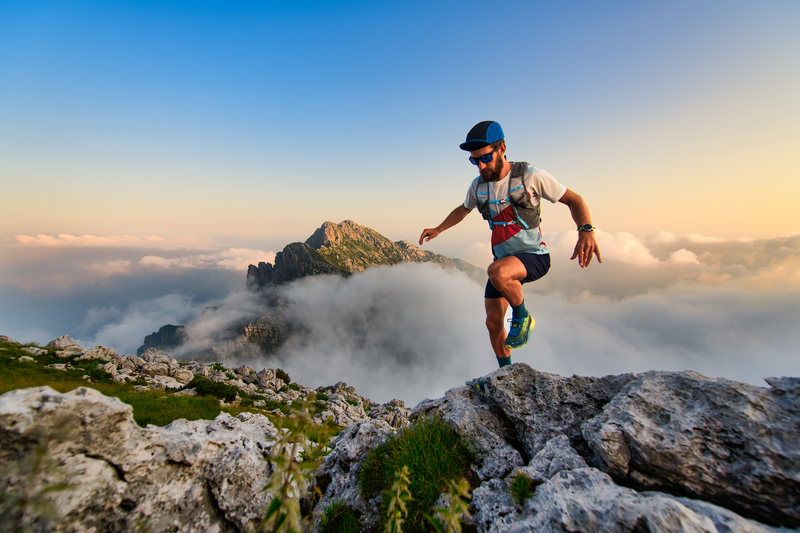
95% of researchers rate our articles as excellent or good
Learn more about the work of our research integrity team to safeguard the quality of each article we publish.
Find out more
STUDY PROTOCOL article
Front. Neurol. , 30 November 2023
Sec. Stroke
Volume 14 - 2023 | https://doi.org/10.3389/fneur.2023.1246888
Background: Stroke is a leading cause of lifelong disability worldwide, partially driven by a reduced ability to use the upper limb in daily life causing increased dependence on caregivers. However, post-stroke functional impairments have only been investigated using limited clinical scores, during short-term longitudinal studies in relatively small patient cohorts. With the addition of technology-based assessments, we propose to complement clinical assessments with more sensitive and objective measures that could more holistically inform on upper limb impairment recovery after stroke, its impact on upper limb use in daily life, and on overall quality of life. This paper describes a pragmatic, longitudinal, observational study protocol aiming to gather a uniquely rich multimodal database to comprehensively describe the time course of upper limb recovery in a representative cohort of 400 Asian adults after stroke. Particularly, we will characterize the longitudinal relationship between upper limb recovery, common post-stroke impairments, functional independence and quality of life.
Methods: Participants with stroke will be tested at up to eight time points, from within a month to 3 years post-stroke, to capture the influence of transitioning from hospital to community settings. We will perform a battery of established clinical assessments to describe the factors most likely to influence upper limb recovery. Further, we will gather digital health biomarkers from robotic or wearable sensing technology-assisted assessments to sensitively characterize motor and somatosensory impairments and upper limb use in daily life. We will also use both quantitative and qualitative measures to understand health-related quality of life. Lastly, we will describe neurophysiological motor status using transcranial magnetic stimulation.
Statistics: Descriptive analyses will be first performed to understand post-stroke upper limb impairments and recovery at various time points. The relationships between digital biomarkers and various domains will be explored to inform key aspects of upper limb recovery and its dynamics using correlation matrices. Multiple statistical models will be constructed to characterize the time course of upper limb recovery post-stroke. Subgroups of stroke survivors exhibiting distinct recovery profiles will be identified.
Conclusion: This is the first study complementing clinical assessments with technology-assisted digital biomarkers to investigate upper limb sensorimotor recovery in Asian stroke survivors. Overall, this study will yield a multimodal data set that longitudinally characterizes post-stroke upper limb recovery in functional impairments, daily-life upper limb use, and health-related quality of life in a large cohort of Asian stroke survivors. This data set generates valuable information on post-stroke upper limb recovery and potentially allows researchers to identify different recovery profiles of subgroups of Asian stroke survivors. This enables the comparisons between the characteristics and recovery profiles of stroke survivors in different regions. Thus, this study lays out the basis to identify early predictors for upper limb recovery, inform clinical decision-making in Asian stroke survivors and establish tailored therapy programs.
Clinical trial registration: ClinicalTrials.gov, identifier: NCT05322837.
At least 50% of stroke survivors suffer from upper limb impairments in the acute stage (1, 2), and the variety of recovery profiles widely vary (3, 4). Gaining a better understanding of the neurophysiological, behavioral and contextual factors affecting recovery post-stroke is essential to developing more tailored and optimized upper limb rehabilitation approaches and reducing the risk of persistent upper limb impairments (5, 6), which are known to lead to poor self-efficacy (7) and low self-reported health-related quality of life (Hr-QOL) (8, 9). However, this relies on rich data sets tracking upper limb sensorimotor recovery with sufficient time resolution and for a sufficiently long time beyond the initial hospitalization, and considering the multi-dimensional nature of upper limb deficits and their impact on quality of life.
During the last few years, understanding the time course of recovery after stroke as well as its major determinants has received increasing attention (10–14). However, only few data sets have collected information (i) with a high temporal resolution (i.e., multiple time points during both acute/subacute and chronic phases), (ii) over a time period longer than 1 year [but (see 14, 15)], (iii) in a large and representative patient sample, and (iv) through the lens of multiple domains of functioning, Hr-QOL and disability. First available data sets, particularly measuring the recovery of upper limb function (3, 4, 16, 17), have revealed important new insights into predicting rehabilitation outcomes (3, 16) and, recently, also trajectories of recovery (4). Here, we aim to investigate the added value of technology-assisted assessments in an Asian cohort longitudinally. “Digital biomarkers,” i.e., metrics derived from technology-assisted assessments (18–20), offer the advantage of providing validated (21–23), objective and traceable descriptions of upper limb behavior on sensitive, continuous scales, often without ceiling effects (24). Digital biomarkers are helpful to complement clinical scores, uniquely inform on movement quality and allow for separating behavioral restitution from compensatory strategies (25), a key aspect that existing longitudinal studies on recovery failed to capture (10, 13).
To reach this aim, we designed a study protocol to gather a rich multimodal data set tracking the time course of upper limb recovery in a representative cohort of 400 Asian adults after ischemic or hemorrhagic stroke for up to 3 years and characterize the longitudinal relationship between upper limb recovery and independence/quality of life of post-stroke individuals. Compared to Caucasian stroke survivors, Asian stroke survivors have a higher incidence of intracerebral hemorrhages (ICH), lacunar ischemic strokes (IS) and strokes in the young (<45 years) as well as differential recovery patterns between ICH and IS strokes (26–28). However, there is scarce evidence on the profiles of the Asian stroke population. To answer this, we will employ a carefully selected battery of standardized clinical tests, technology-assisted assessments, neurophysiological assessments, tools for measuring Hr-QOL, as well as qualitative interviews with longitudinal follow-ups to uncover different recovery profiles in Asian stroke survivors. Our data set will, thus, assess multiple domains of upper limb recovery with a focus on sensorimotor function.
Such a longitudinal, comprehensive data set will yield clinically important information on key factors influencing post-stroke upper limb sensorimotor recovery trajectory, activity capacity, activity performance, real-world participation, and quality of life over time. We will use this data set to answer the following research questions: (i) Can digital biomarkers revealed by technology-based assessments and wearables deepen our understanding of post-stroke upper limb recovery when compared to neurophysiological measurements (transcranial magnetic stimulation, TMS), standardized clinical assessments, patient self-reports, and Hr-QOL? (ii) What is the interaction and interdependence between sensorimotor impairments, clinically assessed performance, neurophysiology, quality of life, and upper limb usage in daily life, for stroke survivors? (iii) What are the major determinants of recovery? This data set will provide a new means for quantifying the “natural course” of post-stroke recovery in accordance with the standard of care, providing a strong data-driven foundation on which future therapy could be potentially optimized.
This study is a single-center, prospective longitudinal, observational cohort study. The study was registered on ClinicalTrials.gov with the following registration number: NCT05322837.
In this study, we aim to recruit up to 400 stroke survivors from the Tan Tock Seng Hospital (TTSH) Rehabilitation Centre inpatient stroke rehabilitation unit. TTSH Rehabilitation Centre is the largest stroke rehabilitation hospital in Singapore with a catchment area covering roughly 1/3 of the country. It provides customized stroke upper limb management which includes one-to-one individualized training, upper limb group intensive training, self-directed upper limb therapy, robotic upper limb retraining and neuromuscular electrical stimulation. Our sample of stroke survivors and can be deemed unselected. The inclusion criteria are as follows.
• Stroke confirmed by neurologists, neurosurgeons, and brain imaging (computed tomography (CT), computed tomography angiogram, magnetic resonance imaging (MRI), magnetic resonance angiogram).
• Asian ethnicity.
• Age 21–90 years.
• Montreal Cognitive Assessment (MoCA) scores 18/30 and above.
• Admission to the inpatient stroke rehabilitation unit within 8 weeks of stroke onset.
• Admission Fugl–Meyer score <66.
For qualitative interviews at >6 months post-stroke:
• Capacity to communicate.
• MOCA >24/30 at 6 months.
The exclusion criteria are below.
• Recurrent stroke with modified Rankin score (mRS) of >2 (i.e., recurrent stroke with mRS 0, 1, 2 can be included).
• Upper limb impairment related to conditions of subarachnoid hemorrhage, traumatic brain injury or brain tumors.
• Bilateral strokes leading to upper limb impairments.
• Uncontrolled medical conditions such as hypertension, hypotension, diabetes mellitus, unstable angina, cardiac failure, or sepsis will be excluded.
• Fractures or arthritis of upper limb joints/bones in the affected upper limb.
• Visual Analogue Scale (VAS) pain >5/10 in the affected upper limb.
• MoCA <18/30.
• Severe behavioral disturbance or agitation or epilepsy or other contradictions preventing the study participation.
• Life expectancy <6 months.
• End organ failures on replacements (renal dialysis or renal replacement therapies).
• Minimally responsive or unresponsive awareness (vegetative) states.
• Pregnancy or lactation states.
• Admission to the inpatient stroke rehabilitation unit later than 8 weeks post-stroke.
• (Related to TMS assessment only) a history of epilepsy or seizures, cranial surgeries, metal implants in the body or head, implanted electronics, metallic valves, skull fracture or brain injury, or head or brain surgeries.
For qualitative interviews only at >6 months post-stroke:
• Inability to communicate (aphasia, apraxia and dysarthria).
All participants will undergo their routine care in the inpatient stroke rehabilitation unit. Participation in this study will not have an impact on their intervention plans over time. Depending on their conditions at the discharge time point, they will be discharged to discontinue rehabilitation or to continue their rehabilitation in a community hospital, nursing home, other facilities such as specialist rehabilitation clinics, day rehabilitation centers or at home. After they are discharged, they will be invited back to the same hospital but at a different study site—TTSH Clinic for Advanced Rehabilitation Therapeutics (CART) for follow-up assessments. All therapists in the TTSH Rehabilitation Centre inpatient stroke rehabilitation unit and CART were jointly trained for all clinical and technology-assisted assessments to ensure good reliability.
To better capture the time course of post-stroke upper limb recovery, we aim to conduct clinical, neurophysiological, Hr-QOL, and technology-assisted assessments at various time points arching early subacute, late subacute, and chronic stages following stroke. To further understand the environmental and personal factors in community-dwelling stroke survivors, qualitative data on Hr-QOL related specifically to upper limb recovery in the chronic stages of stroke will also be collected in a subset of participants who have sufficient cognitive function and are willing to share their experience after stroke.
The fastest rate of neurological and functional recovery typically occurs during the first four to 6 weeks following the onset of stroke. As such, we will assess stroke survivors regularly from recruitment to discharge. To establish a baseline, we will collect comprehensive data covering motor, somatosensory and cognitive functions using standardized clinical, neurophysiological, and technology-assisted assessments as soon as patients are admitted to the inpatient stroke rehabilitation unit and recruited in our study (T0). This typically occurs 2–4 weeks post-stroke, as patients are initially treated in the acute unit of the hospital where we unfortunately are not able to perform any assessments. We then follow up on gains in motor and somatosensory function on a fortnightly basis (T1, 4–6 weeks post stroke). Close to expected discharge (T2, typically within 6–8 weeks post stroke), we will again assess motor and somatosensory functions using standardized clinical and technology-assisted assessments. If a participant is admitted later than 2–4 weeks (but no more than 8 weeks) post-stroke, recruitment in the study will still be considered, and T0 will be administered. Following discharge, the time points commonly reported in the literature of 3, 6, and 12 months post-stroke are selected, in addition to further follow-up assessments at 2 and 3 years post-stroke. Considering the change of living environment from bedside to the community, besides routine assessments on the motor, somatosensory, and cognitive functions, we additionally assess Hr-QOL to understand the impact of stroke-induced deficits on daily life. The overview of the assessment time points and assessment tools is described in Table 1.
As the nature of this study is explorative and as there is no generally accepted approach to estimate the sample size requirements for multivariate regression models (29), a classical sample size estimation was not possible. Instead, our target sample was based on previous studies aiming at understanding post-stroke upper limb recovery and logistical considerations. Existing studies have typically reported longitudinal data in samples between 150 to 450 participants (10, 11). Based on this benchmark and considering the annual number of stroke survivors referred to the TTSH inpatient stroke rehabilitation unit as well as a 20% rate of dropout or loss to follow-up, we targeted to enroll 400 participants in the study.
At admission to the rehabilitation facility, the demographics, stroke characteristics, pertinent MRI or CT findings, important acute care data (Intensive Care Unit entry and duration), brain-related surgeries, acute hospital, and rehabilitation length of stay, and details about the prescribed rehabilitation program (therapy goals and content) will be obtained via TTSH electronic medical records. During the study, clinical, neurophysiological, and technology-assisted assessments as well as qualitative interviews will be performed to describe the multiple domains of post-stroke upper limb recovery (Table 1).
For ischemic stroke patients, the NIHSS will be used to objectively quantify the impairment caused by a stroke (30). The NIHSS (0–42) is composed of 11 items, each of which scores a specific ability between 0 and 4. For each item, a score of 0 typically indicates a normal function in that specific ability, while a higher score is indicative of some level of impairment. We will extract this admission data for ischemic strokes.
For hemorrhagic strokes, the GCS (total and subset eye opening, verbal and motor responses scores) which objectively describes the extent of impaired consciousness in all types of acute medical and trauma patients will be used (31). The scale assesses patients according to three aspects of responsiveness: eye-opening, motor, and verbal responses. Reporting each of these separately provides a clear, communicable picture of a patient. The findings in each component of the scale can aggregate into a total GCS which gives a less detailed description but can provide a useful summary of the overall severity. The GCS and its total score have since been incorporated into numerous clinical guidelines and scoring systems for victims of trauma or critical illness.
We will measure the global disability level of our participants at T4, T5, and T6, corresponding to the time points of qualitative interviews. For this purpose, we will use the recommended clinical instrument—the modified Rankin scale (mRS) (32) for measurement. The mRS is a simple scale from 0–6 that measures the degree of disability or dependence in the daily activities of people who have suffered a stroke or other causes of neurological disability (see Table 2 for the scales of assessment tools).
Upper limb motor impairments will be measured with recommended clinical assessments (33, 34), including the Fugl–Meyer Assessment of the Upper Extremity (FMA-UE), shoulder abduction and finger extension (SAFE) scores, and grip strength.
The FMA-UE is a stroke-specific, performance-based impairment index (35). It is designed to assess motor functioning and coordination/speed in patients with post-stroke hemiplegia. It is applied clinically and in research to determine disease severity, describe motor recovery, and plan and assess treatment.
The SAFE score is commonly used in post-stroke recovery prediction models and is calculated by scoring shoulder abduction and finger extension separately, using the Medical Research Council grades, and summated to the SAFE score (36–38). The participant’s strength for each of these movements is scored between 0 and 5, where 0 is no muscle activity and 5 is normal strength and range of movement.
Grip strength is measured using a hand-held DynEx digital grip dynamometer (Fabrication Enterprises, Inc., NY, United States). The participant squeezes the dynamometer with all of their strength three times and an average score is calculated.
Upper limb activity capacity will be measured by the Action Research Arm Test (ARAT) (39), which evaluates upper limb motor capacity in a standardized format using 19 tests of motor function across 4 subsets: grasp., pinch, grip, and gross movement, both distally and proximally, following a stroke.
Upper limb somatosensory impairment will be measured using the Erasmus version of the Nottingham Sensory Assessment (NSA) (40). The Erasmus NSA comprises assessments of tactile sensation (light pressure, pressure, and pinprick), sharp-blunt discrimination, two-point discrimination, and proprioception. For each item, a score of 0 denotes absent sensation, 1 denotes impaired sensation and 2 denotes normal sensation. The higher the score the better the preservation of sensation after stroke.
We will describe the level of spasticity using the Modified Ashworth Scale (MAS) (41) for shoulder adductors, biceps, wrist flexors, and finger flexors. MAS measures resistance during passive soft-tissue stretching and is used as a simple measure of spasticity. The MAS is performed when moving the participant’s limb within their maximal range of motion within a second. It is scored from 0 to 4, with “0” indicating no increase in muscle tone while “4” indicating the affected part(s) is rigid in flexion and extension.
We will describe the overall level of upper limb pain participants are experiencing using a visual analogue scale (VAS 0–10) (42). The VAS is a measurement instrument that tries to measure a characteristic or attitude that is believed to range across a continuum of values and cannot easily be directly measured. VAS pain is a unidimensional measure of pain intensity.
The participant’s confidence in upper limb use after stroke will be assessed using the Upper Limb Self-Efficacy Test (UPSET) (43). The UPSET is a questionnaire comprised of 20 questions to assess how confident the stroke survivors are in using their paretic upper limbs after stroke. For each question that represents a daily functional activity, the participant is asked to rate their level of confidence in using their paretic hand for that particular activity on a Likert scale from 0 to 10 (i.e., 0: not at all confident, 10: very confident).
The Bells Test will be used to assess the level of visual neglect in the participants with stroke (44). The participants will be asked to circle 35 black bells embedded among black distractors (i.e., pictures of houses and horses) on a page placed at the participant’s midline. The level of visual neglect is scored by how many bells have been omitted by the participant on a scoring sheet.
The Trunk Impairment Scale (TIS) will be used to evaluate the trunk control of the participants (45). The TIS assesses static and dynamic sitting balance and trunk coordination in a sitting position, generating a total score between 0 and 23, where a higher score indicates better trunk control. Static sitting balance (score range 0–7) evaluates the ability to remain in a seated position with both feet on the floor and with the legs crossed. The dynamic sitting balance subscale (score range 0–10) assesses lateral flexion of the trunk, initiated from the upper and lower part of the trunk. The trunk coordination subscale (score range 0–6) assesses rotation from the shoulder and pelvic girdle in the horizontal plane.
We will measure functional independence in daily life based on the Functional Independence Measure (FIM, score range 18–126) upon admission and discharge from the rehabilitation ward. The FIM includes measures of independence for self-care, including sphincter control, transfers, locomotion, communication, and social cognition (46). It is an 18-item, 7-level, ordinal scale intended to be sensitive to changes over the course of a comprehensive inpatient medical rehabilitation program.
Cognitive impairments will be rated by the Montreal Cognitive Assessment (MoCA) (47), which consists of simple tasks such as drawing, object naming, memory recall, reading, and mathematical operations (0, worst score, 30: best score).
We will rely on EQ-5D and Stroke Specific Quality of Life (SSQOL) to measure the quality of life after stroke. EQ-5D is a generic quality-of-life measure and it includes mobility, self-care, usual activities, pain/discomfort, and anxiety/depression (48). The SSQOL measures 12 domains including mobility, energy, upper extremity function, work/productivity, mood, self-care, social & family roles, vision, language, thinking, and personality (49).
Transcranial magnetic stimulation (TMS) will be applied over the motor cortex to depolarize neurons and lead to motor evoked potentials (MEPs) for assessing the cortico-spinal excitability as well as resting motor threshold values (RMT) (50). MEPs are the electrical signals recorded from muscles following stimulation of motor pathways within the brain. Surface electromyography (EMG) electrodes are attached to the extensor carpi radialis (ECR) and first dorsal interosseous (FDI) for EMG recording. The “hot spot” of the MEP from ECR and FDI will be first identified in the contralesional (less affected) hemisphere, followed by the ipsilesional (more affected) hemisphere. TMS will be carried out using MagPro R30 (MagVenture, Inc., Denmark).
The main outcome measure of the TMS assessment is a patient’s MEP status, i.e., whether or not MEPs with consistent latencies can be evoked from the ipsilesional hemisphere.
Participants will be considered MEP positive if MEP of any amplitude but with consistent latency can be elicited while the ECR/FDI muscle is either at rest or is preactivated. If this criterion is not met even when stimuli are delivered at maximal intensity, the participant will be considered MEP negative.
For participants who are unable to achieve a RMT, an active motor threshold will be examined. The participant will perform an active wrist extension/make a fist with the less affected hand and hold it there during stimulation, while the investigator looks for a MEP in the ECR or FDI. For active motor threshold, the participant will be considered MEP positive if any amplitude of consistent latency can be elicited in 50% of at least 8 trials while the muscle is active.
Inspired by the PREP2 algorithm (36, 38), TMS measurements will only be formed at T0 and for patients with SAFE scores less than five. This may aid in the understanding of subgroups with good, limited, and poor recovery potential.
The VPIT is a technology-assisted assessment that provides 10 sensitive and validated metrics describing upper limb movement patterns and hand grip forces (21, 22, 51, 52) (Figure 1). The VPIT consists of a virtual goal-directed pick-and-place task that requires coordinated arm and hand movements. It allows for gathering 3D upper limb endpoint kinematic and kinetic data in persons with mild to moderate upper limb impairments. The participant is instructed to squeeze and hold a commercial haptic device to pick up a peg and then insert it into one of the holes on the other side of the virtual board by releasing the force on the handle. The collected raw kinematic and kinetic data is transformed through a signal processing framework into 10 sensor-based digital biomarkers that provide information on different aspects of task performance, including for example movement smoothness, accuracy, and speed (21). These digital biomarkers and their clinimetric properties were previously validated in different neurological population (21, 22, 53). The data provide an objective, robust, and clinically feasible way to assess functionally relevant sensorimotor impairments in the arm and hand in chronic post-stroke individuals with mild to moderate sensorimotor deficits.
Figure 1. Technology-assisted assessments used in this study. Virtual Peg Insertion Test (VPIT, top), ETH MIKE (middle), and ZurichMove sensors (bottom).
The ETH MIKE is a one-degree-of-freedom end-effector device, that can be used to measure impairment of proprioception, motor and sensorimotor function in the metacarpophalangeal joint of the index finger (23, 54, 55). The ETH MIKE provides well-controlled stimuli to the index finger and sensitively measures the kinematic and kinetic responses, and has been shown to be safe and feasible in post-stroke individuals with mild to severe disability levels (23). Specifically, there is one task for proprioception assessment (gauge position matching (56)), with the absolute position matching error as the main outcome measure. Further, there are three tasks focused on motor impairments (active and passive range of motion, maximum force generation, and fast target reaching), providing as main outcomes active and passive range of motion, maximum finger force, and maximum movement speed for both flexion and extension. Additionally, one task is designed to evaluate the ability to integrate proprioceptive information during the execution of a complex movement, i.e., to assess sensorimotor impairments (trajectory following), providing the root means square tracking error as the main outcome measure. Thus, ETH MIKE is able to provide sensitive and objective measures of proprioception, motor impairments, and sensorimotor impairments that could inform the evolution of somatosensory and sensorimotor functions during recovery. Similar to the VPIT, the digital biomarkers used as outcome measures for the ETH MIKE were previously validated in different neurological population (23, 57, 58).
The ZurichMove sensors are custom-made wearable sensors developed by ETH Zurich (59) that allow unobtrusively monitoring the usage of the upper limb in the context of daily life activities.1 These sensors incorporate a 3-axis accelerometer, a 3-axis gyroscope and a 3-axis magnetometer. They can continuously record for approximately 3 days with a sampling frequency of 50 Hz. In this study, participants have to wear 5 ZurichMove sensors on both wrists, ankles and chest continuously for 48 h while performing all normal daily activities (in the clinic or at home for later time points). These sensors are attached to the body with non-gripping elastic bands by research staff or a caregiver and can be removed for showers and re-strapped by caregivers. Participants will record activities performed during the 48 h of wearing the sensors in a diary. Previously, the safety and usability of the wearable sensors have been established and the following outcome measures were defined and validated (60, 61). We aim to extract the duration of arms use in the clinical setting and during daily living by computing metrics such as upper limb activity counts as an approximation for the amount of limb usage (62), the gross movement score with an aim to identify functional relevant activities (60) and the GMAC (a fusion of both activity count and gross movement score) (63). The laterality ratio can be derived from these metrics to compare activity levels from both arms (64). These wearable sensors will provide digital biomarkers reflecting the usage of the more versus less affected upper limb in daily life.
We will rely on repeated standardized questionnaires and qualitative interviews conducted in English, Mandarin, and Malay, in order to gain knowledge about the perspectives of individuals with stroke at 6, 12, and 24 months post-stroke. We will not conduct interviews at 3 months post-stroke as some stroke survivors may have not returned to the community in the Singaporean healthcare system. We also will not conduct interviews at 36 months post-stroke as the post-stroke experience may not have significantly changed after 2 years since stroke onset. The main aim is to explore stroke survivors’ experiences in the community qualitatively and quantitatively. Questions related to qualitative interviews focus on participants’ perception related to identity, impairments and the rehabilitation journey (see Supplementary Material). Additionally, we will delve into their perspectives on mental health, spirituality and social support with the following questionnaires: Beck Depression Inventory (65), Generalized Anxiety Disorder Scale (66), Modified Fatigue Impact Scale (67), Insomnia Severity Index (68), Social Support Survey (69), Life Balance Inventory (70) and Mindful Attention Awareness Scale (71). With regards to sample size calculation, guidelines recommended for qualitative samples have been relatively small, ranging from 20–40, to account for the complexity of the analysis. Given the longitudinal nature, the qualitative component of the study will include 30 participants or fewer, if data saturation is reached earlier, with sufficient cognitive function and willingness to share experiences coping with stroke. Interviews will be recorded and transcribed verbatim followed by thematic analyses.
Data from standardized clinical assessments will be collected by TTSH therapists and will be stored on the National Healthcare Group (NHG) REDcap platform electronically. Technology-assisted and neurophysiological outcomes will be collected by TTSH therapists and/or researchers from the Future Health Technologies (FHT) Programme and will be stored on the FHT data infrastructure SECure. Qualitative interviews will be conducted by researchers from the Rehabilitation Research Institute of Singapore, Nanyang Technological University (NTU).
Participants who withdraw from the study will not be replaced. All collected data will be used for analysis. We will also maintain contact with participants between time points to minimize the number of dropouts. In case of missing data, we will consider using controlled multiple imputation (72) to handle the missing data. Controlled multiple imputation is based on the assumption of missing at random and can be used as sensitivity analysis to evaluate the impact of missing data. Missing data will first be imputed following the Bayesian paradigm under the assumption of ignorability (i.e., missing at random). Each imputed dataset will then be analyzed using the substantive analysis model of interest. Finally, Rubin’s rules (73) are applied to give a single multiple imputation estimate for inference.
Regarding the data sharing plan, pseudonymized data will be shared in an access-controlled manner between research teams on a biweekly basis. Only anonymized or aggregated data will be shared with the general public such as researchers from other institutes or government agencies upon reasonable request.
To understand post-stroke upper limb impairments and recovery, we will perform descriptive analyses for each outcome measure and various time points. Next, we will explore how different domains are interrelated and how the addition of digital biomarkers can inform key aspects of motor recovery and its dynamics using correlation matrices. We will investigate the relationship between movement quality (e.g., smoothness) and motor recovery, the relationship between somatosensory impairment and motor recovery, the relationship between upper limb use in activities of daily living and motor/somatosensory recovery, and whether these aspects present different time courses, or interact with each other.
To characterize upper limb recovery after stroke longitudinally, we will construct separate statistical models (e.g., mixed-effects models) that characterize the time course of upper limb impairments (FMA-UE) (3), activity capacity (ARAT) (4), activity performance (outcomes of wearable sensors), and quality of life (EQ-5D) post-stroke. We will then construct multivariate mixed models, leveraging on the uniqueness of the rich data set to probe the interaction and interdependence between various outcome measures and identify key determinants of recovery.
A mixture model based on a Bayesian approach (3), a clustering or subgroup identification analysis (74, 75) will then be used to identify subgroups of patients that exhibit distinct, clinically relevant recovery patterns based on a specific subset of variables identified in the above explorative analyses and their dynamics.
To characterize longitudinal post-stroke upper limb recovery in sensorimotor function, functional independence, and quality of life in an Asian population, we designed a study protocol to gather rich information via clinical, technology-assisted, and neurophysiological assessments together with qualitative interviews at multiple time points from within 1 month until up to 3 years post-stroke. Our multimodal data set promises to deepen the understanding of the prevalence and relevance of specific upper limb impairments and their recovery trajectories on individual and group levels in stroke survivors. Based on these insights, refined clinical decision-making and tailored therapy programs could be established.
The uniqueness of our study includes (i) the use of technology-assisted assessments with multimodal assessments including clinical, neurophysiological, and qualitative assessments to understand post-stroke upper limb recovery, (ii) a relatively large Asian stroke population, and (iii) longitudinal follow-up for over 3 years. Clinical assessments are limited in their sensitivity due to ceiling effects and the use of ordinal scales (76). Questionnaires used to capture the usage of the upper limb in daily life have limited reliability and suffer from recollection bias (58). Here, we supplement clinical assessments with already validated assessment technologies including robotic devices, namely VPIT and ETH MIKE, as well as wearable sensors. The promise of digital biomarkers is that this approach will enable healthcare practitioners to objectively quantify recovery with substantially higher resolution, better sensitivity, and over a much longer period than currently available methods (77–79), adding credence to assessing real-world upper limb use from the acute to chronic stages of recovery. Kinematic-based measures may provide unique insights to describe movement quality and how this relates to upper limb impairment, functional ability and ultimately arm use in daily life. Furthermore, wearable sensor-based assessments can be done in the real-world natural environment during everyday activities, both in the hospital or at home, since they capture what stroke survivors actually do (performance) and not only what they could do (capacity) or wish to do (intention), as is the case in clinical assessments (60, 80, 81). Such applications in quantifying recovery over time are needed as they hold the potential to derive new outcome metrics, potentially supporting clinical decision-making and evaluating the efficacy of rehabilitation training.
However, previous post-stroke upper limb recovery studies were predominantly conducted in Caucasian populations with IS (14, 16, 17, 82). Asian stroke survivors have been found to have varied profiles compared to Caucasian populations (19–21), such as a high incidence rate of ICH, lacunar stroke, and young age potentially related to the high prevalence of uncontrolled hypertension and diabetes mellitus (26, 27). Given the difference between the Asian and Caucasian profiles, it is important to fill this knowledge gap by establishing a multimodal data set for monitoring upper limb recovery in Asian stroke survivors.
Additionally, studies that followed upper limb motor recovery post-stroke have found that recovery occurs at the greatest rate during the first 3 to 6 weeks post-stroke (14, 17, 82, 83). Although functional gains are largest during the first 3 months post-stroke and thereafter the recovery curve starts to flatten (16, 17, 82), recovery still occurs till 12 to 18 months post-stroke with some individuals experiencing recovery up to 24 months post-stroke (14) and possibly beyond. It is therefore important to observe and fully understand sensorimotor recovery longitudinally post-stroke in order to optimize neurorehabilitation outcomes even at a later stage after stroke. For these reasons, our study aims to establish longitudinal follow-ups for up to 3 years post-stroke to document how profiles of residual functions evolve, and whether interactions with Hr-QOL and self-efficacy may improve over a longer time frame. As such, the collected data could deepen our understanding of post-stroke upper limb recovery and potentially help reveal major determinants of recovery.
Importantly, our multimodal data set comprises variables that are highly relevant to post-stroke upper limb recovery, such as somatosensory function, self-efficacy, qualitative self-reported measures, Hr-QOL, MEP, and post-stroke experience. The somatosensory function is known to be of high importance in achieving full motor recovery, as recent studies highlighted that stroke survivors who had full motor recovery did not exhibit any impaired somatosensory function (84, 85). It suggests that somatosensory function could be an essential factor that may contribute to refining the resolution of prediction models, especially when it comes to fine functional ability. Nevertheless, there are limited research studies investigating post-stroke upper limb somatosensory recovery, primarily due to a lack of suitable clinical scales to reliably assess these. Upper limb somatosensory recovery, similar to motor recovery was shown to significantly occur 3–4 months post-stroke at the group level, while recovery continued to occur till 6 months post-stroke at the individual level (84). To our knowledge, studies looking into upper limb somatosensory recovery did not follow beyond 6 months post-stroke, hence the long-term trajectory of somatosensory recovery remains mostly unknown (84, 86, 87). These findings show that underlying interactions between motor and somatosensory recovery after stroke warrant further investigation to develop a greater understanding and insight into upper limb somatosensory recovery and its possible interactions with other modalities after stroke. The technology-assisted assessments included in this study will help collect a first data set to more objectively characterize somatosensory function and recovery.
Besides examining upper limb motor and somatosensory recovery after stroke, it is also important to investigate how these physical changes and recovery influence self-efficacy in upper limb use, qualitative self-reported outcomes, and Hr-QOL after stroke. To date, there is still a dearth of information in this area. It has been reported that chronic stroke survivors expressed the loss of their upper limb function as an “enormous loss” (88). It can be seen that physical impairments may possibly give rise to other related problems in self-efficacy, qualitative self-reported measures, and Hr-QOL. Thus, it is important to longitudinally track these parameters beyond 1 year to understand how physical upper limb recovery impacts other aspects of stroke survivors’ lives. Complemented with qualitative interviews, information on post-stroke experiences such as coping strategies and difficulties in daily life could be obtained. It allows researchers to construct a holistic viewpoint of stroke consequences in stroke survivors and gain knowledge about the associations between functional recovery, daily activities, participation, environmental factors, and personal factors. Hence it is the aim of our study to establish a comprehensive database of not only physical recovery but also other factors such as self-efficacy, qualitative self-reported measures, and Hr-QOL to yield information on important factors influencing upper limb sensorimotor recovery trajectory across time. Nevertheless, gathering self-reported outcomes would require sufficient cognitive function from the responders and this might limit the generalizability of our findings.
Additionally, evaluating the integrity of the corticospinal tract with TMS has been used for predicting the upper limb recovery potential of stroke patients as formalized by the PREP2 algorithm (38). However, there has been some debate as to whether MEP measures improve prediction over and above the information provided by clinical tests (89–91), particularly, when the MEP status cannot be tested within the first 7 days post-stroke (37, 38). The current center’s acute stroke unit and rehabilitation unit are in separate physical locations with differential access to TMS machines, hence the predictive power of an early MEP status is limited (36). We will investigate whether MEP-negative stroke survivors have a bad prognosis for recovering upper limb function and whether this information sheds light on the recovery trajectory of individual patients with poor function early after stroke and whether obtaining MEP status would benefit clinical decision-making.
In conclusion, this study aims to develop and implement a technology-assisted digital biomarker (TAILOR) platform for describing upper limb sensorimotor recovery in Asian stroke survivors. Digital biomarkers could reduce measurement errors and improve the accuracy of outcome measures to complement clinical, neurophysiological, and qualitative assessments and could further offer therapeutic and prognostic value in stroke neurorehabilitation. With a gathered multimodal database, it is advantageous to characterize the pattern of upper limb recovery and to identify subgroups of stroke survivors that exhibit distinct, clinically relevant recovery patterns. This data set can consequently be used in the future to establish prediction models of upper limb recovery in stroke survivors receiving conventional rehabilitation training. This will aid clinical decision-making, therapeutic planning, and the development of tailored interventions.
The studies involving human participants were reviewed and approved by ETH Zurich’s Ethics Commission (EK 2021-N-181) in Switzerland and the National Healthcare Group’s Domain Specific Review Board (NHG DSRB Ref: 2021/00919) in Singapore. The participants will provide their written informed consent to participate in this study.
H-JC, LFC, and CMK were involved in the general study design and contribution to the whole manuscript draft. LFC, RL, CWKK, SK, SKW, EJ, TS, AS, PCG, EL, MZ-M, PL, and KC contributed to the design of the assessment protocol. RG, OL, and NW contributed to the study conception and design. All authors contributed to the article and approved the submitted version.
This research is supported by the National Research Foundation Singapore (NRF) under its Campus for Research Excellence and Technological Enterprise (CREATE) programme. Open access funding by ETH Zurich.
The research was conducted at the Future Health Technologies at the Singapore-ETH Centre, which was established collaboratively between ETH Zurich and the National Research Foundation Singapore.
The authors declare that the research was conducted in the absence of any commercial or financial relationships that could be construed as a potential conflict of interest.
All claims expressed in this article are solely those of the authors and do not necessarily represent those of their affiliated organizations, or those of the publisher, the editors and the reviewers. Any product that may be evaluated in this article, or claim that may be made by its manufacturer, is not guaranteed or endorsed by the publisher.
The Supplementary material for this article can be found online at: https://www.frontiersin.org/articles/10.3389/fneur.2023.1246888/full#supplementary-material
1. Persson, HC, Parziali, M, Danielsson, A, and Sunnerhagen, KS. Outcome and upper extremity function within 72 hours after first occasion of stroke in an unselected population at a stroke unit. A part of the SALGOT study. BMC Neurol. (2012) 12:162. doi: 10.1186/1471-2377-12-162
2. de Havenon, A, Heitsch, L, Sunmonu, A, Braun, R, Lohse, KR, Cole, JW, et al. Accurate prediction of persistent upper extremity impairment in patients with ischemic stroke. Arch Phys Med Rehabil. (2022) 103:964–9. doi: 10.1016/j.apmr.2021.10.023
3. van der Vliet, R, Selles, RW, Andrinopoulou, ER, Nijland, R, Ribbers, GM, Frens, MA, et al. Predicting upper limb motor impairment recovery after stroke: a mixture model. Ann Neurol. (2020) 87:383–93. doi: 10.1002/ana.25679
4. Selles, RW, Andrinopoulou, E-R, Nijland, RH, van der Vliet, R, Slaman, J, van Wegen, EEH, et al. Computerised patient-specific prediction of the recovery profile of upper limb capacity within stroke services: the next step. J Neurol Neurosurg Psychiatry. (2021) 92:574–81. doi: 10.1136/jnnp-2020-324637
5. Nichols-Larsen, DS, Clark, PC, Zeringue, A, Greenspan, A, and Blanton, S. Factors influencing stroke survivors’ quality of life during subacute recovery. Stroke. (2005) 36:1480–4. doi: 10.1161/01.STR.0000170706.13595.4f
6. Morris, JH, van Wijck, F, Joice, S, and Donaghy, M. Predicting health related quality of life 6 months after stroke: the role of anxiety and upper limb dysfunction. Disabil Rehabil. (2013) 35:291–9. doi: 10.3109/09638288.2012.691942
7. Szczepańska-Gieracha, J, and Mazurek, J. The role of self-efficacy in the recovery process of stroke survivors. Psychol Res Behav Manag. (2020) 13:897–906. doi: 10.2147/PRBM.S273009
8. Wong, HJ, Lua, PL, Harith, S, and Ibrahim, KA. Health-related quality of life profiles and their dimension-specific associated factors among Malaysian stroke survivors: a cross sectional study. Health Qual Life Outcomes. (2021) 19:210. doi: 10.1186/s12955-021-01847-0
9. Ramos-Lima, MJM, Brasileiro, IC, Lima, TL, and Braga-Neto, P. Quality of life after stroke: impact of clinical and sociodemographic factors. Clinics. (2018) 73:e418. doi: 10.6061/clinics/2017/e418
10. Birke, G, Wolf, S, Ingwersen, T, Bartling, C, Bender, G, Meyer, A, et al. Protocol for a multicenter observational prospective study of functional recovery from stroke beyond inpatient rehabilitation—The Interdisciplinary Platform for Rehabilitation Research and Innovative Care of Stroke Patients (IMPROVE). Neurol Res Pract. (2020) 2:10. doi: 10.1186/s42466-020-00056-2
11. Fleury, L, Koch, PJ, Wessel, MJ, Bonvin, C, San Millan, D, Constantin, C, et al. Toward individualized medicine in stroke—the TiMeS project: protocol of longitudinal, multi-modal, multi-domain study in stroke. Front Neurol. (2022) 13:13. doi: 10.3389/fneur.2022.939640
12. Hayward, KS, Kramer, SF, Thijs, V, Ratcliffe, J, Ward, NS, Churilov, L, et al. A systematic review protocol of timing, efficacy and cost effectiveness of upper limb therapy for motor recovery post-stroke. Syst Rev. (2019) 8:187. doi: 10.1186/s13643-019-1093-6
13. Ingwersen, T, Wolf, S, Birke, G, Schlemm, E, Bartling, C, Bender, G, et al. Long-term recovery of upper limb motor function and self-reported health: results from a multicenter observational study 1 year after discharge from rehabilitation. Neurol Res Pract. (2021) 3:66. doi: 10.1186/s42466-021-00164-7
14. Borschmann, K, and Hayward, K. Recovery of upper limb function is greatest early after stroke but does continue to improve during the chronic phase: a two-year, observational study. Physiotherapy. (2020) 107:216–23. doi: 10.1016/j.physio.2019.10.001
15. Ballester, BR, Maier, M, Duff, A, Cameirão, M, Bermúdez, S, Duarte, E, et al. A critical time window for recovery extends beyond one-year post-stroke. J Neurophysiol. (2019) 122:350–7. doi: 10.1152/jn.00762.2018
16. van Kuijk, AA, Pasman, JW, Hendricks, HT, Zwarts, MJ, and Geurts, AC. Predicting hand motor recovery in severe stroke: the role of motor evoked potentials in relation to early clinical assessment. Neurorehabil Neural Repair. (2009) 23:45–51. doi: 10.1177/1545968308317578
17. Duncan, PW, Goldstein, LB, Horner, RD, Landsman, PB, Samsa, GP, and Matchar, DB. Similar motor recovery of upper and lower extremities after stroke. Stroke. (1994) 25:1181–8. doi: 10.1161/01.STR.25.6.1181
18. FDA-NIH Biomarker Working Group. BEST (Biomarkers, Endpoints, and other Tools) Resource. Maryland: Silver Spring (2016).
19. U.S. Food and Drug Administration. Patient-focused drug development: collecting comprehensive and representative input. Guidance for industry, Food and Drug Administration staff, and other stakeholders. Maryland, United Sates: Center for Drug Evaluation and Research (2020).
20. Vasudevan, S, Saha, A, Tarver, ME, and Patel, B. Digital biomarkers: convergence of digital health technologies and biomarkers. NPJ Digit Med. (2022) 5:36. doi: 10.1038/s41746-022-00583-z
21. Kanzler, CM, Rinderknecht, MD, Schwarz, A, Lamers, I, Gagnon, C, Held, JPO, et al. A data-driven framework for selecting and validating digital health metrics: use-case in neurological sensorimotor impairments. NPJ Digit Med. (2020) 3:80. doi: 10.1038/s41746-020-0286-7
22. Kanzler, CM, Schwarz, A, Held, JPO, Luft, AR, Gassert, R, and Lambercy, O. Technology-aided assessment of functionally relevant sensorimotor impairments in arm and hand of post-stroke individuals. J Neuroeng Rehabil. (2020) 17:128. doi: 10.1186/s12984-020-00748-5
23. Zbytniewska, M, Kanzler, CM, Jordan, L, Salzmann, C, Liepert, J, Lambercy, O, et al. Reliable and valid robot-assisted assessments of hand proprioceptive, motor and sensorimotor impairments after stroke. J Neuroeng Rehabil. (2021) 18:115. doi: 10.1186/s12984-021-00904-5
24. Schwarz, A, Kanzler, CM, Lambercy, O, Luft, AR, and Veerbeek, JM. Systematic review on kinematic assessments of upper limb movements after stroke. Stroke. (2019) 50:718–27. doi: 10.1161/STROKEAHA.118.023531
25. Wang, X, Fu, Y, Ye, B, Babineau, J, Ding, Y, and Mihailidis, A. Technology-based compensation assessment and detection of upper extremity activities of stroke survivors: systematic review. J Med Internet Res. (2022) 24:e34307. doi: 10.2196/34307
26. Ng, WK, Goh, KJ, George, J, Tan, C, Biard, A, and Donnan, GA. A comparative study of stroke subtypes between Asians and Caucasians in two hospital-based stroke registries. Neurol J Southeast Asia. (1998) 3:19–26.
28. Ratha Krishnan, R, Yeo, EQY, Lim, CJ, and Chua, KSG. The impact of stroke subtype on recovery and functional outcome after inpatient rehabilitation: a retrospective analysis of factors. Life. (2022) 12:1295. doi: 10.3390/life12091295
29. Moon, KGM, Altman, DG, Reitsma, JB, Ioannidis, JPA, Macaskill, P, Steyerberg, EW, et al. Transparent reporting of a multivariable prediction model for individual prognosis or diagnosis (TRIPOD): explanation and elaboration. Ann Intern Med. (2015) 162:W1–W73. doi: 10.7326/M14-0698
30. Brott, T, Adams, HP Jr, Olinger, CP, Marler, JR, Barsan, WG, Biller, J, et al. Measurements of acute cerebral infarction: a clinical examination scale. Stroke. (1989) 20:864–70. doi: 10.1161/01.STR.20.7.864
31. Teasdale, G, and Jennett, B. Assessment of coma and impaired consciousness: a practical scale. Lancet. (1974) 304:81–4. doi: 10.1016/S0140-6736(74)91639-0
32. UK-TIA Study Group. United Kingdom transient ischaemic attack (UK-TIA) aspirin trial: interim results. Br Med J. (1988) 296:316–20. doi: 10.1136/bmj.296.6618.316
33. Duncan Millar, J, Van Wijck, F, Pollock, A, and Ali, M. International consensus recommendations for outcome measurement in post-stroke arm rehabilitation trials. Eur J Phys Rehabil Med. (2021) 57:61–8. doi: 10.23736/S1973-9087.20.06575-2
34. Kwakkel, G, Lannin, NA, Borschmann, K, English, C, Ali, M, Churilov, L, et al. Standardized measurement of sensorimotor recovery in stroke trials: consensus-based core recommendations from the stroke recovery and rehabilitation roundtable. Int J Stroke. (2017) 12:451–61. doi: 10.1177/1747493017711813
35. Fugl-Meyer, AR, Jääskö, L, Leyman, I, Olsson, S, and Steglind, S. The post-stroke hemiplegic patient. I. A method for evaluation of physical performance. Scand J Rehabil Med. (1975) 7:13–31. doi: 10.2340/1650197771331
36. Lundquist, C, Nielsen, J, Arguissain, F, and Brunner, I. Accuracy of the upper limb prediction algorithm PREP2 applied 2 weeks poststroke: a prospective longitudinal study. Neurorehabil Neural Repair. (2021) 35:68–78. doi: 10.1177/1545968320971763
37. Stinear, CM, Barber, PA, Petoe, M, Anwar, S, and Byblow, WD. The PREP algorithm predicts potential for upper limb recovery after stroke. Brain. (2012) 135:2527–35. doi: 10.1093/brain/aws146
38. Stinear, CM, Byblow, WD, Ackerley, SJ, Smith, MC, Borges, VM, and Barber, PA. PREP2: a biomarker-based algorithm for predicting upper limb function after stroke. Ann Clin Transl Neurol. (2017) 4:811–20. doi: 10.1002/acn3.488
39. Lyle, RC. A performance test for assessment of upper limb function in physical rehabilitation treatment and research. Int J Rehabil Res. (1981) 4:483–92. doi: 10.1097/00004356-198112000-00001
40. Lincoln, NB, Jackson, JM, and Adams, SA. Reliability and revision of the Nottingham sensory assessment for stroke patients. Physiotherapy. (1998) 84:358–65. doi: 10.1016/S0031-9406(05)61454-X
41. Bohannon, RW, and Smith, MB. Interrater reliability of a modified Ashworth scale of muscle spasticity. Phys Ther. (1987) 67:206–7. doi: 10.1093/ptj/67.2.206
42. Hayes, MHSP, and Patterson, DG. Experimental development of the graphic rating method. Psychol Bull. (1921) 18:98–9.
43. Abdullahi, A. Upper limb self-efficacy test (UPSET): a measure of confidence in the use of the upper limb after stroke. Adv Med Sci. (2016) 1:10–8. doi: 10.5281/zenodo.1308452
44. Gauthier, L, Dehaut, F, and Joanette, Y. The Bells test: a quantitative and qualitative test for visual neglect. Int J Clin Neuropsychol. (1989) 11:49–54.
45. Verheyden, G, Nieuwboer, A, Mertin, J, Preger, R, Kiekens, C, and De Weerdt, W. The trunk impairment scale: a new tool to measure motor impairment of the trunk after stroke. Clin Rehabil. (2004) 18:326–34. doi: 10.1191/0269215504cr733oa
46. Keith, RA, Granger, CV, Hamilton, BB, and Sherwin, FS. The functional independence measure: a new tool for rehabilitation. Adv Clin Rehabil. (1987) 1:6–18.
47. Nasreddine, ZS, Phillips, NA, Bédirian, V, Charbonneau, S, Whitehead, V, Collin, I, et al. The Montreal Cognitive Assessment, MoCA: a brief screening tool for mild cognitive impairment. J Am Geriatr Soc. (2005) 53:695–9. doi: 10.1111/j.1532-5415.2005.53221.x
48. Rabin, R, and de Charro, F. EQ-SD: a measure of health status from the EuroQol Group. Ann Med. (2001) 33:337–43. doi: 10.3109/07853890109002087
49. Williams, LS, Weinberger, M, Harris, LE, Clark, DO, and Biller, J. Development of a stroke-specific quality of life scale. Stroke. (1999) 30:1362–9. doi: 10.1161/01.STR.30.7.1362
50. Lefaucheur, J-P. Chapter 37-transcranial magnetic stimulation In: KH Levin and P Chauvel, editors. Handbook of clinical neurology. Netherlands: Elsevier (2019). 559–80.
51. Fluet, MC, Lambercy, O, and Gassert, R, Upper limb assessment using a Virtual Peg Insertion Test. IEEE International Conference on Rehabilitation Robotics; (2011). 29 June–1 July 2011
52. Kanzler, CM, Averta, G, Schwarz, A, Held, JPO, Gassert, R, Bicchi, A, et al. A low-dimensional representation of arm movements and hand grip forces in post-stroke individuals. Sci Rep. (2022) 12:7601. doi: 10.1038/s41598-022-11806-4
53. Kanzler, CM, Lessard, I, Gassert, R, Brais, B, Gagnon, C, and Lambercy, O. Reliability and validity of digital health metrics for assessing arm and hand impairments in an ataxic disorder. Ann Clin Transl Neurol. (2022) 9:432–43. doi: 10.1002/acn3.51493
54. Zbytniewska, M, Rinderknecht, MD, Lambercy, O, Barnobi, M, Raats, J, Lamers, I, et al., Design and characterization of a robotic device for the assessment of hand proprioceptive, motor, and sensorimotor impairments IEEE 16th International Conference on Rehabilitation Robotics (ICORR) (2019): 441–6.
55. Zbytniewska, M, Siegenthaler, M, Kanzler, CM, Hermsdörfer, J, Gassert, R, and Lambercy, O Design and preliminary evaluation of a perturbation-based robot-assisted assessment of hand sensorimotor impairments. IEEE RAS/EMBS International Conference for Biomedical Robotics and Biomechatronics (BioRob); (2020). 29 November–1 December 2020.
56. Rinderknecht, MD, Popp, WL, Lambercy, O, and Gassert, R. Reliable and rapid robotic assessment of wrist proprioception using a gauge position matching paradigm. Front Hum Neurosci. (2016) 10:316. doi: 10.3389/fnhum.2016.00316
57. Zbytniewska-Mégret, M, Kanzler, CM, Raats, J, Yilmazer, C, Feys, P, Gassert, R, et al. Reliability, validity and clinical usability of a robotic assessment of finger proprioception in persons with multiple sclerosis. Mult Scler Relat Disord. (2023) 70:104521. doi: 10.1016/j.msard.2023.104521
58. Zbytniewska-Mégret, M, Decraene, L, Mailleux, L, Kleeren, L, Kanzler, CM, Gassert, R, et al. Reliable and valid robotic assessments of hand active and passive position sense in children with unilateral cerebral palsy. Front Hum Neurosci. (2022) 16:895080. doi: 10.3389/fnhum.2022.895080
59. Leuenberger, K, and Gassert, R. Low-power sensor module for long-term activity monitoring. Annual International Conference of the IEEE Engineering in Medicine and Biology Society (2011): 2237–2241.
60. Leuenberger, K, Gonzenbach, R, Wachter, S, Luft, A, and Gassert, R. A method to qualitatively assess arm use in stroke survivors in the home environment. Med Biol Eng Comput. (2017) 55:141–50. doi: 10.1007/s11517-016-1496-7
61. Schneider, S, Popp, WL, Brogioli, M, Albisser, U, Ortmann, S, Velstra, IM, et al. Predicting upper limb compensation during prehension tasks in tetraplegic spinal cord injured patients using a single wearable sensor. IEEE 16th International Conference on Rehabilitation Robotics (ICORR) (2019): 1000–6.
62. Brønd, JC, Andersen, LB, and Arvidsson, D. Generating ActiGraph counts from raw acceleration recorded by an alternative monitor. Med Sci Sports. (2017) 49:2351–60. doi: 10.1249/MSS.0000000000001344
63. Subash, T, David, A, Reeta Janet Surekha, S, Gayathri, S, Samuelkamaleshkumar, S, Magimairaj, HP, et al. Comparing algorithms for assessing upper limb use with inertial measurement units. Front Physiol. (2022) 13:13. doi: 10.3389/fphys.2022.1023589
64. Uswatte, G, Giuliani, C, Winstein, C, Zeringue, A, Hobbs, L, and Wolf, SL. Validity of accelerometry for monitoring real-world arm activity in patients with subacute stroke: evidence from the extremity constraint-induced therapy evaluation trial. Arch Phys Med Rehabil. (2006) 87:1340–5. doi: 10.1016/j.apmr.2006.06.006
65. Beck, AT, Ward, CH, Mendelson, M, Mock, J, and Erbaugh, J. An inventory for measuring depression. Arch Gen Psychiatry. (1961) 4:561–71. doi: 10.1001/archpsyc.1961.01710120031004
66. Spitzer, RL, Kroenke, K, Williams, JBW, and Löwe, B. A brief measure for assessing generalized anxiety disorder: the GAD-7. Arch Intern Med. (2006) 166:1092–7. doi: 10.1001/archinte.166.10.1092
67. Fisk, JD, Ritvo, PG, Ross, L, Haase, DA, Marrie, TJ, and Schlech, WF. Measuring the functional impact of fatigue: initial validation of the fatigue impact scale. Clin Infect Dis. (1994) 18:S79–83. doi: 10.1093/clinids/18.Supplement_1.S79
69. Sherbourne, CD, and Stewart, AL. The MOS social support survey. Soc Sci Med. (1991) 32:705–14. doi: 10.1016/0277-9536(91)90150-B
70. Matuska, K. Description and development of the life balance inventory. Occup Ther J Res. (2012) 32:220–8. doi: 10.3928/15394492-20110610-01
71. Brown, KW, and Ryan, RM. The benefits of being present: mindfulness and its role in psychological well-being. J Pers Soc Psychol. (2003) 84:822–48. doi: 10.1037/0022-3514.84.4.822
72. Cro, S, Morris, TP, Kenward, MG, and Carpenter, JR. Sensitivity analysis for clinical trials with missing continuous outcome data using controlled multiple imputation: a practical guide. Stat Med. (2020) 39:2815–42. doi: 10.1002/sim.8569
73. Rubin, DB. Multiple imputation for nonresponse in surveys. New York, NY: John Wiley & Sons, Inc. (1987).
74. Loh, W-Y, Cao, L, and Zhou, P. Subgroup identification for precision medicine: a comparative review of 13 methods. Wiley Interdiscip Rev Data Min Knowl Discov. (2019) 9:e 1326. doi: 10.1002/widm.1326
75. Shen, J, and Qu, A. Subgroup analysis based on structured mixed-effects models for longitudinal data. J Biopharm Stat. (2020) 30:607–22. doi: 10.1080/10543406.2020.1730867
76. Kanzler, CM, Sylvester, R, Gassert, R, Kool, J, Lambercy, O, and Gonzenbach, R. Goal-directed upper limb movement patterns and hand grip forces in multiple sclerosis. Mult Scler J Exp Transl Clin. (2022) 8:20552173221116272. doi: 10.1177/20552173221116272
77. Puleo, V, Gentili, A, Failla, G, Melnyk, A, Di Tanna, G, Ricciardi, W, et al. Digital health technologies: a systematic review of their cost-effectiveness. Eur J Pub Health. (2021) 31:ckab164.273. doi: 10.1093/eurpub/ckab164.273
78. Lambercy, O, Lehner, R, Chua, K, Wee, SK, Rajeswaran, DK, Kuah, CWK, et al. Neurorehabilitation from a distance: can intelligent technology support decentralized access to quality therapy? Front Robot AI. (2021) 8:8. doi: 10.3389/frobt.2021.612415
79. Steinhubl, SR, Wolff-Hughes, DL, Nilsen, W, Iturriaga, E, and Califf, RM. Digital clinical trials: creating a vision for the future. NPJ Digit Med. (2019) 2:126. doi: 10.1038/s41746-019-0203-0
80. DeJong, SL, Birkenmeier, RL, and Lang, CE. Person-specific changes in motor performance accompany upper extremity functional gains after stroke. J Appl Biomech. (2012) 28:304–16. doi: 10.1123/jab.28.3.304
81. Lang, CE, Waddell, KJ, Klaesner, JW, and Bland, MD. A method for quantifying upper limb performance in daily life using accelerometers. J Vis Exp. (2017) 122:e55673. doi: 10.3791/55673-v
82. Verheyden, G, Nieuwboer, A, De Wit, L, Thijs, V, Dobbelaere, J, Devos, H, et al. Time course of trunk, arm, leg, and functional recovery after ischemic stroke. Neurorehabil Neural Repair. (2008) 22:173–9. doi: 10.1177/1545968307305456
83. Au-Yeung, SS, and Hui-Chan, CW. Predicting recovery of dextrous hand function in acute stroke. Disabil Rehabil. (2009) 31:394–401. doi: 10.1080/09638280802061878
84. Zandvliet, SB, Kwakkel, G, Nijland, RHM, van Wegen, EEH, and Meskers, CGM. Is recovery of somatosensory impairment conditional for upper-limb motor recovery early after stroke? Neurorehabil Neural Repair. (2020) 34:403–16. doi: 10.1177/1545968320907075
85. Zbytniewska-Mégret, M, Salzmann, C, Kanzler, CM, Hassa, T, Gassert, R, Lambercy, O, et al. The evolution of hand proprioceptive and motor impairments in the sub-acute phase after stroke. Neurorehabil Neural Repair. (2023). doi: 10.1177/15459683231207355
86. Connell, LA, Lincoln, NB, and Radford, KA. Somatosensory impairment after stroke: frequency of different deficits and their recovery. Clin Rehabil. (2008) 22:758–67. doi: 10.1177/0269215508090674
87. Meyer, S, De Bruyn, N, Lafosse, C, Van Dijk, M, Michielsen, M, Thijs, L, et al. Somatosensory impairments in the upper limb poststroke: distribution and association with motor function and visuospatial neglect. Neurorehabil Neural Repair. (2016) 30:731–42. doi: 10.1177/1545968315624779
88. Barker, RN, and Brauer, SG. Upper limb recovery after stroke: the stroke survivors’ perspective. Disabil Rehabil. (2005) 27:1213–23. doi: 10.1080/09638280500075717
89. Bakker, CD, Massa, M, Daffertshofer, A, Pasman, JW, van Kuijk, AA, Kwakkel, G, et al. The addition of the MEP amplitude of finger extension muscles to clinical predictors of hand function after stroke: a prospective cohort study. Restor Neurol Neurosci. (2019) 37:445–56. doi: 10.3233/RNN-180890
90. Rosso, C, and Lamy, JC. Does resting motor threshold predict motor hand recovery after stroke? Front Neurol. (2018) 9:1020. doi: 10.3389/fneur.2018.01020
91. Hoonhorst, MHJ, Nijland, RHM, van den Berg, PJS, Emmelot, CH, Kollen, BJ, and Kwakkel, G. Does transcranial magnetic stimulation have an added value to clinical assessment in predicting upper-limb function very early after severe stroke? Neurorehabil Neural Repair. (2018) 32:682–90. doi: 10.1177/1545968318785044
Keywords: stroke, neurorehabilitation, upper limb, assessment, recovery, sensorimotor impairments
Citation: Cheng H-J, Chin LF, Kanzler CM, Lehner R, Kuah CWK, Kager S, Josse E, Samkharadze T, Sidarta A, Gonzalez PC, Lie E, Zbytniewska-Mégret M, Wee SK, Liang P, Gassert R, Chua K, Lambercy O and Wenderoth N (2023) Upper limb sensorimotor recovery in Asian stroke survivors: a study protocol for the development and implementation of a Technology-Assisted dIgitaL biOmaRker (TAILOR) platform. Front. Neurol. 14:1246888. doi: 10.3389/fneur.2023.1246888
Received: 24 June 2023; Accepted: 06 November 2023;
Published: 30 November 2023.
Edited by:
Pavel Lindberg, INSERM U1266 Institut de Psychiatrie et Neurosciences de Paris, FranceReviewed by:
Margit Alt Murphy, University of Gothenburg, SwedenCopyright © 2023 Cheng, Chin, Kanzler, Lehner, Kuah, Kager, Josse, Samkharadze, Sidarta, Gonzalez, Lie, Zbytniewska-Mégret, Wee, Liang, Gassert, Chua, Lambercy and Wenderoth. This is an open-access article distributed under the terms of the Creative Commons Attribution License (CC BY). The use, distribution or reproduction in other forums is permitted, provided the original author(s) and the copyright owner(s) are credited and that the original publication in this journal is cited, in accordance with accepted academic practice. No use, distribution or reproduction is permitted which does not comply with these terms.
*Correspondence: Nicole Wenderoth, bmljb2xlLndlbmRlcm90aEBoZXN0LmV0aHouY2g=
†These authors have contributed equally to this work and share first authorship
‡These authors have contributed equally to this work and share senior authorship
Disclaimer: All claims expressed in this article are solely those of the authors and do not necessarily represent those of their affiliated organizations, or those of the publisher, the editors and the reviewers. Any product that may be evaluated in this article or claim that may be made by its manufacturer is not guaranteed or endorsed by the publisher.
Research integrity at Frontiers
Learn more about the work of our research integrity team to safeguard the quality of each article we publish.