUS, mainly evaluated by IVW method; (B) Scatter plot of the causal relationship between Cholesterol esters in large VLDL and ESUS, mainly evaluated by IVW method; (C) Scatter plot of the causal relationship between Cholesterol esters in medium LDL and ESUS, mainly evaluated by IVW method; (D) Scatter plot of the causal relationship between Total lipids in medium LDL and ESUS, mainly evaluated by IVW method; (E) Scatter plot of the causal relationship between Concentration of medium LDL particles and ESUS, mainly evaluated by IVW method; (F) Scatter plot of the causal relationship between Phospholipids in medium LDL and ESUS, mainly evaluated by IVW method; (G) Scatter plot of the causal relationship between Phenylalanine and ESUS, mainly evaluated by IVW method; (H) Scatter plot of the causal relationship between Total cholesterol in small LDL and ESUS, mainly evaluated by IVW method.
3.4 Sensitivity analyses
IVW methods and MR-Egger regression were used for analyzing the heterogeneity. The results revealed that all datasets had p > 0.05, and no heterogeneity was observed. Egger regression orientation analysis was further used to analyze whether there was orientation level multidirectionality. The results showed that all datasets had p > 0.05, and no orientation-level multidirectionality was observed (Table 3). The funnel plot showed that SNPs were symmetrically distributed and the results obtained were relatively stable (Figure 3). The leave-one-out method was used to eliminate SNPs one by one and observe any changes in the effect values. The results indicated that no strong influential SNP loci were identified (Figure 4).
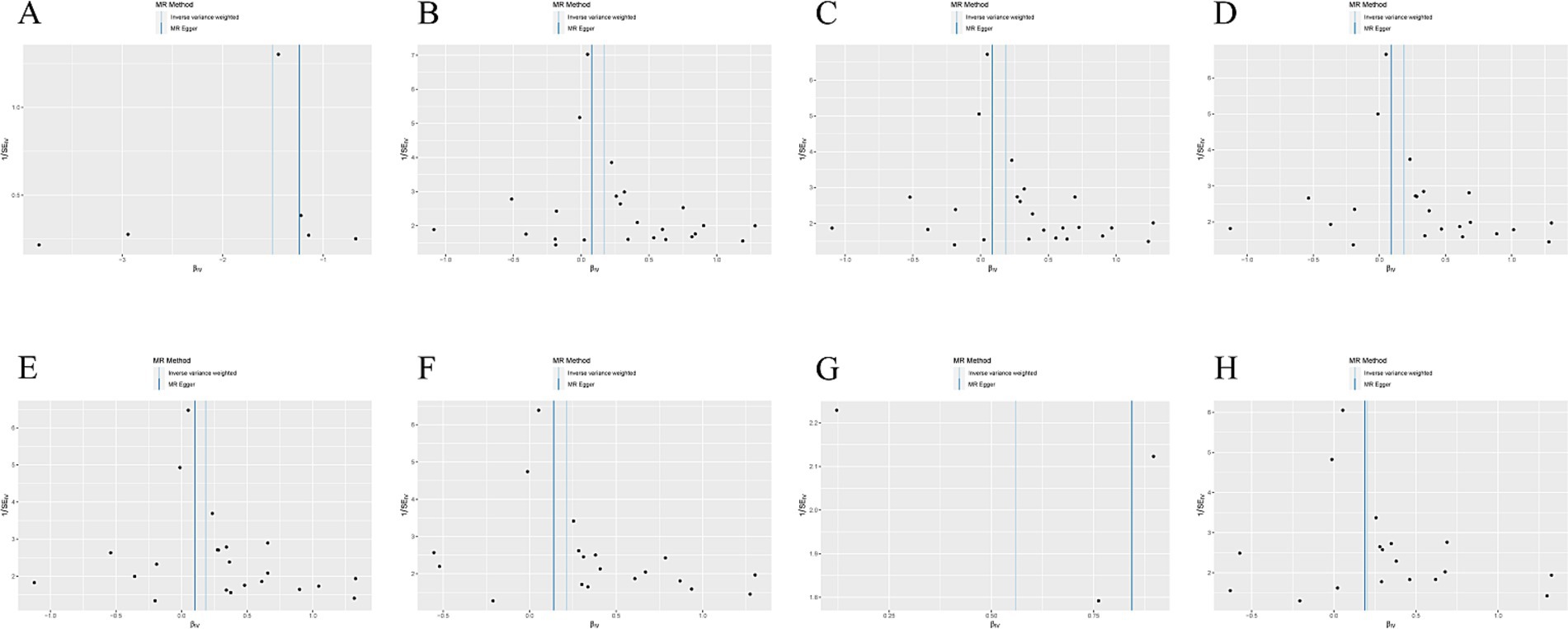
Figure 3. (A) Funnel plot of the causal relationship between X-11593--O-methylascorbate* and ESUS, mainly evaluated by IVW method; (B) Funnel plot of the causal relationship between Cholesterol esters in large VLDL and ESUS, mainly evaluated by IVW method; (C) Funnel plot of the causal relationship between Cholesterol esters in medium LDL and ESUS, mainly evaluated by IVW method; (D) Funnel plot of the causal relationship between Total lipids in medium LDL and ESUS, mainly evaluated by IVW method; (E) Funnel plot of the causal relationship between Concentration of medium LDL particles and ESUS, mainly evaluated by IVW method; (F) Funnel plot of the causal relationship between Phospholipids in medium LDL and ESUS, mainly evaluated by IVW method; (G) Funnel plot of the causal relationship between Phenylalanine and ESUS, mainly evaluated by IVW method; (H) Funnel plot of the causal relationship between Total cholesterol in small LDL and ESUS, mainly evaluated by IVW method.
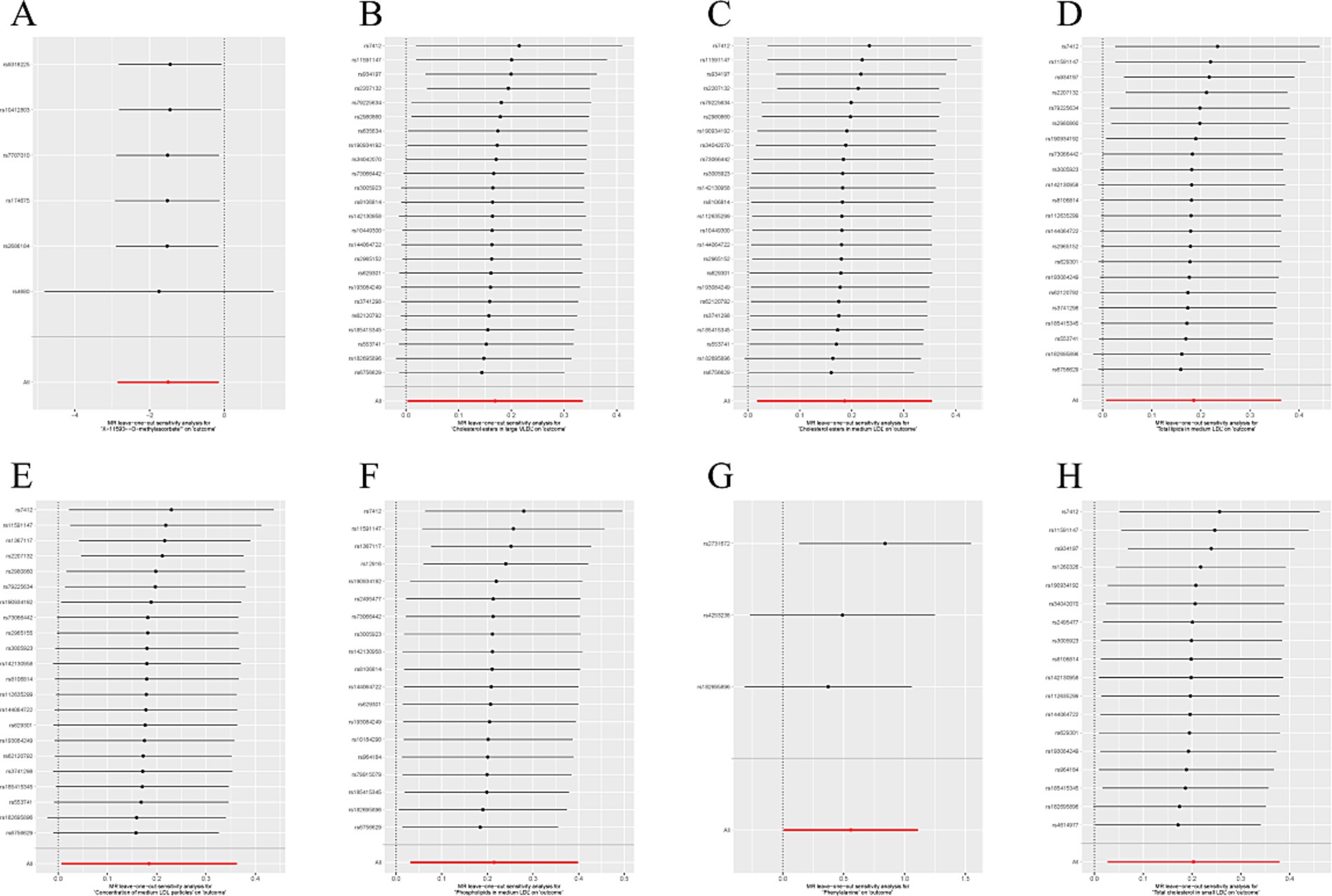
Figure 4. (A) “Leave one method” forest map of the causal relationship between X-11593--O-methylascorbate* and ESUS, mainly evaluated by IVW method; (B) “Leave one method” forest map of the causal relationship between Cholesterol esters in large VLDL and ESUS, mainly evaluated by IVW method; (C) “Leave one method” forest map of the causal relationship between Cholesterol esters in medium LDL and ESUS, mainly evaluated by IVW method; (D) “Leave one method” forest map of the causal relationship between Total lipids in medium LDL and ESUS, mainly evaluated by IVW method; (E) “Leave one method” forest map of the causal relationship between Concentration of medium LDL particles and ESUS, mainly evaluated by IVW method; (F) “Leave one method” forest map of the causal relationship between Phospholipids in medium LDL and ESUS, mainly evaluated by IVW method; (G) “Leave one method” forest map of the causal relationship between Phenylalanine and ESUS, mainly evaluated by IVW method; (H) “Leave one method” forest map of the causal relationship between Total cholesterol in small LDL and ESUS, mainly evaluated by IVW method.
4 Discussion
In this study, public large-sample GWAS data were employed for analyzing the potential causal association between metabolites and ESUS using R language. X-11593--O-methylascorbate* (IWV, p = 0.029, OR = 0.222, 95% CI: 0.058 ~ 0.858) exhibited a causal relation with ESUS and was identified as a protective factor. Cholesterol esters in large VLDL (IWV, p = 0.046, OR = 1.184, 95% CI: 1.003 ~ 1.398), cholesterol esters in medium LDL (IWV, p = 0.030, OR = 1.205, 95% CI: 1.018 ~ 1.426), total lipids in medium LDL (IWV, p = 0.041, OR = 1.204, 95% CI: 1.008 ~ 1.438), concentration of medium LDL (IWV, p = 0.042, OR = 1.203, 95% CI: 1.006 ~ 1.437), phospholipids in medium LDL (IWV, p = 0.022, OR = 1.239, OR = 1.239, 95% CI: 1.031 ~ 1.489), phenylalanine (IWV, p = 0.046, OR = 1.749, 95% CI: 1.009 ~ 3.032), and total cholesterol in small LDL (IWV, p = 0.024, OR = 1.225, 95% CI: 1.028 ~ 1.461) were causally associated with ESUS and were identified as risk factors.
Stroke represents a serious condition that is categorized into ischemic and hemorrhagic strokes. Among them, ischemic stroke without a clear cause is called cryptogenic stroke, with ESUS being one of its subtypes (15, 16). Alterations in metabolite levels are regarded as the primary response of biological systems to various biological events. They can amplify small changes in genes and proteins and, more directly, precisely, and sensitively, reflect the physiological and pathological conditions of the body. Consequently, metabolites are associated with numerous human diseases (17). Recent studies indicate that metabolites are related to stroke and play crucial roles in its onset and progression. Inhibition of metabolite levels has been shown to delay the occurrence and development of ESUS.
This study presents several advantages. Firstly, it utilized large sample GWAS data, ensuring the reliability of the results. Secondly, SNPs were carefully screened through association analysis, LD analysis, and the removal of weak instrumental variables and confounders, effectively mitigating potential bias (18, 19). Thirdly, the symmetrical distribution of SNPs was confirmed by funnel plots, and the relatively stable results were supported by pleiotropy and leave-one-out tests. Lastly, the exploration of the molecular mechanisms by investigating the causal association between metabolites and ESUS, with metabolites as exposure factors, holds significant clinical relevance (20).
This study has several limitations. Although MR-Egger regression and IVW methods indicated no significant heterogeneity between the analyses (p > 0.05), potential sources of heterogeneity may arise from variations in instrumental variables (IVs), including differences in analytical platforms, experimental designs, and population characteristics, necessitating a more detailed exploration of these sources to enhance the validity of the findings. Additionally, the GWAS data are predominantly derived from European populations, resulting in a lack of representation from African and Asian cohorts; thus, future research should include diverse populations for a more comprehensive understanding of the associations examined. The number of IVs for SNPs within each dataset is relatively limited, indicating a need for larger sample sizes to identify additional SNPs as IVs. While this research utilized Mendelian Randomization (MR) to analyze the causal relationship between metabolites and ESUS, no mechanistic studies were conducted to elucidate the underlying biological processes, which could provide valuable insights. Furthermore, the findings may be influenced by residual confounding factors not fully accounted for in the analyses, and it is essential to consider these impacts in future investigations. Lastly, the analytical methods employed may have limitations in detecting all possible causal relationships, warranting the exploration of alternative methodologies, such as sensitivity analyses or different statistical approaches, to validate the robustness of the results.
5 Conclusion
This research evaluated the potential causal effect of metabolites on embolic stroke of undetermined source (ESUS) through Mendelian Randomization (MR) analysis. The findings revealed that X-11593-O-methylascorbate is causally related to ESUS, functioning as a protective factor. Conversely, several metabolites, including cholesterol esters in large very-low-density lipoprotein (VLDL), cholesterol esters in medium low-density lipoprotein (LDL), total lipids in medium LDL, concentration of medium LDL particles, phospholipids in medium LDL, phenylalanine, and total cholesterol in small LDL, were identified as risk factors associated with ESUS.
While these outcomes provide a theoretical basis for further investigations into the dynamics of metabolite levels during ESUS, it is crucial to explore the underlying biological mechanisms connecting these metabolites to ESUS (21). Future studies should incorporate experimental approaches to better understand how specific metabolites influence the pathophysiology of ESUS (22, 23). For example, in vitro studies could be conducted to investigate the effects of these metabolites on endothelial function, coagulation pathways, and inflammatory responses, which are critical in the development of ESUS. Understanding these mechanisms would not only clarify the causal relationships identified but also inform clinical interventions aimed at modifying metabolite levels to reduce the risk of ESUS. In addition to exploring biological mechanisms, it is essential to strengthen the clinical application prospects of the findings (24, 25). The study suggests that managing metabolite levels may help reduce the incidence of ESUS; however, the discussion lacks a thorough exploration of how these findings can be translated into clinical practice. Specifically, there is a need to elaborate on potential directions for drug development and personalized treatment strategies based on the identified metabolites (26).
In summary, while this study lays a solid foundation for understanding the causal relationships between metabolites and ESUS, future research should prioritize elucidating the underlying biological mechanisms and translating these findings into actionable clinical strategies. By doing so, it may be possible to not only mitigate the risk of ESUS but also pave the way for innovative therapeutic approaches that harness the power of metabolite modulation in clinical practice.
Data availability statement
The datasets presented in this study can be found in online repositories. The names of the repository/repositories and accession number(s) can be found in the article/supplementary material.
Ethics statement
Ethical review and approval was not required for the study on human participants in accordance with the local legislation and institutional requirements. Written informed consent from the patients/participants or patients/participants' legal guardian/next of kin was not required to participate in this study in accordance with the national legislation and the institutional requirements.
Author contributions
YH: Writing – original draft, Writing – review & editing. ZC: Conceptualization, Data curation, Formal analysis, Writing – original draft. JR: Data curation, Formal analysis, Investigation, Supervision, Writing – review & editing. YW: Writing – review & editing. KZ: Project administration, Resources, Visualization, Writing – original draft. QZ: Writing – original draft, Writing – review & editing.
Funding
The author(s) declare financial support was received for the research, authorship, and/or publication of this article. This study was supported by Key Project of Nanjing Medical Science and Technology Program, no. ZKX21015.
Conflict of interest
The authors declare that the research was conducted in the absence of any commercial or financial relationships that could be construed as a potential conflict of interest.
Publisher’s note
All claims expressed in this article are solely those of the authors and do not necessarily represent those of their affiliated organizations, or those of the publisher, the editors and the reviewers. Any product that may be evaluated in this article, or claim that may be made by its manufacturer, is not guaranteed or endorsed by the publisher.
Footnotes
References
1. Ntaios, G. Embolic stroke of undetermined source: JACC review topic of the week. J Am Coll Cardiol. (2020) 75:333–40. doi: 10.1016/j.jacc.2019.11.024
2. Hart, RG, Diener, HC, Coutts, SB, Easton, JD, Granger, CB, O’Donnell, MJ, et al. Embolic strokes of undetermined source: the case for a new clinical construct. Lancet Neurol. (2014) 13:429–38. doi: 10.1016/S1474-4422(13)70310-7
3. Johansen, MC, Geocadin, RG, and Kamel, H. Embolic stroke of unknown source clinical trials and advances in research. Ann Neurol. (2020) 88:462–3. doi: 10.1002/ana.25825
4. Bar, N, Korem, T, Weissbrod, O, Zeevi, D, Rothschild, D, Leviatan, S, et al. A reference map of potential determinants for the human serum metabolome. Nature. (2020) 588:135–40. doi: 10.1038/s41586-020-2896-2
5. Pietzner, M, Stewart, ID, Raffler, J, Khaw, KT, Michelotti, GA, Kastenmüller, G, et al. Plasma metabolites to profile pathways in noncommunicable disease multimorbidity. Nat Med. (2021) 27:471–9. doi: 10.1038/s41591-021-01266-0
6. Lin, LJ, Wei, YY, Zhang, RY, and Chen, F. Application of mendelian randomization methods in causal inference of observational study. Zhonghua Yu fang Yi Xue Za Zhi. (2019) 53:619–24. doi: 10.3760/cma.j.issn.0253-9624.2019.06.015
7. Jiang, L, Sun, YQ, Brumpton, BM, Langhammer, A, Chen, Y, and Mai, XM. Body mass index and incidence of lung cancer in the HUNT study: using observational and Mendelian randomization approaches. BMC Cancer. (2022) 22:1152. doi: 10.1186/s12885-022-10215-0
8. Gui, H, Tang, WHW, Francke, S, Li, J, She, R, Bazeley, P, et al. Common variants on FGD5 increase Hazard of mortality or Rehospitalization in patients with heart failure from the ASCEND-HF trial. Circ Heart Fail. (2023) 16:e010438. doi: 10.1161/CIRCHEARTFAILURE.122.010438
9. Nalls, MA, Blauwendraat, C, Vallerga, CL, Heilbron, K, Bandres-Ciga, S, Chang, D, et al. Identification of novel risk loci, causal insights, and heritable risk for Parkinson's disease: a meta-analysis of genome-wide association studies. Lancet Neurol. (2019) 18:1091–102. doi: 10.1016/S1474-4422(19)30320-5
10. Wientjes, YC, Veerkamp, RF, and Calus, MP. The effect of linkage disequilibrium and family relationships on the reliability of genomic prediction. Genetics. (2013) 193:621–31. doi: 10.1534/genetics.112.146290
11. Wan, B, Ma, N, Zhou, Z, and Lu, W. Modifiable risk factors that mediate the effect of educational attainment on the risk of stroke: a network Mendelian randomization study. Mol Brain. (2023) 16:39. doi: 10.1186/s13041-023-01030-0
12. Pairo-Castineira, E, Rawlik, K, Bretherick, AD, Qi, T, Wu, Y, Nassiri, I, et al. GWAS and meta-analysis identifies 49 genetic variants underlying critical COVID-19. Nature. (2023) 617:764–8. doi: 10.1038/s41586-023-06034-3
13. Morales Berstein, F, McCartney, DL, Lu, AT, Tsilidis, KK, Bouras, E, Haycock, P, et al. Assessing the causal role of epigenetic clocks in the development of multiple cancers: a Mendelian randomization study. eLife. (2022) 11:e75374. doi: 10.7554/eLife.75374
14. Alhathli, E, Julian, T, Girach, ZUA, Thompson, AAR, Rhodes, C, Gräf, S, et al. Mendelian randomization study with clinical follow-up links metabolites to risk and severity of pulmonary arterial hypertension. J Am Heart Assoc. (2024) 13:e032256. doi: 10.1161/JAHA.123.032256
15. Navi, BB, Kasner, SE, Elkind, MSV, Cushman, M, Bang, OY, and DeAngelis, LM. Cancer and embolic stroke of undetermined source. Stroke. (2021) 52:1121–30. doi: 10.1161/STROKEAHA.120.032002
16. Diener, HC, Easton, JD, Hart, RG, Kasner, S, Kamel, H, and Ntaios, G. Review and update of the concept of embolic stroke of undetermined source. Nat Rev Neurol. (2022) 18:455–65. doi: 10.1038/s41582-022-00663-4
17. Wang, T, Zhang, Y, Wu, C, Yang, H, and Luo, Z. Causality of blood metabolites and metabolic pathways on Graves' disease and Graves' ophthalmopathy: a two-sample Mendelian randomization study. Endocrine. (2024) 85:786–93. doi: 10.1007/s12020-024-03761-z
18. Huang, Y, Chen, S, Zhang, E, and Han, L. Causal relationship between genetically determined plasma metabolites and stroke: a two sample Mendelian randomization study. Prog Neuro-Psychopharmacol Biol Psychiatry. (2024) 135:111133. doi: 10.1016/j.pnpbp.2024.111133
19. Lin, Z, and Pan, W. A robust cis-Mendelian randomization method with application to drug target discovery. Nat Commun. (2024) 15:6072. doi: 10.1038/s41467-024-50385-y
20. Wojcik, MH, Lemire, G, Berger, E, Zaki, MS, Wissmann, M, Win, W, et al. Genome sequencing for diagnosing rare diseases. N Engl J Med. (2024) 390:1985–97. doi: 10.1056/NEJMoa2314761
21. Koh, JH, Lim, LKE, Tan, YK, Goh, C, Teo, YH, Ho, JSY, et al. Assessment of left atrial fibrosis by cardiac magnetic resonance imaging in ischemic stroke patients without atrial fibrillation: a systematic review and Meta-analysis. J Am Heart Assoc. (2024) 13:e033059. doi: 10.1161/JAHA.123.033059
22. Sposato, LA, Sur, NB, Katan, M, Johansen, MC, de Marchis, GM, Caso, V, et al. Embolic stroke of undetermined source: new data and new controversies on cardiac monitoring and anticoagulation. Neurology. (2024) 103:e209535. doi: 10.1212/WNL.0000000000209535
23. Xie, X, Jing, J, Meng, X, Johnston, SC, Bath, PM, Li, Z, et al. Dual antiplatelet therapy after embolic stroke of undetermined source: a subgroup analysis of the CHANCE-2 trial. Stroke. (2024) 55:1739–47. doi: 10.1161/STROKEAHA.124.046834
24. Rizzo, AC, Schwarz, G, Bonelli, A, di Pietro, A, di Pietro, M, Aruta, F, et al. The role of atrial Cardiopathy as a potential cause of embolic stroke of undetermined source. J Stroke. (2024) 26:330–4. doi: 10.5853/jos.2024.00031
25. Ntaios, G, Baumgartner, H, Doehner, W, Donal, E, Edvardsen, T, Healey, JS, et al. Embolic strokes of undetermined source: a clinical consensus statement of the ESC Council on stroke, the European Association of Cardiovascular Imaging and the European heart rhythm association of the ESC. Eur Heart J. (2024) 45:1701–15. doi: 10.1093/eurheartj/ehae150
Keywords: metabolite, Mendelian randomization, embolic stroke of undetermined source, genome-wide association study, inverse variance-weighted
Citation: Hang Y, Chen Z, Ren J, Wang Y, Zhu K and Zhu Q (2024) Causal relationship between metabolites and embolic stroke: based on Mendelian randomization and metabolomics. Front. Neurol. 15:1460852. doi: 10.3389/fneur.2024.1460852
Edited by:
Patricia Martínez Sánchez, Torrecárdenas University Hospital, SpainReviewed by:
Peiwen Wang, Capital Medical University, ChinaHongliang Wang, Anhui Medical University, China
Jie Shen, Anhui University, China
Copyright © 2024 Hang, Chen, Ren, Wang, Zhu and Zhu. This is an open-access article distributed under the terms of the Creative Commons Attribution License (CC BY). The use, distribution or reproduction in other forums is permitted, provided the original author(s) and the copyright owner(s) are credited and that the original publication in this journal is cited, in accordance with accepted academic practice. No use, distribution or reproduction is permitted which does not comply with these terms.
*Correspondence: Qianhong Zhu, yxhpzqh@163.com