- 1Department of Clinical Neuroscience, Karolinska Institute, Stockholm, Sweden
- 2MSBase Foundation, Melbourne, VIC, Australia
- 3The Danish Multiple Sclerosis Registry, Copenhagen University Hospital, Rigshospitalet, Glostrup, Denmark
- 4Danish Multiple Sclerosis Center, Department of Neurology, Copenhagen University Hospital Rigshospitalet, Glostrup, Copenhagen, Denmark
- 5MS and Neuroimmunology Research, Central Clinical School, Alfred and Box Hill Hospitals, Monash University, Melbourne, VIC, Australia
- 6Department of Neuroscience, Central Clinical School, Monash University, Melbourne, VIC, Australia
- 7Service de Neurologie, Sclérose en Plaques, Pathologies de la Myéline et Neuro-Inflammation, Hôpital Neurologique Pierre Wertheimer, Hospices Civils de Lyon, Lyon, France
- 8Centre des Neurosciences de Lyon, L'Institut national de la santé et de la recherche médicale 1028 et Centre national de la recherche scientifique joint research units5292, Lyon, France
- 9Faculté de Médicine Lyon-Est, Université Claude Bernard Lyon 1, Villeurbanne, Auvergne-Rhône-Alpes, France
- 10Department of Basic Medical Sciences, Neurosciences and Sense Organs, University of Bari Aldo Moro, Bari, Italy
- 11Department of Translational Biomedicine and Neuroscience, DiBraiN, University of Bari Aldo Moro, Bari, Italy
- 12Department of Neurology and Center of Clinical Neuroscience, First Faculty of Medicine, Charles University and General University Hospital, Prague, Czechia
- 13Biogen International GmbH, Zug, Switzerland
- 14Biogen Digital Health, Biogen Spain, Madrid, Spain
- 15University of Montreal Hospital Research Centre and Universite de Montreal, Montreal, QC, Canada
- 16University Newcastle, Callaghan, NSW, Australia
- 17Hunter Medical Research Institute, Hunter New England Health, John Hunter Hospital, New Lambton Heights, NSW, Australia
- 18Department of Neurology, Neuroscience Research Center, Golestan University of Medical Sciences, Gogan, Iran
- 19Faculty of Medicine, Division of Neurology, Geneva University Hospital, Geneva, Switzerland
- 20Isfahan University of Medical Sciences, Isfahan, Iran
- 21Dokuz Eylul University, Konak/Izmir, Türkiye
- 22Department of Neurology, Hospital Universitario Virgen Macarena, Sevilla, Spain
- 23Division of Neurology, Department of Medicine, Amiri Hospital, Sharq, Kuwait
- 24Medical Faculty, 19 Mayis University, Samsun, Türkiye
- 25Clinical Outcomes Research Unit, Department of Medicine, University of Melbourne, Melbourne, VIC, Australia
- 26Neuro Rive-Sud, Longueuil, QC, Canada
- 27Nehme and Therese Tohme Multiple Sclerosis Center, American University of Beirut Medical Center, Beirut, Lebanon
- 28Department of Neurology, Faculty of Health Sciences, University Fernando Pessoa, Porto, Portugal
- 29Academic MS Center Zuyderland, Department of Neurology, Zuyderland Medical Center, Sittard-Geleen, Netherlands
- 30Center of Neuroimmunology, Service of Neurology, Hospital Clinic de Barcelona, Barcelona, Spain
- 31Hacettepe University, Ankara, Türkiye
- 32Department of Neurology, Hospital Clinico San Carlos, Madrid, Spain
- 33Department of Neurology, School of Medicine and Koc University Research Center for Translational Medicine (KUTTAM), Koc University, Istanbul, Türkiye
- 34Royal Victoria Hospital, Belfast, United Kingdom
- 35University of Queensland, Brisbane, QLD, Australia
- 36Nemocnice Jihlava, Jihlava, Czechia
- 37Groene Hart Ziekenhuis, Gouda, Netherlands
- 38St Vincent's University Hospital, Dublin, Ireland
- 39Bakirkoy Education and Research Hospital for Psychiatric and Neurological Diseases, Istanbul, Türkiye
- 40CSSS Saint-Jérôme, Saint-Jerome, QC, Canada
- 41Monash Health, Melbourne, VIC, Australia
- 42Rashid Hospital, Dubai, United Arab Emirates
- 43Department of Neurology, Aarhus University Hospital, Aarhus, Denmark
- 44Department of Neurology, Southwest Jutland Hospital, University Hospital of Southern Denmark, Esbjerg, Denmark
- 45Department of Neurology, Nordsjællands Hospital, Hillerød, Denmark
- 46Department of Neurology, Hospital of Southern Jutland, University of Southern Denmark, Aabenraa, Denmark
- 47Department of Neurology, Physiotherapy and Occupational Therapy, Gødstrup Hospital, Herning, Denmark
- 48NIDO | Centre for Research and Education, Gødstrup Hospital, Herning, Denmark
- 49Department of Neurology, Copenhagen University Hospital Herlev and Gentofte, København, Denmark
Background: Treatment switching is a common challenge and opportunity in real-world clinical practice. Increasing diversity in disease-modifying treatments (DMTs) has generated interest in the identification of reliable and robust predictors of treatment switching across different countries, DMTs, and time periods.
Objective: The objective of this retrospective, observational study was to identify independent predictors of treatment switching in a population of relapsing-remitting MS (RRMS) patients in the Big Multiple Sclerosis Data Network of national clinical registries, including the Italian MS registry, the OFSEP of France, the Danish MS registry, the Swedish national MS registry, and the international MSBase Registry.
Methods: In this cohort study, we merged information on 269,822 treatment episodes in 110,326 patients from 1997 to 2018 from five clinical registries. Patients were included in the final pooled analysis set if they had initiated at least one DMT during the relapsing-remitting MS (RRMS) stage. Patients not diagnosed with RRMS or RRMS patients not initiating DMT therapy during the RRMS phase were excluded from the analysis. The primary study outcome was treatment switching. A multilevel mixed-effects shared frailty time-to-event model was used to identify independent predictors of treatment switching. The contributing MS registry was included in the pooled analysis as a random effect.
Results: Every one-point increase in the Expanded Disability Status Scale (EDSS) score at treatment start was associated with 1.08 times the rate of subsequent switching, adjusting for age, sex, and calendar year (adjusted hazard ratio [aHR] 1.08; 95% CI 1.07–1.08). Women were associated with 1.11 times the rate of switching relative to men (95% CI 1.08–1.14), whilst older age was also associated with an increased rate of treatment switching. DMTs started between 2007 and 2012 were associated with 2.48 times the rate of switching relative to DMTs that began between 1996 and 2006 (aHR 2.48; 95% CI 2.48–2.56). DMTs started from 2013 onwards were more likely to switch relative to the earlier treatment epoch (aHR 8.09; 95% CI 7.79–8.41; reference = 1996–2006).
Conclusion: Switching between DMTs is associated with female sex, age, and disability at baseline and has increased in frequency considerably in recent years as more treatment options have become available. Consideration of a patient's individual risk and tolerance profile needs to be taken into account when selecting the most appropriate switch therapy from an expanding array of treatment choices.
Introduction
Multiple sclerosis (MS) is a chronic, autoimmune, and demyelinating neurological disorder. Accurate diagnosis, disease-modifying therapies, and symptom management are key components of managing MS to improve patients' quality of life and limit disability progression (1, 2). Treatment discontinuation and switching in multiple sclerosis (MS) is a common challenge and opportunity in real-world clinical practice. The increasingly diverse range of disease-modifying treatments (DMTs) currently available to patients and clinicians, coupled with frequent changes in reimbursement and treatment recommendations, has generated interest in the identification of reliable and robust predictors of treatment switching across different countries, DMTs, and epoch changes (3–9). Whilst effectiveness and tolerance remain key drivers of treatment failure, the precise role of demographic and clinical factors remains less clear (10, 11).
This study group previously reported that both rates and reasons for treatment interruption, encompassing both treatment switching and discontinuation, remained largely stable over a 20-year observation period, suggesting that treatment switching was primarily driven by the properties of DMTs themselves and less related to either risk management or market competition (12).
The identification of independent predictors of treatment switching and response in long-term, heterogeneous disease is likely to form a key component in the development of personalized prediction and healthcare in multiple sclerosis. The accuracy and robustness of personalized prediction in RRMS is a key challenge in improving generalisability, often requiring large and representative samples (13–15). A recent systematic review further reported a lack of validated predictive tools for the early and reliable identification of key drivers of treatment failure, such as disease progression (16).
The study aimed to identify independent predictors of treatment switching in the Big MS Data Network in a population of relapsing-remitting MS (RRMS) patients initiating DMT at least once over the course of their disease (12, 17).
Materials and methods
Study design and data
This study was a retrospective analysis of observational, real-world data sourced from five clinical MS registries included in the BMSD network project: the Italian MS registry, the OFSEP of France, the Danish MS registry, the Swedish national MS registry, and the international MSBase Registry. These registries have previously been described in detail (12, 18–22). Treatment episodes and associated patient data complying with minimum dataset requirements were individually extracted from the five contributing datasets and then pooled into a single combined dataset.
Data harmonization and quality
Data quality checks were conducted before merging to minimize outcome assessment and follow-up bias in the cohorts under study. This included ensuring all variables required for the minimum analysis dataset had been extracted and transferred in the correct format, consistent across all five registries. The data were checked for duplicates and date inconsistencies covering key demographics, disease characteristics, and treatment dates. Data counts were then performed to assess the completeness of crucial variables. The harmonization and quality assurance processes have been previously described (12, 17).
Inclusions and exclusions
Patients were included in the final pooled analysis set if they had initiated at least one DMT during the relapsing-remitting MS (RRMS) stage. Patients not diagnosed with RRMS or RRMS patients not initiating DMT therapy during the RRMS phase were excluded from the analysis.
Poser or McDonald criteria were used to confirm MS diagnosis, depending on the time the diagnosis was made in each registry. Subjects were defined as exposed to a DMT if they had received at least one injection/infusion (or at least a one-time consumption of an oral drug). The pre-study period preceding the index date, during which patients were required to have continuous medical service coverage, was defined as the time since the first recorded visit in each registry up until the baseline date. This pre-study period ensured a standard run-in period prior to DMT exposure and a standard period during which the diagnosis of MS was identified.
Variables and definitions
DMT starts recorded between 1996 and 2018, inclusive, were included in the analysis. The primary outcome variable in this analysis was treatment switching. Treatment switching was defined as the initiation of a new DMT within 6 months of ceasing a preceding DMT, consistent with previous studies using data from these registries (3, 23). Demographic and clinical characteristics at treatment start analyzed for association with subsequent treatment switching included age, sex, disease duration, time since diagnosis, EDSS, and calendar year of treatment start. The calendar year was further categorized into three treatment epochs, capturing the platform interferon-β (IFNβ) and glatiramer acetate (GLA) only era (1996–2006), the introduction of natalizumab (NTZ) infusion (2007–2012), and the era of oral treatments (including fingolimod [FTY], dimethyl fumarate [DMF], and teriflunomide [TERI]) (2013–2018).
Ethics
The studies involving human participants were reviewed and approved by each contributing registry to the Big MS Data Network according to their own ethics and operating and inclusion rules. Each registry is required to obtain its own approval prior to the provision of the data and pooling.
Statistical analyses
Categorical variables were summarized using frequency and percentage. Continuous variables were summarized using mean and standard deviation (SD) or median and interquartile range (IQR) as appropriate. The statistical unit of the modeling analysis was the treatment episode. Patients were permitted to contribute multiple DMT episodes to the analysis. Associations between potential clinical and demographic factors at treatment start and the switching end-point were analyzed using a multilevel shared frailty survival model (24). This involved using a mixed-effects Cox model where an indicator variable representing each registry providing data to the pooled analysis was used as a random effect. Hazard proportionality was assessed via the analysis of scaled Schoenfeld residuals. All multivariate models were further assessed for collinearity and heteroskedasticity. The analysis was performed both across the entire treatment cohort and then stratified for treatment epoch (1996–2006, 2007–2012, and 2013 onwards) and drug/drug class (IFNβ, GLA, NTZ, FTY, DMF, and TERI) for which the sample size was sufficient, to assess whether the pattern of switching predictors varied by time and/or DMT product. All analyses were conducted on a complete-case basis, with no imputation for missing data. All analyses were performed in Stata version 16.1 (StataCorp, College Station, Texas) and R version 3.6.3 (R Foundation for Statistical Computing, Vienna, Austria).
Results
Patients and treatment episodes
A total pooled sample of 110,326 patients contributing 269,822 DMT treatment episodes from the five registries was included in the analysis (Table 1). A total of 78,629 (70.9%) of these patients were female. The mean (SD) age at disease onset across the pooled sample was 30.9 years (10.3), whilst the mean (SD) age at first treatment initiation was 36.6 years (11.0). Of the 184,013 observed DMT stops, 159,309 (86.6%) switched to an alternate DMT within 6 months. Alternate platform DMTs (IFNβ or GLA), NTZ, or FTY were the most frequently switched to drugs across the observation period.
Associations between baseline factors and treatment switching
Across the entire pooled sample of treatments, every one-point increase in EDSS at the start of treatment was associated with 1.08 times the rate of subsequent switching, adjusting for age, sex, and calendar year (adjusted hazard ratio [aHR] 1.08; 95% CI 1.07–1.08) (Table 2). Female sex was associated with 1.11 times the rate of switching relative to male sex (HR 1.11; 95% CI 1.08–1.14), whilst older age at baseline was also associated with an increased rate of treatment switching. DMTs started between 2007 and 2012 were associated with 2.48 times the odds of treatment switching relative to DMTs started between 1996 and 2006 (aHR 2.48; 95% CI 2.48–2.56), controlling for age, sex, and baseline EDSS. DMTs commenced from 2013 onwards were even more likely to switch relative to the earlier treatment epoch (aHR 8.09; 95% CI 7.79–8.41; reference = 1996–2006).
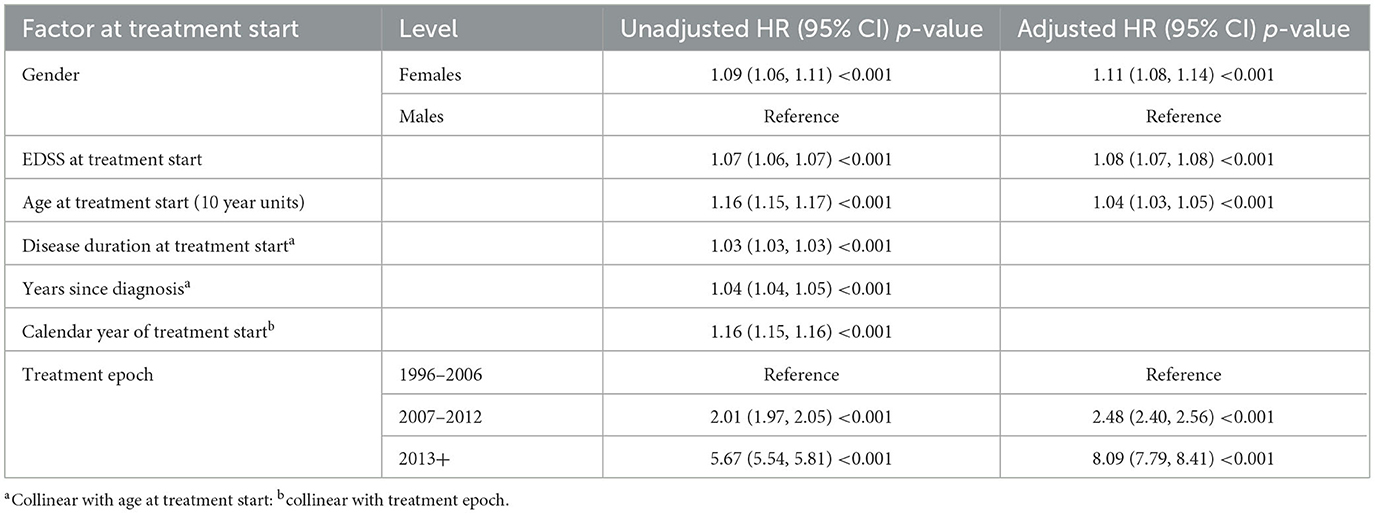
Table 2. Associations between baseline factors and treatment switching (shared frailty survival model).
Switching by epoch
Similar patterns of association were observed when the multilevel modeling was stratified by treatment epoch. When the analysis was limited to the earlier platform DMTs epoch (1996–2006), EDSS at treatment initiation demonstrated a larger influence on subsequent switching relative to the full observation period; every unit of EDSS higher at treatment start was associated with 1.14 times the rate of treatment switching (aHR 1.14; 95% CI 1.13–1.16) (Table 3). Women were associated with 1.13 times the rate of switching compared to men (aHR 1.13; 95% CI 1.07–1.20), whilst DMTs started in later calendar years within the 1996–2006 epoch were associated with greater odds of treatment switching (calendar year aHR 1.17; 95% CI 1.16, 1.18).
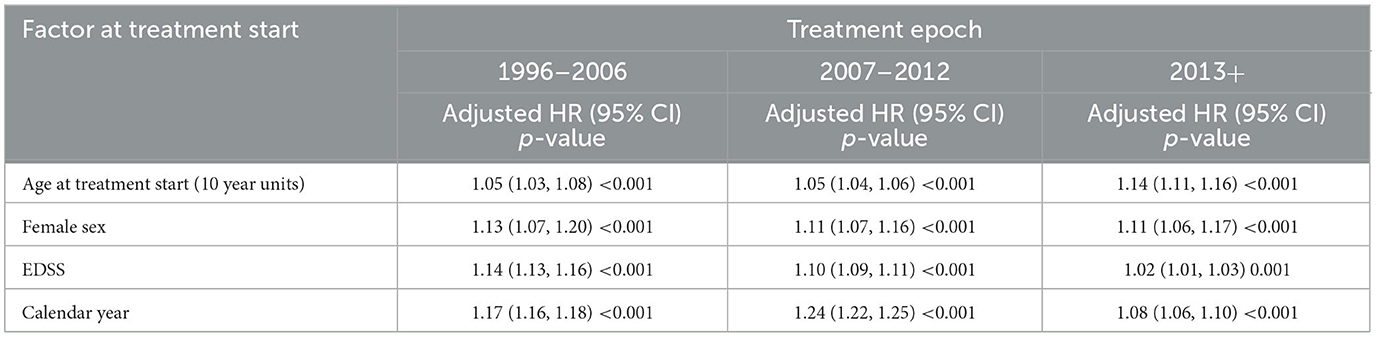
Table 3. Associations between baseline factors and treatment switching–stratified by treatment epoch.
Associations were again similar when the analysis was confined to the 2007–2013 epoch (Table 4). Higher EDSS at treatment start (aHR 1.10; 95% CI 1.09–1.11), female sex (aHR 1.11; 95% CI 1.07–1.16), older age at treatment start (aHR 1.01; 95% CI 1.00–1.01), and later calendar years (aHR 1.24; 95% CI 1.22–1.25) were all associated with a significantly increased rate of treatment switching. When the modeling was limited to the most recent treatment epoch (2013 onwards), baseline EDSS was associated with a significant, albeit considerably smaller, increase in switching rate (relative to the 1996–2006 and 2007–2012 treatment epochs), with every one-point increase in EDSS being associated with 1.02 times the rate of switching on adjusted modeling (aHR 1.02; 95% CI 1.01–1.03). Older age at treatment start, female sex, and later calendar years were all associated with significantly increased switching rates within the 2013+ epoch.
Switching by DMT
Across the entire 1996–2018 observation period, higher EDSS at treatment start (aHR 1.09; 95% CI 1.08–1.11), female sex (aHR 1.17; 95% CI 1.11–1.24), older age (aHR 1.01; 95% CI 1.00–1.01), and calendar year (aHR 1.15; 95% CI 1.14, 1.15) were all associated with increased rates of switching from IFNβ (Table 4). A similar pattern of association was observed when the analysis was limited to GLA, although female sex was no longer associated with an increased rate of switching. Calendar year was again a major driver of switching from NTZ (aHR 1.35; 95% CI 1.33–1.37) and FTY (aHR 1.06; 95% CI 1.04–1.08), although no such association was observed with either DMF or TERI. Older age at baseline was associated with an increased rate of switching from both DMF and TERI. Women treated with TERI were also associated with 1.26 times the rate of switching compared to men treated with TERI (aHR 1.26; 95% CI 1.04–1.51).
Discussion
As previously reported by this study group, treatment switching in RRMS is common. In this new analysis of the same cohort, we observed that older age, female sex, and higher EDSS at the time of index treatment initiation were consistently associated with a significantly higher switching rate. This is consistent with previous observations from registry studies, which have also reported sex and EDSS as independent predictors of treatment interruption in both RRMS and CIS (25). Kalincik et al.'s (26) multivariate predictive algorithm modeling of individual treatment response and persistence using a large number of demographic and clinical factors identified older age as an independent predictor of on-treatment relapse triggering discontinuation. Similarly, Ayrignac et al. (27) reported that patients with a baseline EDSS of 2 or more were strongly associated with treatment failure. A recent Danish study of 3,297 MS patients reported age and sex as key determinants of both the initial first-line DMT choice and subsequent escalation product (28). The most prominent effect, in terms of adjusted hazard ratio size, observed across the whole cohort was the treatment epoch, with later epochs (2007–2012 and 2013+) associated with progressively larger rates of treatment switching, relative to the earlier 1996–2006 epoch where lower-to-moderate efficacious platform DMTs dominated MS treatment and diagnostic delay was more common (29). This progressively larger rate of treatment switching with time likely reflects the relatively broader range of both DMT products available with varying effectiveness and safety profiles and an increase in the diversity of treatment strategies practiced during more recent years (30–32). It may also, in part, reflect a shift in treatment strategy toward the earlier introduction of high-efficacy treatments in response to treatment failure (3, 33).
Whilst the strongest predictors of treatment switching, such as age, sex, and baseline EDSS, were largely consistent across treatment epochs, there were some key differences in the patterns of predictors when the analysis was stratified by DMT. Higher baseline EDSS was a strong correlate of subsequent treatment switching in the older platform drugs (IFNβ and GLA), potentially secondary to longer disease duration whilst awaiting newer switch products to become available, but less so for NTZ (HR 1.01; 95% CI 1.00, 1.03). By further contrast, no association between EDSS and treatment switching was observed for more recent oral and/or higher efficacy preparations, including DMF, TERI, and FTY. This may in part be a function of the original platform DMDs being used more frequently across a broader range of EDSS scores compared with oral first-line therapies such as DMF or TERI. A sex effect was observed for IFNβ (female sex HR 1.17; 95% CI 1.11, 1.24) and TERI (HR 1.26; 95% CI 1.04, 1.51), but not for any of the other DMTs studied. The latter observation may be partially explained by the early TERI discontinuation associated with pregnancy planning. With the exception of TERI, treatment switching became progressively more common across most years in the later years of the observation period.
Consistent with the observation that the observed effect of EDSS at the time of treatment start on switching rates was maximal under the older platform DMTs was the additional observation that this baseline EDSS effect was largest during both the earlier 1996–2006 (HR 1.14; 95% CI 1.13, 1.16) and 2007–2012 periods (HR 1.10; 95% CI 1.09, 1.11). Whilst significant, the EDSS effect in the 2013 onwards period was comparatively much smaller (HR 1.02; 95% CI 1.01, 1.03). This may in part be due to the increasingly greater role of MRI and relapse activity in guiding treatment decisions (34, 35).
Our observation that treatment switching has become more common over time likely reflects, at least in part, a desire by clinicians and patients to optimize disease management, particularly in terms of delaying or preventing disability progression and maintaining neurological function (31, 36, 37). Identifying independent and reliable demographic and clinical predictors also has real-world implications for personalized medicine. A recent French cohort study used similar predictive factors to develop a dynamic scoring system to improve the timing of treatment switch decisions through the earlier identification of non-responders (38). Whilst the results of our study are largely confirmatory, the power conferred by the very large sample provides important validation and is a fundamental tool for individual or personalized risk prediction. As previously explored in our published descriptive study on the same pooled cohort (12), the wider availability, increased choice of DMTs and patient preferences also permit important lifestyle considerations to factor into treatment selection, including pregnancy planning, the management of side effects, work commitments, and travel (39, 40). A key limitation of this study was the lack of sufficient MRI data to include in the predictor analysis. Whilst the effects observed in the regression modeling are independent of confounding from the clinical and demographic included also in the multivariate models (i.e., age, sex, disease duration, time since diagnosis, baseline EDSS, and treatment calendar year/epoch), they do not control for other potential sources of confounding or heterogeneity between registries, including MRI lesion metrics.
Data availability statement
The data analyzed in this study is subject to the following licenses/restrictions: Data availability is subject to the rules and governance of each participating registry. Requests to access these datasets should be directed to TSp, tim.spelman@ki.se.
Author contributions
TSp: Conceptualization, Data curation, Formal analysis, Investigation, Methodology, Resources, Software, Supervision, Validation, Writing—original draft, Writing—review & editing. MM: Conceptualization, Writing—review & editing. HB: Conceptualization, Writing—review & editing. AV: Writing—review & editing. SV: Conceptualization, Writing—review & editing. MTr: Conceptualization, Writing—review & editing. PI: Writing—review & editing. DH: Conceptualization, Writing—review & editing. JD: Conceptualization, Writing—review & editing. FP: Writing—review & editing. RH: Conceptualization, Writing—review & editing. PD: Writing—review & editing. JL-S: Writing—review & editing. SS: Writing—review & editing. PL: Writing—review & editing. VS: Writing—review & editing. SO: Writing—review & editing. SE: Writing—review & editing. RAl: Writing—review & editing. MTe: Writing—review & editing. MG: Writing—review & editing. TK: Writing—review & editing. FG'M: Writing—review & editing. OS: Writing—review & editing. SK: Writing—review & editing. BY: Writing—review & editing. MS: Writing—review & editing. OG: Writing—review & editing. YB: Writing—review & editing. RK: Writing—review & editing. CO-G: Writing—review & editing. AA: Writing—review & editing. SH: Writing—review & editing. PM: Writing—review & editing. RAm: Writing—review & editing. KdG: Writing—review & editing. CM: Writing—review & editing. AS: Writing—review & editing. JP: Writing—review & editing. NJ: Writing—review & editing. JI: Writing—review & editing. LS: Writing—review & editing. AM: Writing—review & editing. LF: Writing—review & editing. FS: Writing—review & editing. AG: Funding acquisition, Project administration, Writing—review & editing. LP: Writing—review & editing. HJ: Writing—review & editing. PR: Writing—review & editing. TSe: Writing—review & editing. MP: Writing—review & editing. JC: Writing—review & editing. MK: Writing—review & editing. MS: Writing—review & editing. HM: Writing—review & editing. JH: Conceptualization, Funding acquisition, Project administration, Supervision, Writing—review & editing.
Funding
The authors declare financial support was received for the research, authorship, and/or publication of this article. This study was supported by an unrestricted grant from Biogen.
Acknowledgments
This article is dedicated to our valued colleague, Dr. Yara Fragoso, who sadly passed away during the development of this manuscript. We also acknowledge the following contributors and members of the MSBase Study Group: Eva Havrdova, Alexandre Prat, Cavit Boz, Pierre Grammond, Katherine Buzzard, Cees Zwanikken, Nevin Shalaby, Vincent Van Pesch, Recai Turkoglu, Jens Kuhle, Michael Barnett, Suzanne Hodgkinson, Thor Petersen, Mark Slee, Bart Van Wijmeersch, Edgardo Cristiano, Maria Edite Rio, Ricardo Fernandez Bolaños, L G F Sinnige, Saloua Mrabet, Riadh Gouider, Farouk Talaat, Eduardo Aguera-Morales, Maria Laura Saladino, Orla Gray, Marcela Fiol, Bruce Taylor, Richard Macdonell, Norma Deri, Jose Luis Sanchez-Menoyo, Jamie Campbell, Tamara Castillo-Triviño, Neil Shuey, Bhim Singhal, Ilya Kister, Tunde Csepany, Guy Laureys, Talal Al-Harbi, Cameron Shaw, Dieter Poehlau, Dheeraj Khurana, Shlomo Flechter, Walter Oleschko Arruda, Marija Cauchi, Norbert Vella, Marzena Fabis-Pedrini, Allan Kermode, Angel Perez Sempere, Claudio Gobbi, Jose Antonio Cabrera-Gomez, Ismail Ramadan, Leontien Den braber-Moerland, Fraser Moore, PM, Aldo Savino, Eli Skromne, Alejandro Caride, Alfredo Firstenfeld, Alberto Rodriguez Alfici, Elizabeth Alejandra Bacile Bacile, Vetere Santiago, Carlos Vrech, Marcos Burgos, Karyn Boundy, John Parratt, Mike Boggild, Ik Lin Tan, Todd Hardy, Steve Vucic, Danny Decoo, Stephane Charest, Karsten Beer, Karim Kotkata, Shereen Fathi, Jose Andres Dominguez, Jose C. Alvarez-Cermeno, Etienne Roullet, Carolyn Young, Magda Tsolaki, Nikolaos Grigoriadis, Alejandro Jose Diaz Jimenez, Imre Piroska, Csilla Rozsa, Magdolna Simo, Krisztina Kovacs, Tunde Erdelyi, Gabor Rum, Attila Sas, Eniko Dobos, Anita Trauninger, Istvan Deme, Cecilia Rajda, Joab Chapman, Dimitrios Karussis, Vladimir Bojkovski, Tatjana Petkovska-Boskova, Norbert Vella, Irene Treviño-Frenk, Joyce Pauline Joseph, Jyh Yung Hor, Pei Chiek The, Yakub Nyandaiti, Jabir Alkhaboori, Ricardo Ginestal, Carmen-Adella Sirbu, Ulku Turk Boru, Kai Chen Wang, Benjamin Greenberg, and Donald McCarren.
Conflict of interest
TSp received compensation for serving on scientific advisory boards, honoraria for consultancy and funding for travel from Biogen; and speaker honoraria from Novartis. MM has served on the scientific advisory board for Sanofi, Novartis, and Merck and has received honoraria for lecturing from Biogen, Merck, Novartis, Roche, Genzyme, and Bristol Myers Squibb. HB is an employee of Monash University and has accepted travel compensation from Merck; his institution receives honoraria for talks, steering committee activities, and research grants from Roche, Merck, Biogen, Novartis, UCB Pharma, Medical Research Future Fund Australia, NHMRC Australia, Trish MS Foundation, MS Australia, and the Pennycook Foundation. He receives personal compensation for steering group activities for the Brain Health Initiative from the Oxford Health Policy Forum and is funded by an NHMRC Australia Investigator Grant. SV received consulting and lecturing fees, travel grants, and research support from Biogen, Celgene, Genentech, Genzyme, Medday Pharmaceuticals, Merck Serono, Novartis, Roche, Sanofi Aventis, and Teva Pharma. MT has served on scientific advisory boards for Biogen, Novartis, Roche, and Genzyme; has received speaker honoraria and travel support from Biogen Idec, Sanofi Aventis, Merck Serono, Teva, Genzyme, and Novartis; and has received research grants for her institution from Biogen Idec, Merck Serono, and Novartis. PI has served on scientific advisory boards for Biogen Idec, Bayer, Teva, Roche, Merck Serono, Novartis, and Genzyme and has received funding for travel and/or speaker honoraria from Sanofi Aventis, Genzyme, Biogen Idec, Teva, Merck Serono, and Novartis. DH was supported by the Charles University Cooperation Program in Neuroscience, the project National Institute for Neurological Research (Programme EXCELES, ID Project No. LX22NPO5107) funded by the European Union (Next Generation EU), and by the General University Hospital in Prague project MH CZ-DRO-VFN64165. She also received compensation for travel, speaker honoraria, and consultant fees from Biogen Idec, Novartis, Merck, Bayer, Sanofi Genzyme, Roche, and Teva, as well as support for research activities from Biogen Idec. FP is an employee of Biogen. RH is an employee of Biogen and holds stock. PD served on editorial boards and has been supported to attend meetings by EMD, Biogen, Novartis, Genzyme, and TEVA Neuroscience. He holds grants from the CIHR and the MS Society of Canada and has received funding for investigator-initiated trials from Biogen, Novartis, and Genzyme. JL-S received travel compensation from Novartis, Biogen, Roche, and Merck. Her institution receives honoraria for talks and advisory board commitments, as well as research grants from Biogen, Merck, Roche, TEVA, and Novartis. SS declared no competing interests. PL received honoraria for speaking and/or travel expenses from Biogen, Merck, Novartis, Roche; consulting fees from Biogen, GeNeuro, Merck, Novartis, Roche; and research support from Biogen, Merck, Novartis. None were related to this work. SE received speaker honoraria and consultant fees from Biogen Idec, Novartis, Merck, Bayer, Sanofi Genzyme, Roche, and Teva. RAI received honoraria as a speaker and for serving on scientific advisory boards from Bayer, Biogen, GSK, Merck, Novartis, Roche, and Sanofi-Genzyme. MT received travel grants from Novartis, Bayer-Schering, Merck, and Teva; and has participated in clinical trials by Sanofi Aventis, Roche, and Novartis. MG received consulting fees from Teva Canada Innovation, Biogen, Novartis, and Genzyme Sanofi; and lecture payments from Teva Canada Innovation, Novartis, and EMD. He has also received a research grant from the Canadian Institutes of Health Research. TK served on scientific advisory boards for MS International Federation and World Health Organization, BMS, Roche, Janssen, Sanofi Genzyme, Novartis, Merck, and Biogen; on the steering committee for Brain Atrophy Initiative by Sanofi Genzyme, received conference travel support and/or speaker honoraria from WebMD Global, Eisai, Novartis, Biogen, Roche, Sanofi-Genzyme, Teva, BioCSL, and Merck and received research or educational event support from Biogen, Novartis, Genzyme, Roche, Celgene, and Merck. FG received honoraria or research funding from Biogen, Genzyme, Novartis, Teva Neurosciences, and ATARA Pharmaceuticals. OS received honoraria and consulting fees from Bayer-Schering, Novartis, Merck, Biogen, and Genzyme. SK received compensation for scientific advisory board activity from Merck and Roche. BY received honoraria as a speaker and member of scientific advisory boards from Sanofi, Bayer, Biogen, Merck, Janssen, Novartis, Roche, and Aspen. MJ received consulting fees, speaker honoraria, and/or travel expenses for scientific meetings from Alexion, Bayer Healthcare, Biogen, Bristol Myers Squibb, Celgene, Janssen, Merck Serono, Novartis, Roche, Sanofi, and Teva. YB received speaker honoraria/consulting fees from Merck, Biogen, Roche, Bristol Myers Squibb, Novartis, Sanofi, and Sandoz. CO-G received honoraria as a consultant on scientific advisory boards from Biogen, Celgene, Merck, Novartis, Roche, Sanofi-Genzyme, and TEVA. AA received speaker honoraria from Novartis and Alexion. SH has received unrestricted educational grants or speaking honoraria from Biogen, Merck Serono, Novartis, Roche, and Sanofi Genzyme. PM received speaker fees and travel grants from Novartis, Biogen, T'évalua, and Sanofi. RAm received conference travel support from Novartis, Teva, Biogen, Bayer, and Merck and has participated in clinical trials by Biogen, Novartis, Teva, and Actelion. KdG served on scientific advisory boards for Roche, Janssen, Sanofi-Genzyme, Novartis, and Merck, received conference fees and travel support from Novartis, Biogen, Sanofi-Genzyme, Teva, AbbVie, and Merck, and received educational event support from Novartis. CM received honoraria as a consultant on scientific advisory boards for Genzyme, BMS, Janssen, Biogen, Merck, Roche, and Novartis; has received travel grants from Roche and Novartis. JP accepted travel compensation from Novartis, Biogen, Genzyme, Teva, and speaking honoraria from Biogen, Novartis, Genzyme, and Teva. NJ is a local principal investigator on commercial studies funded by Novartis, Biogen, Amicus, and Sanofi. JI declared no competing interests. FS has served on scientific advisory boards for, served as a consultant for, received support for congress participation, or received speaker honoraria from Alexion, Biogen, Bristol Myers Squibb, Merck, Novartis, Roche, and Sanofi Genzyme. His laboratory has received research support from Biogen, Merck, Novartis, Roche, and Sanofi Genzyme. HJ declared no competing interests. PR has served on scientific advisory boards for, served as consultant for, received support for congress participation, or received speaker honoraria from Alexion, Biogen, Bristol Myers Squibb, Merck, Novartis, Roche, and Sanofi Genzyme. TSe received and has served on scientific advisory boards for, served as a consultant for, received support for congress participation, or received speaker honoraria from Biogen, Merck, Novartis, Roche, and Sanofi. T. Sejbaeks received unrestricted research grants to his research institution from Biogen, Merck, and Roche and is currently engaged in sponsor-initiated research projects by Eisai, Lundbeck, Roche, and Sanofi. MP declared no competing interests. JC has received speaker honoraria from Biogen. MS has served on scientific advisory boards for, served as a consultant for, received support for congress participation, participated in industrial trials with, or received speaker honoraria from Bayer, Biogen, Merck, Novartis, Roche, and Sanofi Genzyme. JH has received honoraria for serving on advisory boards for Biogen, Sanofi-Genzyme, and Novartis and speaker's fees from Biogen, Novartis, Merck Serono, Bayer-Schering, Teva, and Sanofi-Genzyme. He has served as P.I. for projects or received unrestricted research support from BiogenIdec, Merck Serono, TEVA, Sanofi-Genzyme, and Bayer-Schering. His MS research is funded by the Swedish Research Council and the Swedish Brain Foundation. The authors declare that this study received funding from Biogen. The funder had the following involvement with the study: study design and manuscript review. The funder was not involved in the collection of data, analysis, writing of the article, or the decision to submit it for publication.
The author(s) declared that they were an editorial board member of Frontiers, at the time of submission. This had no impact on the peer review process and the final decision.
Publisher's note
All claims expressed in this article are solely those of the authors and do not necessarily represent those of their affiliated organizations, or those of the publisher, the editors and the reviewers. Any product that may be evaluated in this article, or claim that may be made by its manufacturer, is not guaranteed or endorsed by the publisher.
References
1. Fymat AL. Multiple sclerosis: II. Diagnosis and symptoms management. J Neurol Psychol Res. (2023) 4:1–21.
2. Harding K, Williams O, Willis M, Hrastelj J, Rimmer A, Joseph F, et al. Clinical outcomes of escalation vs early intensive disease-modifying therapy in patients with multiple sclerosis. JAMA Neurol. (2019) 76:536–41. doi: 10.1001/jamaneurol.2018.4905
3. Spelman T, Magyari M, Piehl F, Svenningsson A, Rasmussen PV, Kant M, et al. Treatment escalation vs immediate initiation of highly effective treatment for patients with relapsing-remitting multiple sclerosis: Data from 2 different national strategies. JAMA Neurol. (2021) 78:1197–204. doi: 10.1001/jamaneurol.2021.2738
4. Lorscheider J, Benkert P, Lienert C, Hänni P, Derfuss T, Kuhle J, et al. Comparative analysis of dimethyl fumarate and fingolimod in relapsing–remitting multiple sclerosis. J Neurol. (2021) 268:941–9. doi: 10.1007/s00415-020-10226-6
5. Papp V, Buron MD, Siersma V, Rasmussen PV, Illes Z, Kant M, et al. Real-world outcomes for a complete nationwide cohort of more than 3200 teriflunomide-treated multiple sclerosis patients in The Danish Multiple Sclerosis Registry. PLoS ONE. (2021) 16:e0250820. doi: 10.1371/journal.pone.0250820
6. Setayeshgar S, Kingwell E, Zhu F, Zhang T, Carruthers R, Marrie RA, et al. Persistence and adherence to the new oral disease-modifying therapies for multiple sclerosis: a population-based study. Mult Scler Relat Disord. (2019) 27:364–9. doi: 10.1016/j.msard.2018.11.004
7. Evans C, Marrie RA, Zhu F, Leung S, Lu X, Melesse DY, et al. Adherence and persistence to drug therapies for multiple sclerosis: a population-based study. Mult Scler Relat Disord. (2016) 8:78–85. doi: 10.1016/j.msard.2016.05.006
8. Warrender-Sparkes M, Spelman T, Izquierdo G, Trojano M, Lugaresi A, Grand'Maison F, et al. The effect of oral immunomodulatory therapy on treatment uptake and persistence in multiple sclerosis. Multiple Sclerosis J. (2016) 22:520–532. doi: 10.1177/1352458515594041
9. Bigaut K, Cohen M, Durand-Dubief F, Maillard E, Planque E, Zephir H, et al. How to switch disease-modifying treatments in multiple sclerosis: guidelines from the French Multiple Sclerosis Society (SFSEP). Mult Scler Relat Disord. (2021) 103076. doi: 10.1016/j.msard.2021.103076
10. Patti F, Chisari CG, D'Amico E, Annovazzi P, Banfi P, Bergamaschi R, et al. Clinical and patient determinants of changing therapy in relapsing-remitting multiple sclerosis (SWITCH study). Mult Scler Relat Disord. (2020) 42:102124. doi: 10.1016/j.msard.2020.102124
11. Trojano M, Tintore M, Montalban X, Hillert J, Kalincik T, Iaffaldano P, et al. Treatment decisions in multiple sclerosis—insights from real-world observational studies. Nat Rev Neurol. (2017) 13:105–18. doi: 10.1038/nrneurol.2016.188
12. Hillert J, Magyari M, Soelberg Sørensen P, Butzkueven H, Van Der Welt A, Vukusic S, et al. Treatment switching and discontinuation over 20 years in the big multiple sclerosis data network. Front Neurol. (2021) 12:295. doi: 10.3389/fneur.2021.647811
13. Stühler E, Braune S, Lionetto F, Heer Y, Jules E, Westermann C, et al. Framework for personalized prediction of treatment response in relapsing remitting multiple sclerosis. BMC Med Res Methodol. (2020) 20:1–15. doi: 10.1186/s12874-020-0906-6
14. Rotstein D, Montalban X. Reaching an evidence-based prognosis for personalized treatment of multiple sclerosis. Nat Rev Neurol. (2019) 15:287–300. doi: 10.1038/s41582-019-0170-8
15. Chitnis T, Prat A. A roadmap to precision medicine for multiple sclerosis. Multiple Scler J. (2020) 26:522–32. doi: 10.1177/1352458519881558
16. Havas J, Leray E, Rollot F, Casey R, Michel L, Lejeune F, et al. Predictive medicine in multiple sclerosis: a systematic review. Mult Scler Relat Disord. (2020) 40:101928. doi: 10.1016/j.msard.2020.101928
17. Iaffaldano P, Lucisano G, Butzkueven H, Hillert J, Hyde R, Koch-Henriksen N, et al. Early treatment delays long-term disability accrual in RRMS: results from the BMSD network. Multiple Sclerosis J. (2021) 27:13524585211010128. doi: 10.1177/13524585211010128
18. Kalincik T, Butzkueven H. The MSBase registry: informing clinical practice. Multiple Scler J. (2019) 25:1828–34. doi: 10.1177/1352458519848965
19. Hillert J, Stawiarz L. The Swedish MS registry–clinical support tool and scientific resource. Acta Neurol Scand. (2015) 132:11–9. doi: 10.1111/ane.12425
20. Magyari M, Joensen H, Laursen B, Koch-Henriksen N. The Danish multiple sclerosis registry. Brain Behav. (2021) 11:e01921. doi: 10.1002/brb3.1921
21. Trojano M, Bergamaschi R, Amato MP, Comi G, Ghezzi A, Lepore V, et al. The Italian multiple sclerosis register. Neurol Sci. (2019) 40:155–65. doi: 10.1007/s10072-018-3610-0
22. Vukusic S, Casey R, Rollot F, Brochet B, Pelletier J, Laplaud DA, et al. Observatoire Français de la Sclérose en Plaques (OFSEP): a unique multimodal nationwide MS registry in France. Multiple Scler J. (2020) 26:118–22. doi: 10.1177/1352458518815602
23. Spelman T, Horakova D, Ozakbas S, Alroughani R, Onofrj M, Kalincik T, et al. Switching to natalizumab or fingolimod in multiple sclerosis: comparative effectiveness and effect of pre-switch disease activity. Multiple Scler Relat Disor. (2023) 70:104477. doi: 10.1016/j.msard.2022.104477
24. Gutierrez RG. Parametric frailty and shared frailty survival models. Stata J. (2002) 2:22–44. doi: 10.1177/1536867X0200200102
25. Meyniel C, Spelman T, Jokubaitis VG, Trojano M, Izquierdo G, Grand'Maison F, et al. Country, sex, EDSS change and therapy choice independently predict treatment discontinuation in multiple sclerosis and clinically isolated syndrome. PLoS ONE. (2012) 7:e38661. doi: 10.1371/journal.pone.0038661
26. Kalincik T, Manouchehrinia A, Sobisek L, Jokubaitis V, Spelman T, Horakova D, et al. Towards personalized therapy for multiple sclerosis: prediction of individual treatment response. Brain. (2017) 140:2426–43. doi: 10.1093/brain/awx185
27. Ayrignac X, Bigaut K, Pelletier J, de Seze J, Demortiere S, Collongues N, et al. First line treatment failure: Predictive factors in a cohort of 863 relapsing remitting MS patients. Mult Scler Relat Disord. (2021) 48:102686. doi: 10.1016/j.msard.2020.102686
28. Sorensen PS, Kopp TI, Joensen H, Olsson A, Sellebjerg F, Magyari M. Age and sex as determinants of treatment decisions in patients with relapsing-remitting MS. Mult Scler Relat Disord. (2021) 50:102813. doi: 10.1016/j.msard.2021.102813
29. Patti F, Chisari CG, Arena S, Toscano S, Finocchiaro C, Fermo SL, et al. Factors driving delayed time to multiple sclerosis diagnosis: results from a population-based study. Mult Scler Relat Disord. (2022) 57:103361. doi: 10.1016/j.msard.2021.103361
30. Ghezzi A. European and American guidelines for multiple sclerosis treatment. Neurol Ther. (2018) 7:189–94. doi: 10.1007/s40120-018-0112-1
31. Ziemssen T, Derfuss T, de Stefano N, Giovannoni G, Palavra F, Tomic D, et al. Optimizing treatment success in multiple sclerosis. J Neurol. (2016) 263:1053–65. doi: 10.1007/s00415-015-7986-y
32. Gross RH, Corboy JR. Monitoring, switching, and stopping multiple sclerosis disease-modifying therapies. Continuum. (2019) 25:715–35. doi: 10.1212/CON.0000000000000738
33. Hauser SL, Cree BA. Treatment of multiple sclerosis: a review. Am J Med. (2020) 133:1380–90. doi: 10.1016/j.amjmed.2020.05.049
34. Wattjes MP, Ciccarelli O, Reich DS, Banwell B, de Stefano N, Enzinger C, et al. 2021 MAGNIMS–CMSC–NAIMS consensus recommendations on the use of MRI in patients with multiple sclerosis. Lancet Neurol. (2021) 20:653–70. doi: 10.1016/S1474-4422(21)00095-8
35. Cross A, Riley C. Treatment of multiple sclerosis. Continuum. (2022) 28:1025–51. doi: 10.1212/CON.0000000000001170
36. Stankiewicz JM, Weiner HL. An argument for broad use of high efficacy treatments in early multiple sclerosis. Neurol Neuroimmunol Neuroinflam. (2020) 7:636. doi: 10.1212/NXI.0000000000000636
37. Cree BA, Mares J, Hartung HP. Current therapeutic landscape in multiple sclerosis: an evolving treatment paradigm. Curr Opin Neurol. (2019) 32:365–77. doi: 10.1097/WCO.0000000000000700
38. Sabathé C, Casey R, Vukusic S, Leray E, Mathey G, De Sèze J, et al. Improving the decision to switch from first-to second-line therapy in multiple sclerosis: a dynamic scoring system. Multiple Scler J. (2023) 29:236–47. doi: 10.1177/13524585221139156
39. Berger T, Adamczyk-Sowa M, Csépány T, Fazekas F, Fabjan TH, Horáková D, et al. Factors influencing daily treatment choices in multiple sclerosis: practice guidelines, biomarkers and burden of disease. Ther Adv Neurol Disord. (2020) 13:1756286420975223. doi: 10.1177/1756286420975223
Keywords: multiple sclerosis, disease modifying treatment (DMT), treatment switching, disease registry, real world evidence (RWE)
Citation: Spelman T, Magyari M, Butzkueven H, Van Der Walt A, Vukusic S, Trojano M, Iaffaldano P, Horáková D, Drahota J, Pellegrini F, Hyde R, Duquette P, Lechner-Scott J, Sajedi SA, Lalive P, Shaygannejad V, Ozakbas S, Eichau S, Alroughani R, Terzi M, Girard M, Kalincik T, Grand'Maison F, Skibina O, Khoury SJ, Yamout B, Sa MJ, Gerlach O, Blanco Y, Karabudak R, Oreja-Guevara C, Altintas A, Hughes S, McCombe P, Ampapa R, de Gans K, McGuigan C, Soysal A, Prevost J, John N, Inshasi J, Stawiarz L, Manouchehrinia A, Forsberg L, Sellebjerg F, Glaser A, Pontieri L, Joensen H, Rasmussen PV, Sejbaek T, Poulsen MB, Christensen JR, Kant M, Stilund M, Mathiesen H, Hillert J and the Big MS Data Network: a collaboration of the Czech MS Registry, the Danish MS Registry, Italian MS Registry, Swedish MS Registry, MSBase Study Group, and OFSEP (2023) Predictors of treatment switching in the Big Multiple Sclerosis Data Network. Front. Neurol. 14:1274194. doi: 10.3389/fneur.2023.1274194
Received: 07 August 2023; Accepted: 27 November 2023;
Published: 22 December 2023.
Edited by:
Valentina Tomassini, University of Studies G. d'Annunzio Chieti and Pescara, ItalyReviewed by:
Lucienne Costa-Frossard França, Servicio de Neurología - Hospital Universitario Ramón y Cajal, SpainYinan Huang, University of Mississippi, United States
Copyright © 2023 Spelman, Magyari, Butzkueven, Van Der Walt, Vukusic, Trojano, Iaffaldano, Horáková, Drahota, Pellegrini, Hyde, Duquette, Lechner-Scott, Sajedi, Lalive, Shaygannejad, Ozakbas, Eichau, Alroughani, Terzi, Girard, Kalincik, Grand'Maison, Skibina, Khoury, Yamout, Sa, Gerlach, Blanco, Karabudak, Oreja-Guevara, Altintas, Hughes, McCombe, Ampapa, de Gans, McGuigan, Soysal, Prevost, John, Inshasi, Stawiarz, Manouchehrinia, Forsberg, Sellebjerg, Glaser, Pontieri, Joensen, Rasmussen, Sejbaek, Poulsen, Christensen, Kant, Stilund, Mathiesen, Hillert and the Big MS Data Network: a collaboration of the Czech MS Registry, the Danish MS Registry, Italian MS Registry, Swedish MS Registry, MSBase Study Group, and OFSEP. This is an open-access article distributed under the terms of the Creative Commons Attribution License (CC BY). The use, distribution or reproduction in other forums is permitted, provided the original author(s) and the copyright owner(s) are credited and that the original publication in this journal is cited, in accordance with accepted academic practice. No use, distribution or reproduction is permitted which does not comply with these terms.
*Correspondence: Tim Spelman, tim.spelman@burnet.edu.au