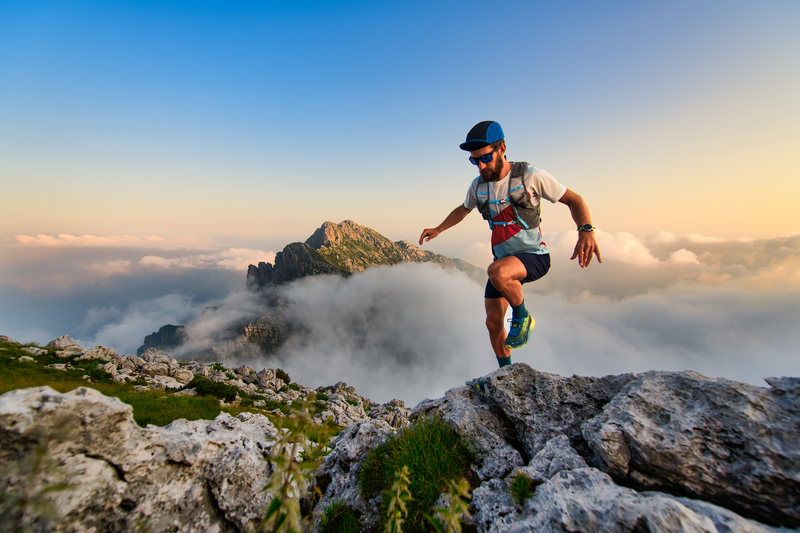
95% of researchers rate our articles as excellent or good
Learn more about the work of our research integrity team to safeguard the quality of each article we publish.
Find out more
SYSTEMATIC REVIEW article
Front. Neurol. , 09 December 2024
Sec. Artificial Intelligence in Neurology
Volume 15 - 2024 | https://doi.org/10.3389/fneur.2024.1413071
This article is part of the Research Topic Exploring the Future of Neurology: How AI is Revolutionizing Diagnoses, Treatments, and Beyond View all 10 articles
Background and aim: Neurodegenerative disorders (e.g., Alzheimer’s, Parkinson’s) lead to neuronal loss; neurocognitive disorders (e.g., delirium, dementia) show cognitive decline. Early detection is crucial for effective management. Machine learning aids in more precise disease identification, potentially transforming healthcare. This comprehensive systematic review discusses how machine learning (ML), can enhance early detection of these disorders, surpassing traditional diagnostics’ constraints.
Methods: In this review, databases were examined up to August 15th, 2023, for ML data on neurodegenerative and neurocognitive diseases using PubMed, Scopus, Google Scholar, and Web of Science. Two investigators used the RAYYAN intelligence tool for systematic reviews to conduct the screening. Six blinded reviewers reviewed titles/abstracts. Cochrane risk of bias tool was used for quality assessment.
Results: Our search found 7,069 research studies, of which 1,365 items were duplicates and thus removed. Four thousand three hundred and thirty four studies were screened, and 108 articles met the criteria for inclusion after preprocessing. Twelve ML algorithms were observed for dementia, showing promise in early detection. Eighteen ML algorithms were identified for Parkinson’s, each effective in detection and diagnosis. Studies emphasized that ML algorithms are necessary for Alzheimer’s to be successful. Fourteen ML algorithms were discovered for mild cognitive impairment, with LASSO logistic regression being the only one with unpromising results.
Conclusion: This review emphasizes the pressing necessity of integrating verified digital health resources into conventional medical practice. This integration may signify a new era in the early detection of neurodegenerative and neurocognitive illnesses, potentially changing the course of these conditions for millions globally. This study showcases specific and statistically significant findings to illustrate the progress in the area and the prospective influence of these advancements on the global management of neurocognitive and neurodegenerative illnesses.
Machine learning (ML) describes circumstances in which machines can mimic human minds in learning and analysis and thus be used to solve problems (1). Recent advances in ML have produced a computational framework by integrating a multitude of patient data and providing unique risk assessments and recommendations to each patient, which has the potential to revolutionize clinical decision-making (2) fundamentally.
Helping with diagnosis is one of the most significant uses of machine learning in this field. The promise of machine learning-based disease diagnosis (MLBDD), which is affordable and time-effective, is demonstrated by numerous researchers and practitioners (2). To identify chronic kidney disease, Ma et al. (2020) suggested a heterogeneous modified artificial neural network (HMANN) model that obtained an accuracy of 87–99% (3). To improve the diagnosis of COVID-19, Apostolopoulos and Mpesiana (2020) used a CNN-based Xception model on an imbalanced dataset of 284 COVID-19 and 967 non-COVID-19 patient chest X-ray images and achieved 89.6% accuracy in diagnosis (4). Regarding the diagnosis of diabetes, Yahyaoui et al. (2019) showed that the machine-learning RF technique works with an accuracy of 83.67% (5). The examples demonstrate how machine learning algorithms can provide more accurate and reliable disease diagnosis than other diagnostic techniques.
Neurodegenerative disorders are characterized by a gradual loss of neurons, often leading to death. The term covers a wide range of clinical diseases and progressive dementing conditions, including Alzheimer’s disease (AD), Parkinson’s disease (PD), and a number of other neurological disorders (6). Neurocognitive disorders, including delirium, mild cognitive impairment and dementia, are characterized by a decrease in cognitive functioning from a previously attained level (7). Many of these diseases are incurable and sometimes fatal, but early detection can significantly improve the ability to control them.
AD is the most prevalent form of dementia. Patients with AD have trouble remembering things, which limits their ability to learn. Due to the slow progression of AD and the difficulty of current diagnostic techniques in identifying it in its early stages, early diagnosis of the disease is crucial.
PD is a progressive and chronic neurodegenerative disease. The overall validity of PD’s clinical diagnosis, particularly in the early stages of the disease, is unsatisfactory (8).
Delirium is acute brain dysfunction that causes cognitive impairment and shifting attention. Numerous symptoms, such as significant psychomotor agitation, a low level of consciousness, or both, may be present. Traditionally, one or more physicians’ evaluations have been used to diagnose delirium clinically. However, this method of diagnosis might contain flaws because of the disease’s unstable nature (9).
As evident, standard clinical diagnostic techniques for neurodegenerative and neurocognitive diseases have flaws, which make it difficult and occasionally impossible to diagnose the disease, especially in its early stages. On the other side, machine learning algorithms can be highly accurate when it comes to diagnosing a variety of diseases. Recently, many studies have been conducted on the efficacy of ML algorithms as a quick and reliable alternative diagnostic method. Therefore, in this article, we aimed to systematically assess different uses of ML algorithms in detecting neurodegenerative and neurocognitive disorders early.
This systematic review study was conducted as stated by Preferred Reporting Items for Systematic Reviews and Meta-Analyses (PRISMA2020) principles (10). This review has been registered on The Open Science Framework (OSF) (registration DOI https://osf.io/rtsyk/).
A comprehensive search of several databases was conducted from inception to August 15th, 2023. The databases included PubMed/MEDLINE, Scopus, Google Scholar and Web of Science. As seen in table 1, the search for AI algorithms used for detecting and screening neurodegenerative and neurocognitive diseases involved a controlled vocabulary supplemented with keywords in each database. Table 1 demonstrates the specific search syntax used for each database involved.
We used the RAYYAN intelligent tool for systematic reviews to screen the search results (11). Titles and abstracts from 7,069 articles obtained from our search strategy were independently and blindly screened by six reviewers (Zh.M., Sh.K., H.D., A.A., H.B., M.Y.). The duplicate records were removed using the same tool. The conflicts were resolved by a seventh reviewer (Sh.K.) using RAYYAN’s compute rating feature.
The study was conducted on this specified list of neurodegenerative and neurocognitive diseases, and the search keywords included items below:
• Huntington
• Tauopathies and the subclassifications
• Neurofibrillary tangles
• Myelitis
• Paraneoplastic polyneuropathy
• Paraneoplastic cerebellar degeneration
• Tourette syndrome
• Neurofibromatoses
• Encephalopathy
• Neuropathy
• ALS
• Alzheimer’s disease (AD)
• Mild cognitive impairment (MCI)
• Parkinson’s disease (PD)
• Frontotemporal dementia (FTD)
• Lewy Body’s disease (LBD)
• Progressive supranuclear palsy (PSP)
• Corticobasal degeneration (CBD)
• Wernicke-Korsakoff syndrome
• Normal pressure hydrocephalus (NPH)
• Prion diseases, such as Creutzfeldt-Jakob disease
• Vascular dementia
Studies that were not available as open access were in languages other than English were conducted on animals, and were published as book chapters, Conference papers were excluded.
Two assessors (MY and HD) evaluated each study separately based on the Cochrane risk of bias tool, evaluating all included studies (12). With a focus on six domains—sequence generation, allocation concealment, blinding, incomplete data, and selective reporting—the Cochrane risk of bias tool is a widely used and standard tool that contains all the necessary questions to evaluate methodological quality and bias risk. The two assessors settled other biases and disagreements through discussion and consensus.
Our search strategies in four databases yielded 7,069 studies, 1,365 were eliminated as duplicates. At least two individuals screened each of 4,334 remaining studies through title and abstract. Unrelated studies whose full text was unavailable, did not meet our inclusion criteria, and were not in English were excluded. At last, 108 studies were included for interpretation. Figure 1 depicts the study selection procedure.
The included studies were published between 2015 and 2023. A study was carried out in Africa, another in Australia, 17 in Europe, 29 in America, and the remaining in Asia.
In the included studies, 3,723,329 participants were examined. Thirty-four studies on AD, 14 on PD, 13 on MCI, 10 on dementia, 7 on MS and the remaining studies were carried out on other neurodegenerative and neurocognitive disorders.
In 10 studies conducted on dementia, 12 ML algorithms were used: XGBoost classification, Binary logistic regression (LR), A logistic model tree classifier combined with information gain feature selection, 3D convolutional neural networks (3D CNN), k-NearestNeighbor (kNN), support vector machine (SVM), random forest (RF), parallel recurrent convolutional neural network (PRCNN), support vector machine classifiers (SVC), support vector regression (SVR), partial least squares regression (PLSR) and Deep Neural Network (DNN), All of which showing promising results in early detection and screening of the disease. Table 2 summarizes our included studies.
Table 2. Summary of included studies on the machine learning algorithms for early detection of NCDs and NDDs.
SVM and XGBoost are prominent models for early dementia detection, each with distinct advantages and disadvantages regarding sensitivity and specificity. SVM excels in handling unbalanced datasets, achieving high sensitivity and specificity (over 90% in some studies), making identifying subtle early-stage symptoms practical. However, it can struggle with scalability and requires significant computational resources. In contrast, XGBoost offers flexibility and speed, handling various input features well, with sensitivities reaching between 80 and 85%. Yet, it may only perform in specificity compared to SVM if carefully tuned, which demands advanced cross-validation methods and more computational power. Both models demonstrate effectiveness; however, SVM offers enhanced specificity, which is vital for precise diagnostic accuracy. However, XGBoost excels in sensitivity but necessitates meticulous tuning to achieve optimal performance.
The ML algorithms used for PD are as follows: Center of Pressure, Load Distribution, Random forest algorithm, Neural Network (NN), Support vector machine, and affine registration using the FSL library developed by the Oxford Centre for Functional MRI of the Brain (FMRIB), Multi-Layer Perceptron (MLP), Vertical Ground Reaction Force (VGRF), logistic regression (LR), linear discriminant analysis (LDA), kNN, classification and regression tree (CART), Naive Bayes (NB), bagged decision tree (BDT), extra tree classifier (ETC), AdaBoost classifier (AC), gradient boosting classifier (GBC), Extremely Randomized Trees (ERT), Discriminant Analysis (DIS), Deep Learning (DEEP). All mentioned algorithms showed significant early PD detection, diagnosis and screening capabilities, and most had considerable sensitivity and specificity.
According to the review of studies, various algorithmic models have been employed for the early diagnosis of Parkinson’s disease, with deep learning models demonstrating exceptional effectiveness. These models achieve nearly 100% accuracy, along with high sensitivity and specificity. Their advantages include remarkable accuracy, non-invasive techniques utilizing medical imaging data, and automated feature extraction, which minimizes the need for manual data handling. However, deep learning models necessitate substantial computational resources and large volumes of labeled data, and their “black box” nature poses challenges for interpretability.
In the included studies, much attention was paid to using ML algorithms for diagnosing and progressing Alzheimer’s. The following algorithms were used for detection, screening and progression of AD, all of which were successful for the purposes: Sequential minimal optimization (SMO), Naive Bayes (NB), tree augmented Naive Bayes (TAN), K2, MATLAB PatternRecognition toolbox, TF-IDF, CountVectorizer (CV), Word2Vec, FastText, VGG16 with XGB, stacked fusion models//hybrid stacked fusion model, PRS, AAO, KNN, decision tree, random forest, ANN, 3D-CNN model, Boruta FS algorithm, Gradient, Information Gain (IG), Multi-view Separable Pyramid Network (MiSePyNet), PyWinEA using Mono-objective and Multi-objective Genetic Algorithms (NSGAII), Elastic Net (EN), Gaussian Processes (GP), kNN, (LR), Linear Discriminant, Support Vector Machine, Voting classifiers, Multi-Classifier Network (MCN), Gradient Boosted Trees (GBTs), basic three-layer Neural Network architecture using the OASIS, Sparse K-means w/Resampling, a deep neural network architecture, Adaboost, graph convolutional and recurrent neural network (graph-CNN-RNN), Single hidden layer neural network, Single-layer bidirectional, LSTM, Three-layer CNN, Deep Belief Network (DBN), stacked auto-encoder (SAE), SVR, SVC, PLSR, Shallow Models, Feature Pyramid Network (FPN) and temporally structured SVM (TS-SVM).
Studies in our review suggested different algorithms for best accuracy to early detection of Alzheimer’s disease but Deep learning models especially CNNs, and SVM reported more effective than others. SVM and CNN each offer distinct advantages and limitations. SVM is advantageous due to its reliable classification accuracy and specificity, reaching about 93% accuracy and 87% sensitivity in some studies, making it efficient for handling smaller datasets with feature selection methods. However, it can struggle with high-dimensional data unless combined with dimensionality reduction techniques. In contrast, CNN models have shown high sensitivity and specificity in early detection of Alzheimer’s disease, with some studies reporting accuracies above 95%. Their advantages include high accuracy, automated feature extraction, and the non-invasive nature of using medical imaging data. However, these models require significant computational resources, large amounts of labeled data, and are often considered “black boxes” due to their lack of interpretability.
ML algorithms used for MCI included 3D-CNN, support vector machines, Gaussian Naive Bayes(GNB), EMCI identification framework, LASSO logistic regression, Naïve Bayes, Decision Tree, RF, Gaussian, Polynomial-kernel Support Vector Machines, kNN, LR, Adaboost and TS-SVM model. Except for LASSO logistic regression, all showed remarkable performance in early detection of the disease and its development to AD and dementia.
For the early detection of MCI, 3D-CNNs have proven to be highly effective, with studies demonstrating over 95% accuracy, high sensitivity and specificity. The advantages of CNNs include their ability to automatically extract relevant features from complex datasets and their non-invasive application of medical imaging. However, they require substantial computational resources and large amounts of labeled data, and their decision-making processes are often not easily interpretable, rendering them “black boxes.” SVMs are another viable option, offering moderate sensitivity and good interpretability. However, they necessitate extensive parameter tuning and may overlook fine spatial features critical for accurate diagnosis. RF and Decision Trees provide high interpretability and effectively manage non-linear data, though they typically exhibit lower sensitivity than CNN models. Ensemble methods, such as combinations of LASSO logistic regression and Naïve Bayes, offer a balanced approach in terms of sensitivity and specificity. These methods can serve as cost-effective options for initial screenings, particularly when integrated with clinical biomarkers. Overall, the choice of model should consider the trade-offs between performance, interpretability, and resource requirements to optimize early detection of MCI.
Random forest, LR, support vector machine, LightGBM, kNN, Decision tree, Gaussian Naïve Bayes (gNB), Auto-sklearn, Gaussian Processes (GP) regression, Gaussian Process regression (GPR), CNN, Adaboost, NN and LDR algorithms were also successfully used for detection and progression of Huntington’s disease, Multiple Sclerosis, Amyotrophic lateral sclerosis (ALS), Corticobasal Syndrome (CS), Neurofibromatosis type 1, Amyloid and Delirium.
In this study, we investigated 108 studies evaluating patients with neurological diseases for early detection using ML algorithms. This study showcases specific and statistically significant findings to illustrate the progress in the area and the prospective influence of these advancements on the global management of neurocognitive and neurodegenerative illnesses.
AI technologies can retrieve data from medical texts and generate diagnostic and prediction models using this data. An extensive collection of electronic medical records amassed over a considerable period can serve as the fundamental data for this form of research (13). Traditional diagnostic methods for PD diagnosis may misdiagnose because they evaluate small movements that are hard to classify. Early non-motor symptoms of PD may be minor and caused by other illnesses. Thus, these symptoms are typically missed, making early PD diagnosis difficult. ML algorithms have been used to classify PD and healthy controls or patients with comparable clinical presentations to overcome these issues and improve PD diagnosis and evaluation. Multiple ML-based computer-aided diagnosis and detection (CADD) systems have shown promise in identifying PD patients from healthy controls (14). Using preclinical indicators of non-motor symptoms, including sleep Behavior Disorder (RBD) and olfactory loss, CSF measures, and dopaminergic imaging to classify early PD and healthy normal Prashanth et al. found SVM classification near-perfect (15). Balaji et al. proposed a multi-class learning technique that differs from earlier machine learning approaches, which often focus on binary classification to identify the existence of PD. In contrast, the proposed approach can not only classify but also quantify the stages of PD (16).
Individuals afflicted with MCI typically experience a deterioration in cognitive abilities, which significantly affects their general health. Importantly, failure to promptly identify this illness by medical professionals can readily progress into dementia. Using artificial intelligence, a dimensional assessment technique may seamlessly combine classical neuropsychological measurements and facilitate the diagnosis of AD (17). Similarly, Raees et al. present an initial automated deep learning system that utilizes a large MRI dataset of normal and 111 patients to predict AD. By evaluating the effectiveness of SVM and DNN models, they demonstrate that Deep learning has a significant level of accuracy, ranging from 80 to 90%, in predicting AD (18). Goenka and Tiwari introduced a three-class CNN that utilizes three computational approaches for neuroimaging to classify AD. Their suggested model was empirically validated, demonstrating classification accuracies of 97.48, 96.62, and 86.49% for big, medium, and small patches, respectively (19). Artificial neural networks revealed an intricate correlation between cognitive state and auditory function that cannot be easily anticipated only by considering the cognitive differences between individuals with and without AD (20).
Zhao et al. reported that Support Vector Machines (SVM), which integrate short-term clinical and brain MRI data, show potential in predicting the course of MS illness and identifying individuals who would benefit from more aggressive treatment approaches (21). Similarly, Law et al. found that the possibility of disability in MS was most accurately predicted using non-parametric machine learning techniques. It also can select those with the highest and lowest progression risk for inclusion in secondary progressive M.S. (22). Concordantly, Zhang et al. found that computational approaches (Lesion Segmentation Toolbox) provide more accurate conversion predictions from CIS to MS than human visual analysis (23). Goyal et al. utilized a machine learning technique to predict MS by analyzing serum cytokines. Their findings indicate that the RF model achieved an accuracy of 91%, suggesting its potential for predicting MS using serum cytokine levels. Moreover, the RF model demonstrated a 70% accuracy in classifying MS patients into remitting and non-remitting categories (24). These data were similar to other studies (25–27).
This comprehensive systematic study of 108 articles includes papers that illustrate significant patterns in using artificial intelligence for early detection of neurological illnesses. Therefore, the authors confine their focus to presenting factual information on utilizing AI techniques in various tasks without evaluating the quality of these investigations. Further studies are required to evaluate additional aspects, such as new neuroimaging measurements and blood and genetic biomarkers. The utilization of predictive algorithms, as detailed in this study, may enhance the development of collaborative visualization and decision-making tools for physicians and patients, as previously explored in another research. Future research could focus on decreasing the number of attributes without compromising accuracy. The proposed strategies can also be extended to tackle other chronic disorders. When creating AI models for medical issues, it is advisable to use simple computational methods with the available datasets to make it easier to implement the predictive tool in healthcare settings and solve economic issues.
The original contributions presented in the study are included in the article/supplementary material, further inquiries can be directed to the corresponding author/s.
MY: Writing – original draft, Writing – review & editing. MA: Writing – original draft, Writing – review & editing. ZM: Writing – original draft, Writing – review & editing. SK: Writing – original draft, Writing – review & editing. HD: Writing – original draft, Writing – review & editing. AA: Writing – original draft, Writing – review & editing. SS: Writing – original draft, Writing – review & editing. MAD: Writing – original draft, Writing – review & editing. HB: Writing – original draft, Writing – review & editing. NM: Writing – original draft, Writing – review & editing. SV: Writing – original draft, Writing – review & editing. ZR: Writing – original draft, Writing – review & editing. MAA: Investigation, Methodology, Validation, Writing – original draft, Writing – review & editing. DS: Writing – original draft, Writing – review & editing, Validation, Conceptualization. AM: Writing – original draft, Validation, Writing – review & editing, Conceptualization.
The author(s) declare that no financial support was received for the research, authorship, and/or publication of this article.
The authors declare that the research was conducted in the absence of any commercial or financial relationships that could be construed as a potential conflict of interest.
All claims expressed in this article are solely those of the authors and do not necessarily represent those of their affiliated organizations, or those of the publisher, the editors and the reviewers. Any product that may be evaluated in this article, or claim that may be made by its manufacturer, is not guaranteed or endorsed by the publisher.
1. Huang, G, Huang, G-B, Song, S, and You, K. Trends in extreme learning machines: a review. Neural Netw. (2015) 61:32–48. doi: 10.1016/j.neunet.2014.10.001
2. Ahsan, MM, and Siddique, Z. Machine learning-based heart disease diagnosis: a systematic literature review. Artif Intell Med. (2022) 128:102289. doi: 10.1016/j.artmed.2022.102289
3. Ma, F, Sun, T, Liu, L, and Jing, H. Detection and diagnosis of chronic kidney disease using deep learning-based heterogeneous modified artificial neural network. Futur Gener Comput Syst. (2020) 111:17–26. doi: 10.1016/j.future.2020.04.036
4. Apostolopoulos, ID, and Mpesiana, TA. COVID-19: automatic detection from x-ray images utilizing transfer learning with convolutional neural networks. Physical Engin Sci Med. (2020) 43:635–40. doi: 10.1007/s13246-020-00865-4
5. Yahyaoui, A, Jamil, A, Rasheed, J, and Yesiltepe, M, editors. A decision support system for diabetes prediction using machine learning and deep learning techniques. In 2019 1st international informatics and software engineering conference (UBMYK) (2019)
6. Mendez, MF, and McMurtray, AM. Neurodegenerative Disorders In: G Fink, editor. Encyclopedia of stress. Second ed. New York: Academic Press (2007). 840–4.
7. Sachdev, PS, Blacker, D, Blazer, DG, Ganguli, M, Jeste, DV, Paulsen, JS, et al. Classifying neurocognitive disorders: the DSM-5 approach. Nat Rev Neurol. (2014) 10:634–42. doi: 10.1038/nrneurol.2014.181
8. Rizzo, G, Copetti, M, Arcuti, S, Martino, D, Fontana, A, and Logroscino, G. Accuracy of clinical diagnosis of Parkinson disease: a systematic review and meta-analysis. Neurology. (2016) 86:566–76. doi: 10.1212/WNL.0000000000002350
9. Zou, Y, Cole, MG, Primeau, FJ, McCusker, J, Bellavance, F, and Laplante, J. Detection and diagnosis of delirium in the elderly: psychiatrist diagnosis, confusion assessment method, or consensus diagnosis? Int Psychogeriatr. (1998) 10:303–8. doi: 10.1017/S1041610298005390
10. Page, MJ, McKenzie, JE, Bossuyt, PM, Boutron, I, Hoffmann, TC, Mulrow, CD, et al. The PRISMA 2020 statement: an updated guideline for reporting systematic reviews. Int J Surg. (2021) 88:105906. doi: 10.1016/j.ijsu.2021.105906
11. Ouzzani, M, Hammady, H, Fedorowicz, Z, and Elmagarmid, A. Rayyan—a web and mobile app for systematic reviews. Syst Rev. (2016) 5:1–10. doi: 10.1186/s13643-016-0384-4
12. Gingras-Charland, M-E, Côté, A-M, Girard, P, Grenier, A, Pasquier, J-C, and Sauvé, N. Pre-eclampsia educational tool impact on knowledge, anxiety, and satisfaction in pregnant women: a randomized trial. J Obstet Gynaecol Can. (2019) 41:960–70. doi: 10.1016/j.jogc.2018.10.003
13. Danilov, G, Shifrin, M, Kotik, K, Ishankulov, T, Orlov, YN, Kulikov, A, et al. Artificial intelligence technologies in neurosurgery: a systematic literature review using topic modeling. Part II: research objectives and perspectives. Mod Technol Med. (2020) 12:111. doi: 10.17691/stm2020.12.6.12
14. Dash, S. A systematic review of adaptive machine learning techniques for early detection of Parkinson's disease. Artificial Intelligence Neurol Disord. (2023):361–85. doi: 10.1016/B978-0-323-90277-9.00018-3
15. Prashanth, R, Roy, SD, Mandal, PK, and Ghosh, S. High-accuracy detection of early Parkinson's disease through multimodal features and machine learning. Int J Med Inform. (2016) 90:13–21. doi: 10.1016/j.ijmedinf.2016.03.001
16. Balaji, E, Brindha, D, and Balakrishnan, R. Supervised machine learning based gait classification system for early detection and stage classification of Parkinson’s disease. Appl Soft Comput. (2020) 94:106494. doi: 10.1016/j.asoc.2020.106494
17. Cavedoni, S, Chirico, A, Pedroli, E, Cipresso, P, and Riva, G. Digital biomarkers for the early detection of mild cognitive impairment: artificial intelligence meets virtual reality. Front Hum Neurosci. (2020) 14:245. doi: 10.3389/fnhum.2020.00245
18. Raees, PM, and Thomas, V. Automated detection of Alzheimer’s disease using deep learning in MRI. J Phys Conf Ser. (2021). doi: 10.1088/1742-6596/1921/1/012024
19. Goenka, N, and Tiwari, S. AlzVNet: a volumetric convolutional neural network for multiclass classification of Alzheimer’s disease through multiple neuroimaging computational approaches. Biomed Signal Process Control. (2022) 74:103500. doi: 10.1016/j.bspc.2022.103500
20. Krishnamurti, S, Drake, L, and King, J. Neural network modeling of central auditory dysfunction in Alzheimer’s disease. Neural Netw. (2011) 24:646–51. doi: 10.1016/j.neunet.2011.02.010
21. Zhao, Y, Healy, BC, Rotstein, D, Guttmann, CR, Bakshi, R, Weiner, HL, et al. Exploration of machine learning techniques in predicting multiple sclerosis disease course. PLoS One. (2017) 12:e0174866. doi: 10.1371/journal.pone.0174866
22. Law, MT, Traboulsee, AL, Li, DK, Carruthers, RL, Freedman, MS, Kolind, SH, et al. Machine learning in secondary progressive multiple sclerosis: an improved predictive model for short-term disability progression. Multiple Sclerosis J Experiment Transl Clin. (2019) 5:2055217319885983. doi: 10.1177/2055217319885983
23. Zhang, H, Alberts, E, Pongratz, V, Mühlau, M, Zimmer, C, Wiestler, B, et al. Predicting conversion from clinically isolated syndrome to multiple sclerosis–an imaging-based machine learning approach. NeuroImage. (2019) 21:101593. doi: 10.1016/j.nicl.2018.11.003
24. Goyal, M, Khanna, D, Rana, PS, Khaibullin, T, Martynova, E, Rizvanov, AA, et al. Computational intelligence technique for prediction of multiple sclerosis based on serum cytokines. Front Neurol. (2019) 10:781. doi: 10.3389/fneur.2019.00781
25. Vázquez-Marrufo, M, Sarrias-Arrabal, E, García-Torres, M, Martín-Clemente, R, and Izquierdo, G. A systematic review of the application of machine-learning algorithms in multiple sclerosis. Neurología. (2022) 38:577–90. doi: 10.1016/j.nrleng.2020.10.013
26. Trentzsch, K, Schumann, P, Śliwiński, G, Bartscht, P, Haase, R, Schriefer, D, et al. Using machine learning algorithms for identifying gait parameters suitable to evaluate subtle changes in gait in people with multiple sclerosis. Brain Sci. (2021) 11:1049. doi: 10.3390/brainsci11081049
27. Moazami, F, Lefevre-Utile, A, Papaloukas, C, and Soumelis, V. Machine learning approaches in study of multiple sclerosis disease through magnetic resonance images. Front Immunol. (2021) 12:700582. doi: 10.3389/fimmu.2021.700582
28. Gao, XR, Chiariglione, M, Qin, K, Nuytemans, K, Scharre, DW, Li, YJ, et al. Explainable machine learning aggregates polygenic risk scores and electronic health records for Alzheimer's disease prediction. Sci Rep. (2023) 13:450. doi: 10.1038/s41598-023-27551-1
29. Fayemiwo, MA, Olowookere, TA, Olaniyan, OO, Ojewumi TOOyetade, IS, Freeman, S, et al. Immediate word recall in cognitive assessment can predict dementia using machine learning techniques. Alzheimers Res Ther. (2023) 15:111. doi: 10.1186/s13195-023-01250-5
30. Bhandari, N, Walambe, R, Kotecha, K, and Kaliya, M. Integrative gene expression analysis for the diagnosis of Parkinson's disease using machine learning and explainable AI. Comput Biol Med. (2023) 163:107140. doi: 10.1016/j.compbiomed.2023.107140
31. Li, Q, Yang, X, Xu, J, Guo, Y, He, X, Hu, H, et al. Early prediction of Alzheimer's disease and related dementias using real-world electronic health records. Alzheimers Dement. (2023) 19:3506–18. doi: 10.1002/alz.12967
32. Ostertag, C, Visani, M, Urruty, T, and Beurton-Aimar, M. Long-term cognitive decline prediction based on multi-modal data using Multimodal3DSiameseNet: transfer learning from Alzheimer's disease to Parkinson's disease. Int J Comput Assist Radiol Surg. (2023) 18:809–18. doi: 10.1007/s11548-023-02866-6
33. Ponce de Leon-Sanchez, ER, Dominguez-Ramirez, OA, Herrera-Navarro, AM, Rodriguez-Resendiz, J, Paredes-Orta, C, and Mendiola-Santibañez, JD. A deep learning approach for predicting multiple sclerosis. Micromachines (Basel). (2023) 14:749. doi: 10.3390/mi14040749
34. Russo, M, Amboni, M, Barone, P, Pellecchia, MT, Romano, M, Ricciardi, C, et al. Identification of a gait pattern for detecting mild cognitive impairment in Parkinson's disease. Sensors. (2023) 23:1985. doi: 10.3390/s23041985
35. Syam, V, Safal, S, Bhutia, O, Singh, AK, Giri, D, Bhandari, SS, et al. A non-invasive method for prediction of neurodegenerative diseases using gait signal features. Procedia Comput Sci. (2023) 218:1529–41. doi: 10.1016/j.procs.2023.01.131
36. Tan, WY, Hargreaves, C, Chen, C, and Hilal, S. A machine learning approach for early diagnosis of cognitive impairment using population-based data. J Alzheimers Dis. (2023) 91:449–61. doi: 10.3233/JAD-220776
37. Tayyab, M, Metz, LM, Li, DKB, Kolind, S, Carruthers, R, Traboulsee, A, et al. Accounting for uncertainty in training data to improve machine learning performance in predicting new disease activity in early multiple sclerosis. Front Neurol. (2023) 14:1165267. doi: 10.3389/fneur.2023.1165267
38. Tena, A, Clarià, F, Solsona, F, and Povedano, M. Voiceprint and machine learning models for early detection of bulbar dysfunction in ALS. Comput Methods Prog Biomed. (2023) 229:107309. doi: 10.1016/j.cmpb.2022.107309
39. Mueller, B, Street, WN, Carnahan, RM, and Lee, S. Evaluating the performance of machine learning methods for risk estimation of delirium in patients hospitalized from the emergency department. Acta Psychiatr Scand. (2023) 147:493–505. doi: 10.1111/acps.13551
40. Swarnalatha, R. A greedy optimized intelligent framework for early detection of Alzheimer's disease using EEG signal. Comput Intell Neurosci. (2023) 2023:4808841. doi: 10.1155/2023/4808841
41. Ahmed, H, Soliman, H, El-Sappagh, S, Abuhmed, T, and Elmogy, M. Early detection of Alzheimer's disease based on Laplacian re-decomposition and XGBoosting. Comput Syst Sci Engin. (2023) 46:2773–95. doi: 10.32604/csse.2023.036371
42. Ahmed, H, Soliman, H, and Elmogy, M. Early detection of Alzheimer's disease using single nucleotide polymorphisms analysis based on gradient boosting tree. Comput Biol Med. (2022) 146:105622. doi: 10.1016/j.compbiomed.2022.105622
43. García-Gutierrez, F, Díaz-Álvarez, J, Matias-Guiu, JA, Pytel, V, Matías-Guiu, J, Cabrera-Martín, MN, et al. GA-MADRID: design and validation of a machine learning tool for the diagnosis of Alzheimer's disease and frontotemporal dementia using genetic algorithms. Med Biol Eng Comput. (2022) 60:2737–56. doi: 10.1007/s11517-022-02630-z
44. Kavitha, C, Mani, V, Srividhya, SR, Khalaf, OI, and Tavera Romero, CA. Early-stage Alzheimer's disease prediction using machine learning models. Front Public Health. (2022) 10:853294. doi: 10.3389/fpubh.2022.853294
45. Kumar, MR, Vekkot, S, Lalitha, S, Gupta, D, Govindraj, VJ, Shaukat, K, et al. Dementia detection from speech using machine learning and deep learning architectures. Sensors. (2022) 22:9311. doi: 10.3390/s22239311
46. Li, Z, Li, H, Braimah, A, Dillman, JR, Parikh, NA, and He, L. A novel ontology-guided attribute partitioning ensemble learning model for early prediction of cognitive deficits using quantitative structural MRI in very preterm infants. NeuroImage. (2022) 260:119484. doi: 10.1016/j.neuroimage.2022.119484
47. Liu, S, Schlesinger, JJ, McCoy, AB, Reese, TJ, Steitz, B, Russo, E, et al. New onset delirium prediction using machine learning and long short-term memory (LSTM) in electronic health record. J Am Med Inform Assoc. (2022) 30:120–31. doi: 10.1093/jamia/ocac210
48. Mehra, N, and Mittal, P. Design and implementation of ML model for early diagnosis of Parkinson’s disease using gait data analysis in IoT environment. Int J Adv Comput Sci Appl. (2022) 13:580–9. doi: 10.14569/IJACSA.2022.0130769
49. Nelson, CA, Bove, R, Butte, AJ, and Baranzini, SE. Embedding electronic health records onto a knowledge network recognizes prodromal features of multiple sclerosis and predicts diagnosis. J Am Med Inform Assoc. (2022) 29:424–34. doi: 10.1093/jamia/ocab270
50. Penfold, RB, Carrell, DS, Cronkite, DJ, Pabiniak, C, Dodd, T, Glass, AM, et al. Development of a machine learning model to predict mild cognitive impairment using natural language processing in the absence of screening. BMC Med Inform Decis Mak. (2022) 22:129. doi: 10.1186/s12911-022-01864-z
51. Qiu, A, Xu, L, and Liu, C. Predicting diagnosis 4 years prior to Alzheimer's disease incident. Neuroimage Clin. (2022) 34:102993. doi: 10.1016/j.nicl.2022.102993
52. Revathi, A, Kaladevi, R, Ramana, K, Jhaveri, RH, Rudra Kumar, M, Kumar, SP, et al. Early detection of cognitive decline using machine learning algorithm and cognitive ability test. Secur Commun Networks. (2022) 2022:1–13. doi: 10.1155/2022/4190023
53. Riad, R, Lunven, M, Titeux, H, Cao, XN, Hamet Bagnou, J, Lemoine, L, et al. Predicting clinical scores in Huntington's disease: a lightweight speech test. J Neurol. (2022) 269:5008–21. doi: 10.1007/s00415-022-11148-1
54. Schumann, P, Scholz, M, Trentzsch, K, Jochim, T, Śliwiński, G, Malberg, H, et al. Detection of fall risk in multiple sclerosis by gait analysis-an innovative approach using feature selection ensemble and machine learning algorithms. Brain Sci. (2022) 12:1477. doi: 10.3390/brainsci12111477
55. Sun, X, Ren, X, Zhang, J, Nie, Y, Hu, S, Yang, X, et al. Discovering miRNAs associated with multiple sclerosis based on network representation learning and deep learning methods. Front Genet. (2022) 13:899340. doi: 10.3389/fgene.2022.899340
56. Tufail, AB, Anwar, N, Othman, MTB, Ullah, I, Khan, RA, Ma, YK, et al. Early-stage Alzheimer's disease categorization using PET neuroimaging modality and convolutional neural networks in the 2D and 3D domains. Sensors. (2022) 22:4609. doi: 10.3390/s22124609
57. Wang, Q, and Xu, R. AANet: attentive all-level fusion deep neural network approach for multi-modality early Alzheimer's disease diagnosis. AMIA Annu Symp Proc. (2022) 2022:1125–34. doi: 10.1016/j.matpr.2021.03.06118
58. Yu, X, Srivastava, S, Huang, S, Hayden, EY, Teplow, DB, and Xie, YH. The feasibility of early Alzheimer's disease diagnosis using a neural network hybrid platform. Biosensors. (2022) 12:753. doi: 10.3390/bios12090753
59. Zhang, W, Shen, J, Wang, Y, Cai, K, Zhang, Q, and Cao, M. Blood SSR1: a possible biomarker for early prediction of Parkinson's disease. Front Mol Neurosci. (2022) 15:762544. doi: 10.3389/fnmol.2022.762544
60. Valencia, L, Clèrigues, A, Valverde, S, Salem, M, Oliver, A, Rovira, À, et al. Evaluating the use of synthetic T1-w images in new T2 lesion detection in multiple sclerosis. Front Neurosci. (2022) 16:954662. doi: 10.3389/fnins.2022.954662
61. Adhikari, S, Thapa, S, Naseem, U, Singh, P, Huo, H, Bharathy, G, et al. Exploiting linguistic information from Nepali transcripts for early detection of Alzheimer's disease using natural language processing and machine learning techniques. Int J Hum Comput Stud. (2022) 160:102761. doi: 10.1016/j.ijhcs.2021.102761
62. Alkenani, AH, Li, Y, Xu, Y, and Zhang, Q. Predicting Alzheimer's disease from spoken and written language using fusion-based stacked generalization. J Biomed Inform. (2021) 118:103803. doi: 10.1016/j.jbi.2021.103803
63. Etminani, K, Soliman, A, Davidsson, A, Chang, JR, Martínez-Sanchis, B, Byttner, S, et al. A 3D deep learning model to predict the diagnosis of dementia with Lewy bodies, Alzheimer's disease, and mild cognitive impairment using brain 18F-FDG PET. Eur J Nucl Med Mol Imaging. (2022) 49:563–84. doi: 10.1007/s00259-021-05483-0
64. Herzog, NJ, and Magoulas, GD. Brain asymmetry detection and machine learning classification for diagnosis of early dementia. Sensors. (2021) 21:778. doi: 10.3390/s21030778
65. James, C, Ranson, JM, Everson, R, and Llewellyn, DJ. Performance of machine learning algorithms for predicting progression to dementia in memory clinic patients. JAMA Netw Open. (2021) 4:e2136553. doi: 10.1001/jamanetworkopen.2021.36553
66. Kleiman, MJ, Barenholtz, E, and Galvin, JE. Screening for early-stage Alzheimer's disease using optimized feature sets and machine learning. J Alzheimers Dis. (2021) 81:355–66. doi: 10.3233/JAD-201377
67. Noh, B, Yoon, H, Youm, C, Kim, S, Lee, M, Park, H, et al. Prediction of decline in global cognitive function using machine learning with feature ranking of gait and physical fitness outcomes in older adults. Int J Environ Res Public Health. (2021) 18:11347. doi: 10.3390/ijerph182111347
68. Roshanzamir, A, Aghajan, H, and Soleymani, BM. Transformer-based deep neural network language models for Alzheimer's disease risk assessment from targeted speech. BMC Med Inform Decis Mak. (2021) 21:92. doi: 10.1186/s12911-021-01456-3
69. Sánchez-Reyna, AG, Celaya-Padilla, JM, Galván-Tejada, CE, Luna-García, H, Gamboa-Rosales, H, Ramirez-Morales, A, et al. Multimodal early Alzheimer's detection, a genetic algorithm approach with support vector machines. Healthcare. (2021) 9:971. doi: 10.3390/healthcare9080971
70. Singhania, U, Tripathy, B, Hasan, MK, Anumbe, NC, Alboaneen, D, Ahmed, FRA, et al. A predictive and preventive model for onset of Alzheimer's disease. Front Public Health. (2021) 9:751536. doi: 10.3389/fpubh.2021.751536
71. Syed, ZS, Syed, MSS, Lech, M, and Pirogova, E. Automated recognition of Alzheimer's dementia using bag-of-deep-features and model Ensembling. IEEE Access. (2021) 9:88377–90. doi: 10.1109/ACCESS.2021.3090321
72. Tsai, CF, Chen, CC, Wu, EH, Chung, CR, Huang, CY, Tsai, PY, et al. A machine-learning-based assessment method for early-stage neurocognitive impairment by an immersive virtual supermarket. IEEE Trans Neural Syst Rehabil Eng. (2021) 29:2124–32. doi: 10.1109/TNSRE.2021.3118918
73. Uehara, Y, Ueno, SI, Amano-Takeshige, H, Suzuki, S, Imamichi, Y, Fujimaki, M, et al. Non-invasive diagnostic tool for Parkinson's disease by sebum RNA profile with machine learning. Sci Rep. (2021) 11:18550. doi: 10.1038/s41598-021-98423-9
74. Venugopalan, J, Tong, L, Hassanzadeh, HR, and Wang, MD. Multimodal deep learning models for early detection of Alzheimer's disease stage. Sci Rep. (2021) 11:3254. doi: 10.1038/s41598-020-74399-w
75. Wang, L, Laurentiev, J, Yang, J, Lo, YC, Amariglio, RE, Blacker, D, et al. Development and validation of a deep learning model for earlier detection of cognitive decline from clinical notes in electronic health records. JAMA Netw Open. (2021) 4:e2135174. doi: 10.1001/jamanetworkopen.2021.35174
76. Zhu, Y, Kim, M, Zhu, X, Kaufer, D, and Wu, G. Long range early diagnosis of Alzheimer's disease using longitudinal MR imaging data. Med Image Anal. (2021) 67:101825. doi: 10.1016/j.media.2020.101825
77. Mehmood, A, Yang, S, Feng, Z, Wang, M, Ahmad, AS, Khan, R, et al. A transfer learning approach for early diagnosis of Alzheimer's disease on MRI images. Neuroscience. (2021) 460:43–52. doi: 10.1016/j.neuroscience.2021.01.002
78. Montolío, A, Martín-Gallego, A, Cegoñino, J, Orduna, E, Vilades, E, Garcia-Martin, E, et al. Machine learning in diagnosis and disability prediction of multiple sclerosis using optical coherence tomography. Comput Biol Med. (2021) 133:104416. doi: 10.1016/j.compbiomed.2021.104416
79. Sudharsan, M, and Thailambal, G. Alzheimer's disease prediction using machine learning techniques and principal component analysis (PCA). Materials Today. (2021) 133:104416. doi: 10.1016/j.matpr.2021.03.061
80. Wang, R, Luo, W, Liu, Z, Liu, W, Liu, C, Liu, X, et al. Integration of the extreme gradient boosting model with electronic health records to enable the early diagnosis of multiple sclerosis. Mult Scler Relat Disord. (2021) 47:102632. doi: 10.1016/j.msard.2020.102632
81. Zeng, A, Rong, H, Pan, D, Jia, L, Zhang, Y, Zhao, F, et al. Discovery of genetic biomarkers for Alzheimer's disease using adaptive convolutional neural networks ensemble and genome-wide association studies. Interdiscip Sci. (2021) 13:787–800. doi: 10.1007/s12539-021-00470-3
82. Peng, Y, Zheng, Y, Tan, Z, Liu, J, Xiang, Y, Liu, H, et al. Prediction of unenhanced lesion evolution in multiple sclerosis using radiomics-based models: a machine learning approach. Mult Scler Relat Disord. (2021) 53:102989. doi: 10.1016/j.msard.2021.102989
83. Lee, J, Ko, W, Kang, E, and Suk, HI. A unified framework for personalized regions selection and functional relation modeling for early MCI identification. NeuroImage. (2021) 236:118048. doi: 10.1016/j.neuroimage.2021.118048
84. Buegler, M, Harms, R, Balasa, M, Meier, IB, Exarchos, T, Rai, L, et al. Digital biomarker-based individualized prognosis for people at risk of dementia. Alzheimers Dement. (2020) 12:e12073. doi: 10.1002/dad2.12073
85. Alkhatib, R, Diab, MO, Corbier, C, and El Badaoui, M. Machine learning algorithm for gait analysis and classification on early detection of Parkinson. IEEE Sensors Letters. (2020) 4:1–4. doi: 10.1109/LSENS.2020.2994938
86. Gill, S, Mouches, P, Hu, S, Rajashekar, D, MacMaster, FP, Smith, EE, et al. Using machine learning to predict dementia from neuropsychiatric symptom and neuroimaging data. J Alzheimers Dis. (2020) 75:277–88. doi: 10.3233/JAD-191169
87. Cheng, J, Liu, HP, Lin, WY, and Tsai, FJ. Identification of contributing genes of Huntington's disease by machine learning. BMC Med Genet. (2020) 13:176. doi: 10.1186/s12920-020-00822-w
88. Pan, X, Phan, TL, Adel, M, Fossati, C, Gaidon, T, Wojak, J, et al. Multi-view separable pyramid network for AD prediction at MCI stage by (18)F-FDG brain PET imaging. IEEE Trans Med Imaging. (2021) 40:81–92. doi: 10.1109/TMI.2020.3022591
89. Balea-Fernandez, FJ, Martinez-Vega, B, Ortega, S, Fabelo, H, Leon, R, Callico, GM, et al. Analysis of risk factors in dementia through machine learning. J Alzheimers Dis. (2021) 79:845–61. doi: 10.3233/JAD-200955
90. Jauk, S, Kramer, D, Großauer, B, Rienmüller, S, Avian, A, Berghold, A, et al. Risk prediction of delirium in hospitalized patients using machine learning: an implementation and prospective evaluation study. J Am Med Inform Assoc. (2020) 27:1383–92. doi: 10.1093/jamia/ocaa113
91. Liu, J, Tan, G, Lan, W, and Wang, J. Identification of early mild cognitive impairment using multi-modal data and graph convolutional networks. BMC Bioinform. (2020) 21:123. doi: 10.1186/s12859-020-3437-6
92. Martynova, E, Goyal, M, Johri, S, Kumar, V, Khaibullin, T, Rizvanov, AA, et al. Serum and cerebrospinal fluid cytokine biomarkers for diagnosis of multiple sclerosis. Mediat Inflamm. (2020) 2020:1–10. doi: 10.1155/2020/2727042
93. Pelka, O, Friedrich, CM, Nensa, F, Mönninghoff, C, Bloch, L, Jöckel, KH, et al. Sociodemographic data and APOE-ε4 augmentation for MRI-based detection of amnestic mild cognitive impairment using deep learning systems. PLoS One. (2020) 15:e0236868. doi: 10.1371/journal.pone.0236868
94. Wang, W, Lee, J, Harrou, F, and Sun, Y. Early detection of Parkinson's disease using deep learning and machine learning. IEEE Access. (2020) 8:147635–46. doi: 10.1109/ACCESS.2020.3016062
95. Zhu, F, Li, X, McGonigle, D, Tang, H, He, Z, Zhang, C, et al. Analyze informant-based questionnaire for the early diagnosis of senile dementia using deep learning. IEEE J Transl Eng Health Med. (2020) 8:1–6. doi: 10.1109/JTEHM.2019.2959331
96. Magesh, PR, Myloth, RD, and Tom, RJ. An explainable machine learning model for early detection of Parkinson's disease using LIME on DaTSCAN imagery. Comput Biol Med. (2020) 126:104041. doi: 10.1016/j.compbiomed.2020.104041
97. Marziyeh Ghoreshi Beyrami, S, and Ghaderyan, P. A robust, cost-effective and non-invasive computer-aided method for diagnosis three types of neurodegenerative diseases with gait signal analysis. Measurement. (2020):156. doi: 10.1016/j.measurement.2020.107579
98. Roca, P, Attye, A, Colas, L, Tucholka, A, Rubini, P, Cackowski, S, et al. Artificial intelligence to predict clinical disability in patients with multiple sclerosis using FLAIR MRI. Diagn Interv Imaging. (2020) 101:795–802. doi: 10.1016/j.diii.2020.05.009
99. Park, C, Ha, J, and Park, S. Prediction of Alzheimer's disease based on deep neural network by integrating gene expression and DNA methylation dataset. Expert Syst Appl. (2020) 140:112873. doi: 10.1016/j.eswa.2019.112873
100. Braga, D, Madureira, AM, Coelho, L, and Ajith, R. Automatic detection of Parkinson’s disease based on acoustic analysis of speech. Eng Appl Artif Intell. (2019) 77:148–58. doi: 10.1016/j.engappai.2018.09.018
101. Abd El Hamid, MM, Mabrouk, MS, and Omar, YM. Developing an early predictive system for identifying genetic biomarkers associated to Alzheimer’s disease using machine learning techniques. Biomed Engin. (2019) 31:1950040. doi: 10.4015/S1016237219500406
102. Bi, X, and Wang, H. Early Alzheimer's disease diagnosis based on EEG spectral images using deep learning. Neural Netw. (2019) 114:119–35. doi: 10.1016/j.neunet.2019.02.005
103. Maitra, S, Hossain, T, Al-Sakin, A, Inzamamuzzaman, S, Or Rashid, MM, and Hasan, SS. A gender-neutral approach to detect early Alzheimer's disease applying a three-layer NN. Int J Adv Comput Sci Appl. (2019) 10:530–8. doi: 10.14569/IJACSA.2019.0100368
104. Pérez Del Palomar, A, Cegoñino, J, Montolío, A, Orduna, E, Vilades, E, Sebastián, B, et al. Swept source optical coherence tomography to early detect multiple sclerosis disease. The use of machine learning techniques. PLoS One. (2019) 14:e0216410. doi: 10.1371/journal.pone.0216410
105. Petrone, PM, Casamitjana, A, Falcon, C, Artigues, M, Operto, G, Cacciaglia, R, et al. Prediction of amyloid pathology in cognitively unimpaired individuals using voxel-wise analysis of longitudinal structural brain MRI. Alzheimers Res Ther. (2019) 11:72. doi: 10.1186/s13195-019-0526-8
106. Prasad, BS. Supervised machine learning algorithms for early diagnosis of Alzheimer’s disease. Int J Recent Technol Engin. (2019) 8:7964–7. doi: 10.35940/ijrte.c6646.098319
107. Rehman, RZU, Del Din, S, Guan, Y, Yarnall, AJ, Shi, JQ, and Rochester, L. Selecting clinically relevant gait characteristics for classification of early Parkinson's disease: a comprehensive machine learning approach. Sci Rep. (2019) 9:17269. doi: 10.1038/s41598-019-53656-7
108. Shen, T, Jiang, J, Lu, J, Wang, M, Zuo, C, Yu, Z, et al. Predicting Alzheimer disease from mild cognitive impairment with a deep belief network based on 18F-FDG-PET images. Mol Imaging. (2019) 18:1536012119877285. doi: 10.1177/1536012119877285
109. Spasov, S, Passamonti, L, Duggento, A, Liò, P, and Toschi, N. A parameter-efficient deep learning approach to predict conversion from mild cognitive impairment to Alzheimer's disease. NeuroImage. (2019) 189:276–87. doi: 10.1016/j.neuroimage.2019.01.031
110. Peña-Bautista, C, Durand, T, Oger, C, Baquero, M, Vento, M, and Cháfer-Pericás, C. Assessment of lipid peroxidation and artificial neural network models in early Alzheimer disease diagnosis. Clin Biochem. (2019) 72:64–70. doi: 10.1016/j.clinbiochem.2019.07.008
111. Saccà, V, Sarica, A, Novellino, F, Barone, S, Tallarico, T, Filippelli, E, et al. Evaluation of machine learning algorithms performance for the prediction of early multiple sclerosis from resting-state FMRI connectivity data. Brain Imaging Behav. (2019) 13:1103–14. doi: 10.1007/s11682-018-9926-9
112. Cai, Z, Gu, J, Wen, C, Zhao, D, Huang, C, Huang, H, et al. An intelligent Parkinson's disease diagnostic system based on a chaotic bacterial foraging optimization enhanced fuzzy KNN approach. Comput Math Methods Med. (2018) 2018:1–24. doi: 10.1155/2018/2396952
113. Amoroso, N, La Rocca, M, Monaco, A, Bellotti, R, and Tangaro, S. Complex networks reveal early MRI markers of Parkinson's disease. Med Image Anal. (2018) 48:12–24. doi: 10.1016/j.media.2018.05.004
114. Bairagi, V. EEG signal analysis for early diagnosis of Alzheimer disease using spectral and wavelet based features. Int J Inf Technol. (2018) 10:403–12. doi: 10.1007/s41870-018-0165-5
115. Parisi, L, RaviChandran, N, and Manaog, ML. Feature-driven machine learning to improve early diagnosis of Parkinson's disease. Expert Syst Appl. (2018) 110:182–90. doi: 10.1016/j.eswa.2018.06.003
116. Grassi, M, Perna, G, Caldirola, D, Schruers, K, Duara, R, and Loewenstein, DA. A clinically-translatable machine learning algorithm for the prediction of Alzheimer's disease conversion in individuals with mild and premild cognitive impairment. J Alzheimers Dis. (2018) 61:1555–73. doi: 10.3233/JAD-170547
117. He, L, Li, H, Holland, SK, Yuan, W, Altaye, M, and Parikh, NA. Early prediction of cognitive deficits in very preterm infants using functional connectome data in an artificial neural network framework. Neuroimage Clin. (2018) 18:290–7. doi: 10.1016/j.nicl.2018.01.032
118. Schütze, M, de Souza, CD, de Paula, JJ, Malloy-Diniz, LF, Malamut, C, Mamede, M, et al. Use of machine learning to predict cognitive performance based on brain metabolism in Neurofibromatosis type 1. PLoS One. (2018) 13:e0203520. doi: 10.1371/journal.pone.0203520
119. Oh, J, Cho, D, Park, J, Na, SH, Kim, J, Heo, J, et al. Prediction and early detection of delirium in the intensive care unit by using heart rate variability and machine learning. Physiol Meas. (2018) 39:035004. doi: 10.1088/1361-6579/aaab07
120. Lu, D, Popuri, K, Ding, GW, Balachandar, R, and Beg, MF. Multiscale deep neural network based analysis of FDG-PET images for the early diagnosis of Alzheimer's disease. Med Image Anal. (2018) 46:26–34. doi: 10.1016/j.media.2018.02.002
121. Mishra, S, Gordon, BA, Su, Y, Christensen, J, Friedrichsen, K, Jackson, K, et al. AV-1451 PET imaging of tau pathology in preclinical Alzheimer disease: defining a summary measure. NeuroImage. (2017) 161:171–8. doi: 10.1016/j.neuroimage.2017.07.050
122. Pereira, T, Lemos, L, Cardoso, S, Silva, D, Rodrigues, A, Santana, I, et al. Predicting progression of mild cognitive impairment to dementia using neuropsychological data: a supervised learning approach using time windows. BMC Med Inform Decis Mak. (2017) 17:110. doi: 10.1186/s12911-017-0497-2
123. Peng, B, Wang, SH, Zhou, ZY, Liu, Y, Tong, BT, Zhang, T, et al. A multilevel-ROI-features-based machine learning method for detection of morphometric biomarkers in Parkinson's disease. Neurosci Lett. (2017) 651:88–94. doi: 10.1016/j.neulet.2017.04.034
124. Zhu, Y, Zhu, X, Kim, M, Shen, D, and Wu, G. Early diagnosis of Alzheimer's disease by joint feature selection and classification on temporally structured support vector machine. Med Image Comput Comput Assist Interv. (2016) 9900:264–72. doi: 10.1007/978-3-319-46720-7_31
125. Cabral, C, Morgado, PM, Campos Costa, D, and Silveira, M. Predicting conversion from MCI to AD with FDG-PET brain images at different prodromal stages. Comput Biol Med. (2015) 58:101–9. doi: 10.1016/j.compbiomed.2015.01.003
126. Moradi, E, Pepe, A, Gaser, C, Huttunen, H, and Tohka, J. Machine learning framework for early MRI-based Alzheimer's conversion prediction in MCI subjects. NeuroImage. (2015) 104:398–412. doi: 10.1016/j.neuroimage.2014.10.002
127. Suk, HI, Lee, SW, and Shen, D. Latent feature representation with stacked auto-encoder for AD/MCI diagnosis. Brain Struct Funct. (2015) 220:841–59. doi: 10.1007/s00429-013-0687-3
128. Maestú, F, Peña, JM, Garcés, P, González, S, Bajo, R, Bagic, A, et al. A multicenter study of the early detection of synaptic dysfunction in mild cognitive impairment using magnetoencephalography-derived functional connectivity. NeuroImage. (2015) 9:103–9. doi: 10.1016/j.nicl.2015.07.011
129. Mandal, I, and Sairam, N. New machine-learning algorithms for prediction of Parkinson's disease. Int J Syst Sci. (2014) 45:647–66. doi: 10.1080/00207721.2012.724114
130. Mundt, JC, Freed, DM, and Greist, JH. Lay person-based screening for early detection of Alzheimer's disease: development and validation of an instrument. J Gerontol B Psychol Sci Soc Sci. (2000) 55:P163–70. doi: 10.1093/geronb/55.3.P163
131. Velazquez, M, and Lee, Y. Random forest model for feature-based Alzheimer's disease conversion prediction from early mild cognitive impairment subjects. PLoS One. (2021) 16:e0244773. doi: 10.1371/journal.pone.0244773
132. Minhad, KN, Farayez, A, Reaz, MBI, Bhuiyan, MAS, Samdin, SB, and Miraz, MH. Early diagnosis of dementia patients by SPADE activity prediction algorithm. Measurement Sci Rev. (2023) 23:86–91. doi: 10.2478/msr-2023-0011
Keywords: neurodegenerative disorder, neurocognitive disorder, machine learning, early detection, AI
Citation: Yousefi M, Akhbari M, Mohamadi Z, Karami S, Dasoomi H, Atabi A, Sarkeshikian SA, Abdoullahi Dehaki M, Bayati H, Mashayekhi N, Varmazyar S, Rahimian Z, Asadi Anar M, Shafiei D and Mohebbi A (2024) Machine learning based algorithms for virtual early detection and screening of neurodegenerative and neurocognitive disorders: a systematic-review. Front. Neurol. 15:1413071. doi: 10.3389/fneur.2024.1413071
Received: 06 April 2024; Accepted: 05 November 2024;
Published: 09 December 2024.
Edited by:
Rafeed Alkawadri, University of Pittsburgh Medical Center, United StatesReviewed by:
Bin Yi, Army Medical University, ChinaCopyright © 2024 Yousefi, Akhbari, Mohamadi, Karami, Dasoomi, Atabi, Sarkeshikian, Abdoullahi Dehaki, Bayati, Mashayekhi, Varmazyar, Rahimian, Asadi Anar, Shafiei and Mohebbi. This is an open-access article distributed under the terms of the Creative Commons Attribution License (CC BY). The use, distribution or reproduction in other forums is permitted, provided the original author(s) and the copyright owner(s) are credited and that the original publication in this journal is cited, in accordance with accepted academic practice. No use, distribution or reproduction is permitted which does not comply with these terms.
*Correspondence: Mahsa Asadi Anar, bWFoc2EuYm96QGdtYWlsLmNvbQ==; Daniel Shafiei, ZGFuaWVsc2hhZmllaS5tZEBnbWFpbC5jb20=
‡ORCID: Matin Akhbari, https://orcid.org/0009-0005-6286-8125
Mahsa Asadi Anar, https://orcid.org/0000-0002-5772-2472
Daniel Shafiei, https://orcid.org/0000-0003-0615-7765
†These authors have contributed equally to this work and share first authorship
Disclaimer: All claims expressed in this article are solely those of the authors and do not necessarily represent those of their affiliated organizations, or those of the publisher, the editors and the reviewers. Any product that may be evaluated in this article or claim that may be made by its manufacturer is not guaranteed or endorsed by the publisher.
Research integrity at Frontiers
Learn more about the work of our research integrity team to safeguard the quality of each article we publish.