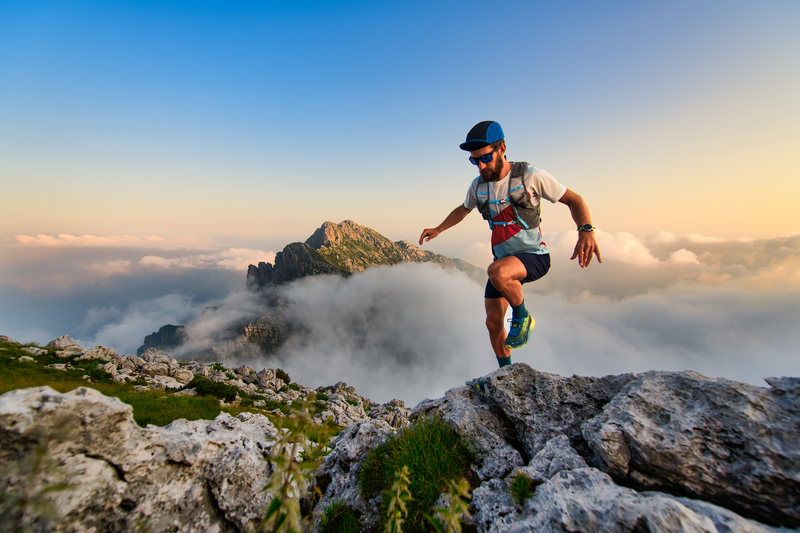
95% of researchers rate our articles as excellent or good
Learn more about the work of our research integrity team to safeguard the quality of each article we publish.
Find out more
ORIGINAL RESEARCH article
Front. Neurol. , 31 January 2024
Sec. Stroke
Volume 15 - 2024 | https://doi.org/10.3389/fneur.2024.1322472
This article is part of the Research Topic Advances and controversies in ischemic stroke management: from prevention to diagnosis and acute treatment View all 95 articles
Background: Observational studies have suggested a possible association between hypothyroidism and increased risk of ischemic stroke. However, a causal relationship remains unclear.
Methods: Data on single nucleotide polymorphisms (SNPs) associated with hypothyroidism and ischemic stroke were sourced from the FinnGens database and the UK Biobank of European descent. Both databases underwent separate two-sample Mendelian randomization (MR) analyses. A subsequent meta-analysis of MR results using a random-effects model was conducted to determine the causal relationship between hypothyroidism and ischemic stroke.
Results: All five analyses indicated a positive causal relationship between hypothyroidism and ischemic stroke. MR analysis of the association between hypothyroidism and ischemic stroke yielded a result of the inverse variance weighted (IVW) method at 4.7411 (1.3598–16.5308), p = 0.0146. The analysis of ischemic stroke (without excluding controls) yielded a result of the IVW method of 4.5713 (1.3570–15.3986), p = 0.0142. MR analysis with cerebral infarction yielded a result of the IVW method at 1.0110 (1.0006–1.0215), p = 0.0373. The MR analysis with cerebrovascular disease sequelae yielded an IVW method result of 2.4556 (1.0291–5.8595), p = 0.0429. Analysis for the sequelae of cerebrovascular disease (without excluding controls) yielded an IVW method result of 2.4217 (1.0217–5.7402), p = 0.0446. No evidence of heterogeneity or horizontal pleiotropy was found. The meta-analysis of the five MR results was 2.24 (1.18–4.26), p = 0.025.
Conclusion: Our two-sample Mendelian randomization study suggested a causal relationship between hypothyroidism and ischemic stroke, indicating that hypothyroidism could be a risk factor for ischemic stroke. However, further studies are required to elucidate the underlying biological mechanisms.
Stroke is the second leading cause of global mortality and the third leading cause of severe disability (1). In 2019, ischemic strokes accounted for a significant proportion of documented stroke incidents, resulting in an estimated 50,000 mortalities and a decline of approximately 910.2 million disability-adjusted life years (DALYs). Low-income countries bear a disproportionately larger burden of this disease compared to high-income countries (1–4). IS caused by arterial occlusion is the cause of most strokes. Given that rapid reperfusion such as, endovascular therapy (EVT) and intravenous thrombolysis, is an effective treatment for neurological injuries induced by acute ischemic stroke (AIS), timely and accurate identification of ischemic injuries is paramount for implementing acute interventions and devising strategies to prevent recurrence. Research indicates that both absolute and relative deficiencies in thyroid hormones can intensify cerebrovascular atherosclerosis, thereby influencing the prevalence and outcomes of cerebrovascular diseases (5–7). Some cross-sectional studies have identified a correlation between the concentration of thyroid-stimulating hormone (TSH) and the intensification of symptoms associated with AIS. Compared with euthyroidism, higher incidence of stroke was associated with high TSH or low free T4 levels (TSH >5.5 mIU/L, free T4 < 0.7 ng/dL) (8). Interestingly, this correlation was not observed in the patients with normal thyroid function. The differential effects of thyroid hormone levels across diverse patient groups suggest a multifaceted interaction between hypothyroidism and ischemic stroke. However, such associations observed in observational studies could be influenced by confounding factors and potential biases in the data collection. Thus, a clearer understanding of this potential causal relationship is pivotal for advancing the prevention and management strategies for ischemic stroke.
Using aggregated data from genome-wide association studies (GWAS), we performed two-sample mendelian randomization (MR) analysis to investigate the association between hypothyroidism and ischemic stroke. Rooted in human genetics, this approach utilizes single nucleotide polymorphisms (SNPs) as instrumental variables (IVs) (9). This method employs SNPs as IVs (9), which, in the context of GWAS, are associated with exposure phenomena (9). The MR analysis approach aims to clarify the causal relationship between exposure and outcomes, minimizing the biases present in traditional randomized controlled trials (10).
In this study, we utilized two-sample MR analysis to investigate the relationship between hypothyroidism (exposure) and ischemic stroke (outcome) using SNPs as IVs. To ensure the reliability of MR analysis, three critical assumptions must be met: (1) SNPs are significantly associated with exposure; that is, hypothyroidism. (2) The SNPs were not associated with any potential confounders. (3) SNPs influenced ischemic stroke risk solely via their association with hypothyroidism. We determined the causal link between hypothyroidism and ischemic stroke using distinct outcome databases in the MR analysis. A subsequent meta-analysis provided a consolidated estimate, as illustrated in Figure 1.
Figure 1. (A) Basic assumptions of Mendelian randomization. Assumption 1: SNPs were closely associated with exposure. Assumption 2: SNPs were not associated with any potential confounders. Assumption 3: SNPs are only linked to the outcome through exposure. (B) The study design of our MR analysis. (C) Flowchart of the study process. IVW, inverse variance weighted; MR, Mendelian randomization; MR-Egger, MR-Egger regression, SNPs, single nucleotide polymorphisms.
We sourced genetic summary data from two prominent GWAS databases, the FinnGens database and UK Biobank (UKB), as detailed in Table 1. From the UKB,1 we procured hypothyroidism-related GWAS data (inquiry code: ukb-b-19732), which comprised of 462,933 participants of European descent, covering a total of 9,851,867 SNPs. Additionally, the cerebral infarction GWAS summary statistics (inquiry code: ukb-d-I63) from the UKB included 361,194 participants of European origin and 10,889,323 SNPs. The FinnGens database,2 we accessed two GWAS datasets on ischemic stroke. The first (inquiry code: finn-b-I9_STR_EXH) involved 212,774 individuals and contained 16,380,445 SNPs, whereas the second (inquiry code: finn-b-I9_STR_EXH_EXNONE) consisted of 218,792 individuals and covered 16,380,466 SNPs. Two additional datasets related to cerebrovascular disease sequelae from FinnGens include the first (inquiry code: finn-b-I9_SEQULAE) with 207,706 individuals and 16,380,409 SNPs, and the second (inquiry code: finn-b-I9_SEQULAE_EXNONE) with 218,792 individuals and 16,380,466 SNPs. For all datasets, we gathered details on several SNPs, including effect frequency (EAF), effect size (β), effect allele (EA), and p-value. As all the data were publicly accessible, no additional ethical clearance was required. The genetic background of all study participants was of European origin, minimizing the potential bias from ethnic confounding factors.
First, genetic polymorphisms that were substantially correlated with hypothyroidism on a genome-wide scale (p < 5 × 10−10) were employed as IVs. To ensure the independence of these IVs and eliminate potential bias from linkage disequilibrium (LD), we set an r-squared threshold of less than 0.0001. This meticulous approach effectively restricted the genetic distance to a maximum of 10,000 kilobases (kb), thereby enhancing the robustness and precision of our methodology. Subsequently, SNPs that exhibited a significant correlation with exposure elements were screened using the F-statistic of SNPs, and strong IVs were recognized by satisfying F > 10. Information on hypothyroidism and ischemic stroke outcomes was extracted using GWAS to determine the relationship between SNPs satisfying Hypotheses 1, 2, and 3 and outcomes. Next, the data extracted from the two databases of exposure and outcome variables were combined and collated, and palindromic sequences were removed to ensure that all SNPs were the same effector allele.
To explore the potential relationships between the specified variables, we conducted a two-sample MR analysis using five analytical techniques: inverse variance weighted (IVW), MR-Egger regression, weighted median (WME) (11), simple mode, and the weighted mode (11). Our primary approach, the IVW method, assessed the direct association between hypothyroidism and ischemic stroke. Cochran’s Q test was used to evaluate the heterogeneity among singular genetic variance estimations. When the p-value from Cochran’s Q test was below 0.05, we adopted the random effects model within IVW for the final MR analysis; otherwise, the fixed-effects model was selected (12). Outcomes in the study were presented using odds ratios (OR) and 95% confidence intervals (CI), constituting the statistical framework for interpretation. The establishment of a p-value threshold of less than 0.05 served as the demarcation line, delineating statistically significant differences from those that fall within the realm of statistical insignificance. We visualized our results using scatter and forest plots, highlighting the relationship between hypothyroidism-associated SNPs and ischemic stroke. Forest plots further facilitated the exploration of potential multiplicity and heterogeneity. While examining the symmetry in the funnel plots, an assessment of the stability of the results was attainable. In the final stage of the analysis, the results yielded from the IVW method were meticulously scrutinized through the employment of multinomial residuals and outliers, utilizing the MR-PRESSO (13) technique. This specialized model underwent careful examination and calibration, particularly with respect to horizontal multinomials and outliers, to ensure a robust and precise interpretation of underlying relationships.
To determine the causative link between hypothyroidism and ischemic stroke, we sourced and assessed data from separate outcome databases. The datasets for ischemic stroke and its variants (no controls excluded), along with cerebrovascular disease sequelae and its variants (no controls excluded), were derived from the FinnGens database. In contrast, data specific to cerebral infarction were obtained from the UKB. MR analyses were independently conducted for each database. Subsequent to these individual MR analyses, the results of the IVW method were aggregated into a meta-analysis. Based on the detected heterogeneity (I2), models for analysis were chosen, and a fixed-effects model was adopted when heterogeneity was below 50%, whereas a random-effects model was utilized if this metric exceeded the given threshold. This methodological approach allowed us to generate a more comprehensive and nuanced overall estimate by incorporating the inherent variations inherent in each study.
To evaluate the potential for horizontal multiplicity, we used the MR-Egger intercept term test, which has p > 0.05, indicating that horizontal multiplicity is missing (13). Through the application of the Egger-intercept method, as referenced in prior research (14), the analysis provided evidence for the absence of lateral pleiotropy, a phenomenon that could have potentially skewed the results. The statistical insignificance marked by a p-value greater than 0.05 further substantiates this finding, reinforcing the robustness of the result and aligning with the expectations of the study’s hypothesis. The MR-PRESSO test, utilized to identify horizontal pleiotropy anomalies, produced a p-value above 0.05, further affirming the non-existence of horizontal pleiotropy and the presence of outliers. The influence of each SNP on the outcomes was evaluated by sequentially omitting individual SNPs using the “leave-one-out” method (15). Cochran’s Q test was employed to evaluate heterogeneity within singular genetic variance estimations, with p > 0.05, denoting an absence of heterogeneity. All analytical procedures were diligently executed in the R software environment, specifically 4.1.3 version, esteemed for reliability in statistical analyses. For the MR investigation, we employed the “Two-Sample-MR” and “MR-PRESSO” packages, both of which are recognized for their specialized functionalities tailored for genetic causal inference tasks. Additionally, the “Meta” package was judiciously employed to conduct the meta-analysis, streamlining the integration and synthesis of results across different studies and thereby enhancing the rigor and comprehensiveness of our findings.
This study used two-sample MR analysis to investigate the direct influence of hypothyroidism on susceptibility to ischemic stroke and its manifestations such as cerebral infarction and other cerebrovascular disease consequences. Ultimately, 64 SNPs were used as IVs for hypothyroidism and ischemic stroke, 64 SNPs were used as IVs for hypothyroidism and ischemic stroke (without exclusion of controls), 66 SNPs were used as IVs for hypothyroidism and cerebral infarction, 64 SNPs were used as IVs for hypothyroidism and sequelae of cerebrovascular disease, and 64 SNPs as IVs for hypothyroidism and sequelae of cerebrovascular disease (without excluding controls).
A comprehensive analysis was conducted using five different MR studies to examine the effects of hypothyroidism on five types of ischemic stroke. Using the scatter plots shown in Figure 2, we evaluated the direct causal relationship between hypothyroidism and various aspects of ischemic stroke (Table 2, Figure 3). The analysis illuminated a consistent and positive causal connection across all the investigations. Specifically, MR analysis exploring the relationship between hypothyroidism and ischemic stroke presented an OR of 4.7411 (95% CI:1.3598–16.5308, p = 0.0146) using the IVW method. The same relationship was further validated without excluding controls, resulting in an OR of 4.5713 (95% CI, 1.3570–15.3986; p = 0.0142). Additional MR analyses assessed correlations with specific manifestations, such as cerebral infarction (OR = 1.0110; 95% CI:1.0006–1.0215, p = 0.0373) and sequelae of cerebrovascular disease (OR = 2.4556; 95% CI:1.0291–5.8595, p = 0.0429). The latter was assessed in scenarios with and without controls, registering an OR of 2.4217 (95% CI,1.0217–5.7402, p = 0.0446). These findings further substantiate the underlying hypothesis, reinforcing the causal effect of hypothyroidism on the various dimensions of ischemic stroke. Intriguingly, with the exception of the MR outcome between hypothyroidism and cerebral infarction, the other four MR investigations indicated that the MR-Egger, WME, simple mode, and weighted mode analyses failed to reach statistical significance. The MR analysis results between hypothyroidism and cerebral infarction suggested that the MR-Egger and WME analysis results were in the same direction as the IVW analysis results, and none of the simple mode and weighted mode analysis results showed statistical significance. This discrepancy might stem from the inherent capabilities of the IVW methodology to handle variations in sample size, inherent statistical heterogeneity, or potential unidentified confounders. Meanwhile, a meta-analysis of the five MR study IVW outputs revealed pronounced heterogeneity (I2 = 80%, p = 0.001), prompting the utilization of the random-effects model. The statistical significance in the IVW results of the five MR analyses was confirmed by meta-analysis [2.24 (1.18–4.26), p = 0.025, Figure 4], following this adjustment. These findings substantiate the hypothesized existence of a causal effect, further supporting the conclusions drawn from the study. Despite the observed heterogeneity, potentially due to database variations, the overarching conclusions of the meta-analysis remained undeterred, thereby validating the conclusions of this study.
Figure 2. Scatterplots of the causal effect of hypothyroidism and ischemic stroke complications in primary MR. Analyses were conducted using IVW, MR-Egger regression, weighted median method, simple mode, weighted mode. The slope of each line corresponds to the estimated MR effect per method. IVW, inverse variance weighted; MR, Mendelian randomization; MR-Egger, MR-Egger regression. (A) Ischemic stroke; (B) ischemic stroke (no controls excluded); (C) cerebral infarction; (D) cerebrovascular disease sequelae; (E) cerebrovascular disease sequelae (without excluding controls).
Figure 3. MR effect for hypothyroidism and ischemic stroke complications. MR, Mendelian randomization. (A) Ischemic stroke; (B) ischemic stroke (no controls excluded); (C) cerebral infarction; (D) cerebrovascular disease sequelae; (E) cerebrovascular disease sequelae (without excluding controls).
The results of the five MR sensitivity analyses are shown in Table 3. The Cochran Q tests consistently yielded p-values greater than 0.05 for all the IVW outcomes, indicating an absence of heterogeneity in our MR investigations. The egger-intercept results, with p > 0.05, signify no evidence of horizontal pleiotropy in the study findings. Similarly, the MR-PRESSO results detailed in Table 4 display p > 0.05, indicating the absence of outliers and horizontal pleiotropy. A graphical representation in the form of a funnel plot, presented in Figure 5, shows a symmetrical distribution of causal effect estimates. This symmetry indicates an unbiased estimation, reaffirming the reliability of the SNPs used as IVs. Moreover, the resilience of our findings was verified through a “Leave-one-out” sensitivity analysis. During the “Leave-one-out” sensitivity analysis, it was discerned that the direct relationship estimations remained robust, unaffected by the inclusion or exclusion of any individual SNPs. This consistency was maintained even when each SNPs was sequentially omitted, as shown in Figure 6. All five results showed no heterogeneity or pleiotropy in the MR analysis between hypothyroidism and ischemic stroke.
Figure 5. The funnel plots of MR Results between hypothyroidism and ischemic stroke. MR, Mendelian randomization. (A) Ischemic stroke; (B) ischemic stroke (no controls excluded); (C) cerebral infarction; (D) cerebrovascular disease sequelae; (E) cerebrovascular disease sequelae (without excluding controls).
Figure 6. The “Leave-one-out” sensitivity analysis of MR Results between hypothyroidism and ischemic stroke. MR, Mendelian randomization. (A) Ischemic stroke; (B) ischemic stroke (no controls excluded); (C) cerebral infarction; (D) cerebrovascular disease sequelae; (E) cerebrovascular disease sequelae (without excluding controls).
In the current study, the potential direct relationship between hypothyroidism and ischemic stroke was investigated using MR analysis. The MR analysis included five different outcome variables, and a comprehensive meta-analysis revealed a significant positive correlation between hypothyroidism and ischemic stroke.
Ischemic strokes is a notable factor that contributes to global morbidity and mortality. Ischemic stroke arises from the disruption of cerebral blood flow due to thrombosis or embolism, which leads to hypoxia and nutrient deprivation in the brain tissue, in turn causing severe neurological disability (16, 17). Its main etiological factors include endothelial dysfunction and atherosclerosis. Hypothyroidism is characterized by the thyroid gland’s inability to produce adequate thyroid hormone, essential for meeting the body’s metabolic needs. Hypothyroidism leads to a variety of clinical signs and symptoms, which are frequently nonspecific. Due to significant clinical variability, its diagnosis predominantly relies on biochemical markers. Overt hypothyroidism is characterized by elevated TSH and low free thyroid hormone levels, while mild or subclinical forms present with high TSH but normal free thyroid hormone levels (18). It has been suggested that DNA methylation of genes that regulate lipid concentrations in vivo may affect the evolution of ischemic stroke through fatty acid metabolic pathways and lipid modulation (19, 20). Furthermore, hypothyroidism and subclinical hypothyroidism may promote increases in low-density lipoprotein (LDL) (21) and total cholesterol (TC) levels. Such an elevation can exacerbate blood pressure (22), foster the development of insulin resistance, and ultimately induce obesity (22, 23). As a result, all of the above factors may increase the risk of atherosclerosis and impact stroke incidence. A meta-analysis demonstrated that for subjects with AIS, compared to those with normal levels of triiodothyronine (T3), patients with low T3 showed significant stroke severity in terms of NIHSS scores (MD = 3.18; 95% CI = 2.74–3.63; I2 = 61.9%) (24). In a substantial number of retrospective studies, the conspicuous impact of diminished serum T3 levels on the functional or recovery prospects of individuals suffering from AIS has been corroborated, with a particular emphasis on the elderly population (25, 26). A strong association was observed between reduced levels of free triiodothyronine (FT3) at the time of admission and unfavorable outcomes, as demonstrated by OR of 0.348 and a 95% CI of 0.18 to 0.72 (p = 0.007) (27). Furthermore, elevated TSH levels may be associated with higher mortality (28) and depression at the time of admission in individuals afflicted with stroke (29). In contrast, a meta-analysis revealed no substantial correlation between TSH concentration and ischemic heart disease or cerebrovascular events (30). These findings indicate a potential connection between untreated subclinical hypothyroidism and stroke events, although this association was not significant (29). These results contradict the findings of the previous studies. This discrepancy may be attributable to the impact of confounding variables and limited sample sizes in conventional observational investigations. However, the current MR study mitigates the influence of these confounding factors by utilizing large-scale GWAS data, thereby bolstering the confidence in the results.
The effects of hypothyroidism on ischemic stroke can be attributed to several potential mechanisms. First, the activation of NOD-like receptor thermal protein domain-associated protein 3 (NLRP3) inflammasomes may be intimately linked to the homeostatic imbalance of thyroid hormone (TH) (31, 32). Concurrently, studies have demonstrated that T3 treatment curbs hypoxia-induced DNA methylation and apoptosis, diminishes histone modifications, and provides protection to primary cortical neurons in hypoxic environments (33–35). In addition, T3 regulates various mechanisms of neuronal plasticity (36), stimulates astrocyte fatty acid oxidation, and augments neuroprotection and functional outcomes after stroke (37). Serum FT3 levels less than 4.38 pmol/L acted as a substantial prognostic indicator (sensitivity:78%; specificity:73%; AUC:0.77) (27). A comprehensive retrospective study illustrated that both hyperthyroidism and hypothyroidism might elevate the risk of stroke (38) and identified a unique inverted U-shaped correlation between high or low FT4 levels and overall cerebral perfusion (39) (nonlinear p = 0.002, correlation p = 0.024). This correlation suggests that hypothyroidism might amplify the risk of stroke via suboptimal cerebral circulation (39, 40). Based on MR analysis outcomes, hypothyroidism may potentially increase the risk of ischemic stroke. Therefore, vigilant monitoring of thyroid function may offer an effective preventive strategy against ischemic stroke.
This study provides genetic evidence to confirm that hypothyroidism increases the risk of ischemic stroke, as ascertained through two-sample MR analysis. This study has several strengths. First, it serves as a trailblazer in MR analysis. Extensive GWAS data were utilized in this innovative approach. This study aimed to examine the causal link between hypothyroidism and ischemic stroke. Second, MR analysis is effective in diminishing the impact of confounding factors by leveraging genetic variants that are constant and largely independent of environmental influences. Finally, MR analysis addresses the issue of reverse causation found in observational studies, effectively sidestepping the effects of reverse causation due to the unidirectional influence of genetic variants on phenotypes.
Nevertheless, our investigation possesses certain limitations. First, the results may not be generalizable to other populations as the study sample was exclusively drawn from Europe. Second, despite our best efforts to mitigate it, we cannot completely rule out the presence of pleiotropic factors such as horizontal pleiotropy, which could potentially bias the causal inference between hypothyroidism and ischemic stroke. More extensive research is necessary to confirm the validity of our findings.
Our study identified a direct link between hypothyroidism and ischemic stroke, which suggests that hypothyroidism is a potential risk factor. Further comprehensive research is needed to elucidate the complex underlying biological mechanisms that connect these two conditions.
The original contributions presented in the study are included in the article further inquiries can be directed to the corresponding author.
YT: Writing – original draft, Writing – review & editing. XS: Methodology, Writing – review & editing. JS: Data curation, Methodology, Writing – review & editing. XL: Methodology, Writing – review & editing. YB: Methodology, Writing – review & editing. LY: Methodology, Writing – review & editing. YL: Methodology, Writing – review & editing.
The author(s) declare financial support was received for the research, authorship, and/or publication of this article. This work was supported by the China Postdoctoral Science Foundation projects (2022MD723718) and (20YY14).
The authors declare that the research was conducted in the absence of any commercial or financial relationships that could be construed as a potential conflict of interest.
All claims expressed in this article are solely those of the authors and do not necessarily represent those of their affiliated organizations, or those of the publisher, the editors and the reviewers. Any product that may be evaluated in this article, or claim that may be made by its manufacturer, is not guaranteed or endorsed by the publisher.
1. GBD 2019 Stroke Collaborators. Global, regional, and national burden of stroke and its risk factors, 1990–2019: a systematic analysis for the Global Burden of Disease Study 2019. Lancet Neurol. (2021) 20:795–820. doi: 10.1016/S1474-4422(21)00252-0
2. Adeoye, O, Nyström, KV, Yavagal, DR, Luciano, J, Nogueira, RG, Zorowitz, RD, et al. Recommendations for the establishment of stroke systems of care: a 2019 update. Stroke. (2019) 50:e187–210. doi: 10.1161/STR.0000000000000173
3. Krishnamurthi, RV, Ikeda, T, and Feigin, VL. Global, regional and country-specific burden of ischaemic stroke, intracerebral haemorrhage and subarachnoid haemorrhage: a systematic analysis of the Global Burden of Disease Study 2017. Neuroepidemiology. (2020) 54:171–9. doi: 10.1159/000506396
4. Tsivgoulis, G, Katsanos, AH, Ornello, R, and Sacco, S. Ischemic stroke epidemiology during the COVID-19 pandemic: navigating uncharted waters with changing tides. Stroke. (2020) 51:1924–6. doi: 10.1161/STROKEAHA.120.030791
5. Gkantzios, A, Karapepera, V, Tsiptsios, D, Liaptsi, E, Christidi, F, Gkartzonika, E, et al. Investigating the predictive value of thyroid hormone levels for stroke prognosis. Neurol Int. (2023) 15:926–53. doi: 10.3390/neurolint15030060
6. Murolo, M, Di Vincenzo, O, Cicatiello, AG, Scalfi, L, and Dentice, M. Cardiovascular and neuronal consequences of thyroid hormones alterations in the ischemic stroke. Metabolites. (2022) 13:22. doi: 10.3390/metabo13010022
7. Talhada, D, Santos, CRA, Gonçalves, I, and Ruscher, K. Thyroid hormones in the brain and their impact in recovery mechanisms after stroke. Front Neurol. (2019) 10:1103. doi: 10.3389/fneur.2019.01103
8. Liu, F, Feng, J, Hao, M, Wang, X, Pan, N, Zhang, G, et al. Thyroid stimulating hormone correlates with triglyceride levels but is not associated with the severity of acute ischemic stroke in patients with euthyroidism: a cross-sectional study. Ann Transl Med. (2023) 11:67. doi: 10.21037/atm-22-6374
9. Boehm, FJ, and Zhou, X. Statistical methods for Mendelian randomization in genome-wide association studies: a review. Comput Struct Biotechnol J. (2022) 20:2338–51. doi: 10.1016/j.csbj.2022.05.015
10. Yang, J, Ferreira, T, Morris, AP, Medland, SE, Madden, PA, Heath, AC, et al. Conditional and joint multiple-SNP analysis of GWAS summary statistics identifies additional variants influencing complex traits. Nat Genet. (2012) 44:369–75. doi: 10.1038/ng.2213
11. Bowden, J, Davey Smith, G, Haycock, PC, and Burgess, S. Consistent estimation in Mendelian randomization with some invalid instruments using a weighted median estimator. Genet Epidemiol. (2016) 40:304–14. doi: 10.1002/gepi.21965
12. Bowden, J, Del Greco, MF, Minelli, C, Davey Smith, G, Sheehan, N, and Thompson, J. A framework for the investigation of pleiotropy in two-sample summary data Mendelian randomization. Stat Med. (2017) 36:1783–802. doi: 10.1002/sim.7221
13. Verbanck, M, Chen, CY, Neale, B, and Do, R. Detection of widespread horizontal pleiotropy in causal relationships inferred from Mendelian randomization between complex traits and diseases. Nat Genet. (2018) 50:693–8. doi: 10.1038/s41588-018-0099-7
14. Burgess, S, and Thompson, SG. Interpreting findings from Mendelian randomization using the MR-Egger method. Eur J Epidemiol. (2017) 32:377–89. doi: 10.1007/s10654-017-0255-x
15. Bo, L, Wang, L, and Jiao, L. Feature scaling for kernel fisher discriminant analysis using leave-one-out cross validation. Neural Comput. (2006) 18:961–78. doi: 10.1162/089976606775774642
16. Tutwiler, V, Singh, J, Litvinov, RI, Bassani, JL, Purohit, PK, and Weisel, JW. Rupture of blood clots: mechanics and pathophysiology. Sci Adv. (2020) 6:eabc0496. doi: 10.1126/sciadv
18. Chaker, L, Bianco, AC, Jonklaas, J, and Peeters, RP. Hypothyroidism. Lancet. (2017) 390:1550–62. doi: 10.1016/S0140-6736(17)30703-1
19. Teixeira, PFDS, Dos Santos, PB, and Pazos-Moura, CC. The role of thyroid hormone in metabolism and metabolic syndrome. Ther Adv Endocrinol Metab. (2020) 11:2042018820917869. doi: 10.1177/2042018820917869
20. Qin, X, Li, J, Wu, T, Wu, Y, Tang, X, Gao, P, et al. Overall and sex-specific associations between methylation of the ABCG1 and APOE genes and ischemic stroke or other atherosclerosis-related traits in a sibling study of Chinese population. Clin Epigenetics. (2019) 11:189. doi: 10.1186/s13148-019-0784-0
21. Alsamghan, AS, Alsaleem, SA, Alzahrani, MAS, Patel, A, Mallick, AK, and Sheweita, SA. Effect of hypovitaminosis D on lipid profile in hypothyroid patients in Saudi Arabia. Oxid Med Cell Longev. (2020) 2020:6640402–8. doi: 10.1155/2020/6640402
22. Iwen, KA, Oelkrug, R, Kalscheuer, H, and Brabant, G. Metabolic syndrome in thyroid disease. Front Horm Res. (2018) 49:48–66. doi: 10.1159/000485996
23. Ali, A, Bain, S, Hicks, D, Newland Jones, P, Patel, DC, Evans, M, et al. SGLT2 inhibitors: cardiovascular benefits beyond HbA1c-translating evidence into practice. Diabetes Ther. (2019) 10:1595–622. doi: 10.1007/s13300-019-0657-8
24. Lamba, N, Liu, C, Zaidi, H, Broekman, MLD, Simjian, T, Shi, C, et al. A prognostic role for low tri-iodothyronine syndrome in acute stroke patients: a systematic review and meta-analysis. Clin Neurol Neurosurg. (2018) 169:55–63. doi: 10.1016/j.clineuro.2018.03.025
25. Li, LQ, Xu, XY, Li, WY, Hu, XY, and Lv, W. The prognostic value of total T3 after acute cerebral infarction is age-dependent: a retrospective study on 768 patients. BMC Neurol. (2019) 19:54. doi: 10.1186/s12883-019-1264-z
26. Suda, S, Shimoyama, T, Nagai, K, Arakawa, M, Aoki, J, Kanamaru, T, et al. Low free triiodothyronine predicts 3-month poor outcome after acute stroke. J Stroke Cerebrovasc Dis. (2018) 27:2804–9. doi: 10.1016/j.jstrokecerebrovasdis.2018.06.009
27. Zhang, S, Zhao, X, Xu, S, Yuan, J, Si, Z, Yang, Y, et al. Low free triiodothyronineis predicts worsen neurological outcome of patients with acute ischemic stroke: a retrospective study with bioinformatics analysis. BMC Neurol. (2019) 19:272. doi: 10.1186/s12883-019-1509-x
28. Guo, J, Wang, J, Xia, Y, Jiang, S, Xu, P, Tao, C, et al. Thyroid function affects the risk of post-stroke depression in patients with acute lacunar stroke. Front Neurol. (2022) 13:792843. doi: 10.3389/fneur.2022.792843
29. Bauer, BS, Azcoaga-Lorenzo, A, Agrawal, U, Fagbamigbe, AF, and McCowan, C. The impact of the management strategies for patients with subclinical hypothyroidism on long-term clinical outcomes: an umbrella review. PLoS One. (2022) 17:e0268070. doi: 10.1371/journal.pone.0268070
30. Møllehave, LT, Skaaby, T, Linneberg, A, Knudsen, N, Jørgensen, T, and Thuesen, BH. The association of thyroid stimulation hormone levels with incident ischemic heart disease, incident stroke, and all-cause mortality. Endocrine. (2020) 68:358–67. doi: 10.1007/s12020-020-02216-5
31. De Luca, R, Davis, PJ, Lin, HY, Gionfra, F, Percario, ZA, Affabris, E, et al. Thyroid hormones interaction with immune response, inflammation and non-thyroidal illness syndrome. Front Cell Dev Biol. (2021) 8:614030. doi: 10.3389/fcell.2020.614030
32. van der Spek, AH, Fliers, E, and Boelen, A. Thyroid hormone and deiodination in innate immune cells. Endocrinology. (2021) 162:bqaa200. doi: 10.1210/endocr/bqaa200
33. Li, J, Abe, K, Milanesi, A, Liu, YY, and Brent, GA. Thyroid hormone protects primary cortical neurons exposed to hypoxia by reducing DNA methylation and apoptosis. Endocrinology. (2019) 160:2243–56. doi: 10.1210/en.2019-00125
34. Abe, K, Li, J, Liu, YY, and Brent, GA. Thyroid hormone-mediated histone modification protects cortical neurons from the toxic effects of hypoxic injury. J Endocr Soc. (2022) 6:bvac139. doi: 10.1210/jendso/bvac139
35. Kim, J, Lee, H, Yi, SJ, and Kim, K. Gene regulation by histone-modifying enzymes under hypoxic conditions: a focus on histone methylation and acetylation. Exp Mol Med. (2022) 54:878–89. doi: 10.1038/s12276-022-00812-1
36. Talhada, D, Feiteiro, J, Costa, AR, Talhada, T, Cairrão, E, Wieloch, T, et al. Triiodothyronine modulates neuronal plasticity mechanisms to enhance functional outcome after stroke. Acta Neuropathol Commun. (2019) 7:216. doi: 10.1186/s40478-019-0866-4
37. Huang, S, Liu, L, Tang, X, Xie, S, Li, X, Kang, X, et al. Research progress on the role of hormones in ischemic stroke. Front Immunol. (2022) 13:1062977. doi: 10.3389/fimmu.2022.1062977
38. Papaleontiou, M, Levine, DA, Reyes-Gastelum, D, Hawley, ST, Banerjee, M, and Haymart, MR. Thyroid hormone therapy and incident stroke. J Clin Endocrinol Metab. (2021) 106:e3890–900. doi: 10.1210/clinem/dgab444
39. Fani, L, Roa Dueñas, O, Bos, D, Vernooij, MW, Klaver, CCW, Ikram, MK, et al. Thyroid status and brain circulation: the Rotterdam study. J Clin Endocrinol Metab. (2022) 107:e1293–302. doi: 10.1210/clinem/dgab744
Keywords: hypothyroidism, stroke, ischemic stroke, cerebral infarction, Mendelian randomization analysis
Citation: Tian Y, Shi XQ, Shui JW, Liu XY, Bu Y, Liu Y and Yin LP (2024) Exploring the causal factor effects of hypothyroidism on ischemic stroke: a two-sample Mendelian randomization study. Front. Neurol. 15:1322472. doi: 10.3389/fneur.2024.1322472
Received: 16 October 2023; Accepted: 18 January 2024;
Published: 31 January 2024.
Edited by:
Raffaele Ornello, University of L’Aquila, ItalyReviewed by:
Christian Urbanek, Klinikum Ludwigshafen, GermanyCopyright © 2024 Tian, Shi, Shui, Liu, Bu, Liu and Yin. This is an open-access article distributed under the terms of the Creative Commons Attribution License (CC BY). The use, distribution or reproduction in other forums is permitted, provided the original author(s) and the copyright owner(s) are credited and that the original publication in this journal is cited, in accordance with accepted academic practice. No use, distribution or reproduction is permitted which does not comply with these terms.
*Correspondence: Li Ping Yin, MTMyMDgyNTMzMzhAMTYzLmNvbQ==
Disclaimer: All claims expressed in this article are solely those of the authors and do not necessarily represent those of their affiliated organizations, or those of the publisher, the editors and the reviewers. Any product that may be evaluated in this article or claim that may be made by its manufacturer is not guaranteed or endorsed by the publisher.
Research integrity at Frontiers
Learn more about the work of our research integrity team to safeguard the quality of each article we publish.