- 1Department of Neurology, Beijing Tiantan Hospital, Capital Medical University, Beijing, China
- 2China National Clinical Research Center for Neurological Diseases, Beijing, China
- 3Center of Stroke, Beijing Institute for Brain Disorders, Beijing, China
- 4Research Unit of Artificial Intelligence in Cerebrovascular Disease, Chinese Academy of Medical Sciences, Beijing, China
Background: This study aimed to identify the risk factors and construct a prediction model for the prognosis of intracerebral hemorrhage (ICH) at discharge, 3 months, and 12 months.
Methods: A total of 269 patients with ICH were retrospectively enrolled at our hospital between January 2014 and August 2016. The prognosis of ICH was assessed using the modified Rankin Scale (mRS); an mRS score > 2 was considered a poor outcome. The primary endpoint was the 3-month mRS, whereas the secondary endpoints included the mRS scores at discharge and 12 months, and mortality.
Results: The Glasgow Coma Scale (GCS), National Institutes of Health (NIH) stroke scale, International Normalized Ratio (INR), blood urea nitrogen (BUN), epencephalon hemorrhage, and primary hematoma volume were significantly associated with a poor mRS score at 3 months. The predictive value of the prediction model based on these factors for a poor mRS score was 87.8%. Furthermore, a poor mRS score at discharge was affected by the GCS, NIH stroke scale, and primary hematoma volume; the constructed model based on these factors had a predictive value of 87.6%. In addition, the GCS, NIH stroke scale, and surgery were significantly related to a poor mRS score at 12 months; the predictive value of the constructed model based on the aforementioned factors for a poor mRS score was 86.5%. Finally, primary hematoma volume is significantly associated with the risk of 12 months mortality.
Conclusions: The study identified risk factors and constructed a prediction model for poor mRS scores and mortality at discharge, 3 and 12 months in patients with ICH. The prediction models for mRS scores showed a relatively high predictive performance.
1 Introduction
Stroke accounted for 12.2 million incident case of stroke in 2019, and 101 million prevalent cases of stroke (1). In China, there was an estimated 17.8 million adults presented a stroke, and 2.3 million of cases dying as a result (2–4). Stroke can be classified as ischemic or hemorrhagic, with the latter including intracerebral hemorrhage (ICH) and subarachnoid hemorrhage. ICH accounts for 15–20% of stroke cases, with an annual incidence of 24.3 per 100,000 population, and the disability rate can reach as high as 75% (5, 6). Currently, there are no effective therapeutic strategies for ICH, and prognostic factors, such as hyperglycemia, hypertension, hematoma volume, and hematoma expansion, need to be identified to improve the prognosis of ICH (7).
The prognostic factors for ICH have already been identified, including older age, higher blood plasma glutamate, tumor necrosis factor alpha (TNF-α), initial ICH volume, Scandinavian Stroke Scale score, dialysis, diabetes, Glasgow Coma Scale (GCS) score, bilateral dilated pupils, higher international normalized ratio (INR), hematoma in the cerebellum or brainstem, hematoma volume, and the presence of intraventricular hematoma (8–10). However, these studies did not assess the role of cerebral microhemorrhages (CMs) in ICH prognosis. CMs have been observed in 5% of healthy elderly individuals; the risk factors for CMs include a history of stroke or dementia, hypertension, and diabetes (11). Studies have illustrated that the number and location of CMs are significantly associated with the progression of ICH (12, 13); however, whether they affect the prognosis of ICH remains unclear.
Therefore, we conducted this study to identify the potential prognostic factors for ICH and constructed a predictive model for functional status based on CMs and clinical factors.
2 Methods
2.1 Study design and population
Patients with ICH admitted to our hospital between January 2014 and August 2016 were retrospectively collected. This study was approved by the institutional review board of Beijing Tiantan Hospital, Capital Medical University (KY2014-023-02), and informed consent was signed from all patients after explaining the purpose of the study. All procedures performed in studies involving human participants were in accordance with the ethical standards of the institutional and/or national research committee and with the 1964 Helsinki declaration and its later amendments or comparable ethical standards. Patients were included if the following criteria were met: (1) adult patients (age ≥ 18.0 years); (2) patients firstly presenting with the onset of ICH; (3) patients admitted in our department within 24 h; (4) a diagnosis of ICH on the basis of brain computerized tomography; and (5) available information regarding CMs, clinical factors, and modified Rankin Scale (mRS) at discharge, 3 and 12 months. Patients were excluded if they (1) had a primary ventricular hemorrhage; (2) were diagnosed with secondary ICH, including head trauma, brain tumor, aneurysm, cavernous hemangioma, arteriovenous malformations, acute thrombolysis, coagulopathy, or moyamoya disease; or (3) had incomplete information regarding CMs or outcomes.
2.2 Data collection and variable definition
Patients information was collected from electronic medical records, including age, sex, time from onset to admission, smoking, alcohol, body mass index (BMI), hypertension, diabetes mellitus (DM), hyperlipidemia, history of ischemic stroke, history of hemorrhagic stroke, history of subarachnoid hemorrhage, antiplatelet drugs, anticoagulant drugs, antihypertensive drugs, lipid-lowering drugs, antidiabetic drugs, systolic blood pressure (SBP), diastolic blood pressure (DBP), GCS, National Institutes of Health (NIH) stroke scale, white blood cell (WBC), platelet, fasting glucose, INR, creatinine, blood urea nitrogen (BUN), total cholesterol (TC), triglyceride (TG), high density lipoprotein (HDL), low density lipoprotein (LDL), glytamic-pyruvic transaminase (ALT), glutamic oxalacetic transaminase (AST), alkaline phosphatase (ALP), surgical treatment, location, primary hematoma volume, deep CMs, lobar CMs, subtentorial CMs, and total CMs. Blood samples were obtained in all patients within 1 h after admission. The fasting lipid profiles were obtained on the second day of admission. The susceptibility-weighted imaging showed very low signal intensity lesions was defined as CMs. The signal of CMs was similar to the venous on the susceptibility-weighted imaging phase image, and opposite the calcification signal. The inter- and intra-observer reliability for detecting CMs with Cohen's kappa values of 0.96 and 0.98, respectively. Similarly, the Cohen's kappa values of inter- and intra-observer reliability for assessing the location of CMs were 0.96 and 0.98, respectively. The modified Rankin Scale (mRS) was used to assess the prognosis of ICH, and a poor outcome was defined as an mRS score > 2 (14). Functional outcomes (mRS) were assessed after discharge, at 3 months, and at 12 months. The primary endpoint was the 3-months mRS, whereas the secondary endpoints included the mRS scores at discharge and 12 months, and mortality.
2.3 Statistical analysis
The baseline characteristics of the patients with ICH with good or poor functional outcomes were assigned as continuous and categorical variables, respectively. Continuous variables are presented as mean (standard deviation) or median (interquartile range) according to the data distribution, while categorical variables are shown as number and frequency. Differences between good and poor functional outcomes were analyzed using the independent t test, Kruskal–Wallis test, or chi-square test. Univariate logistic regression analysis was performed to identify potential risk factors, and the factors were subjected to the multivariate logistic regression analysis using α = 0.05 and β = 0.10. The deep CMs, lobar CMs, subtentorial CMs, and total CMs were mandatory inclusion model, and the prediction model for functional outcomes at discharge, at 3 months, and 12 months was assessed using the receiver operating characteristic (ROC) curve with the area under the curve (AUC). All tests were two-sided, and P < 0.05 was regarded as statistically significant. SPSS version 18 for Windows (SPSS, Chicago, IL, USA) was used to perform statistical analyses.
3 Results
3.1 Baseline characteristics
Of 269 included patients, the mean age was 56.05 years, and 71% of included patients were male. The median time from onset to admitted was 5.16 h. Thirteen patients (4.83%) received surgical treatment, while the remaining patients treated with conservative therapy. The baseline characteristics according to the 3-month functional outcomes are shown in Table 1. There were significant differences between mRS ≤ 2 and mRS > 2 for age (P = 0.002), time from onset to admission (P = 0.029), the use of antidiabetic drugs (P = 0.015), GCS (P < 0.001), NIH stroke scale (P < 0.001), WBC (P = 0.026), fasting glucose (P < 0.001), INR (P = 0.009), BUN (P = 0.041), LDL (P = 0.002), and primary hematoma volume (P < 0.001). However, we did not find significant differences between the groups regarding sex, smoking, alcohol consumption, BMI, hypertension, DM, hyperlipidemia, history of ischemic stroke, history of hemorrhagic stroke, history of subarachnoid hemorrhage, antiplatelet drugs, anticoagulant drugs, antihypertensive drugs, lipid-lowering drugs, SBP, DBP, platelet, creatinine, TC, TG, HDL, ALT, AST, ALP, surgical treatment, location, deep CMs, lobar CMs, subtentorial CMs, and total CMs.
3.2 Three-month mRS
The identified risk factors for the 3-month mRS are shown in Table 2. The univariate analysis found that poor functional outcomes could be affected by age [odds ratio (OR): 1.03; P = 0.002], time from onset to admission (OR: 0.97; P = 0.011), no use of antidiabetic drugs (P = 0.048) or unclear use of antidiabetic drugs (P = 0.007), GCS (P < 0.001), NIH stroke scale (P < 0.001), WBC (P = 0.005), INR (P = 0.023), BUN (P = 0.046), LDL (P = 0.005), AST (P = 0.031), surgical treatment (P = 0.049), basal ganglia hemorrhage (P = 0.027), and primary hematoma volume (P < 0.001). After adjusting for potential confounders, we noted that the GCS (OR: 1.93; 95% CI: 1.33–2.79; P < 0.001), NIH stroke scale (OR: 1.53; 95% CI: 1.32–1.78; P < 0.001), INR (OR: 1804.29; 95% CI: 6.44–505855.5; P = 0.009), BUN (OR: 1.47; 95% CI: 1.08–2.01; P = 0.014), epencephalon hemorrhage (OR: 115.80; 95% CI: 9.89–1356.22; P < 0.001), and primary hematoma volume (OR: 1.05; 95% CI: 1.01–1.08; P = 0.007) were significantly associated with a poor 3-month mRS score. The predictive value of the prediction model based on these factors was 87.8% (95% CI: 83.4–92.2%; Figure 1).
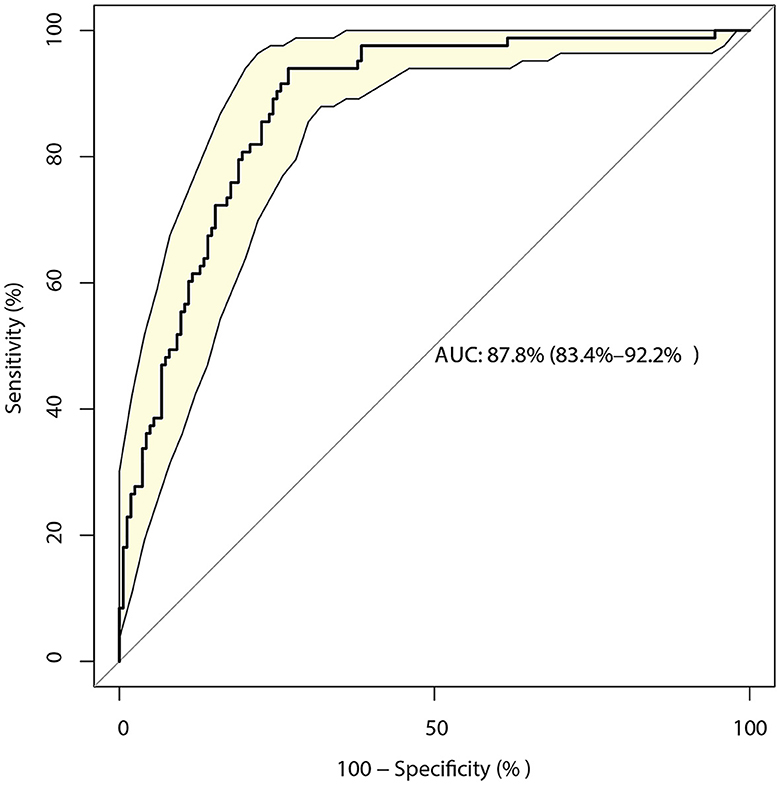
Figure 1. Receiver operating characteristic curve for the risk of a 3-month modified Rankin Scale (mRS) score > 2 in patients with intracerebral hemorrhage (ICH), including the six-component risk factor model.
3.3 mRS at discharge
The identified risk factors for the mRS score at discharge are presented in Table 3. The univariate analyses revealed that the time from onset to admission (P = 0.018), GCS score (P < 0.001), NIH stroke scale score (P < 0.001), AST level (P = 0.002), surgical treatment (P = 0.016), basal ganglia hemorrhage (P = 0.022), and primary hematoma volume (P < 0.001) were significantly associated with poor functional outcomes. After adjusting for potential confounders, the mRS at discharge could be affected by the GCS (OR: 1.48; 95% CI: 1.08–2.03; P = 0.015), NIH stroke scale (OR: 1.47; 95% CI: 1.30–1.66; P < 0.001), and primary hematoma volume (OR: 1.04; 95% CI: 1.01–1.06; P = 0.002). The model constructed based on these factors had a predictive value of 87.6% (95% CI: 83.0–92.2%; Figure 2).
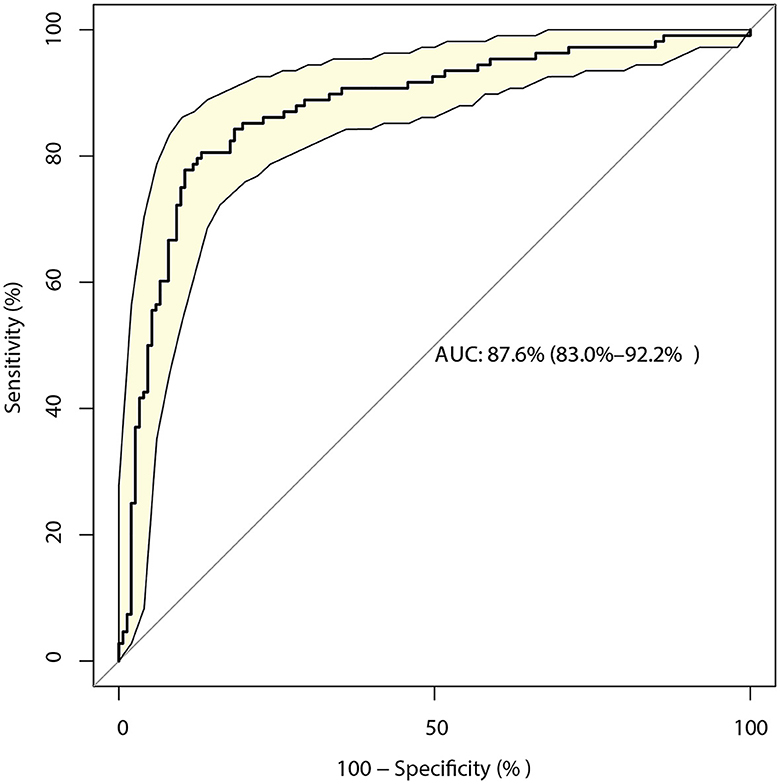
Figure 2. Receiver operating characteristic curve for the risk of a modified Rankin Scale (mRS) score > 2 at discharge in patients with intracerebral hemorrhage (ICH), including the three-component risk factor model.
3.4 Twelve-month mRS
The identified risk factors for the mRS score at 12 months are presented in Table 4. The univariate analyses found that the 12-month mRS score was affected by age (P < 0.001), history of ischemic stroke (P = 0.008), unclear information regarding the use of antidiabetic drugs (P = 0.012), GCS (P < 0.001), NIH stroke scale (P < 0.001), TC (P = 0.006), LDL (P = 0.002), surgical treatment (P = 0.004), and primary hematoma volume (P < 0.001). After adjusting for potential confounders, we noted that the GCS (OR: 2.18; 95% CI: 1.41–3.38; P < 0.001), NIH stroke scale (OR: 1.54; 95% CI: 1.30–1.81; P < 0.001), and treated with surgery (OR: 0.04; 95% CI: 0.00–0.29; P = 0.002) were significantly associated with a poor mRS after 12 months. The prediction model based on the above factors had a predictive value of 86.5% (95% CI: 81.8–91.1%; Figure 3).
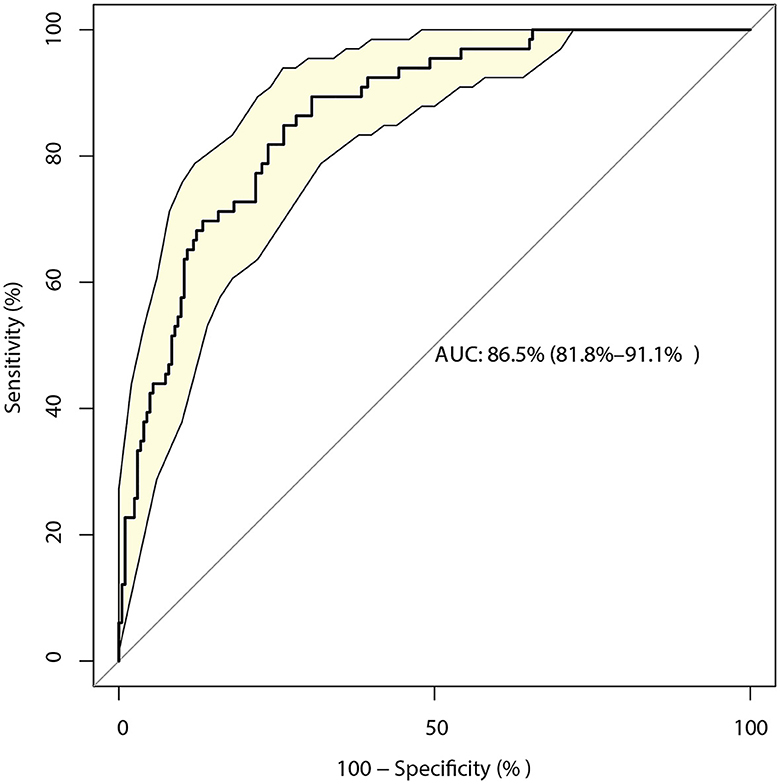
Figure 3. Receiver operating characteristic curve for the risk of a 12-month modified Rankin Scale (mRS) score > 2 in patients with intracerebral hemorrhage (ICH), including the three-component risk factor model.
3.5 Mortality
The number of death occurred at discharge, 3 and 12-months were 1, 5, and 13, respectively. Considering lower incidences of mortality at discharge, and 3-months, the risk factors for 12-months were identified and shown in Table 5. The univariate analyses found that the 12-month mortality was affected by age (P = 0.002), history of ischemic stroke (P < 0.001), GCS (P = 0.046), platelet (P = 0.011), LDL (P = 0.049), and primary hematoma volume (P = 0.024). After adjusting for potential confounders, we noted primary hematoma volume was associated with an increased risk of 12-months mortality (OR: 1.04; 95% CI: 1.00–1.08; P = 0.029).
4 Discussion
This study first identified the risk factors for functional outcomes and mortality at discharge, at 3 months, and at 12-months in patients with ICH. A multifactorial predictive model for the risk of poor functional outcomes was constructed, which could be used to screen high-risk patients, and effective treatments could be applied to improve the prognosis of ICH patients. Our study recruited 269 patients with ICH, of which 94 patients reported a 3-month mRS > 2. The 3-month mRS score was affected by the GCS, NIH stroke scale, INR, BUN, epencephalon hemorrhage, and primary hematoma volume. Moreover, the GCS, NIH stroke scale, and primary hematoma volume could affect the mRS at discharge, while the mRS at 12 months could be affected by the GCS, NIH stroke scale, and surgical treatment. Furthermore, the risk of 12-months mortality could affected by primary hematoma volume. The constructed prediction model for mRS scores at discharge, at 3 months, and at 12 months showed a relatively high predictive performance.
Several studies have constructed prediction models for ICH patients (15–19). Fukuda et al. identified 187 patients with aneurysmal subarachnoid hemorrhage and found that a constructed model containing D-dimer was associated with a better discrimination ability for poor outcomes (15). Wang et al. (16) applied an automated machine learning-based approach to construct a prognostic model for patients with ICH and pointed out that the random forest provides the best predictive performance. Katsuki et al. (17) used data from 140 patients with hypertensive ICH and found that the prediction model constructed using a deep learning framework was superior to the model derived from the ICH score, ICH Grading Scale, and FUNC score. Trevisi et al. (18) found that the important features for functional outcomes included the GCS, Charlson Comorbidity Index, ICH score, ICH volume, pupillary status, brainstem location, age, anticoagulant/antiplatelet agents, intraventricular hemorrhage, and cerebellar location, and the discriminative ability was high. Wu et al. (19) identified 83 patients with ICH and found that the combined model applied radiomic scores obtained from intraparenchymal hemorrhage, intraventricular hemorrhage, and clinical characteristics with high accuracy in predicting poor functional outcomes. However, these studies did not systematically identify the risk factors for poor functional outcomes in patients with ICH, and the characteristics of CMs have not been addressed. Thus, the current study was performed to identify risk factors and construct a prediction model for functional outcomes at discharge, at 3 months, and at 12-months in patients with ICH.
Our study found that the risk factors at various time points differed; similar risk factors included the GCS and NIH stroke scale. A potential reason for this could be that these two scales reflect the neurological status of patients with ICH (20, 21). Additional factors potentially influencing functional outcomes included INR, BUN, epencephalon hemorrhage, surgery, and primary hematoma volume. Several reasons could explain these results: (1) elevated INR has already been demonstrated to be associated with a poor prognosis in patients with ICH, especially in patients treated with warfarin (22); (2) BUN is an important index to assess renal function, which could reflect the severity of disease status in patients with ICH (23); (3) hemorrhage location is significantly related to the prognosis of ICH because the invasion sites are associated with the severity of disease (24); and (4) patients treated with surgery are significantly related to hematoma volume, which could affect the prognosis of ICH (25, 26). Therefore, early intervention strategies should be implemented for identified modifiable risk factors in order to improve patient prognosis.
Our study reported the risk of 12-months mortality could affected by primary hematoma volume, which could explained by the primary hematoma volume are significantly related to the severity of disease and subsequent treatments. Moreover, considering the incidences of mortality at discharge and 3-months were lower than expected, thus the power was not enough to detect potential associations. In addition, although our study found that functional outcomes were not affected by CMs, especially deep, lobar, subtentorial, and total CMs, the prediction model based on CMs and clinical factors for mRS > 2 at discharge, 3 and 12 months had a relatively high predictive performance. Considering that the risk factors for poor functional outcomes have already been identified, effective strategies should be applied to patients at a high risk of poor functional outcomes.
This study has some limitations. First, the analysis was based on retrospective data, and the results of our study could have been affected by selection and recall biases. Second, our study was restricted by single-center study with a small sample size, thus the conclusions of this study should be recommended cautiously. Third, the severity of ICH was not restricted as an inclusion criterion, and the prognosis of ICH could be affected by the presence of more severe or mild symptoms. Fourth, the background therapies for ICH differed among the included patients, which could have affected the prognosis of ICH. Fifth, biomarkers levels change over the course of follow-up was not addressed, which needed further explored. Sixth, the predictive model was not verified using an external cohort.
This study identified the risk factors for functional outcomes and mortality at discharge, at 3 months, and at 12 months in patients with ICH, and the CMs were addressed. Moreover, a prediction model was constructed based on the identified risk factors with relatively high predictive performance, which could be applied in clinical practice to identify high-risk patients. Further large-scale prospective studies are required to validate the constructed model.
Data availability statement
The original contributions presented in the study are included in the article/supplementary material, further inquiries can be directed to the corresponding author.
Ethics statement
The studies involving humans were approved by the Institutional Review Board of Beijing Tiantan Hospital, Capital Medical University (KY2014-023-02). The studies were conducted in accordance with the local legislation and institutional requirements. The participants provided their written informed consent to participate in this study.
Author contributions
HF: Conceptualization, Data curation, Formal analysis, Writing—original draft. XW: Data curation, Formal analysis, Writing—review & editing. WW: Data curation, Formal analysis, Writing—review & editing. XZ: Conceptualization, Formal analysis, Funding acquisition, Project administration, Writing—review & editing.
Funding
The author(s) declare financial support was received for the research, authorship, and/or publication of this article. This study was funded by National Science and Technology Major Project (2017ZX09304018) and National Key Research and Development Program of China (Grant No. 2018YFC1312200/2018YFC1312204).
Conflict of interest
The authors declare that the research was conducted in the absence of any commercial or financial relationships that could be construed as a potential conflict of interest.
Publisher's note
All claims expressed in this article are solely those of the authors and do not necessarily represent those of their affiliated organizations, or those of the publisher, the editors and the reviewers. Any product that may be evaluated in this article, or claim that may be made by its manufacturer, is not guaranteed or endorsed by the publisher.
References
1. Collaborators GBDS. Global, regional, and national burden of stroke and its risk factors, 1990-2019: a systematic analysis for the global burden of disease study 2019. Lancet Neurol. (2021) 20:795–820. doi: 10.1016/S1474-4422(21)00252-0
2. Tu WJ, Zhao Z, Yin P, Cao L, Zeng J, Chen H, et al. Estimated burden of stroke in China in 2020. JAMA Netw Open. (2023) 6:e231455. doi: 10.1001/jamanetworkopen.2023.1455
3. Tu WJ, Wang LD. Special writing group of China stroke surveillance report. China stroke surveillance report 2021. Mil Med Res. (2023) 10:33. doi: 10.1186/s40779-023-00463-x
4. Wang W, Jiang B, Sun H, Ru X, Sun D, Wang L, et al. Prevalence, incidence, and mortality of stroke in China: results from a nationwide population-based survey of 480 687 adults. Circulation. (2017) 135:759–71. doi: 10.1161/CIRCULATIONAHA.116.025250
5. van Asch CJ, Luitse MJ, Rinkel GJ, van der Tweel I, Algra A, Klijn CJ. Incidence, case fatality, and functional outcome of intracerebral haemorrhage over time, according to age, sex, and ethnic origin: a systematic review and meta-analysis. Lancet Neurol. (2010) 9:167–76. doi: 10.1016/S1474-4422(09)70340-0
6. Ikram MA, Wieberdink RG, Koudstaal PJ. International epidemiology of intracerebral hemorrhage. Curr Atheroscler Rep. (2012) 14:300–6. doi: 10.1007/s11883-012-0252-1
7. Gong Y, Wang Y, Chen D, Teng Y, Xu F, Yang P. Predictive value of hyperglycemia on prognosis in spontaneous intracerebral hemorrhage patients. Heliyon. (2023) 9:e14290. doi: 10.1016/j.heliyon.2023.e14290
8. On S, Poh R, Salor RS, Philip RG, Chekkattu RH, Lim MA, et al. The burden and risks factors for intracerebral hemorrhage in a Southeast Asian population. Clin Neurol Neurosurg. (2022) 214:107145. doi: 10.1016/j.clineuro.2022.107145
9. Overvad TF, Andersen SD, Larsen TB, Lip GYH, Sogaard M, Skjoth F, et al. Incidence and prognostic factors for recurrence of intracerebral hemorrhage in patients with and without atrial fibrillation: a cohort study. Thromb Res. (2020) 191:1–8. doi: 10.1016/j.thromres.2020.03.024
10. Rendevski V, Aleksovski B, Stojanov D, Rendevska AM, Aleksovski V, Gjorgoski I. Modeling prognostic factors for poor neurological outcome in conservatively treated patients with intracerebral hemorrhage: a focus on TNF-alpha. Clin Neurol Neurosurg. (2018) 172:51–8. doi: 10.1016/j.clineuro.2018.06.027
11. Cordonnier C, Al-Shahi Salman R, Wardlaw J. Spontaneous brain microbleeds: systematic review, subgroup analyses and standards for study design and reporting. Brain. (2007) 130:1988–2003. doi: 10.1093/brain/awl387
12. Jeon SB, Kang DW, Cho AH, Lee EM, Choi CG, Kwon SU, et al. Initial microbleeds at MR imaging can predict recurrent intracerebral hemorrhage. J Neurol. (2007) 254:508–12. doi: 10.1007/s00415-006-0406-6
13. Jouvent E, Puy L, Chabriat H. Cerebral microhemorrhages: significance, associations, diagnosis, and treatment. Curr Treat Options Neurol. (2016) 18:35. doi: 10.1007/s11940-016-0418-1
14. van Swieten JC, Koudstaal PJ, Visser MC, Schouten HJ, van Gijn J. Interobserver agreement for the assessment of handicap in stroke patients. Stroke. (1988) 19:604–7. doi: 10.1161/01.STR.19.5.604
15. Fukuda H, Lo B, Yamamoto Y, Handa A, Yamamoto Y, Kurosaki Y, et al. Plasma D-dimer may predict poor functional outcomes through systemic complications after aneurysmal subarachnoid hemorrhage. J Neurosurg. (2017) 127:284–90. doi: 10.3171/2016.5.JNS16767
16. Wang HL, Hsu WY, Lee MH, Weng HH, Chang SW, Yang JT, et al. Automatic machine-learning-based outcome prediction in patients with primary intracerebral hemorrhage. Front Neurol. (2019) 10:910. doi: 10.3389/fneur.2019.00910
17. Katsuki M, Kakizawa Y, Nishikawa A, Yamamoto Y, Uchiyama T. Postsurgical functional outcome prediction model using deep learning framework (prediction one, sony network communications Inc.) for hypertensive intracerebral hemorrhage. Surg Neurol Int. (2021) 12:203. doi: 10.25259/SNI_222_2021
18. Trevisi G, Caccavella VM, Scerrati A, Signorelli F, Salamone GG, Orsini K, et al. Machine learning model prediction of 6-month functional outcome in elderly patients with intracerebral hemorrhage. Neurosurg Rev. (2022) 45:2857–67. doi: 10.1007/s10143-022-01802-7
19. Wu TC, Liu YL, Chen JH, Ho CH, Zhang Y, Su MY. Prediction of poor outcome in stroke patients using radiomics analysis of intraparenchymal and intraventricular hemorrhage and clinical factors. Neurol Sci. (2023) 44:1289–300. doi: 10.1007/s10072-022-06528-4
20. Wang WJ, Lu JJ, Wang YJ, Wang CX, Wang YL, Hoff K, et al. Clinical characteristics, management, and functional outcomes in Chinese patients within the first year after intracerebral hemorrhage: analysis from China national stroke registry. CNS Neurosci Ther. (2012) 18:773–80. doi: 10.1111/j.1755-5949.2012.00367.x
21. Finocchi C, Balestrino M, Malfatto L, Mancardi G, Serrati C, Gandolfo C. National Institutes of Health Stroke Scale in patients with primary intracerebral hemorrhage. Neurol Sci. (2018) 39:1751–5. doi: 10.1007/s10072-018-3495-y
22. Curtze S, Strbian D, Meretoja A, Putaala J, Eriksson H, Haapaniemi E, et al. Higher baseline international normalized ratio value correlates with higher mortality in intracerebral hemorrhage during warfarin use. Eur J Neurol. (2014) 21:616–22. doi: 10.1111/ene.12352
23. Gao B, Gu H, Yu W, Liu S, Zhou Q, Kang K, et al. Admission dehydration is associated with significantly lower in-hospital mortality after intracerebral hemorrhage. Front Neurol. (2021) 12:637001. doi: 10.3389/fneur.2021.637001
24. Eslami V, Tahsili-Fahadan P, Rivera-Lara L, Gandhi D, Ali H, Parry-Jones A, et al. Influence of intracerebral hemorrhage location on outcomes in patients with severe intraventricular hemorrhage. Stroke. (2019) 50:1688–95. doi: 10.1161/STROKEAHA.118.024187
25. Wang N, Lin W, Zhu X, Tu Q, Zhu D, Qu S, et al. Conventional craniotomy versus conservative treatment in patients with minor spontaneous intracerebral hemorrhage in the basal ganglia. Chin Neurosurg J. (2022) 8:26. doi: 10.1186/s41016-022-00288-y
Keywords: risk factors, prediction model, prognosis, intracerebral hemorrhage, cerebral microhemorrhage
Citation: Feng H, Wang X, Wang W and Zhao X (2023) Risk factors and a prediction model for the prognosis of intracerebral hemorrhage using cerebral microhemorrhage and clinical factors. Front. Neurol. 14:1268627. doi: 10.3389/fneur.2023.1268627
Received: 28 July 2023; Accepted: 30 October 2023;
Published: 23 November 2023.
Edited by:
Zhe Kang Law, National University of Malaysia, MalaysiaReviewed by:
Wen-Jun Tu, Chinese Academy of Medical Sciences and Peking Union Medical College, ChinaJafri Malin Abdullah, University of Science Malaysia, Malaysia
Copyright © 2023 Feng, Wang, Wang and Zhao. This is an open-access article distributed under the terms of the Creative Commons Attribution License (CC BY). The use, distribution or reproduction in other forums is permitted, provided the original author(s) and the copyright owner(s) are credited and that the original publication in this journal is cited, in accordance with accepted academic practice. No use, distribution or reproduction is permitted which does not comply with these terms.
*Correspondence: Xingquan Zhao, enhxQHZpcC4xNjMuY29t