- 1Ningbo Institute of Materials Technology and Engineering, Chinese Academy of Sciences, Ningbo, China
- 2University of Chinese Academy of Sciences, Beijing, China
- 3Ningbo Cixi Institute of Biomedical Engineering, Ningbo, China
- 4Department of Neurological Rehabilitation, Ningbo Rehabilitation Hospital, Ningbo, China
- 5Robotics Institute, Ningbo University of Technology, Ningbo, China
Introduction: Upper limb rehabilitation assessment plays a pivotal role in the recovery process of stroke patients. The current clinical assessment tools often rely on subjective judgments of healthcare professionals. Some existing research studies have utilized physiological signals for quantitative assessments. However, most studies used single index to assess the motor functions of upper limb. The fusion of surface electromyography (sEMG) and functional near-infrared spectroscopy (fNIRS) presents an innovative approach, offering simultaneous insights into the central and peripheral nervous systems.
Methods: We concurrently collected sEMG signals and brain hemodynamic signals during bilateral elbow flexion in 15 stroke patients with subacute and chronic stages and 15 healthy control subjects. The sEMG signals were analyzed to obtain muscle synergy based indexes including synergy stability index (SSI), closeness of individual vector (CV) and closeness of time profile (CT). The fNIRS signals were calculated to extract laterality index (LI).
Results: The primary findings were that CV, SSI and LI in posterior motor cortex (PMC) and primary motor cortex (M1) on the affected hemisphere of stroke patients were significantly lower than those in the control group (p < 0.05). Moreover, CV, SSI and LI in PMC were also significantly different between affected and unaffected upper limb movements (p < 0.05). Furthermore, a linear regression model was used to predict the value of the Fugl-Meyer score of upper limb (FMul) (R2 = 0.860, p < 0.001).
Discussion: This study established a linear regression model using force, CV, and LI features to predict FMul scale values, which suggests that the combination of force, sEMG and fNIRS hold promise as a novel method for assessing stroke rehabilitation.
1 Introduction
Stroke is a neurological disorder characterized by vascular blockages, posing a severe threat to human health and life. Upper limb motor impairments are observed in 73 to 88% of first-time stroke survivors and 55 to 75% of chronic stroke patients (1). Therefore, a rational and effective strategy for assessing motor function is crucial for guiding the rehabilitation of stroke patients. In clinical practice, the Fugl-Meyer score of upper limb (FMul) is often used to evaluate upper limb motor function in stroke patients. However, the accuracy of this scale is typically dependent on the experience and subjective assessment of healthcare professionals. The quantitative assessment model that can elucidate the upper limb recovery process is necessary for better organizing rehabilitation strategies and enhancing overall recovery. Advanced imaging techniques have provided valuable information for diagnosis and functional prognosis (2).
In recent years, many studies have explored the use of various physiological signal indices for quantitative assessment of motor function in stroke patients, including surface electromyography (sEMG), electroencephalogram (EEG), and functional near-infrared spectroscopy (fNIRS), among others (3–5). However, many types of single evaluation index have better assessment performance over a specific time period, but could be less effective when covering long-term rehabilitation recovery process (6, 7). Therefore, most studies have strict requirements regarding the time of onset are imposed when selecting patients, often focusing on subacute periods within 3 weeks (4), subacute stage within 8 weeks (3) or chronic phases exceeding two months (5). Applying different assessment indices or parameters to patients with different onset times, while potentially increasing accuracy, also significantly complicates the assessment process, and it remains uncertain whether time-based categorization is the most reasonable approach.
Pino et al. (8) has proposed an assessment model based on structural reserve, the bimodal balance-recovery model, where structural reserve may be influenced by the severity of clinical damage, the integrity of brain regions, age, and other factors. Therefore, for a more precise assessment of patients with varying onset times and degrees of damage, a multi-dimensional evaluation is required. Both muscle synergy indices and indices of brain region activation are entry points for many studies to quantitatively assess stroke patients. A study of a set of upper extremity isometric strength tasks shows different muscle synergies in mild to severe stroke patients (9). Another study found that muscle synergy-related indices were positively correlated with function of neuromuscular control at the joint and task levels in stroke patients (5). These early studies strongly suggest that muscle synergy analysis based on sEMG may be a promising approach for assessing motor function in stroke patients. The fNIRS offers advantages such as non-invasiveness, mobility, resistance to motion and electromagnetic interference, high spatial resolution, and the ability to facilitate long-term monitoring, making it conducive to researching changes in cerebral hemodynamics during muscle contraction tasks (7, 10). fNIRS is also increasingly used to assess motor function or motor rehabilitation training effects (2, 11). Upper limb motor is the result of the coordination of the nervous system with the corresponding muscles. During the process of movement, there is varying degrees of information exchange between the brain and muscles, and motor dysfunction in stroke patients is due to problems in the brain’s control of muscle pathways (12, 13). The advantages of the fNIRS device make it very appropriate for the acquisition of cerebral hemodynamic changes during upper limb movement. Combined with the acquisition of sEMG signals, it can simultaneously obtain neurological information of the brain and muscles, which is helpful for analyzing the brain-muscle characteristic during movement. Although, few studies have analyzed sEMG signal and fNIRS signal of changes in cortical blood oxygen concentration simultaneously. Based on our knowledge, there is no previous research using them to the assessment of motor function in stroke. More recently, a significant positive correlation has been found between changes of muscle activation based on sEMG and cortical network dynamics based on fNIRS during isometric elbow contractions in healthy subjects (14). Therefore, combining fNIRS with sEMG likely provide a new perspective on stroke rehabilitation assessment.
In this study, sEMG and fNIRS were applied to extract effective information on peripheral muscle contraction and brain region activation, respectively, during specific motor tasks. Therefore, the aim of the present study was to explore muscle synergy indices and interhemispheric balance indices to co-assess upper limb motor function in stroke patients. We hypothesize that a linear regression model built from sEMG, fNIRS, and force indices collectively provide a robust assessment of upper limb motor function in patients.
2 Materials and methods
2.1 Subjects
This study encompassed two groups: stroke patients and healthy control group. The inclusion criteria for stroke patients were as follows: (1) first unilateral stroke; (2) possession of cognitive abilities required for task execution; (3) FMul score for the affected upper limb ranging from 10 to 66 out of a maximum score of 66; (4) time since stroke onset between 7 days and 2 years. Exclusion criteria included: (1) visual defects or achromatopsia; (2) presence of other neurological disorders such as Parkinson’s or Alzheimer’s disease. Following these inclusion and exclusion criteria, a total of 15 stroke patients (average age 56.27 ± 18.49 years, including 4 females) and 15 age-matched healthy control subjects (average age 52.20 ± 12.94 years, including 8 females) were recruited from the Rehabilitation Hospital of Ningbo. All participants provided written informed consent to participate in this study, which was approved by the Ethics Committee of the Rehabilitation Hospital of Ningbo in accordance with the Declaration of Helsinki (2023-16).
Furthermore, prior to the experiment, trained therapists assessed the motor function of the stroke patients using common clinical rating scales, including the FMul, Fugl-Meyer score of arm (FMarm) and Brunnstrom Scale (BS). The clinical characteristics of the stroke patients are presented in Table 1.
2.2 Motor task paradigm
Referring to a similar paradigm of using fNIRS to probe brain function in patients (7), participants were seated comfortably in a designated chair, facing a computer monitor, with both arms resting on the chair’s armrests, palms facing upward, as depicted in Figure 1. The arm to be moved during the task was securely fastened to the armrest using a 5 cm wide, slightly elastic strap to prevent the elbow from lifting off the armrest during elbow flexion. Additionally, a non-extendable strap connected to a dynamometer was secured around the wrist of the arm to be moved, with the dynamometer fixed to the chair’s leg. The force signal is recorded at a sampling frequency of 10 Hz and synchronized with the sEMG equipment. The force sensor is used for real-time feedback of actual force magnitude to the subjects during paradigm tasks and for subsequent sEMG data segmentation and screening of valid data.
Before the formal commencement of the task, participants were instructed to avoid unnecessary movements and thoughts once the experiment began. Each participant also had a prior opportunity to familiarize themselves with the task paradigm to ensure a correct understanding and execution of the instructed actions. Initially, participants performed three maximal voluntary contraction (MVC) of elbow flexion, and 30% of the mean of these three values was set as the target force for the actual task. After participants were adequately prepared, the equipment provided the “raise a hand “command, and participants performed 15 s of intermittent isometric muscle contractions of the forearm on the designated arm, guided by an auditory cue with a 0.5 Hz frequency and 2/4 -beat, followed by a 25-s rest period after the equipment issued the “relax” command. Each block comprised six trials. During the task, the monitor in front of the participants displayed a target range of ±5% of the target force, providing feedback on the real-time actual force output. Participants were required to control the force within the target range as accurately as possible. There were two blocks in total, with the first block being completed by the participants’ unaffected arm (the dominant hand for the control group) and the second block by the participants’ affected arm (the non-dominant hand for the control group), with at least a 5-min rest period between the two blocks.
2.3 Data acquisition
2.3.1 sEMG and force data acquisition
In this experiment, the Trigno Wireless EMG System (Delsys Inc., Boston, MA, United States) was used to capture sEMG signals. A dynamometer (SH-100 N, HANDPI company, China, precision of 0.01 N) was used to capture force signals. The sEMG signals and force signals were synchronized using data analysis software and sampled at frequency of 1,925.9 Hz and 10 Hz, respectively. To facilitate subsequent data segmentation, the sEMG data with dynamometer data were synchronized sampled at 10 Hz, ensuring that each sEMG signal was marked with the corresponding dynamometer data at each acquisition point. The recorded muscles included the bilateral anterior deltoid (DA), posterior deltoid (DP), biceps brachii (BI), triceps brachii (TI), and brachioradialis (BIO). Before attaching the sensors, the muscles corresponding to both upper limbs of the participants were wiped twice with 75% alcohol to ensure more accurate signal acquisition. Subsequently, accordance with the guidelines of SENIAM and a therapist, the sensors were affixed to the belly of the respective muscles (15).
2.3.2 Brain hemodynamic data acquisition
This study utilized Optical Topography system with wavelengths of 830 nm and 704 nm (ETG-4100, Hitachi Medical Co., Japan) to collect data on cerebral blood flow changes at a frequency of 10 Hz. Two sets of 3 × 3 probe arrays were employed, with each probe array consisting of 5 sources and 4 detectors, forming measurement channels (for a total of 24 channels). The placement of the probes followed the international 10/20 electrode placement system, with a spacing of 30 mm between sources and detectors. These channels symmetrically covered the bilateral pre-motor cortex (PMC), primary motor cortex (M1), and somatosensory cortex (S1) on both sides, as illustrated in Figure 2. To facilitate data analysis and result presentation, the right hemisphere was defined as the affected hemisphere, and the left and right hemispheric channels of the patients whose lesions were originally located in the left hemisphere were switched.
2.4 sEMG data preprocess and analysis
2.4.1 sEMG data preprocess
The collected raw sEMG signals underwent a series of preprocessing steps. Initially, a fourth-order bandpass Butterworth filter (20-450 Hz) was applied to the signals to eliminate any noise (16). Subsequently, the signals were detrended and rectified to obtain the full-wave rectified sEMG signal. To calculate the envelope of the sEMG signal, a fourth-order low-pass Butterworth filter with a cutoff frequency of 5 Hz was employed (16, 17). Subsequent segmentation of the sEMG signal window was performed on the basis of the change in signal acquired by the dynamometer. Complete sEMG data for a single hand lift from two time periods before dynamometer readings rise to return to baseline. Among them, data with a single lifting cycle of more than 3 s and force readings that deviate from the target value by more than 15% will be excluded. Finally, the segmented data were normalized using both maximum and minimum values and time normalization to facilitate the subsequent extraction of muscle synergy.
2.4.2 Muscle synergy extraction
For the computation of muscle synergy, the Multivariate Curve Resolution Alternating Least Squares (MCR-ALS) algorithm was employed (17). Because compared with other commonly used muscle synergy algorithms, MCR-ALS solves the problem that NMF and ICA algorithms tend to fall into local optimal solutions and lead to poor reproducibility by finding pure variables based on the SMMA algorithm (18). It also improves the problem that SVD-NMF and FA can hardly ensure the natural properties of muscle synergy by alternating least squares (ALS) and imposing non-negative constraints to ensure that the decomposition results are physically meaningful. Therefore, MCR-ALS has better consistency and internal stability in the context of stroke patients with motor impairments (19). The algorithm was applied to the sEMG signals recorded during upper limb movements on both arms for all participants. In carrying out the calculation of indices, it is also necessary to obtain baseline synergies, denoted as VB and TB. This value was also calculated from this algorithm, using data from the healthy control group separately by dominant hand and non-dominant hand and averaged overlay. The synergy decomposition process can be formulated as follows:
In this equation, E represents the residual, V includes the base vectors representing the synergy space, and T is the matrix of time profiles. Parameters p, q, and n correspond to the number of sEMG channels, the time-normalized number of samples (which is 50 in this case), and the number of synergies, respectively.
To determine the most suitable number of synergies, variance accounted for (VAF) was utilized to evaluate the goodness of fit for different numbers of synergies (17, 20). The VAF was calculated using the following equation:
Here, M represents the reconstructed sEMG matrix, and the “mean” operator generates a matrix of the same size as X, with columns representing the mean values of the corresponding columns in X. In this study, when more than half of the participants in both groups had VAF values exceeding 80%, n was defined as the minimum value (5). Consequently, a single synergy (n = 1) was sufficient to meet this criterion, and thus, a single synergy was utilized for subsequent indices calculations.
2.4.3 Index of muscle synergy
In order to find better indices of muscle synergy that could potentially contribute to the quantitative assessment, we selected three indices from three areas, namely SSI, CV and CT. Where SSI was used to assess the stability of muscle synergy between trials in the same subject; CV was used to assess the proximity of the degree of activation of each muscle in the patient to that of the healthy control; and CT was used to assess the similarity of muscle activation on the timeline between the patient and the healthy control. To quantitatively assess the outcomes of muscle synergy decomposition for different participants, several indices were utilized for evaluation. These indices encompass the SSI (21), CV (5) and CT (22). Below, you will find the key formulas for these three indices:
Here, i denotes the total number of unilateral upper limb raising occurrences by a participant. K represents the number of synergies. Vl and Vq represent the base vectors for the l and q instances of limb raising. r denotes the Pearson correlation coefficient.
Since this study employed a single synergy (n = 1), the presented formulas are simplified versions that do not include synergy number variables. In these formulas, i denotes the number of times the limb was raised, while V and VB represent the muscle synergy’s base vectors for individual instances and the mean baseline synergy from all control subjects, respectively. T and TB similarly represent the corresponding coefficient time profiles. I(τ) is the circular cross-correlation function with a time-lag between the two profiles. The range of CV and CT falls between 0 and 1, with 1 indicating the highest degree of similarity.
2.5 Brain hemodynamic data preprocess and analysis
2.5.1 Brain hemodynamic data preprocess
Since oxygenated hemoglobin (HbO) signal data shows more pronounced activation compared to deoxygenated hemoglobin (HbR) signal data (23, 24), all subsequent analyses were conducted exclusively on HbO signals. After obtaining the raw optical intensity signals from the equipment, preprocessing was carried out using data analysis software and codes from the open-source Homer2 toolbox (25). The preprocessing steps encompassed the following: (1) Transformation of the raw optical intensity signals into optical density. (2) Removal of motion artifacts using a kurtosis-based wavelet algorithm (k = 3.3) (26). (3) Application of a third-order bandpass Butterworth filter with a frequency band of 0.01–0.08 Hz to eliminate unwanted physiological signals or environmental noise. (4) Conversion of optical density data into hemoglobin concentration data using the modified Beer–Lambert law (27). (5) Computation of β coefficients for all 24 channels via the General Linear Model (GLM) codes from NIRS-KIT (28) and NIRS-SPM (29), which represented the degree of neural activation during each activity (30).
2.5.2 Brain hemodynamic data preprocess
The Laterality Index (LI) is regarded as a potential index in stroke functional neuroimaging research, enabling the assessment of hemispheric activation balance during upper limb movement, and was used in fNIRS study (7, 13). LI is calculated based on β values and defined as follows:
Here, βC-region represents the average β value for channels in the contralateral brain region. βI-region represents the average β value for channels in the ipsilateral brain region. The “region” includes PMC, M1, and S1, which are the three regions of interest, that is, we calculated the LI values for PMC, M1, and S1. A higher LI value indicates a greater degree of contralateral activation for healthy or contralesional activation for patients, while a smaller or negative LI value indicates more pronounced ipsilateral activation for healthy or ipsilesional activation for patients.
2.6 Regression analysis
This study employed a multiple linear regression approach to examine the correlations between various indices or factors and clinical scale scores. Recognizing that the indices of the unaffected arms of the patients may also provide insights into motor function, the indices LIregion (LIPMC, LIM1 and LIS1), SSI, CV and CT considered for regression analysis included both the unaffected and affected sides. Additionally, we incorporated the ratios of these four indices between the unaffected and affected arms (LIregion-r, SSIr, CV-r and CT-r). It has been suggested in prior studies that the correlation between patient motor function scale scores and various factors such as age and time since the onset of the condition is significant (2). Therefore, in addition to the aforementioned indices, we also incorporated age, time since onset, and the ratio of maximum voluntary contraction force between the affected and unaffected hands Fr into our analysis. To identify the most influential factors for predicting clinical scale scores, this study systematically evaluated all combinations of the 21 predictor variables to determine the four variables that yielded the highest goodness of fit in the multiple linear regression equation. Subsequently, we employed these parameters separately to establish multiple linear regression equations with the FMul scale scores. Finally, scatter plots of fitted and actual scores for healthy individuals and patients were constructed.
2.7 Statistical analyses
Statistical analyses of the various indices were conducted using SPSS (V.27, IBM, United States) and R (4.3.3, Lucent, United States). Initially, the Shapiro–Wilk test and Levene’s test were used to assess the normality and homoscedasticity of the indices data. If both assumptions were met, independent-sample t-tests were employed to analyze significant differences in indices between patients and the control group, paired-sample t-tests were used to analyze differences between indices of both upper limbs, and two-way analysis of variance (ANOVA) was utilized to examine the interaction effects of group (cross-side, namely the pool of affected arm and unaffected arm or non-dominant hand and dominant hand) and arm (cross-group, namely the pool of patients and control subjects). If the assumptions were not met, non-parametric tests such as the Mann–Whitney U test, Wilcoxon signed-rank test, and Scheirer-Ray-Hare test were applied. For the multiple linear regression models, F-tests were used to assess the overall significance of the regression equations, while t-tests were used to evaluate the significance of individual coefficients. A two-tailed analysis was performed, and the level of statistical significance was set at p < 0.05.
3 Results
3.1 Muscle synergy activation mode
Figure 3 illustrates the average muscle synergy patterns for the dominant and non-dominant arms of the control group and the unaffected and affected arms of the patients. From the images, it can be observed that the synergy patterns for the control group’s dominant and non-dominant hands are quite similar, as are those for the unaffected arms of the patients. However, the patient’s affected side, while primarily activating the biceps brachii like the healthy side, also exhibits more activation of the anterior deltoid and brachioradialis muscles. Although there are slight differences in the details of the time profile vectors, the overall patterns are similar.
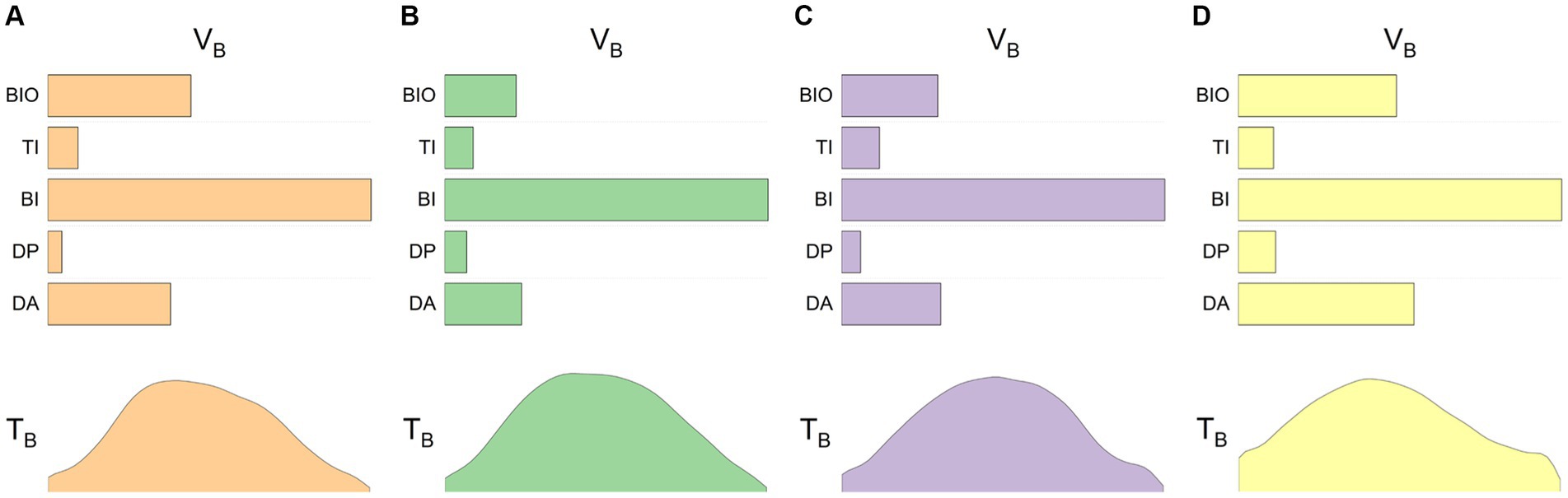
Figure 3. Baseline synergies in control subjects and patients. The four sets of images represent the averaged data for four different conditions: (A) control subjects’ dominant arm, (B) control subjects’ non-dominant arm, (C) stroke patients’ unaffected arm, and (D) stroke patients’ affected arm. The upper half of each image shows the base vectors in the synergy space, while the lower half provides the corresponding time profile information.
3.2 Index of muscle synergy
The statistical analysis results of the SSI, CV and CT indices for both arms of the patients and healthy controls are depicted in Figure 4. Significant differences were found between the patient’s affected arm and the control group’s non-dominant hand in terms of SSI (p < 0.001) and CV (p = 0.007). Differences were also observed in the indices SSI (p = 0.036) and CT (p = 0.023) between the patient’s unaffected and affected sides. Although there was no significant difference in the CV index between the patient’s unaffected and affected arms (p > 0.05), their means were notably different, possibly due to the non-parametric test leading to a loss of information and reduced test efficiency. There was a difference in the CV index between the control group’s healthy and affected arms (p = 0.031). Furthermore, all three indices, SSI (p = 0.005), CV (p = 0.036), and CT (p = 0.013), exhibited significant differences in the cross-group.
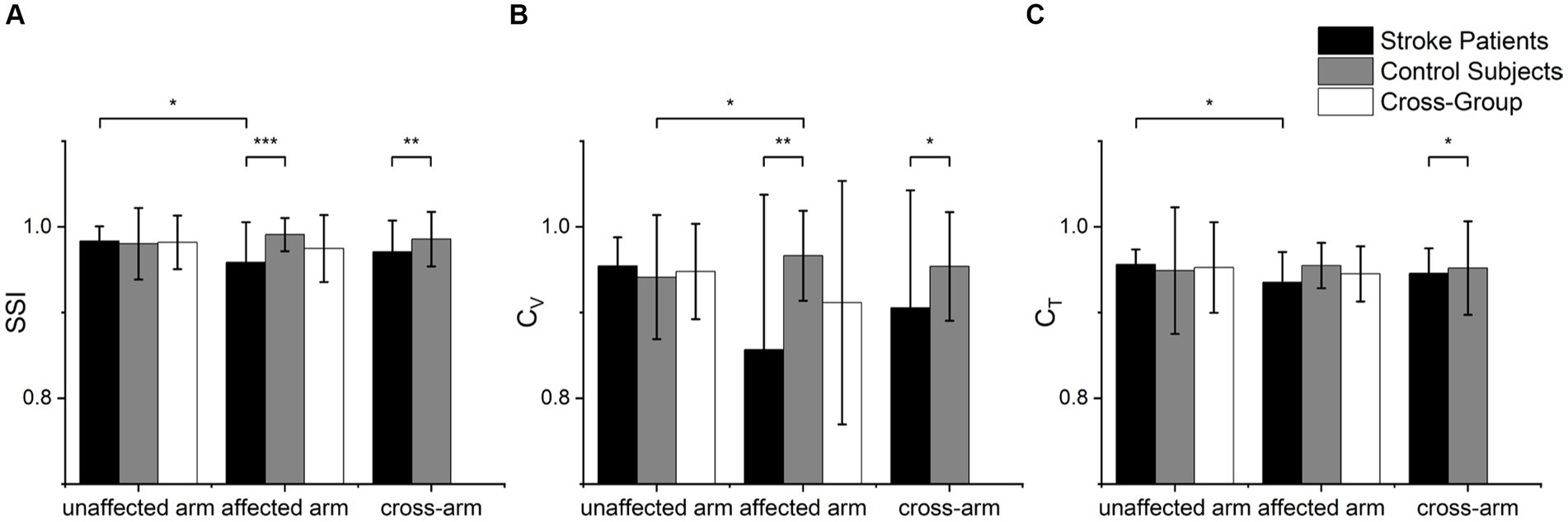
Figure 4. Statistical analysis of muscle synergy indices of (A) SSI, (B) CV and (C) CT. Scheirer-Ray-Hare tests were used to analyze group factors (cross-arm) and arm factors (cross-group), while Mann–Whitney U tests were used to examine significant differences in various index values between different groups. Wilcoxon signed-rank tests were employed to analyze index values within different sides (*p < 0.05, **p < 0.01, ***p < 0.001).
3.3 Cortical activation patterns
Figure 5 shows cortical activation patterns with respect to HbO of β, which was created by BrainNet Viewer (31). Overall, activation appeared to be stronger in contralateral brain regions in all groups and conditions. The healthy control had the highest overall degree of activation during dominant hand movements, while its ipsilateral brain regions had lower activation during non-dominant hand movements compared to all other groups and conditions. Using the Wilcoxon signed-rank test to compare activation between the unaffected and affected arm of the patients revealed no significant differences in any of the brain regions. In contrast, there were two ipsilateral brain regions in the control group that had significantly higher activation during dominant hand movements than non-dominant hand movements, IM1 (p = 0.003) and IPMC (p < 0.001). No significant differences were found when comparing patients and controls using the Mann–Whitney U test. These results suggest that healthy controls had greater differences in activation of brain regions during bilateral upper limb movements, whereas patients had more similar activation of each brain region during bilateral upper limb movements.
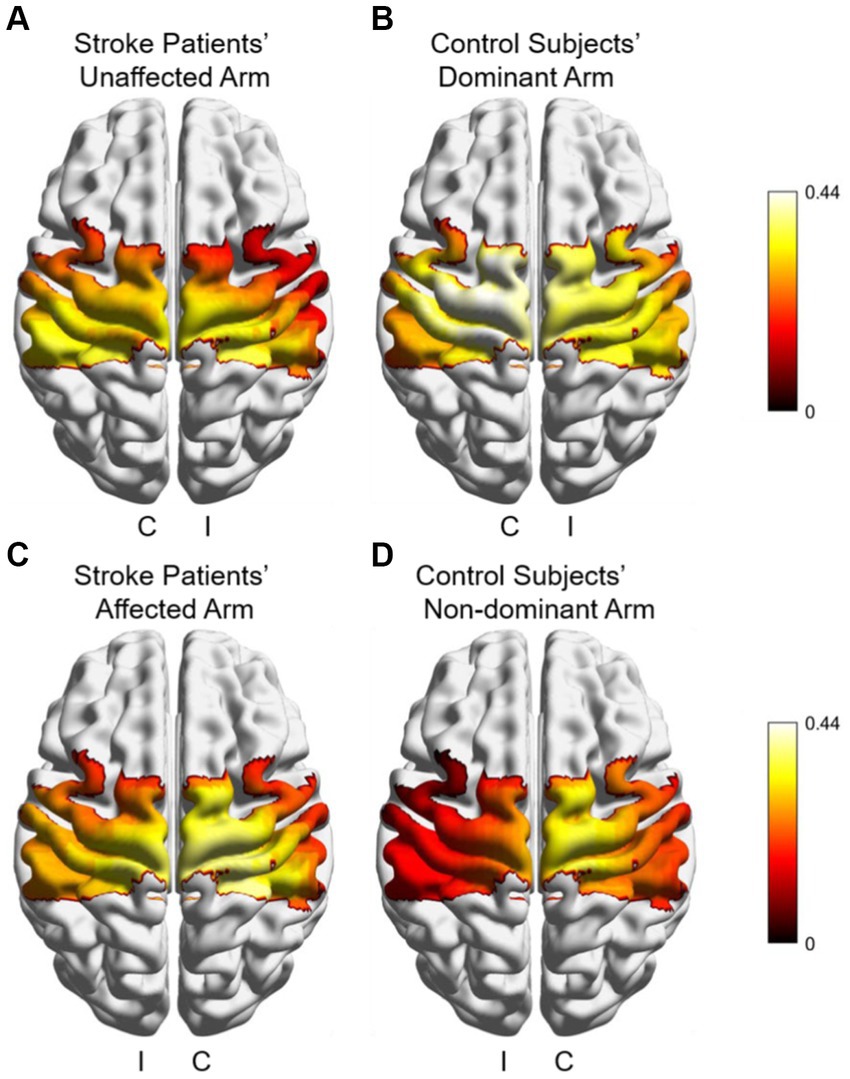
Figure 5. Cortical activation patterns with respect to HbO of β during different arms and groups in subjects (A) Stroke patient’s unaffected arm; (B) controls’ dominant arm; (C) patient’s affected arm, and (D) controls’ non-dominant arm. I: ipsilateral brain region, C: contralateral brain region.
3.4 Index of brain lateralization
The statistical analysis results of LI in different brain regions for both arms of the patients and both arms of the control group are presented in Figure 6. Significant differences were observed in the M1 (p = 0.044) and PMC (p = 0.026) brain regions between the patient’s affected arm and the control group. Additionally, differences were noted in the PMC brain region concerning the patient’s unaffected and affected hemisphere (p = 0.008). However, no differences were observed in the S1 brain region. These results suggest that changes in PMC brain region lateralization may better differentiate between the patient’s unaffected and affected arms or between the patient and the control group in terms of motor function.
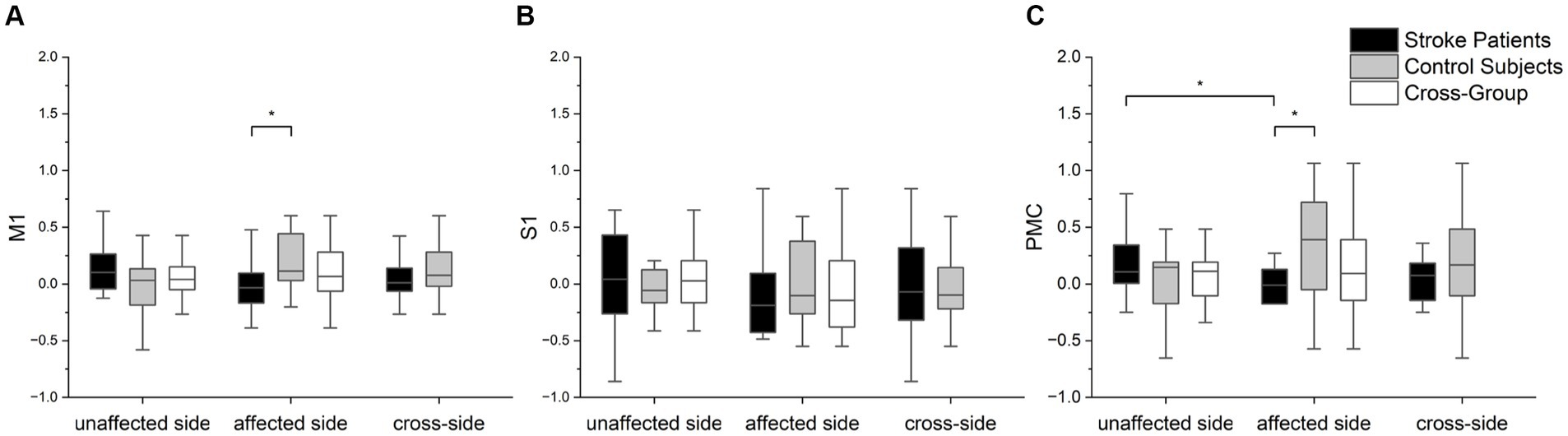
Figure 6. The statistical analysis of three brain regions of interest (LI values) between the two groups and arms: (A) M1, (B) S1, and (C) PMC. Scheirer-Ray-Hare tests were performed with the factor of group (cross-task) and factor of group (cross-group). Mann–Whitney U tests were employed to assess the significance of differences in LI values among different groups for each brain region. Furthermore, we used Wilcoxon signed-rank tests to statistically analyze differences in LI values within groups for different arms. In the box plots within the figure, the lower, middle (bold), and upper horizontal lines represent the 25th, 50th (median), and 75th percentiles, respectively. Significance levels are indicated as *p < 0.05.
3.5 Regression analysis
We conducted regression analysis using the previously mentioned method, exploring all combinations of factors. Among them, the regression model with the highest goodness of fit to the FMul included the following factors: CV-af (af means affected side), LIPMC-un (un means unaffected side,), Fr and CV-r, which the adjusted R2 value stands at 0.860. Correlation analysis revealed no significant correlations between any two of the three predictive factors: CV-af, Fr, and LIPMC-un. Table 2 presents a comprehensive list of all model parameters, featuring B values, β coefficients, and p-values. The regression model showed that the predictive factors CV-r, Fr, and LIPMC-un could all predict upper limb motor function in patients and healthy controls (Figure 7), and the prediction model was significant (p < 0.001). Nine of the fitted score values for healthy people were greater than 60. The regression equation was as follows:
where CV-af represented the CV of affected side, CV-r represented the CV on the unaffected arm to that on the affected side, LIPMC-un represented the LI of unaffected arm in PMC region and Fr represented the ratio of MVC force on the unaffected arm to that on the affected side.
The goodness of fit and significance of the simple linear regression of each of the above parameters with the scale FMul are shown in Table 2. The goodness of fit for each parameter was not high, with the highest being Fr at 0.375.
4 Discussion
This study aimed to explore muscle synergy indices and interhemispheric balance indices to co-assess upper limb motor function in stroke patients. When compared to the healthy control group, stroke patients exhibited significantly reduced sEMG index CV on the affected arm and decreased cerebral blood flow LI values in the region of PMC. Moreover, during movements on the affected side, stroke patients demonstrated lower LI values in the PMC compared to movements on the unaffected side. These results indicate abnormal muscle synergy patterns and altered activation of specific brain regions in stroke patients, which can jointly predict clinical scale scores and serve as assessment indices for stroke.
All three indices of muscle synergy, including SSI (stability), CV (closeness), and CT (time), indicate that the muscle synergy pattern on the affected arm of stroke patients is weaker than that of healthy individuals. In our study, we attempted three different indices: SSI, CV and CT. SSI are often used to assess the stability of muscle synergy between different trials in the same patient. Studies have shown that assessing the stability of the synergy structure vectors V across different trials can be used to evaluate clinical motor function and is positively correlated with clinical assessment scores of upper limb motor function (5, 32). However, the findings showed a low correlation with clinical scores, and we speculate that it may be that some patients are also able to have more stable control of their muscles with diligent rehabilitation. CV and CT indices assess the closeness between muscle synergy decomposition vectors V and time-profile vectors T from the perspective of muscle synergy (22, 33, 34). The statistical results show that both the stability and closeness of the muscle synergy structure vectors V in stroke patients are significantly different from those in the control group, but there is no difference in the time profile vectors T. The time course of the patient’s performance in the index value CT was not significantly different from that of healthy individuals, suggesting that this is not a good indicator to differentiate the patient’s motor function. The CV values are a good response not only to whether the patient’s synergistic pattern of the muscles during motion is closer to that of a healthy individual, but also to the ability to efficiently carry out the muscle utilization, and more severe co-movement is associated with lower clinical scores, and therefore has a better performance in the evaluation model has a better performance. The changes in these muscle synergy vectors not only reveal damage to individual muscle control but also reflect neural reorganization in the brain, which is an important index of motor function recovery after stroke (35).
In the study of brain hemodynamic data, the results showed significant ipsilateral activation of both M1 and PMC during dominant hand compared to non-dominant hand in the control group, and significant differences in LI indices were observed in the PMC and regions between stroke patients and healthy controls. GLM has been shown to be an effective method for examining hemodynamic responses in fNIRS analysis (36–38). In this study, we considered β values as a measure of cortical activation, with higher β values indicating higher activation in the brain regions corresponding to this channel. The activation of ipsilateral M1 and PMC brain regions was significantly higher in the control group during dominant hand movements than during non-dominant hand ones, which may be due to the degree of brain activation related to the redistribution of brain resources (39). In order to achieve more precise force control, the brain regions controlling unilateral movements will be more active during sharp hand movements, whereas skillful reallocation of brain resources is difficult to achieve with non-sharp hand movements. However, the activation of various brain regions during movement on the affected arm is altered in stroke patients, and the recovery of motor function following stroke is closely related to the reconstruction of the motor system in both the contralateral and ipsilateral hemispheres (40). LI, as a quantitative method for functional neuroimaging studies in stroke recovery, describes the contrast in activation levels between contralateral and ipsilateral hemisphere (7, 13). Combined with β values as a measure of activation, LI is suitable for assessing patient motor function. We evaluated LI during movements on the unaffected and affected hemisphere of stroke patients and found significantly lower LI indices in the M1 and PMC regions during affected arm movements compared to non-dominant hand movements in healthy individuals. The M1 plays a key role in improving control of distal arm muscles during movement (41), and the PMC is also involved in the recovery of motor function in chronic stroke patients (42). The decrease in LI in these two brain regions suggests impairment of upper limb function corresponding to these regions (7, 43), resulting in increased activation in the ipsilateral hemisphere during affected arm movements, indicative of a compensatory mode within the recovery model. Interestingly, we also observed that the LI values in the PMC during movements on the unaffected arm of stroke patients were significantly higher than the LI values during affected arm movements. According to Figure 6, the LI values in the PMC during movements on the healthy arm of stroke patients were slightly higher than those in the healthy control group. This may be explained by another recovery model—interhemispheric inhibition.
CV, LI, and Fr as quantitative methods for functional neuroimaging studies in stroke recovery, can predict upper limb motor function in patients. This provides new insights for guiding stroke patient rehabilitation, as well as assessing and predicting the recovery status of patients. In this study, we attempted to fit linear regression equations with various features, including sEMG signals, cerebral blood flow, and force, to FMul scores. The results showed that the equation with the highest goodness of fit (R2 = 0.860) included information from sEMG, cerebral blood flow, and force, indicating that the combination of physiological signals reflected by the brain and muscles during the movement process can better assess patient motor function. This assessment model contains Fr, CV, and LI, which corresponds to the patient’s residual force magnitude, synergistic motor function of the muscles, and hemispheric lateralization of cerebral activation, which is well adapted to the two important indices of residual motion function, and inter-hemispheric cerebral balance, proposed by the bimodal balance-recovery model. Age, days after stroke, and other indices were poorly assessed in this study for stroke patients, which may mean that these factors are weakly related to the assessment of motor function in stroke patients, or it may be that these factors are already expressed by Fr, CV, and LI. However, the goodness of fit for simple linear regression equations with individual features and FMul scores was generally low, suggesting a relatively weak linear correlation between single indices and the scores. A single index may be useful for evaluating a specific range of stroke patients, but it will be much less stable when applied to a wider range of situations, or it may be understood that an increase in the value of this index does not imply an increase in the patient’s actual motor function. This result is consistent with many longitudinal studies, where the LI in stroke patients tends to increase initially after stroke, then decrease around two months to three months (7), with some studies showing a significant average decrease in LI at approximately one week after stroke, followed by an increase after three to six months (44). Some studies have not found enough significant difference in LI (11, 45). Similar patterns have been observed for motor evoked potentials (MEPs) and sEMG (46, 47). At this point, the advantage of co-assessment of multiple indices comes into play. Our model improves the stability and accuracy of the model by extracting the force signal, sEMG signal, and fNIRS signal and by building a multivariate linear assessment model, assigning different weights to each index, so as to minimize the problem that a single feature fails in some situations. In addition, we applied the model to a healthy control group and found that most of the fitting scores were above 60. It was further verified that the physiological indices used in the model had practical significance, meaning that the better-fit scores showed that the patient’s indices were closer to those of a healthy person. In conclusion, the combination of physiological indices in this quantitative assessment model can be well used to predict the score values of the clinical scale FMul. Our next step is to continue to track the patient’s recovery and to try other indices in order to establish a more stable and accurate model.
There are some limitations to this study. Firstly, the sample size was relatively small. To make the analysis results more convincing, future studies should recruit a larger number of stroke patients. Secondly, the paradigm design of this study required patients to have a certain level of motor function to resist gravity and perform lifting movements. Therefore, this study lacked severely impaired patients. Additionally, due to some patients not being able to perform isolated elbow flexion movements effectively, there may be variations in the force generation method, which could potentially influence the results. From an assessment modeling perspective, manual segmentation of the sEMG signal data is required before calculating the muscle synergy metric values, which makes the calculation of the assessment results take some time. Lack of analysis of DeoxyHb change in fNIRS signals, which may result in the absence of valid information. The indices and their algorithms used for modeling in this study are basic, and better indices or algorithms may exist.
5 Conclusion
To the best of our knowledge, this is the first study to combine force, sEMG, and fNIRS features during elbow flexion for stroke assessment. Furthermore, we included both subacute and chronic phase patients in the study. The results indicate that stroke patients exhibit significantly lower muscle synergy structure vector indices (SSI, CV) and reduced LI values in the PMC and M1 regions compared to healthy controls, reflecting potential mechanisms underlying functional impairment in stroke patients. Moreover, we established a linear regression model using CV and LI features to predict FMul scale values. Fr, CV, and LI hold promise as beneficial biomarkers during the stroke motor recovery process.
Data availability statement
The raw data supporting the conclusions of this article will be made available by the authors, without undue reservation.
Ethics statement
The studies involving humans were approved by Ethics Committee of the Rehabilitation Hospital of Ningbo. The studies were conducted in accordance with the local legislation and institutional requirements. The participants provided their written informed consent to participate in this study.
Author contributions
SY: Conceptualization, Data curation, Formal analysis, Methodology, Software, Writing – original draft. LT: Conceptualization, Methodology, Writing – original draft. SG: Investigation, Methodology, Writing – original draft. YM: Methodology, Writing – original draft. JW: Software, Writing – original draft. WL: Project administration, Writing – original draft. JK: Investigation, Writing – original draft. MT: Conceptualization, Resources, Supervision, Writing – review & editing. GZ: Conceptualization, Funding acquisition, Supervision, Writing – review & editing. CS: Conceptualization, Funding acquisition, Methodology, Project administration, Supervision, Writing – review & editing.
Funding
The author(s) declare financial support was received for the research, authorship, and/or publication of this article. This work was supported by National Key R&D Program of China (2022YFC3601700); the Science and Technology Innovation 2025 Major Project of Ningbo (2020Z082, 2020Z022); the Key Research and Development Program of Zhejiang Province (2023C03168); the Natural Science Foundation of Zhejiang Province (LQ23C090005); the Fund of the Director of Ningbo Institute of Materials Technology and Engineering (2021SZKY0205); and the Natural Science Foundation of Ningbo (2022J042, 2023J184).
Acknowledgments
We are grateful to all volunteers for their participation in this study.
Conflict of interest
The authors declare that the research was conducted in the absence of any commercial or financial relationships that could be construed as a potential conflict of interest.
Publisher’s note
All claims expressed in this article are solely those of the authors and do not necessarily represent those of their affiliated organizations, or those of the publisher, the editors and the reviewers. Any product that may be evaluated in this article, or claim that may be made by its manufacturer, is not guaranteed or endorsed by the publisher.
References
1. Coscia, M, Wessel, MJ, Chaudary, U, Millan, JR, Micera, S, Guggisberg, A, et al. Neurotechnology-aided interventions for upper limb motor rehabilitation in severe chronic stroke. Brain. (2019) 142:2182–97. doi: 10.1093/brain/awz181
2. Liang, J, Song, Y, Belkacem, AN, Li, F, Liu, S, Chen, X, et al. Prediction of balance function for stroke based on Eeg and Fnirs features during ankle dorsiflexion. Front Neurosci. (2022) 16:968928. doi: 10.3389/fnins.2022.968928
3. Lamberti, N, Manfredini, F, Nardi, F, Baroni, A, Piva, G, Crepaldi, A, et al. Cortical oxygenation during a motor task to evaluate recovery in subacute stroke patients: a study with near-infrared spectroscopy. Neurol Int. (2022) 14:322–35. doi: 10.3390/neurolint14020026
4. Bartur, G, Pratt, H, and Soroker, N. Changes in mu and Beta amplitude of the Eeg during upper limb movement correlate with motor impairment and structural damage in subacute stroke. Clin Neurophysiol. (2019) 130:1644–51. doi: 10.1016/j.clinph.2019.06.008
5. Li, S, Zhuang, C, Niu, CM, Bao, Y, Xie, Q, and Lan, N. Evaluation of functional correlation of task-specific muscle synergies with motor performance in patients Postsroke. Front Neurol. (2017) 8:337. doi: 10.3389/fneur.2017.00337
6. Calautti, C, and Baron, JC. Functional neuroimaging studies of motor recovery after stroke in adults—a review. Stroke. (2003) 34:1553–66. doi: 10.1161/01.Str.0000071761.36075.A6
7. Delorme, M, Vergotte, G, Perrey, S, Froger, J, and Laffont, I. Time course of sensorimotor cortex reorganization during upper extremity task accompanying motor recovery early after stroke: an Fnirs study. Restor Neurol Neurosci. (2019) 37:207–18. doi: 10.3233/rnn-180877
8. Di Pino, G, Pellegrino, G, Assenza, G, Capone, F, Ferreri, F, Formica, D, et al. Modulation of brain plasticity in stroke: a novel model for neurorehabilitation. Nat Rev Neurol. (2014) 10:597–608. doi: 10.1038/nrneurol.2014.162
9. Roh, J, Rymer, WZ, and Beer, RF. Evidence for altered upper extremity muscle synergies in chronic stroke survivors with mild and moderate impairment. Front Hum Neurosci. (2015) 9:6. doi: 10.3389/fnhum.2015.00006
10. Zimmermann, R, Marchal-Crespo, L, Edelmann, J, Lambercy, O, Fluet, M-C, Riener, R, et al. Detection of motor execution using a hybrid Fnirs-biosignal Bci: a feasibility study. J Neuroeng Rehabil. (2013) 10:10. doi: 10.1186/1743-0003-10-4
11. Xie, H, Li, X, Huang, W, Yin, J, Luo, C, Li, Z, et al. Effects of robot-assisted task-oriented upper limb motor training on neuroplasticity in stroke patients with different degrees of motor dysfunction: a neuroimaging motor evaluation index. Front Neurosci. (2022) 16:957972. doi: 10.3389/fnins.2022.957972
12. Odwyer, NJ, Ada, L, and Neilson, PD. Spasticity and muscle contracture following stroke. Brain. (1996) 119:1737–49. doi: 10.1093/brain/119.5.1737
13. Cramer, SC, Nelles, G, Benson, RR, Kaplan, JD, Parker, RA, Kwong, KK, et al. A functional Mri study of subjects recovered from Hemiparetic stroke. Stroke. (1997) 28:2518–27. doi: 10.1161/01.Str.28.12.2518
14. Wang, X, Luo, Z, Zhang, M, Zhao, W, Xie, S, Wong, SF, et al. The interaction between changes of muscle activation and cortical network dynamics during isometric elbow contraction: a Semg and Fnirs study. Front Bioeng Biotechnol. (2023) 11:1176054. doi: 10.3389/fbioe.2023.1176054
15. Hermens, HJ, Freriks, B, Disselhorst-Klug, C, and Rau, G. Development of recommendations for Semg sensors and sensor placement procedures. J Electromyogr Kinesiol. (2000) 10:361–74. doi: 10.1016/s1050-6411(00)00027-4
16. Al-Timemy, AH, Bugmann, G, Escudero, J, and Outram, N. Classification of finger movements for the dexterous hand prosthesis control with surface electromyography. IEEE J. Biomed. Health Inform. (2013) 17:608–18. doi: 10.1109/jbhi.2013.2249590
17. Ma, Y, Shi, C, Xu, J, Ye, S, Zhou, H, and Zuo, G. A novel muscle synergy extraction method used for motor function evaluation of stroke patients: a pilot study. Sensors. (2021) 21:3833. doi: 10.3390/s21113833
18. Windig, W, and Guilment, J. Interactive self-modeling mixture analysis. Anal Chem. (1991) 63:1425–32. doi: 10.1021/ac00014a016
19. Ma, Y, Ye, S, Zhao, D, Liu, X, Cao, L, Zhou, H, et al. Using different matrix factorization approaches to identify muscle synergy in stroke survivors. Med Eng Phys. (2023) 117:103993. doi: 10.1016/j.medengphy.2023.103993
20. Cheung, VCK, Piron, L, Agostini, M, Silvoni, S, Turolla, A, and Bizzi, E. Stability of muscle synergies for voluntary actions after cortical stroke in humans. Proc Natl Acad Sci USA. (2009) 106:19563–8. doi: 10.1073/pnas.0910114106
21. Wojtara, T, Alnajjar, F, Shimoda, S, and Kimura, H. Muscle synergy stability and human balance maintenance. J Neuroeng Rehabil. (2014) 11:11. doi: 10.1186/1743-0003-11-129
22. Chen, HY, Chen, SC, Chen, JJJ, Fu, LL, and Wang, YL. Kinesiological and kinematical analysis for stroke subjects with asymmetrical cycling movement patterns. J Electromyogr Kinesiol. (2005) 15:587–95. doi: 10.1016/j.jelekin.2005.06.001
23. Strangman, G, Culver, JP, Thompson, JH, and Boas, DA. A quantitative comparison of simultaneous bold Fmri and Nirs recordings during functional brain activation. NeuroImage. (2002) 17:719–31. doi: 10.1006/nimg.2002.1227
24. Bai, Z, Fong, KNK, Zhang, J, and Hu, Z. Cortical mapping of Mirror visual feedback training for unilateral upper extremity: a functional near-infrared spectroscopy study. Brain Behav. (2020) 10:e01489. doi: 10.1002/brb3.1489
25. Huppert, TJ, Diamond, SG, Franceschini, MA, and Boas, DA. Homer: a review of time-series analysis methods for near-infrared spectroscopy of the brain. Appl Opt. (2009) 48:D280–98. doi: 10.1364/ao.48.00d280
26. Chiarelli, AM, Maclin, EL, Fabiani, M, and Gratton, G. A kurtosis-based wavelet algorithm for motion artifact correction of Fnirs data. NeuroImage. (2015) 112:128–37. doi: 10.1016/j.neuroimage.2015.02.057
27. Kocsis, L, Herman, P, and Eke, A. The modified Beer-Lambert law revisited. Phys Med Biol. (2006) 51:N91–8. doi: 10.1088/0031-9155/51/5/n02
28. Hou, X, Zhang, Z, Zhao, C, Duan, L, Gong, Y, Li, Z, et al. Nirs-kit: a Matlab toolbox for both resting-state and task Fnirs data analysis. Neurophotonics. (2021) 8:010802. doi: 10.1117/1.NPh.8.1.010802
29. Chul, J, Tak, S, Jang, KE, Jung, J, and Jang, J. Nirs-Spm: statistical parametric mapping for near-infrared spectroscopy. NeuroImage. (2009) 44:428–47. doi: 10.1016/j.neuroimage.2008.08.036
30. Zhao, C, Li, D, Guo, J, Li, B, Kong, Y, Hu, Y, et al. The neurovascular couplings between electrophysiological and hemodynamic activities in anticipatory selective attention. Cereb Cortex. (2022) 32:4953–68. doi: 10.1093/cercor/bhab525
31. Xia, M, Wang, J, and He, Y. Brainnet viewer: a network visualization tool for human brain Connectomics. PLoS One. (2013) 8:e68910. doi: 10.1371/journal.pone.0068910
32. Tang, L, Chen, X, Cao, S, Wu, D, Zhao, G, and Zhang, X. Assessment of upper limb motor dysfunction for children with cerebral palsy based on muscle synergy analysis. Front Hum Neurosci. (2017) 11:11. doi: 10.3389/fnhum.2017.00130
33. Ambrosini, E, De Marchis, C, Pedrocchi, A, Ferrigno, G, Monticone, M, Schmid, M, et al. Neuro-mechanics of recumbent leg cycling in post-acute stroke patients. Ann Biomed Eng. (2016) 44:3238–51. doi: 10.1007/s10439-016-1660-0
34. Cheung, VCK, d'Avella, A, Tresch, MC, and Bizzi, E. Central and sensory contributions to the activation and Organization of Muscle Synergies during Natural Motor Behaviors. J Neurosci. (2005) 25:6419–34. doi: 10.1523/jneurosci.4904-04.2005
35. Hashiguchi, Y, Ohata, K, Kitatani, R, Yamakami, N, Sakuma, K, Osako, S, et al. Merging and fractionation of muscle synergy indicate the recovery process in patients with hemiplegia: the first study of patients after subacute stroke. Neural Plast. (2016) 2016:1–7. doi: 10.1155/2016/5282957
36. Huppert, TJ. Commentary on the statistical properties of noise and its implication on general linear models in functional near-infrared spectroscopy. Neurophotonics. (2016) 3:010401. doi: 10.1117/1.NPh.3.1.010401
37. Santosa, H, Zhai, X, Fishburn, F, Sparto, PJ, and Huppert, TJ. Quantitative comparison of correction techniques for removing systemic physiological signal in functional near-infrared spectroscopy studies. Neurophotonics. (2020) 7:035009. doi: 10.1117/1.NPh.7.3.035009
38. Yucel, MA, Luhmann, AV, Scholkmann, F, Gervain, J, Dan, I, Ayaz, H, et al. Best practices for fNIRS publications. Neurophotonics. (2021) 8:012101. doi: 10.1117/1.NPh.8.1.012101
39. Li, X, Yin, J, Li, H, Xu, G, Huo, C, Xie, H, et al. Effects of ordered grasping movement on brain function in the performance virtual reality task: a near-infrared spectroscopy study. Front Hum Neurosci. (2022) 16:798416. doi: 10.3389/fnhum.2022.798416
40. Binder, E, Leimbach, M, Pool, E-M, Volz, LJ, Eickhoff, SB, Fink, GR, et al. Cortical reorganization after motor stroke: a pilot study on differences between the upper and lower limbs. Hum Brain Mapp. (2021) 42:1013–33. doi: 10.1002/hbm.25275
41. Mohapatra, S, Harrington, R, Chan, E, Dromerick, AW, Breceda, EY, and Harris-Love, M. Role of Contralesional hemisphere in paretic arm reaching in patients with severe arm paresis due to stroke: a preliminary report. Neurosci Lett. (2016) 617:52–8. doi: 10.1016/j.neulet.2016.02.004
42. Johansen-Berg, H, Rushworth, MFS, Bogdanovic, MD, Kischka, U, Wimalaratna, S, and Matthews, PM. The role of ipsilateral premotor cortex in hand movement after stroke. Proc Natl Acad Sci USA. (2002) 99:14518–23. doi: 10.1073/pnas.222536799
43. Lotze, M, Markert, J, Sauseng, P, Hoppe, J, Plewnia, C, and Gerloff, C. The role of multiple Contralesional motor areas for complex hand movements after internal capsular lesion. J Neurosci. (2006) 26:6096–102. doi: 10.1523/jneurosci.4564-05.2006
44. Marshall, RS, Perera, GM, Lazar, RM, Krakauer, JW, Constantine, RC, and DeLaPaz, RL. Evolution of cortical activation during recovery from corticospinal tract infarction. Stroke. (2000) 31:656–61. doi: 10.1161/01.Str.31.3.656
45. Calautti, C, Leroy, F, Guincestre, JY, Marie, RM, and Baron, JC. Sequential activation brain mapping after subcortical stroked: changes in hemispheric balance and recovery. Neuroreport. (2001) 12:3883–6. doi: 10.1097/00001756-200112210-00005
46. Hesam-Shariati, N, Trinh, T, Thompson-Butel, AG, Shiner, CT, and McNulty, PA. A longitudinal electromyography study of complex movements in Poststroke therapy. 2: changes in coordinated muscle activation. Front Neurol. (2017) 8:277. doi: 10.3389/fneur.2017.00277
Keywords: motor assessment, sEMG, fNIRS, stroke, elbow flexion
Citation: Ye S, Tao L, Gong S, Ma Y, Wu J, Li W, Kang J, Tang M, Zuo G and Shi C (2024) Upper limb motor assessment for stroke with force, muscle activation and interhemispheric balance indices based on sEMG and fNIRS. Front. Neurol. 15:1337230. doi: 10.3389/fneur.2024.1337230
Edited by:
Ping Zhou, University of Health and Rehabilitation Sciences, ChinaReviewed by:
Stephane Perrey, Université de Montpellier, FrancePeng Fang, Chinese Academy of Sciences (CAS), China
Le Li, Northwestern Polytechnical University, China
Copyright © 2024 Ye, Tao, Gong, Ma, Wu, Li, Kang, Tang, Zuo and Shi. This is an open-access article distributed under the terms of the Creative Commons Attribution License (CC BY). The use, distribution or reproduction in other forums is permitted, provided the original author(s) and the copyright owner(s) are credited and that the original publication in this journal is cited, in accordance with accepted academic practice. No use, distribution or reproduction is permitted which does not comply with these terms.
*Correspondence: Min Tang, 2398493251@qq.com; Guokun Zuo, moonstone@nimte.ac.cn; Changcheng Shi, changchengshi@nimte.ac.cn