- 1China National Clinical Research Center for Neurological Diseases, Beijing Tiantan Hospital, Capital Medical University, Beijing, China
- 2Department of Neurology, Beijing Tiantan Hospital, Capital Medical University, Beijing, China
- 3Pharmacology Department, School of Biomedical Sciences, Faculty of Medicine and Health, UNSW Sydney, Sydney, NSW, Australia
- 4Department of Neurobiology, Neurology and Geriatrics, National Clinical Research Center for Geriatric Disorders, Xuanwu Hospital of Capital Medical University, Beijing, China
Background: We have shown that genetic factors associating with motor progression of Parkinson’s disease (PD), but their roles in cognitive function is poorly understood. One reason is that while cognitive performance in PD can be evaluated by various cognitive scales, there is no definitive guide indicating which tool performs better.
Methods: Data were obtained from the Parkinson’s Progression Markers Initiative, where cognitive performance was assessed using five cognitive screening tools, including Symbol Digit Modalities Test (SDMT), Montreal Cognitive Assessment, Benton Judgment of Line Orientation, Modified Semantic Fluency Test, and Letter Number Sequencing Test, at baseline and subsequent annual follow-up visit for 5 years. Genetic data including ApoE and other PD risk genetic information were also obtained. We used SPSS-receiver operating characteristic and ANOVA repeated measures to evaluate which cognitive assessment is the best reflecting cognitive performance in PD at early stage and over time. Logistic regression analyses were used to determine the genetic associations with the rapidity of cognitive decline in PD.
Results: SDMT performed better in detecting mild cognitive impairment at baseline (AUC = 0.763), and SDMT was the only tool showing a steady cognitive decline during longitudinal observation. Multigenetic factors significantly associated with cognitive impairment at early stage of the disease (AUC = 0.950) with IP6K2 rs12497850 more evident, and a significantly faster decline (AUC = 0.831) within 5 years after motor onset, particularly in those carrying FGF20 rs591323.
Conclusion: SDMT is a preferable cognitive assessment tool for PD and genetic factors synergistically contribute to the cognitive dysfunction in PD.
1 Introduction
Parkinson’s disease (PD) is the second most common neurodegenerative disease after Alzheimer’s disease (AD), which affects 1% of people over 65 years and 3% of those over 80 years old (1). Apart from typical motor symptoms including rest tremor, bradykinesia, and rigidity, non-motor symptoms such as cognitive impairment, autonomic dysfunction, hyposmia, and rapid eye movement sleep behavior disorder (RBD) are also important clinical features of PD. Cognitive impairment is one of the most common non-motor symptoms, and its incidence in patients with PD is 2.5 to 6 times higher than that of healthy controls (2). Cognitive functions in PD are heterogeneous, with a subset of patients experiencing reversible cognitive alterations. However, when considering the entire PD patient population, there is a discernible longitudinal decline in cognitive function over time (3–7). The prevalence of mild cognitive impairment (MCI) accounts for up to 40% patients with PD (8), and dementia is observed in 24%–31% of patients with PD (9). Furthermore, more than 10 years following after the diagnosis of PD, over 75% of patients will suffer from dementia (10). Undeniably, cognitive impairment in PD patients drastically reduces their quality of life, imposes significant economic burdens on families and society, making it a pressing and unmet challenge.
Cognitive impairment in PD can be reported by patients and caregivers, or observed by clinicians. However, due to different observation perspectives, consensus on a patient’s cognitive status is often difficult to reach. Therefore, standardized cognitive rating scales are often used in clinical and research settings to assess cognitive function of patients with PD. Currently, there are multiple cognitive assessment scales used in different centers around the world for cognitive screening in PD, including Mini-Mental State Examination (MMSE), Montreal Cognitive Assessment (MoCA), Symbol Digit Modalities Test (SDMT), Benton Judgment of Line Orientation (JLO), Modified Semantic Fluency Test (SF), Letter Number Sequencing Test (LNS), and Addenbrooke’s Cognitive Examination (ACE), etc. (11–15). However, the use of cognitive assessment scales was highly variable between the different studies (16). In addition, to the best of our knowledge, there is no study comparing multiple cognitive assessment scales to evaluate their applicability and accuracy in detecting cognitive impairment in PD. Most studies have compared two cognitive assessment scales in cross-sectional PD cohorts, rather than in a longitudinal fashion. Reliable cognitive assessment is essential to evaluate the progression of cognitive changes in PD and implement timely cognitive intervention so as to deploy staff resources efficiently and improve outcomes. Therefore, it is important to identify the most reliable cognitive assessment tool that reflects cognitive dysfunction in PD patients, which requires the application of multiple cognitive assessment tools to the same longitudinal observation cohort.
Genetic factors have been shown to contribute to the rapidity of motor deterioration in PD (17–20), but the extent of genetic contribution to the cognitive decline in PD is poorly understood, partially due to the lack of a stable cognitive assessment tool for PD. Since the application of genome-wide association studies (GWAS) in PD, more than 90 independent single nucleotide polymorphisms (SNPs) have been identified in association with PD (21, 22), implicating multiple molecular pathways involved in the process of disease initiation. The progressive cognitive decline of PD is likely due to multiple molecular processes, among which apolipoprotein E (ApoE) has been shown as a vital gene for cognitive performance in PD (23, 24). Other genes such as glucocerebrosidase (GBA), microtubule-associated protein tau (MAPT), and catechol O-methyltransferase (COMT), may also be associated with cognitive decline in PD (25–29). Genes influence cognitive function through diverse pathways. For instance, GBA mutations promote the accumulation and aggregation of α-synuclein, ApoE significantly enhances the accumulation and subsequent deposition of amyloid-β in brain, and COMT is a regulator of synaptic dopamine (23, 27, 30, 31). While SNPs associated with PD may not solely determine the risk of disease onset, they could potentially shape the cognitive patterns exhibited by PD patients. Nonetheless, the intricate relationships between numerous PD related SNPs and cognitive function markers remain understudied and deserve further investigation. Identification of genetic contributions to cognitive deterioration in PD will enable us to better predict cognitive outcomes and eventually offer personalized treatment and care for patients. For this purpose, this study aims to determine a more suitable cognitive assessment scale in a longitudinal PD cohort, and further explore any genetic associations with the rapidity of cognitive decline.
2 Materials and methods
2.1 Participants
The data containing information of all participants were obtained from Parkinson’s Progression Markers Initiative (PPMI) funded by Michael J. Fox Foundation (MJFF) (32). PPMI was launched in 2011 to enroll participants, and the data pertaining to this study were retrieved from PPMI in January 2022. This study was approved by the scientific committee of PPMI and the Ethics Board of the Beijing Tiantan Hospital, Capital Medical University of China (KY 2018-031-02). The inclusion criteria for PD were as follow: (1) participants were Caucasians; (2) participants were diagnosed with idiopathic PD without other neurological diseases; (3) participants were not diagnosed with prodromal status, or without dopaminergic deficit (SWEDD), as scanned by dopamine transporter deficit on 123I ioflupane imaging (DaTscan); (4) participants were evaluated at least five times with missing data representing ≤20%, including baseline evaluation. The inclusion criteria for healthy controls (HCs) were as follows: (1) participants were diagnosed without PD or other neurological diseases; (2) participants had no family history of PD; (3) participants were evaluated at least five times with missing data representing ≤20%, including baseline evaluation. After screening, a total of 306 patients with PD and 99 HCs were included in this study.
2.2 Baseline and 5-year follow-up evaluation
2.2.1 Clinical data
2.2.1.1 Baseline evaluation
Unified Parkinson’s Disease Rating Scale (UPDRS) part III (UPDRS-III) was used to assess motor symptoms. MoCA, SDMT, JLO, SF, and LNS were applied to evaluate cognitive function. MoCA is a comprehensive cognitive screening tool that encompasses various aspects including orientation, attention, language, visuospatial, memory, and executive domains. SDMT is a neuropsychological test specifically designed to evaluate attention and processing speed, encompassing diverse domains like attention, visuospatial, memory, and executive function. LNS is tailored to assess working memory and executive function (33), while SF is related to language function. Furthermore, JLO has been applied in PD for examining visuospatial function (34). According to the MCI criteria in PPMI, MCI was delineated as scores on two or more cognitive screening scales, encompassing Hopkins Verbal Learning Test (HVLT), JLO, LNS, SF, and SDMT, that fell more than 1.5 standard deviations below the normal range, while exhibiting no functional impairment stemming from cognitive decline (32). Additionally, this definition aligns with MDS Level 1 criteria (35). For other non-motor symptoms, Rapid Eye Movement Behavior Disorder Screening Questionnaire (RBDSQ), Scales for Outcomes in Parkinson’s Disease-Autonomic (SCOPA-AUT), and Geriatric Depression Scale (GDS) were used for the evaluation of RBD, autonomic dysfunction, and depression, respectively.
2.2.1.2 Five-year follow-up evaluation
Participants from PPMI received regular follow-up evaluations since enrollment at baseline. According to the PPMI protocol, standard follow-up visits were scheduled for every 3 months during the initial year, every 6 months for the subsequent 4 years, and annually thereafter. Although cognitive function was assessed using different scales by different researchers from different PPMI locations, all researchers underwent and completed cognitive assessments training and quality control had been strictly monitored according to standardized protocols, thereby mitigating potential biases. Data on MoCA, SDMT, SF, and LNS scores for cognitive assessment and UPDRS-III for motor assessment were collected at baseline and annually throughout the following 5 years. We identified rapid cognitive and motor declines in patients who experienced changes in scores greater than the 75th percentile of all participants.
2.2.2 Genetic data
Genotyping results for ApoE and other PD-associated SNPs were obtained from NeuroX array. NeuroX array is an Illumina Infinium iSelect PD Custom Genotyping array designed for neurological disease studies. Genetic data were processed and analyzed using PLINK software (version 1.9). Quality controls were conducted for all samples and genotypes, and the threshold call rate was 95%. Quality controls of sample processing were determined by comparing the subject’s sex reported by Coriell Institute for Medical Research with the genotypic sex estimated from X chromosome heterogeneity. X chromosome heterogeneity calculations were based on common SNPs from the HapMap Project with <5% genotype deletions and Hardy–Weinberg equilibrium (HWE) p values >1 × 10−6. Samples containing discrepancies between reported sex and genotypic estimated sex were excluded. To exclude outliers, we performed principal component analysis (PCA) based on genotype using GCTA (version 1.93). Finally, we obtained genotype information of ApoE and 44 PD-risk SNPs from NeuroX array database of PPMI (Supplementary Table S1).
2.3 Statistical analysis
IBM SPSS 26 was used for statistical analysis in this study. We standardized the cognitive testing scores by z transformation using mean and standard deviation for PD and HCs, and z scores were calculated. Chi-squared test and one-way ANOVA were used for comparative analysis between groups. Receiver operating characteristic (ROC) curve analysis was used to evaluate the diagnostic value for MCI. Disease duration, gender, years of education, and age at onset were selected as covariates. The test–retest reliability was measured by intraclass correlation coefficients (ICC). Data between baseline scores and scores at each follow-up visit over 5 years were analyzed using ANOVA repeated measures, and Bonferroni’s correction was used for post hoc analysis.
3 Results
3.1 Baseline data observation
A total of 306 PD patients (mean age 61.0 ± 9.5 years; 61.8% male) and 99 HCs (mean age 60.4 ± 11.1 years; 59.6% male) were included in this study (Supplementary Table S2). In the PD cohort, the mean SDMT, MoCA, LNS, SF, and JLO scores were 41.4 ± 10.0, 26.7 ± 2.6, 10.5 ± 2.8, 22.0 ± 5.4, and 12.7 ± 2.4, respectively, and the mean UPDRS-III score was 19.6 ± 8.8 at baseline (Supplementary Table S2). SDMT and MoCA scores in PD patients were significantly lower than those of HCs (p < 0.001), while there were no significant differences between PD and HCs in LNS, SF, and JLO scores (Supplementary Table S2). As for motor and other non-motor variables, apart from GDS, there were significant differences between PD and healthy controls in UPDRS-III, RBDSQ, and SCOPA-AUT scores (p < 0.001). Among the patients with PD, 35 participants (11.4%) were classified as MCI. SDMT showed better performance in detecting MCI than the other four scales with the highest area under curve (AUC = 0.763; Supplementary Figure S1; Supplementary Table S3). Among the 306 PD patients, genotype data were available on 197 patients.
3.2 Longitudinal cognitive observation
Analyses revealed that SDMT, LNS, and JLO scores in PD patients significantly declined over 5 years (p < 0.001; Table 1; Figure 1). Entire PD patient population shows a discernible longitudinal decline in cognitive function (5–7, 36). In this study, SDMT scores showed a steady decrease over time, while the other four scales had more fluctuating declines over 5 years of follow-up (Figure 1). JLO scores had significant changes at year one, three, and five follow-ups compared to the previous visit, and overall, the scores were unstable (Figure 1). MoCA scores decreased at year one follow-up and remained steady thereafter (Figure 1). LNS scores showed a step-wise decline with plateaus at year one to year two and year three to year four (Figure 1). Repeated ANOVA analysis also showed that SDMT was the more suitable cognitive scale to assess cognitive function in PD (Figure 1). In addition, SDMT had a better reliability based on intraclass correlation coefficients (ICC = 0.75), while ICC for MoCA, JLO, LNS, and SF were 0.68, 0.58, 0.69, and 0.65, respectively. Additionally, only SDMT was significantly correlated with rapid motor progression (p < 0.010; Supplementary Table S4). In the healthy control group, only MoCA score showed significant changes over 5 years (p < 0.001; Table 1), while SDMT, LNS, JLO, and SF scores did not change significantly during the 5-year follow-up.
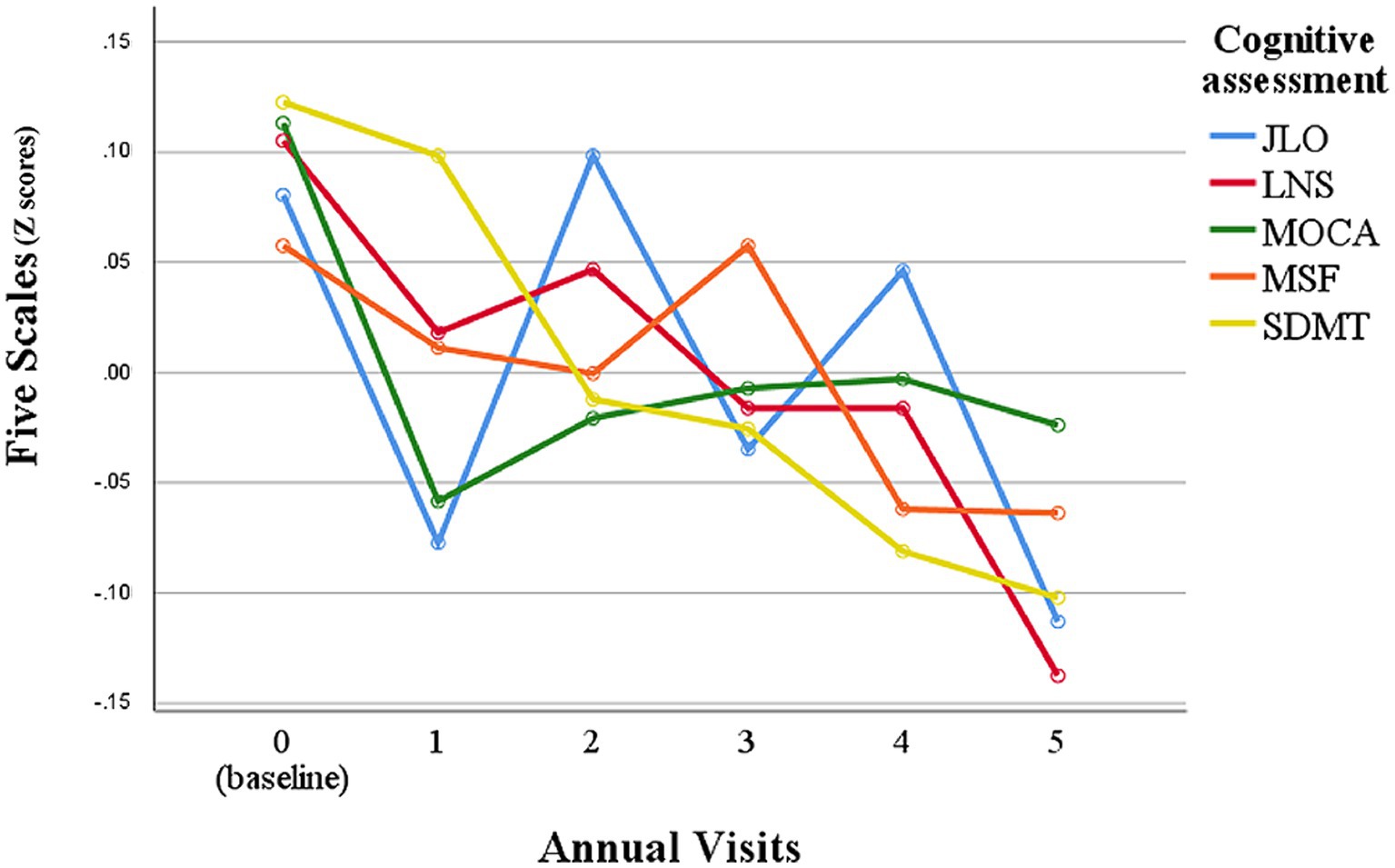
Figure 1. Longitudinal observation of the performance of each cognitive assessment scale in PD patients. Z scores were calculated by z transformation using mean and standard deviation. JLO, Benton Judgment of Line Orientation; MoCA, Montreal Cognitive Assessment; SDMT, Symbol Digit Modalities Test; LNS, Letter Number Sequencing Test; SF, Modified Semantic Fluency Test.
After determining that SDMT was the most suitable measure reflecting cognitive function in patients with PD among five cognitive screening scores at the early stage of the disease, we identified rapid cognitive decline in those patients who experienced changes in scores greater than the 75th percentile of all participants. There were no significant differences in demographics and cognitive function at baseline between the rapid cognitive decline group and others (Supplementary Table S5). Patients with rapid cognitive decline in SDMT also showed significant declines in MoCA and JLO. Rapid cognitive progressors had higher RBDSQ and SCOPA-AUT scores, and they also had faster motor symptom decline in UPDRS-III. We then investigated genetic factors contributing to cognitive performance. Four SNPs (rs4653767, rs6808178, rs12497850, and rs14235) were associated with poorer cognitive performance at baseline at p < 0.05, and only rs12497850 (residing in IP6K2, p = 0.0009; Table 2) was significantly associated with weaker cognitive performance after correction for multiple testing (p < 0.0011). For cognitive deterioration over the disease course, six SNPs (rs115185635, rs11724635, rs591323, rs13294100, rs2251776, and rs12456492) and ApoE ε4 were associated with faster cognitive decline at p < 0.05, and only rs591323 (residing in FGF20, p = 0.0007; Table 2) passed correction for multiple testing (p < 0.0011). IP6K2 rs12497850 yielded a model with an AUC of 0.661 for predicting cognitive impairment at baseline, while related SNPs reached a higher AUC at 0.809, and all 44 SNPs with ApoE reached a better prediction with AUC at 0.950 (Figure 2A). For the prediction of cognitive decline after disease onset, the AUC for rs591323 was 0.604, it reached 0.732 when considering related SNPs, and AUC for all 44 SNPs with ApoE was 0.831 (Figure 2B).
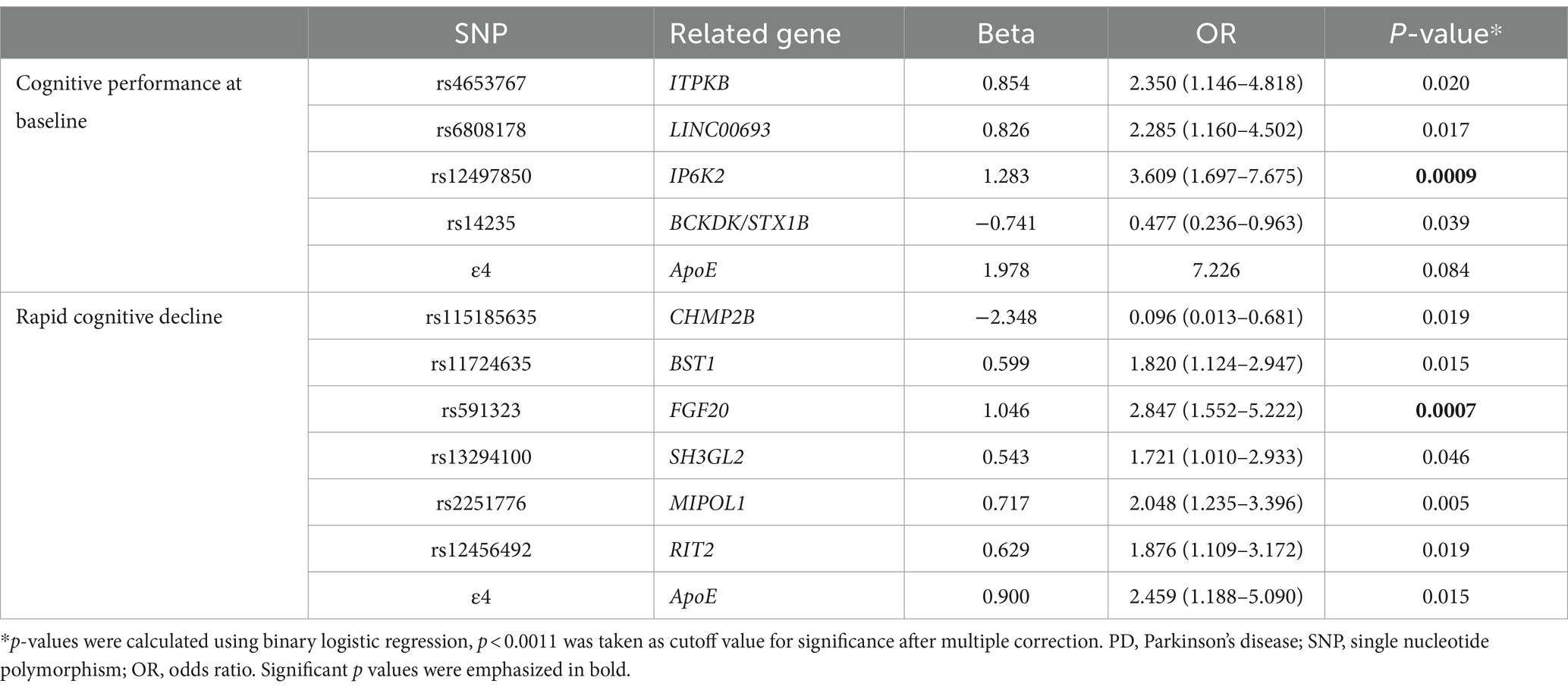
Table 2. Genetic associations with cognitive performance at baseline and rapid cognitive decline in PD.
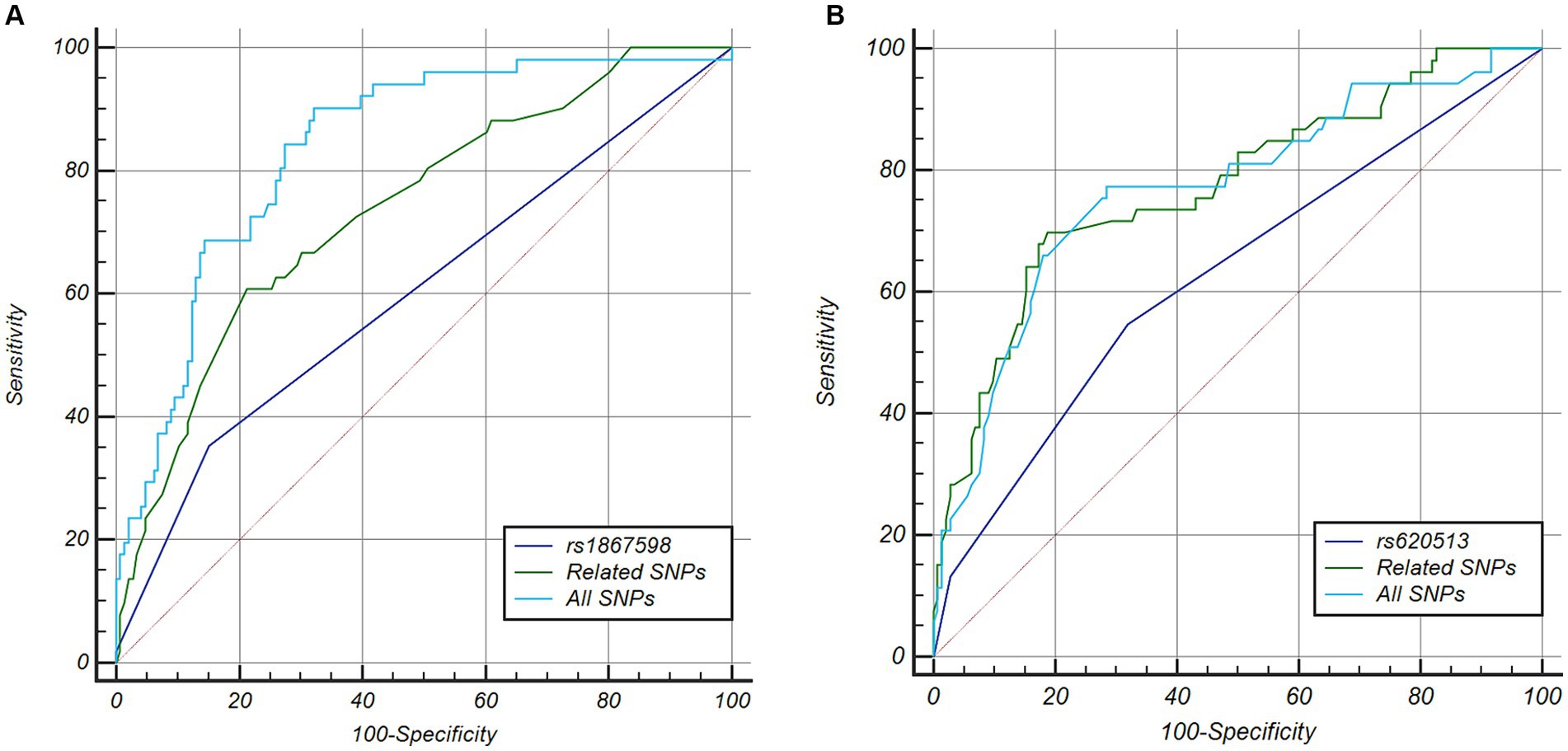
Figure 2. ROC curve analysis of five cognitive scales and genetic factors for detecting and predicting cognitive function in PD. (A) For predicting cognitive impairment at motor onset of PD, the AUC of rs12497850 was 0.661, four related SNPs reach a higher AUC at 0.809, but less than all SNPs together (AUC = 0.950). (B) For predicting cognitive impairment within 5 years of PD diagnosis, the AUC of rs591323 is 0.604, seven related SNPs reached a higher AUC at 0.723, but less than all SNPs together (AUC = 0.831). PD, Parkinson’s disease; JLO, Benton Judgment of Line Orientation; MoCA, Montreal Cognitive Assessment; SDMT, Symbol Digit Modalities Test; LNS, Letter Number Sequencing Test; SF, Modified Semantic Fluency Test; ROC, receiver operating characteristic; AUC, area under curve.
4 Discussion
In this study, we found that the SDMT may serve as a more suitable measure for reflecting cognitive function in patients PD. This is due to its steady downward trend, superior reliability, enhanced capability in detecting MCI, and significant association with motor progression. IP6K2 was associated with cognitive impairment prior to the onset of motor symptoms and FGF20 was associated with rapid cognitive decline after the onset of motor symptoms in PD.
There is a wide range of severity, progression rate, and affected cognitive domains in PD, from subjective cognitive complaints without objective evidence, MCI and dementia (37). The molecular basis of cognitive impairment in PD is likely related to neurotransmitter systems such as dopamine, acetylcholine, norepinephrine, and serotonin. Among them, dopamine depletion may lead to deficiencies in attention, working memory, planning, and response inhibition, while cholinergic loss has been associated with the decline of memory, language, and visuospatial function (38). Eventually, multiple domains were reported to be impaired in PD with cognitive impairment, especially executive function, followed by memory, attention, and visuospatial functions (39, 40). In addition, early cognitive function changes in PD primarily manifest in executive and visuospatial functions (31).
We found that SDMT was a more suitable tool not only for initial cognitive screening, but also for tracking cognitive changes over the course of PD. SDMT is a digit substitution test that requires the participant to swiftly pair each symbol with its corresponding number, adhering to the matching pattern provided at the top of the page. It is a neuropsychological test that assesses attention and processing speed, which involves multiple domains of attention, visuospatial, memory, and executive function (41). The mean change in SDMT from baseline to 5-year follow-up was 2.1 in a previous study (42), which was consistent to our findings (2.5). In this study, we found that MoCA scores declined most obviously at the first-year visit, and then remained stable in the following years, while it was a sensitive cognitive assessment tool in controls (Table 1), suggesting a ceiling effect of cognitive assessment in PD. MoCA, as a cognition screening instrument, was devised based on clinical insights into the typical impairment domains encountered in MCI, ensuring its optimal suitability for screening purposes. This comprehensive cognitive screening tool encompasses various cognitive domains such as orientation, attention, language, visuospatial skills, memory, and executive functions. MoCA has exhibited good test–retest reliability, internal consistency, and equivalence (43). It has good interrater reliability (0.81) and test–retest reliability (0.79) in PD (44). A 30-month follow-up PD study showed that MoCA scores were significantly lower compared with those at baseline (45). However, some MoCA subtests may be so challenging for PD patients that there may be floor effects (46, 47). Furthermore, executive and visuospatial domains tend to be more vulnerable during the early stages of the disease, which may result in potentially less sensitivity in detecting declines in the total scores of the MoCA compared to SDMT (31). As the disease advances to later stages, a broader range of cognitive domains are impacted, and MoCA may demonstrate better performance, but it needs further validation. LNS is influenced by various factors, including reading level, digit span forward and backward, arithmetic, and visual spatial learning. It serves as a scale tailored specifically for assessing processing speed and visual spatial working memory (33, 48). SF necessitates the proficiency in accessing and retrieving semantic knowledge, and it maintains a profound association with semantic memory and language function (49). Both LNS and SF have been used in detecting cognitive function in PD (25, 50, 51). Compared to SDMT, SF and LNS scores decreased significantly later in the fourth and fifth-year follow-up. Thus, LNS and SF seem to be more suitable for tracking related cognitive domains in PD for long-term follow-up. Moreover, JLO is regarded as a reliable assessment tool for evaluating visuospatial perception, extensively utilized in the context of PD to examine visuospatial function (34). Although JLO scores decreased significantly across 5 years, they fluctuated during follow-ups, which made JLO less stable for long-term follow-up evaluations. Despite the common use of MMSE, MMSE in not included in PPMI database perhaps due to its copyright, and it is less recommended in PD because of its multiple shortcomings, including insufficient executive and visuospatial components and less sensitivity in capturing mild degrees of deterioration (52).
Interestingly, SDMT appeared to be more suitable for PD cognitive assessment, although MoCA is currently more widely used than SDMT. SDMT has been reported as a stable screening scale in the same PPMI cohort in several studies (53, 54). It has been convincingly demonstrated that SDMT outperforms other scales in conducting longitudinal observations for another movement disorder, specifically Huntington’s disease (55, 56). Furthermore, SDMT can improve the sensitivity to detect vascular cognitive impairment (VCI) compared to MMSE and MoCA (57), and performing SDMT consumes far less time than MoCA and MMSE. In this study, SDMT exhibited superior reliability, enhanced performance in detecting MCI, and a significant association with motor progression. Therefore, we propose that SDMT may be more suitable to evaluate cognitive function in PD compared to MoCA, JLO, LNS, and SF. The reason may be that the cognitive domains detected by SDMT are more substantially and gradually affected by the process of PD, which makes SDMT a better cognitive assessment tool in both cross-sectional screening and longitudinal follow-up tracking, especially at early stage of the disease (42, 58). However, despite the fact that visuomotor impairment rarely affects SDMT performance, SDMT may still be influenced by bradykinesia (42, 58). To minimize the impact of motor symptoms to the utmost extent, we collect data annually, primarily focusing on evaluating longitudinal changes in cognitive scales rather than cross-sectional performance.
There is increasing evidence suggesting that genetic factors are key drivers of cognitive decline in PD. Variants in ApoE, GBA, MAPT, α-synuclein (SNCA) have been proven to be associated with cognitive decline and dementia in PD (23, 25, 26, 59–61). However, the question of whether PD-related SNPs recently discovered by GWAS are associated with cognitive performance warrants attention. In this study, we found that IP6K2 rs12497850 was associated with cognitive impairment at the time of motor symptoms onset, and FGF20 rs591323 was associated with more rapid cognitive decline after motor symptoms onset. After analysis in 3DSNP,1 rs12497850 may serve as a potential genic enhancer in tissues such as the hippocampus, despite being an intron variant (62). While rs591323 is also an intron variant, the extent to which its variation impacts the activity of FGF20 remains to be investigated (63). In our previous study, IP6K2 rs12497850 also displayed a potential association with motor progression, yet it did not meet the threshold for significance after multiple comparison correction (17). IP6K2 has been shown to participate in the process of cell death and apoptosis. It functions in maintaining mitochondrial homeostasis, promoting neuroprotection, and regulating Purkinje cell morphology (64–66). Experimental models have demonstrated that Purkinje cell dysfunction may be associated with cognitive function in the early stage of disease (67). With the accumulation of pathological protein(s), this effect might reach plateau and become less obvious, as IP6K2 is not associated with cognitive deterioration after motor onset of PD. FGF20 encodes a protein of the fibroblast growth factor family. FGF20 is preferentially expressed in the substantia nigra pars compacta of the brain and significantly improves the survival of midbrain dopaminergic neurons (68). FGF20 mutations are also related to decreases in hippocampal volume and diminished verbal episodic memory (69), which could account for our findings. Some studies have shown that ApoE ε4 carriers in PD had a faster cognitive deterioration rate (23, 25, 26, 60, 70). ApoE ε4 has been shown to accumulate along with the deposition of β amyloid, and it promotes α-synuclein aggregation, thus being associated with the severity of Lewy body pathology (71, 72), which may be the molecular mechanisms of worsening cognitive function in ApoE ε4 carriers of PD patients. In this study, ApoE was associated with rapid cognitive decline in PD with p < 0.05, but it failed to pass multiple testing correction. The lack of ApoE association with cognitive deficits in PD in another study was attributed to the early disease stage of that cohort (59). As participants in PPMI are mostly at an early stage of PD, associations between ApoE and cognitive changes may become obvious with longer observation windows.
There are several limitations in this study. First, although we have compared five different cognitive scales to identify an optimal method for cognitive assessment, we did not include other cognitive assessments, such as ACE-III (73), etc., because they were not included in the PPMI protocol. Therefore, we cannot comment on whether the use of SDMT has an advantage over ACE-III in cognitive evaluation in patients with PD. Second, patients enrolled in this study were mostly at early stage of PD, and the follow-up period was only up to 5 years. Cognitive changes would become more obvious in the middle and late stages of PD, and different molecular mechanisms might be involved at different disease stages. Third, genetic information was not available for the entire PPMI cohort, and other ethnicities were not considered in this study. Finally, the number of participants in this study cohort is relatively small. Therefore, further studies should incorporate a longer follow-up period, a larger multi-ethnic sample size, and a broader range of cognitive screening scales.
5 Conclusion
Our study indicates that SDMT is a preferable cognitive screening tool for tracking cognitive function in both cross-sectional and longitudinal studies of patients with PD during the early stages of the disease. Furthermore, the rapidity of cognitive decline in PD could be attributable to heterogeneous genetic factors, which may need to be verified in other PD cohorts with comprehensive clinical and genetic information.
Data availability statement
The original contributions presented in the study are included in the article/Supplementary material, further inquiries can be directed to the corresponding author.
Ethics statement
The studies involving humans were approved by Beijing Tiantan Hospital and PPMI. The studies were conducted in accordance with the local legislation and institutional requirements. The participants provided their written informed consent to participate in this study.
Author contributions
L-XC: Formal analysis, Investigation, Methodology, Validation, Writing – original draft. WK: Investigation, Validation, Writing – original draft. PC: Funding acquisition, Methodology, Supervision, Validation, Writing – review & editing. WZ: Resources, Supervision, Validation, Writing – review & editing. MM: Resources, Supervision, Writing – review & editing. YH: Conceptualization, Funding acquisition, Project administration, Resources, Supervision, Writing – review & editing.
Funding
The author(s) declare that financial support was received for the research, authorship, and/or publication of this article. This study was supported by National Natural Science Foundation of China (NSFC, 82071417, YH) and The National Key R&D Program of China (no. 2021YFC2501200, PC).
Acknowledgments
Data used in the preparation of this article were obtained from the Parkinson’s Progression Markers Initiative (PPMI) database (www.ppmi-info.org/data). For up-to-date information on the study, visit www.ppmi-info.org. PPMI is a public-private partnership funded by the Michael J. Fox Foundation for Parkinson’s Research and funding partners, including Abbvie, Allergan, Amathus Therapeutics, Avid Radiopharmaceuticals, Biogen, Biolegend, Bristol-Myers Squibb, Celgene, Covance, GE Healthcare, Genentech, Golub Capital, Handl Therapeutics, Insitro, Janssen Neuroscience, Lilly, Lundbeck, Merck, Meso Scale Discovery, Neurocrine, Pfizer, Piramal, Prevail Therapeutics, Roche, Sanofi Genzyme, Servier, Takeda, Teva, Ucb, Verily, and Voyager Therapeutics.
Conflict of interest
The authors declare that the research was conducted in the absence of any commercial or financial relationships that could be construed as a potential conflict of interest.
The author(s) declared that they were an editorial board member of Frontiers, at the time of submission. This had no impact on the peer review process and the final decision.
Publisher’s note
All claims expressed in this article are solely those of the authors and do not necessarily represent those of their affiliated organizations, or those of the publisher, the editors and the reviewers. Any product that may be evaluated in this article, or claim that may be made by its manufacturer, is not guaranteed or endorsed by the publisher.
Supplementary material
The Supplementary material for this article can be found online at: https://www.frontiersin.org/articles/10.3389/fneur.2024.1413187/full#supplementary-material
Footnotes
References
1. Pringsheim, T, Jette, N, Frolkis, A, and Steeves, TD. The prevalence of Parkinson's disease: a systematic review and Meta-analysis. Mov Disord. (2014) 29:1583–90. doi: 10.1002/mds.25945
2. Aarsland, D, Andersen, K, Larsen, JP, Lolk, A, Nielsen, H, and Kragh-Sorensen, P. Risk of dementia in Parkinson's disease: a community-based, Prospective Study. Neurology. (2001) 56:730–6. doi: 10.1212/wnl.56.6.730
3. Kong, WL, Huang, Y, Qian, E, and Morris, MJ. Constipation and sleep behaviour disorder associate with processing speed and attention in males with Parkinson's disease over five years follow-up. Sci Rep. (2020) 10:19014. doi: 10.1038/s41598-020-75800-4
4. Aarsland, D, Creese, B, Politis, M, Chaudhuri, KR, Ffytche, DH, Weintraub, D, et al. Cognitive decline in Parkinson disease. Nat Rev Neurol. (2017) 13:217–31. doi: 10.1038/nrneurol.2017.27
5. Pigott, K, Rick, J, Xie, SX, Hurtig, H, Chen-Plotkin, A, Duda, JE, et al. Longitudinal study of Normal cognition in Parkinson disease. Neurology. (2015) 85:1276–82. doi: 10.1212/WNL.0000000000002001
6. Pedersen, KF, Larsen, JP, Tysnes, OB, and Alves, G. Prognosis of mild cognitive impairment in early Parkinson disease: the Norwegian Parkwest study. JAMA Neurol. (2013) 70:580–6. doi: 10.1001/jamaneurol.2013.2110
7. Hu, MT, Szewczyk-Krolikowski, K, Tomlinson, P, Nithi, K, Rolinski, M, Murray, C, et al. Predictors of cognitive impairment in an early stage Parkinson's disease cohort. Mov Disord. (2014) 29:351–9. doi: 10.1002/mds.25748
8. Baiano, C, Barone, P, Trojano, L, and Santangelo, G. Prevalence and clinical aspects of mild cognitive impairment in Parkinson's disease: a Meta-analysis. Mov Disord. (2020) 35:45–54. doi: 10.1002/mds.27902
9. Aarsland, D, Zaccai, J, and Brayne, C. A systematic review of prevalence studies of dementia in Parkinson's disease. Mov Disord. (2005) 20:1255–63. doi: 10.1002/mds.20527
10. Aarsland, D, and Kurz, MW. The epidemiology of dementia associated with Parkinson disease. J Neurol Sci. (2010) 289:18–22. doi: 10.1016/j.jns.2009.08.034
11. Wilson, H, Pagano, G, Yousaf, T, Polychronis, S, De Micco, R, Giordano, B, et al. Predict cognitive decline with clinical markers in Parkinson's disease (Precode-1). J Neural Transm. (2020) 127:51–9. doi: 10.1007/s00702-019-02125-6
12. Gullett, JM, Price, CC, Nguyen, P, Okun, MS, Bauer, RM, and Bowers, D. Reliability of three Benton judgment of line orientation short forms in idiopathic Parkinson's disease. Clin Neuropsychol. (2013) 27:1167–78. doi: 10.1080/13854046.2013.827744
13. Hoops, S, Nazem, S, Siderowf, AD, Duda, JE, Xie, SX, Stern, MB, et al. Validity of the Moca and Mmse in the detection of mci and dementia in Parkinson disease. Neurology. (2009) 73:1738–45. doi: 10.1212/WNL.0b013e3181c34b47
14. Lucza, T, Ascherman, Z, Kovacs, M, Makkos, A, Harmat, M, Juhasz, A, et al. Comparing sensitivity and specificity of Addenbrooke's cognitive examination-I, iii and Mini-Addenbrooke's cognitive examination in Parkinson's disease. Behav Neurol. (2018) 2018:5932028. doi: 10.1155/2018/5932028
15. Hoogland, J, van Wanrooij, LL, Boel, JA, Goldman, JG, Stebbins, GT, Dalrymple-Alford, JC, et al. Detecting mild cognitive deficits in Parkinson's disease: comparison of neuropsychological tests. Mov Disord. (2018) 33:1750–9. doi: 10.1002/mds.110
16. Roheger, M, Kalbe, E, and Liepelt-Scarfone, I. Progression of cognitive decline in Parkinson's disease. J Parkinsons Dis. (2018) 8:183–93. doi: 10.3233/JPD-181306
17. Cao, LX, Jiang, Y, Piao, YS, and Huang, Y. Rapid motor Progression of Parkinson's disease associates with clinical and genetic variants. Front Biosci. (2021) 26:1503–12. doi: 10.52586/5044
18. Huang, Y, Rowe, DB, and Halliday, GM. Interaction between alpha-Synuclein and tau genotypes and the Progression of Parkinson's disease. J Parkinsons Dis. (2011) 1:271–6. doi: 10.3233/JPD-2011-11027
19. Wang, G, Huang, Y, Chen, W, Chen, S, Wang, Y, Xiao, Q, et al. Variants in the Snca gene associate with motor Progression while variants in the Mapt gene associate with the severity of Parkinson's disease. Parkinsonism Relat Disord. (2016) 24:89–94. doi: 10.1016/j.parkreldis.2015.12.018
20. Paul, KC, Schulz, J, Bronstein, JM, Lill, CM, and Ritz, BR. Association of Polygenic Risk Score with cognitive decline and motor Progression in Parkinson disease. JAMA Neurol. (2018) 75:360–6. doi: 10.1001/jamaneurol.2017.4206
21. Chang, D, Nalls, MA, Hallgrimsdottir, IB, Hunkapiller, J, van der Brug, M, Cai, F, et al. A Meta-analysis of genome-wide association studies identifies 17 new Parkinson's disease risk loci. Nat Genet. (2017) 49:1511–6. doi: 10.1038/ng.3955
22. Nalls, MA, Blauwendraat, C, Vallerga, CL, Heilbron, K, Bandres-Ciga, S, Chang, D, et al. Identification of novel risk loci, causal insights, and heritable risk for Parkinson's disease: a Meta-analysis of genome-wide association studies. Lancet Neurol. (2019) 18:1091–102. doi: 10.1016/S1474-4422(19)30320-5
23. Szwedo, AA, Dalen, I, Pedersen, KF, Camacho, M, Backstrom, D, Forsgren, L, et al. Gba and Apoe impact cognitive decline in Parkinson's disease: a 10-year population-based study. Mov Disord. (2022) 37:1016–27. doi: 10.1002/mds.28932
24. Tan, MMX, Lawton, MA, Jabbari, E, Reynolds, RH, Iwaki, H, Blauwendraat, C, et al. Genome-wide association studies of cognitive and motor Progression in Parkinson's disease. Mov Disord. (2021) 36:424–33. doi: 10.1002/mds.28342
25. Mata, IF, Leverenz, JB, Weintraub, D, Trojanowski, JQ, Hurtig, HI, Van Deerlin, VM, et al. Apoe, Mapt, and Snca genes and cognitive performance in Parkinson disease. JAMA Neurol. (2014) 71:1405–12. doi: 10.1001/jamaneurol.2014.1455
26. Paul, KC, Rausch, R, Creek, MM, Sinsheimer, JS, Bronstein, JM, Bordelon, Y, et al. Apoe, Mapt, and Comt and Parkinson's disease susceptibility and cognitive symptom Progression. J Parkinsons Dis. (2016) 6:349–59. doi: 10.3233/JPD-150762
27. Fang, YJ, Tan, CH, Tu, SC, Liu, CY, and Yu, RL. More than an "inverted-U"? An exploratory study of the association between the catechol-O-Methyltransferase gene polymorphism and executive functions in Parkinson's disease. PLoS One. (2019) 14:e0214146. doi: 10.1371/journal.pone.0214146
28. Yu, RL, Tu, SC, Wu, RM, Lu, PA, and Tan, CH. Interactions of Comt and Aldh2 genetic polymorphisms on symptoms of Parkinson's disease. Brain Sci. (2021) 11:361. doi: 10.3390/brainsci11030361
29. Ahmed, SS, Husain, RS, Kumar, S, and Ramakrishnan, V. Association between Mdr1 gene polymorphisms and Parkinson's disease in Asian and Caucasian populations: a Meta-analysis. J Neurol Sci. (2016) 368:255–62. doi: 10.1016/j.jns.2016.07.041
30. Fagan, ES, and Pihlstrom, L. Genetic risk factors for cognitive decline in Parkinson's disease: a review of the literature. Eur J Neurol. (2017) 24:561–e20. doi: 10.1111/ene.13258
31. Aarsland, D, Batzu, L, Halliday, GM, Geurtsen, GJ, Ballard, C, Ray Chaudhuri, K, et al. Parkinson disease-associated cognitive impairment. Nat Rev Dis Primers. (2021) 7:47. doi: 10.1038/s41572-021-00280-3
32. Parkinson Progression Marker, I . The Parkinson Progression Marker initiative (Ppmi). Prog Neurobiol. (2011) 95:629–35. doi: 10.1016/j.pneurobio.2011.09.005
33. Murtagh, A, Hurley, AL, Kinsella, A, Corvin, A, Donohoe, G, Gill, M, et al. The letter-number sequencing test and its association with potential to work among people with psychotic illness. Eur Psychiatry. (2010) 25:101–4. doi: 10.1016/j.eurpsy.2009.06.004
34. Montse, A, Pere, V, Carme, J, Francesc, V, and Eduardo, T. Visuospatial deficits in Parkinson's disease assessed by judgment of line orientation test: error analyses and practice effects. J Clin Exp Neuropsychol. (2001) 23:592–8. doi: 10.1076/jcen.23.5.592.1248
35. Litvan, I, Goldman, JG, Troster, AI, Schmand, BA, Weintraub, D, Petersen, RC, et al. Diagnostic criteria for mild cognitive impairment in Parkinson's disease: Movement Disorder Society task force guidelines. Mov Disord. (2012) 27:349–56. doi: 10.1002/mds.24893
36. Muslimovic, D, Post, B, Speelman, JD, De Haan, RJ, and Schmand, B. Cognitive decline in Parkinson's disease: a prospective longitudinal study. J Int Neuropsychol Soc. (2009) 15:426–37. doi: 10.1017/S1355617709090614
37. Goldman, JG, Williams-Gray, C, Barker, RA, Duda, JE, and Galvin, JE. The Spectrum of cognitive impairment in Lewy body diseases. Mov Disord. (2014) 29:608–21. doi: 10.1002/mds.25866
38. Kehagia, AA, Barker, RA, and Robbins, TW. Cognitive impairment in Parkinson's disease: the dual syndrome hypothesis. Neurodegener Dis. (2013) 11:79–92. doi: 10.1159/000341998
39. Smirnov, DS, Galasko, D, Edland, SD, Filoteo, JV, Hansen, LA, and Salmon, DP. Cognitive decline profiles differ in Parkinson disease dementia and dementia with Lewy bodies. Neurology. (2020) 94:e2076–87. doi: 10.1212/WNL.0000000000009434
40. Harvey, PD . Domains of cognition and their assessment. Dialogues Clin Neurosci. (2019) 21:227–37. doi: 10.31887/DCNS.2019.21.3/pharvey
41. Fellows, RP, and Schmitter-Edgecombe, M. Symbol digit modalities test: regression-based normative data and clinical utility. Arch Clin Neuropsychol. (2019) 35:105–15. doi: 10.1093/arclin/acz020
42. Wills, AA, Elm, JJ, Ye, R, Chou, KL, Parashos, SA, Hauser, RA, et al. Cognitive function in 1736 participants in Ninds exploratory trials in Pd long-term Study-1. Parkinsonism Relat Disord. (2016) 33:127–33. doi: 10.1016/j.parkreldis.2016.10.005
43. Nasreddine, ZS, Phillips, NA, Bedirian, V, Charbonneau, S, Whitehead, V, Collin, I, et al. The Montreal cognitive assessment, Moca: a brief screening tool for mild cognitive impairment. J Am Geriatr Soc. (2005) 53:695–9. doi: 10.1111/j.1532-5415.2005.53221.x
44. Gill, DJ, Freshman, A, Blender, JA, and Ravina, B. The Montreal cognitive assessment as a screening tool for cognitive impairment in Parkinson's disease. Mov Disord. (2008) 23:1043–6. doi: 10.1002/mds.22017
45. Chen, L, Yu, C, Zhang, N, Liu, J, and Liu, W. Cognitive impairment in patients with Parkinson's disease: a 30-month follow-up study. Clin Neurol Neurosurg. (2016) 151:65–9. doi: 10.1016/j.clineuro.2016.09.021
46. Tumas, V, Borges, V, Ballalai-Ferraz, H, Zabetian, CP, Mata, IF, Brito, MMC, et al. Some aspects of the validity of the Montreal cognitive assessment (Moca) for evaluating cognitive impairment in Brazilian patients with Parkinson's disease. Dement Neuropsychol. (2016) 10:333–8. doi: 10.1590/s1980-5764-2016dn1004013
47. Federico, A, Tinazzi, M, and Tamburin, S. Moca for cognitive screening in Parkinson's disease: beware of floor effect. Mov Disord. (2018) 33:499. doi: 10.1002/mds.27329
48. Crowe, SF . Does the letter number sequencing task measure anything more than digit span? Assessment. (2000) 7:113–7. doi: 10.1177/107319110000700202
49. Yang, J, McMahon, KL, Copland, DA, Pourzinal, D, Byrne, GJ, Angwin, AJ, et al. Semantic fluency deficits and associated brain activity in Parkinson's disease with mild cognitive impairment. Brain Imaging Behav. (2022) 16:2445–56. doi: 10.1007/s11682-022-00698-7
50. Zhang, G, Ma, J, Chan, P, and Ye, Z. Graph theoretical analysis of semantic fluency in patients with Parkinson's disease. Behav Neurol. (2022) 2022:1–7. doi: 10.1155/2022/6935263
51. Barrett, MJ, Sperling, SA, Blair, JC, Freeman, CS, Flanigan, JL, Smolkin, ME, et al. Lower volume, more impairment: reduced cholinergic basal forebrain Grey matter density is associated with impaired cognition in Parkinson disease. J Neurol Neurosurg Psychiatry. (2019) 90:1251–6. doi: 10.1136/jnnp-2019-320450
52. Skorvanek, M, Goldman, JG, Jahanshahi, M, Marras, C, Rektorova, I, Schmand, B, et al. Global scales for cognitive screening in Parkinson's disease: critique and recommendations. Mov Disord. (2018) 33:208–18. doi: 10.1002/mds.27233
53. Oltra, J, Segura, B, Uribe, C, Monte-Rubio, GC, Campabadal, A, Inguanzo, A, et al. Sex differences in brain atrophy and cognitive impairment in Parkinson's disease patients with and without probable rapid eye movement sleep behavior disorder. J Neurol. (2022) 269:1591–9. doi: 10.1007/s00415-021-10728-x
54. Wang, F, Pan, Y, Zhang, M, and Hu, K. Predicting the onset of freezing of gait in Parkinson's disease. BMC Neurol. (2022) 22:213. doi: 10.1186/s12883-022-02713-2
55. Lemiere, J, Decruyenaere, M, Evers-Kiebooms, G, Vandenbussche, E, and Dom, R. Cognitive changes in patients with Huntington's disease (Hd) and asymptomatic carriers of the Hd mutation--a longitudinal follow-up study. J Neurol. (2004) 251:935–42. doi: 10.1007/s00415-004-0461-9
56. Tabrizi, SJ, Scahill, RI, Owen, G, Durr, A, Leavitt, BR, Roos, RA, et al. Predictors of phenotypic Progression and disease onset in Premanifest and early-stage Huntington's disease in the Track-Hd study: analysis of 36-month observational data. Lancet Neurol. (2013) 12:637–49. doi: 10.1016/S1474-4422(13)70088-7
57. Dong, Y, Slavin, MJ, Chan, BP, Venketasubramanian, N, Sharma, VK, Collinson, SL, et al. Improving screening for vascular cognitive impairment at three to six months after mild ischemic stroke and transient ischemic attack. Int Psychogeriatr. (2014) 26:787–93. Epub 2014/01/16. doi: 10.1017/S1041610213002457
58. Pascoe, M, Alamri, Y, Dalrymple-Alford, J, Anderson, T, and MacAskill, M. The symbol-digit modalities test in mild cognitive impairment: evidence from Parkinson's disease patients. Eur Neurol. (2018) 79:206–10. doi: 10.1159/000485669
59. Phongpreecha, T, Cholerton, B, Mata, IF, Zabetian, CP, Poston, KL, Aghaeepour, N, et al. Multivariate prediction of dementia in Parkinson's disease. NPJ Parkinsons Dis. (2020) 6:20. doi: 10.1038/s41531-020-00121-2
60. Jo, S, Kim, SO, Park, KW, Lee, SH, Hwang, YS, and Chung, SJ. The role of Apoe in cognitive trajectories and motor decline in Parkinson's disease. Sci Rep. (2021) 11:7819. doi: 10.1038/s41598-021-86483-w
61. Winder-Rhodes, SE, Evans, JR, Ban, M, Mason, SL, Williams-Gray, CH, Foltynie, T, et al. Glucocerebrosidase mutations influence the natural history of Parkinson's disease in a community-based incident cohort. Brain. (2013) 136:392–9. doi: 10.1093/brain/aws318
62. Quan, C, Ping, J, Lu, H, Zhou, G, and Lu, Y. 3dsnp 2.0: update and expansion of the noncoding genomic variant annotation database. Nucleic Acids Res. (2022) 50:D950–5. doi: 10.1093/nar/gkab1008
63. Chiang, HL, Wu, YR, Chen, YC, Fung, HC, and Chen, CM. Fibroblast growth factor 20 gene polymorphism in Parkinson's disease in Asian population: a Meta-analysis. Genes. (2021) 12:674. doi: 10.3390/genes12050674
64. Nagpal, L, Fu, C, and Snyder, SH. Inositol Hexakisphosphate Kinase-2 in cerebellar granule cells regulates Purkinje cells and motor coordination via protein 4.1n. J Neurosci. (2018) 38:7409–19. doi: 10.1523/JNEUROSCI.1165-18.2018
65. Nagpal, L, Kornberg, MD, and Snyder, SH. Inositol Hexakisphosphate Kinase-2 non-catalytically regulates Mitophagy by attenuating Pink1 signaling. Proc Natl Acad Sci USA. (2022) 119:e2121946119. doi: 10.1073/pnas.2121946119
66. Morrison, BH, Bauer, JA, Kalvakolanu, DV, and Lindner, DJ. Inositol Hexakisphosphate kinase 2 mediates growth suppressive and apoptotic effects of interferon-Beta in ovarian carcinoma cells. J Biol Chem. (2001) 276:24965–70. doi: 10.1074/jbc.M101161200
67. Chaudhari, K, Wang, L, Kruse, J, Winters, A, Sumien, N, Shetty, R, et al. Early loss of cerebellar Purkinje cells in human and a transgenic mouse model of Alzheimer's disease. Neurol Res. (2021) 43:570–81. doi: 10.1080/01616412.2021.1893566
68. Ohmachi, S, Mikami, T, Konishi, M, Miyake, A, and Itoh, N. Preferential neurotrophic activity of fibroblast growth Factor-20 for dopaminergic neurons through fibroblast growth factor receptor-1c. J Neurosci Res. (2003) 72:436–43. doi: 10.1002/jnr.10592
69. Lemaitre, H, Mattay, VS, Sambataro, F, Verchinski, B, Straub, RE, Callicott, JH, et al. Genetic variation in Fgf20 modulates hippocampal biology. J Neurosci. (2010) 30:5992–7. doi: 10.1523/JNEUROSCI.5773-09.2010
70. Shahid, M, Kim, J, Leaver, K, Hendershott, T, Zhu, D, Cholerton, B, et al. An increased rate of longitudinal cognitive decline is observed in Parkinson's disease patients with low Csf Ass42 and an Apoe Epsilon4 allele. Neurobiol Dis. (2019) 127:278–86. doi: 10.1016/j.nbd.2019.02.023
71. Dickson, DW, Heckman, MG, Murray, ME, Soto, AI, Walton, RL, Diehl, NN, et al. Apoe Epsilon4 is associated with severity of Lewy body pathology independent of Alzheimer pathology. Neurology. (2018) 91:e1182–95. doi: 10.1212/WNL.0000000000006212
72. Davis, AA, Inman, CE, Wargel, ZM, Dube, U, Freeberg, BM, Galluppi, A, et al. Apoe genotype regulates pathology and disease Progression in Synucleinopathy. Sci Transl Med. (2020) 12:3069. doi: 10.1126/scitranslmed.aay3069
Keywords: Parkinson’s disease, cognitive assessment scale, cognitive decline, genetic association, single nucleotide polymorphism
Citation: Cao L-X, Kong WL, Chan P, Zhang W, Morris MJ and Huang Y (2024) Assessment tools for cognitive performance in Parkinson’s disease and its genetic contributors. Front. Neurol. 15:1413187. doi: 10.3389/fneur.2024.1413187
Edited by:
Yih-Ru Wu, Chang Gung Memorial Hospital, TaiwanReviewed by:
Rwei-Ling Yu, National Cheng Kung University, TaiwanGunchan Paul, Dayanand Medical College and Hospital, India
Copyright © 2024 Cao, Kong, Chan, Zhang, Morris and Huang. This is an open-access article distributed under the terms of the Creative Commons Attribution License (CC BY). The use, distribution or reproduction in other forums is permitted, provided the original author(s) and the copyright owner(s) are credited and that the original publication in this journal is cited, in accordance with accepted academic practice. No use, distribution or reproduction is permitted which does not comply with these terms.
*Correspondence: Yue Huang, yue.huang@ncrcnd.org.cn