- 1Department of Neurology, The First Affiliated Hospital, Guangxi Medical University, Nanning, Guangxi Zhuang Autonomous Region, China
- 2Collaborative Innovation Centre of Regenerative Medicine and Medical BioResource Development and Application Co-constructed by the Province and Ministry, Guangxi Medical University, Nanning, Guangxi Zhuang Autonomous Region, China
- 3Guangxi Key Laboratory of Regenerative Medicine and Guangxi Collaborative Innovation Center for Biomedicine, Guangxi Medical University, Nanning, Guangxi Zhuang Autonomous Region, China
Introduction: Acute ischemic stroke (AIS) and lung adenocarcinoma (LUAD) are associated with some of the highest morbidity and mortality rates worldwide. Despite reports on their strong correlation, the causal relationship is not fully understood. The study aimed to identify and annotate the biological functions of hub genes with clinical diagnostic efficacy in AIS and LUAD.
Methods: Transcriptome and single-cell datasets were obtained from the Gene Expression Omnibus (GEO) and The Cancer Genome Atlas (TCGA). We identified the differentially expressed genes (DEGs) upregulated in AIS and LUAD and found 372 genes intersecting both datasets. Hub genes were identified using protein-protein interaction (PPI) networks, and the diagnostic and prognostic utility of these hub genes was then investigated using receiver operating characteristic (ROC) curves, survival analysis, and univariable Cox proportional hazard regression. Single-cell analysis was used to detect whether the hub genes were expressed in tumor epithelial cells. The immune microenvironment of AIS and LUAD was assessed using the CIBERSORT algorithm. The protein expression of these hub genes was tracked using the Human Protein Atlas (HPA). We calculated the number of positive cells using the digital pathology software QuPath. Finally, we performed molecular docking after using the Enrichr database to predict possible medicines.
Results: We identified the molecular mechanisms underlying hub genes in AIS and LUAD and found that CCNA2, CCNB1, CDKN2A, and CDK1 were highly expressed in AIS and LUAD tissue samples compared to controls. The hub genes were mainly involved in the following pathways: the cell cycle, cellular senescence, and the HIF-1 signaling pathway. Using immunohistochemical slices from the HPA database, we confirmed that these hub genes have a high diagnostic capability for AIS and LUAD. Further, their high expression is associated with poor prognosis. Finally, curcumin was tested as a potential medication using molecular docking modeling.
Discussion: Our findings suggest that the hub genes we found in this study contribute to the development and progression of AIS and LUAD by altering the cellular senescence pathway. Thus, they may be promising markers for diagnosis and prognosis.
1. Introduction
Human health is in peril from conditions like cancer and stroke (1). Over 795,000 acute ischemic stroke (AIS) occurrences are reported each year in the United States alone, making it the second most frequent cause of mortality and long-term disability worldwide (2). A study found that in 2020, the incidence and mortality rates of stroke in China were 505.2 and 343.4 per 100,000 person-years, respectively (3). The proportions of acute ischemic stroke due to atherosclerosis and cardiogenic embolism were 39% and 22%, respectively (4). AIS is a multifactorial disease influenced by atherosclerosis, hypertension, alcohol consumption, smoking, and D-dimer levels. In many low-income and middle-income nations, stroke incidence and fatality rates have increased in recent decades (5). Genetic variables, which are essential for determining the etiology of AIS, are suggested by epidemiological research to be connected to the prevalence of stroke (6).
One of the molecular mechanisms of AIS includes immune activation, which can lead to both neuroprotective and neurotoxic effects (7, 8). Thrombolysis and antiplatelet therapy are currently the main treatment methods for stroke, but it is unclear whether these treatments improve prognosis in patients (9). Lung cancer is the leading cause of cancer-related deaths globally. Additionally, lung cancer has a terrible prognosis (10, 11). The most common histological subtype of malignant lung cancer is lung adenocarcinoma (LUAD). Deaths are mostly attributed to cancer cell invasion and distant metastasis (12). Gene abnormalities are the initial factors that influence the biological behavioral changes of heterogenic cancer cells, including cell signaling and the immune microenvironment. According to reports, several genes play a role in the development of LUAD tumors (13–15). During tumor progression, changes in genes that mediate important biological processes can occur, resulting in the rapid proliferation of malignant cells. However, the mechanism by which this process occurs is still unclear (16). As a result of studies on the molecular basis of lung cancer, programmed death ligand 1 and epidermal growth factor receptor tyrosine kinase inhibitors are two newly emerging treatment targets. Even though lung cancer treatment choices have increased thanks to genetic testing, novel targeted therapy, and immunotherapy, its 5-year survival rate is as low as 20% in many nations (10, 17), and the recurrence rate remains high (18). Recently, an increasing number of reports have documented AIS caused by malignant tumors (19) and demonstrated that patients with lung cancer and metastatic cancer display a higher risk of stroke. Notably, the cumulative incidence of stroke among lung cancer patients was 5.1% (20). The connection between lung cancer and stroke significantly decreased the patients' life longevity and quality (19). LUAD may lead to multiple acute cerebral infarction lesions involving multiple arterial blood supply areas (21). CCNB2 may be a possible target against lung cancer and AIS, according to earlier research (22). Moreover, the stroke is the second most frequent neurological condition associated with death in cancer patients. Risk factors for cancer-related AIS include high levels of D-dimer, fibrinogen breakdown products, and C-reactive protein (23). LUAD and AIS are closely associated with the occurrence of gene abnormalities. However, the regulatory mechanisms of the genes involved have not yet been elucidated. Therefore, it is crucial to comprehend how they interact and find relevant indicators to provide a plan for diagnosis and treatment. In this study, we used bioinformatics to identify hub genes in AIS and LUAD, investigated their biological functions, and determined their clinical significance.
2. Materials and methods
2.1. Data sources
Using the expression data of AIS in the GEO database, we discovered the expression data and clinical details about LUAD on the Xena platform. The inclusion criteria were as follows: (i) case and control groups, (ii) microarray data of mRNA, (iii) the specimen was a peripheral blood sample or tissue, and (iv) Homo sapiens. The GSE122709 dataset was included according to the above criteria, including five control peripheral blood samples and 10 AIS peripheral blood samples. The Cancer Genome Atlas (TCGA)-LUAD array from the Xena platform was selected, which included 59 surrounding normal samples and 524 tumor samples. Furthermore, we also downloaded LUAD validation datasets GSE42127, GSE68465, GSE50081, GSE13231, and GSE31210. In addition, we downloaded the validation datasets of AIS (GSE58294 and GSE140275) from GEO. All of these datasets met the above inclusion criteria. Datasets obtained were subjected to logarithmic-scale conversion and other data processing. We downloaded the single-cell dataset GSE146100 and then did quality assurance and data filtering.
2.2. Differential gene expression analysis
We performed a difference analysis using the R package “limma”. Differential genes were defined as those with a P-value < 0.05 and |log2FC (fold change)| ≥ 1 values. The number of 372 differentially expressed genes (DEGs) was found after using a Venn diagram to find the intersecting genes.
2.3. Functional analysis
We examined the Gene Ontology (GO) and Kyoto Encyclopedia of Genes and Genomes (KEGG) of the elevated DEGs using the R package “clusterProfiler.” P-values < 0.05 were used to determine whether an enrichment function was significant. Cellular components, molecular functions, and biological processes are all included in the GO category. The top 10 GO biological processes were chosen in ascending order of P-values. Ten significant KEGG in total were found. The increased DEGs were annotated using the disease ontology (DO) analysis R package “DOSE”. Gene set enrichment analysis (GSEA) was used to define hub genes to pinpoint the signaling pathways connected to AIS and LUAD. Patients were split into two groups (control and case group) based on the levels of expression of the four hub genes. GSEA analysis reveals differences in signaling pathways. The gene sets with a significant enrichment were then sorted. GSEA was used to investigate the relationship between illness categories and biological processes. The cutoff was established at P < 0.05.
2.4. Building a protein-protein interaction (PPI) network and choosing hub genes
We chose DEGs from the KEGG pathway for cellular senescence for the PPI network. Using the STRING internet database (http://stringdb.org), we looked for connections between proteins. We constructed a PPI network for genes with a rating scale >0.4 using Cytoscape.
2.5. Immune infiltration analysis
CIBRSORT was used to estimate the proportion of immune cells in the AIS gene expression matrix. We use the R package “ggplot2” to draw the boxplots. Statistics were deemed significant at P < 0.05.
2.6. Receiver operating characteristic (ROC) analysis
We investigated the mRNA expression levels of the hub genes in patients with AIS and LUAD using ROC curves and boxplots. To create the ROC curves, we utilized the R package “pROC”.
2.7. Survival analysis
TCGA-LUAD expression data and clinical data were used to conduct a survival analysis using the R packages “survminer” and “survival”. The prognosis of LUAD patients was examined using Kaplan-Meier (KM) analysis. The time-dependent ROC curve (timeROC) method was applied to assess the hub genes prediction accuracy at 1, 3, and 5 years.
2.8. Analysis of single-cell data and identification of cell subpopulations
We downloaded the scRNA-seq dataset GSE146100 of LUAD. We used the TISCH database (http://tisch.comp-genomics.org) and Maestro analysis for quality control, clustering and cell type annotation, making the expression of four hub genes in the epithelial cell type comparable.
2.9. Protein expression and validation
Information about the distribution of proteins in human organs and cells can be found in the HPA database (https://www.proteinatlas.org). We acquired immunohistochemistry (IHC) pictures of LUAD tissues and investigated the differential protein expression of hub genes in matched HPA normal tissues. QuPath, a program for digital pathology, was utilized to count the positive cells. We analyzed The Clinical Proteomic Tumor Analysis Consortium (CPTAC) dataset using the UALCAN tool (https://ualcan.path.uab.edu). The protein levels of the four hub genes were compared between LUAD and normal tissues.
2.10. Drug prediction and molecular docking
We used the DSigDB dataset in Enrichr (http://amp.pharm.mssm.edu/Enrichr) for drug prediction of hub genes. Structural information of curcumin was downloaded from the pubChem compound database (https://pubchem.ncbi.nlm.nih.gov). The 3D structures of the proteins of the four hub genes were downloaded using the PDB database (https://www.rcsb.org). “Dockeasy” (https://www.dockeasy.cn/) was used to achieve molecular docking.
2.11. Statistical analysis
All data were analyzed using R version 4.2.1. Statistics were deemed significant at P < 0.05.
3. Results
3.1. Differently expressed genes (DEGs) in AIS and LUAD
The flowchart of our study is as follows (Figure 1). The GEO database provided the AIS-related GSE122709 dataset. Expression data for LUAD were obtained from the Xena database. The specific sample information for the two datasets is listed below (Table 1). Using the standard of |log2FC| > 1 and P < 0.05, 4452 and 2013 upregulated DEGs were identified in the AIS and TCGA-LUAD datasets, respectively. We found 372 upregulated DEGs intersecting the two datasets, which may be connected to the pathophysiology of AIS and LUAD.
3.2. Functional analyses
The 372 elevated DEGs were examined to seek into the shared biological processes and signaling pathways. The GO analysis revealed that these genes were mainly enriched in nuclear, organic fission, and mitotic nuclear divisions. The main KEGG enrichment pathways were the cell cycle, cellular senescence, and the HIF-1 signaling pathway in AIS and LUAD (Figure 2A). DO analysis revealed that the DEGs were linked to many malignancies. These results imply that LUAD and AIS development and incidence may be caused by the differential expression of these genes. These pathways were primarily enriched in the cell cycle, cellular senescence, and human T-cell leukemia virus 1 infection in LUAD, according to the 372 elevated DEGs discovered by GSEA (Figure 2B). In contrast, no related pathways were enriched in the 372 upregulated DEGs in AIS. In AIS, CDKN2A is mainly localized in the calcium signaling pathway, NOD-like receptor signaling pathway and olfactory transduction pathway (Figure 3A). In LUAD, CDKN2A was mostly implicated in alpha-linolenic acid metabolism, drug metabolism-cytochrome P450, and Linoleic acid metabolism (Figure 3B). We selected DEGs in the cellular senescence pathway using KEGG for analysis. We discovered hub genes and elucidated the potential link between DEG-encoded proteins. Using the STRING database, we built a PPI network and identified the top 10 genes (Table 2), and then we defined four hub genes CCNA2, CCNB1, CDKN2A, and CDK1 from the top 10 genes using Cytoscape.
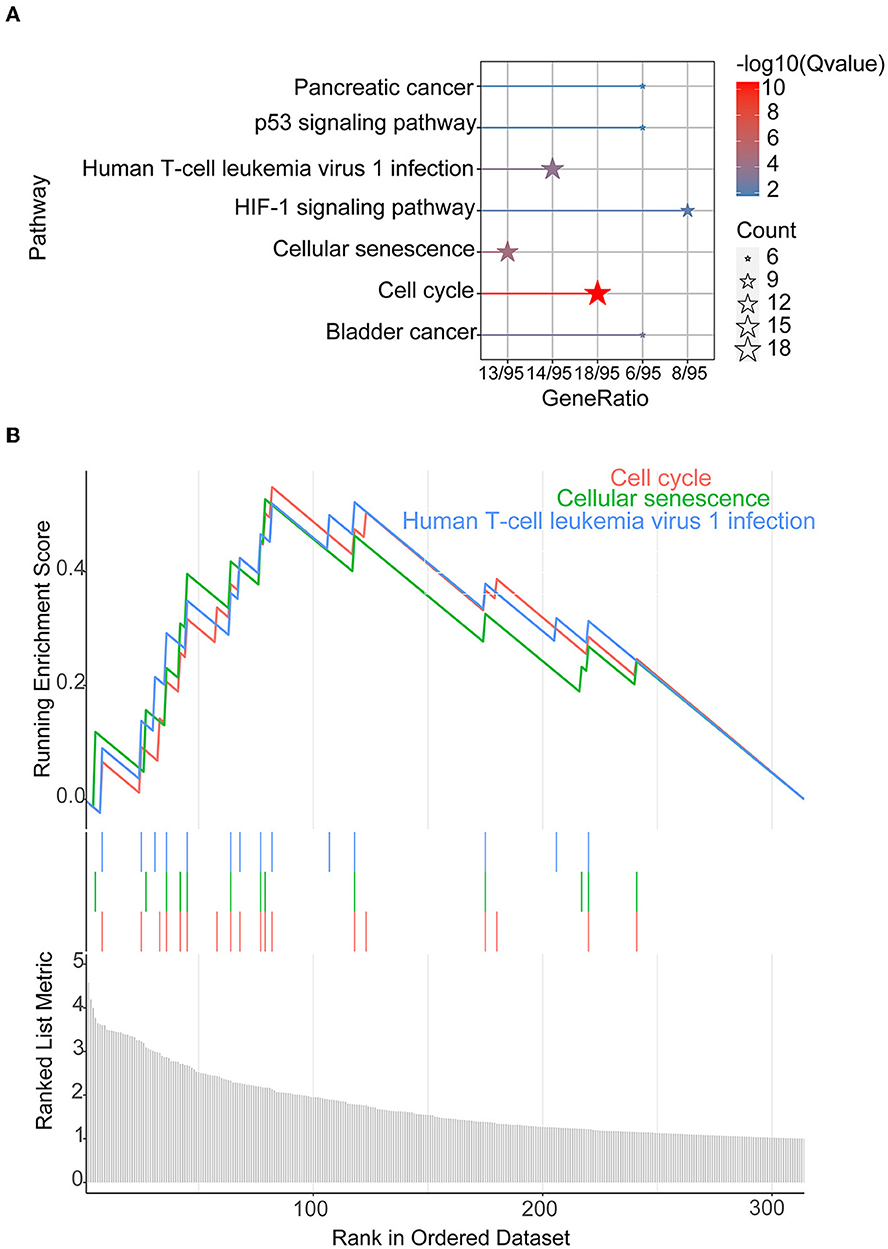
Figure 2. KEGG and GSEA. (A) KEGG of 372 upregulated DEGs in AIS and LUAD. (B) We conducted GSEA on 372 upregulated DEGs in LUAD.
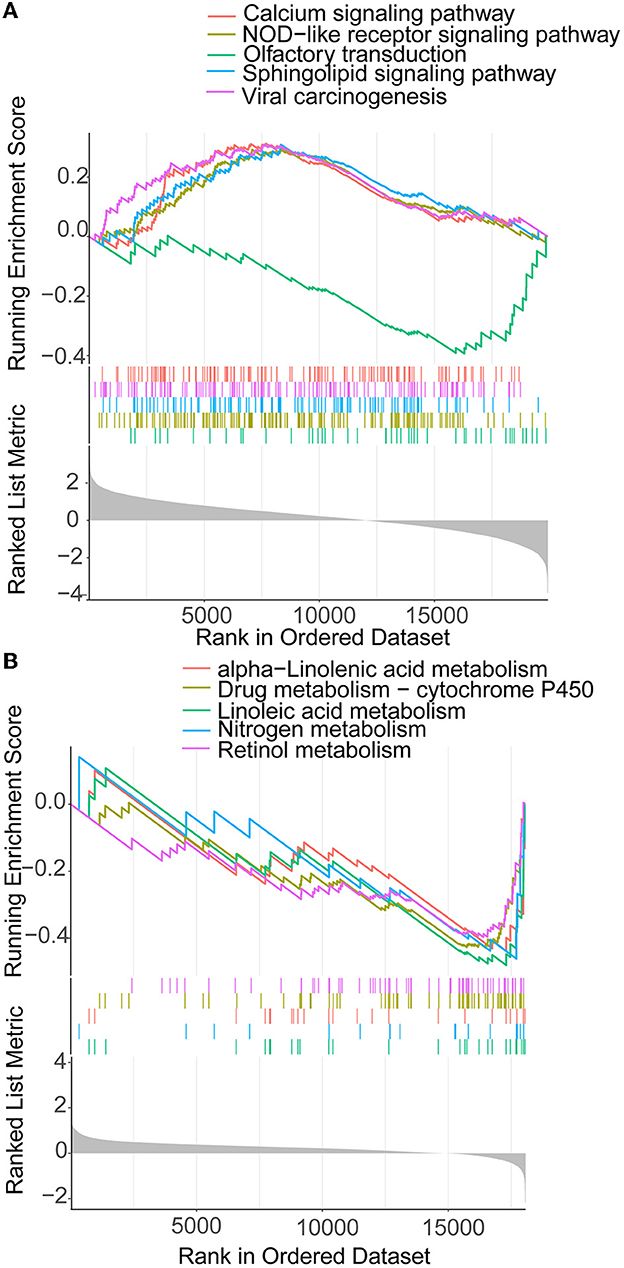
Figure 3. GSEA of CDKN2A in AIS and LUAD. (A) CDKN2A is involved in the main pathway of AIS. (B) CDKN2A is involved in the main pathway of LUAD.
3.3. Validation of hub genes expression and assessment of diagnostic value
The hub genes displayed considerably higher expression in LUAD and AIS patients compared to the control group. In addition, we checked the expression of four hub genes in AIS using the validation datasets (GSE58294 and GSE140275). According to our findings, AIS patients had higher levels of CCNA2, CCNB1, and CDK1 expression compared to the control group (Supplementary Figure 1). However, the four hub genes are insignificant in the GSE140275 dataset, which would account for the limited sample size. Single-cell data analysis revealed that 10996 cells were divided into 11 clusters. The results indicated that CCNA2, CCNB1, CDK1 and CDKN2A were expressed in cancer epithelial cells (Supplementary Figure 2). The diagnostic effectiveness of these hub genes was further investigated using ROC curves, which revealed that these genes have great diagnostic efficacy for LUAD and AIS (Figure 4). This work analyzed immunohistochemistry slices from LUAD patients and typical tissues from healthy people. The four hub genes' protein expression levels were higher in LUAD tissues than those in healthy tissues (Figures 5, 6). We used the digital pathology software QuPath to count the number of positive cells in LUAD and normal lung tissue figures. As a result, we observed that there were more positive cells overall in the LUAD field than there were in the normal lung tissue (Supplementary Figure 3). It's showed that four hub genes had higher protein levels in LUAD compared with normal tissues (Supplementary Figure 4).
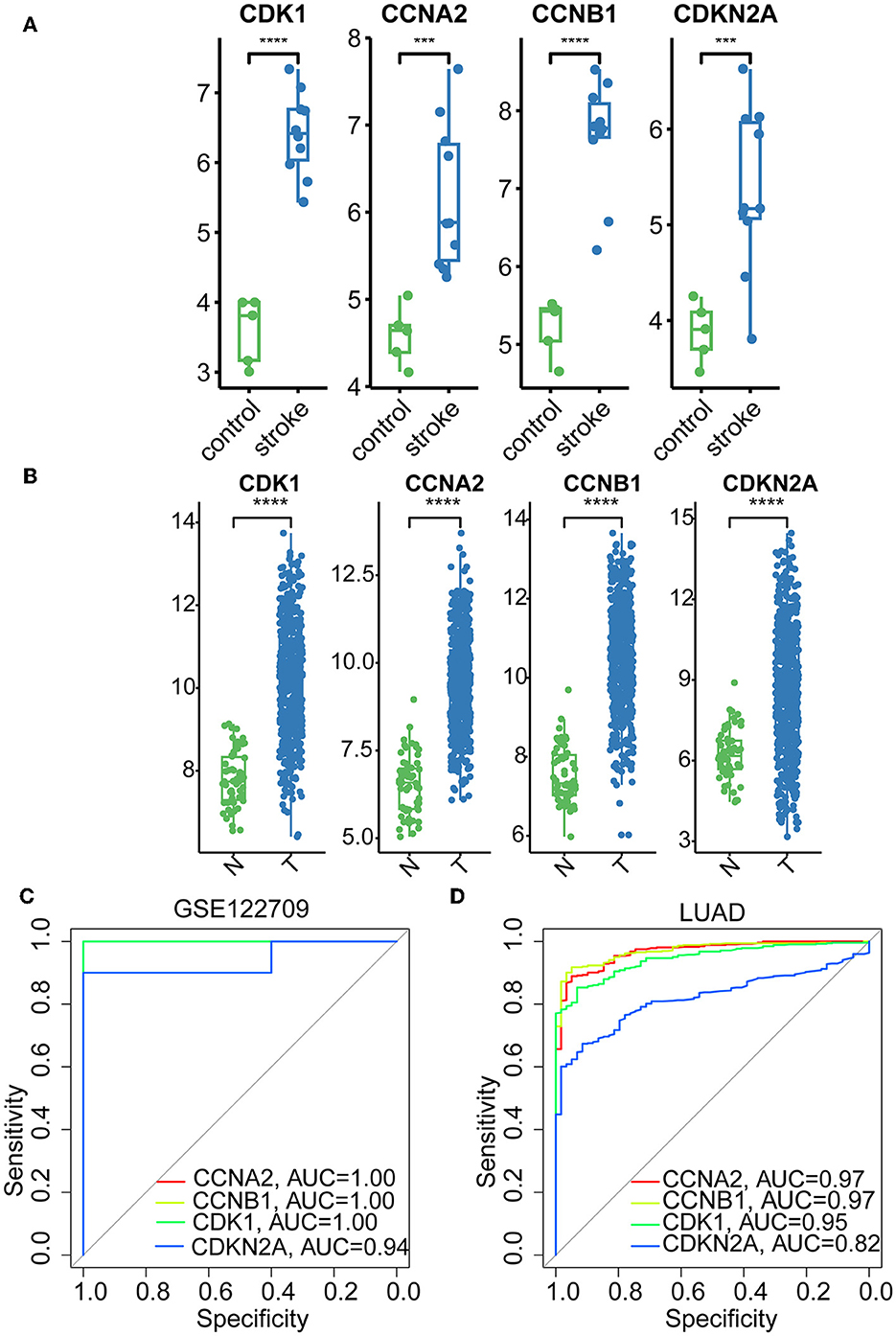
Figure 4. Boxplots and ROC curves of four hub genes in AIS and LUAD. (A) Boxplots of the four hub genes in AIS. (B) Boxplots of the four hub genes in LUAD. (C) ROC curves of the four hub genes in AIS. (D) ROC curves of the four hub genes in LUAD (***, p < 0.001; ****, p < 0.0001; N, normal; T, tumor).
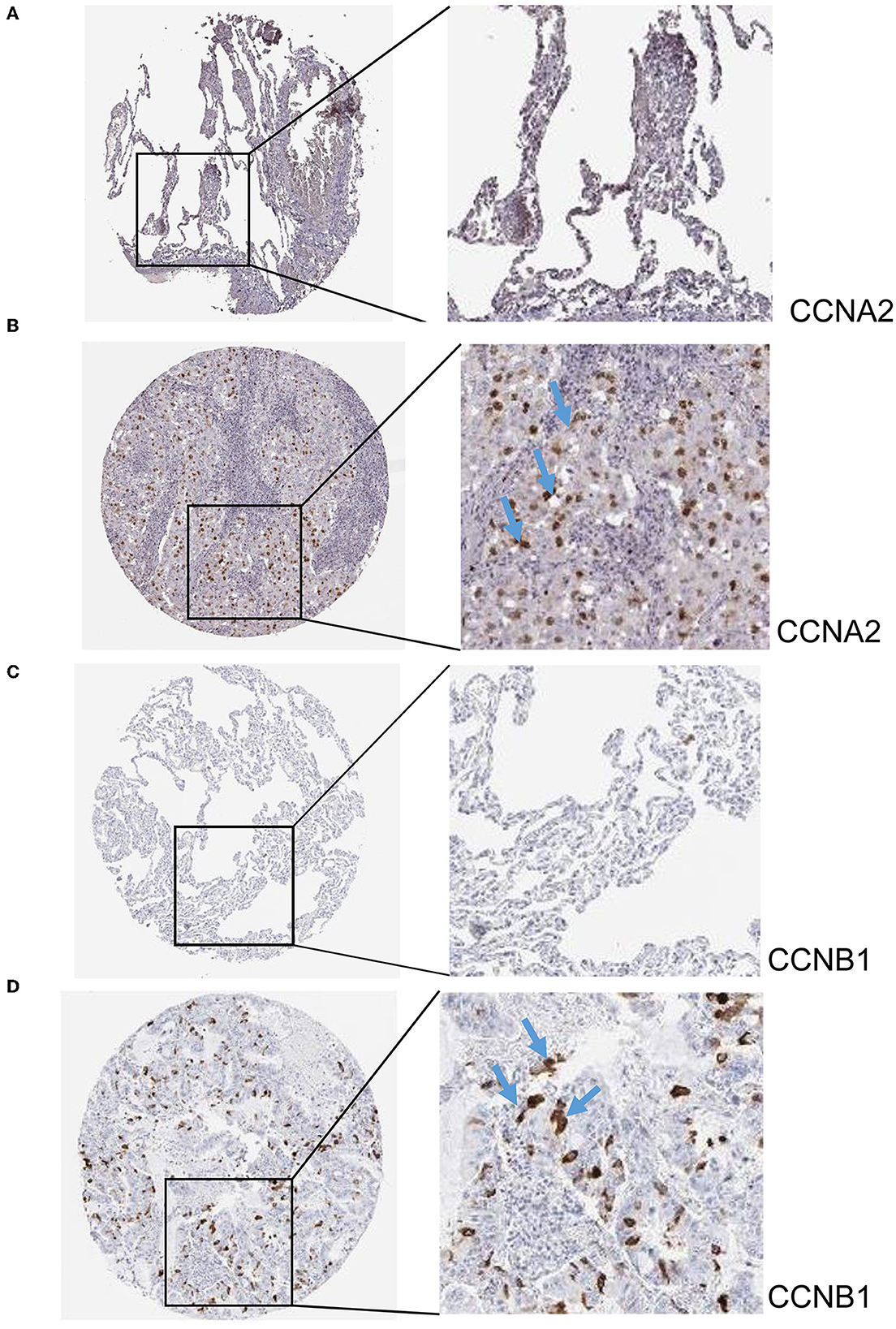
Figure 5. Immunohistochemistry slices of CCNA2 and CCNB1. (A) Immunohistochemistry slices of normal lung tissues of CCNA2. (B) Immunohistochemistry slices of LUAD of CCNA2. (C) Immunohistochemistry slices of normal lung tissues of CCNB1. (D) Immunohistochemistry slices of LUAD of CCNB1.
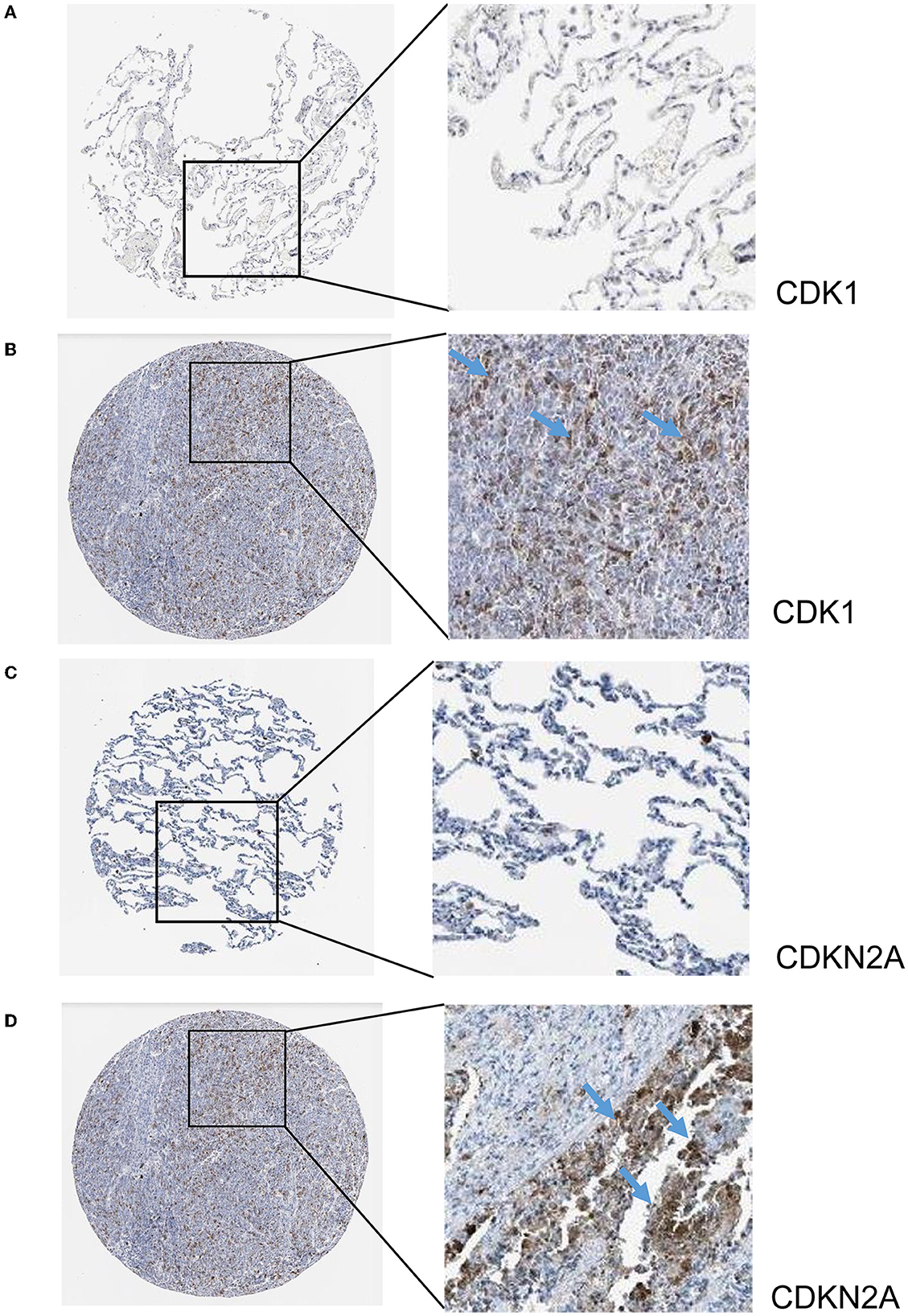
Figure 6. Immunohistochemistry slices of CDK1 and CDKN2A. (A) Immunohistochemistry slices of normal lung tissues of CDK1. (B) Immunohistochemistry slices of LUAD of CDK1. (C) Immunohistochemistry slices of normal lung tissues of CDKN2A. (D) Immunohistochemistry slices of LUAD of CDKN2A.
3.4. Survival analysis and validation
We performed a survival study where patients were classified into high-risk and low-risk groups depending on their gene expression level to confirm the prognosis of the four hub genes in LUAD. The findings revealed that hub genes with high expression have a poor prognosis in LUAD (Figure 7). It was revealed that the OS time of patients with a high risk score was significantly shorter than that of patients with a low risk score. The AUC values of 1,3 and 5 years of four hub genes were about 0.5–0.6 (Figure 8). By plotting KM curves and univariate Cox regression analysis, we obtained 5-year AUC, HR and 95% CI (Supplementary Table 1).
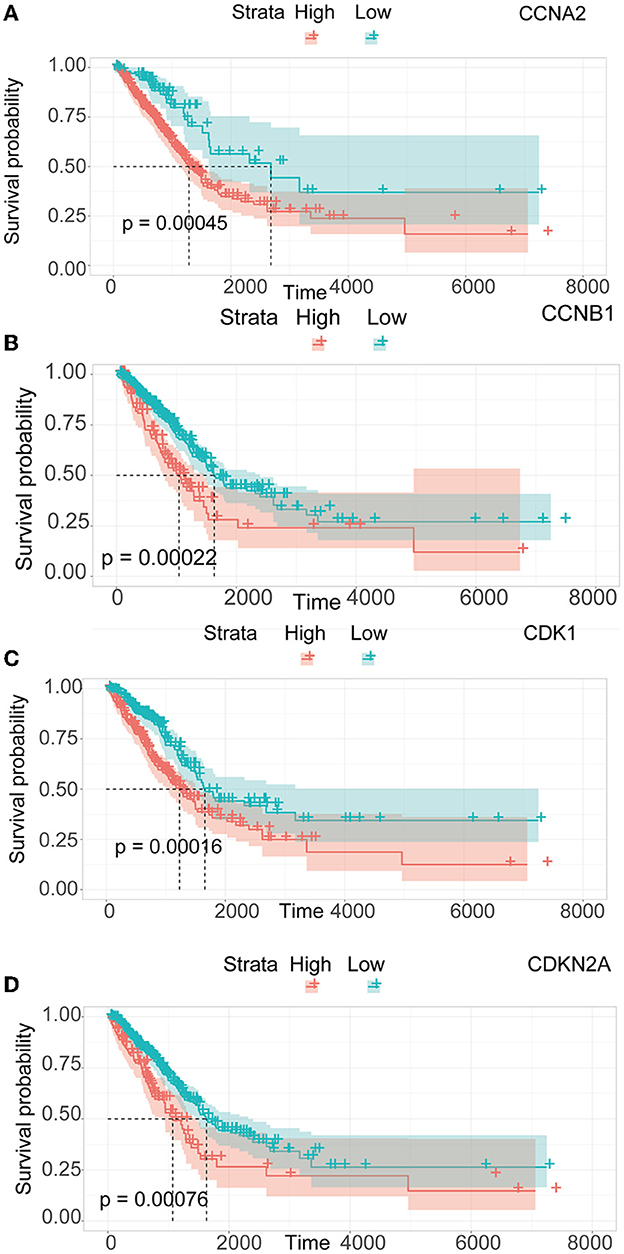
Figure 7. Survival analysis of LUAD. (A) Survival analysis of CCNA2. (B) Survival analysis of CCNB1. (C) Survival analysis of CDK1. (D) Survival analysis of CDKN2A.
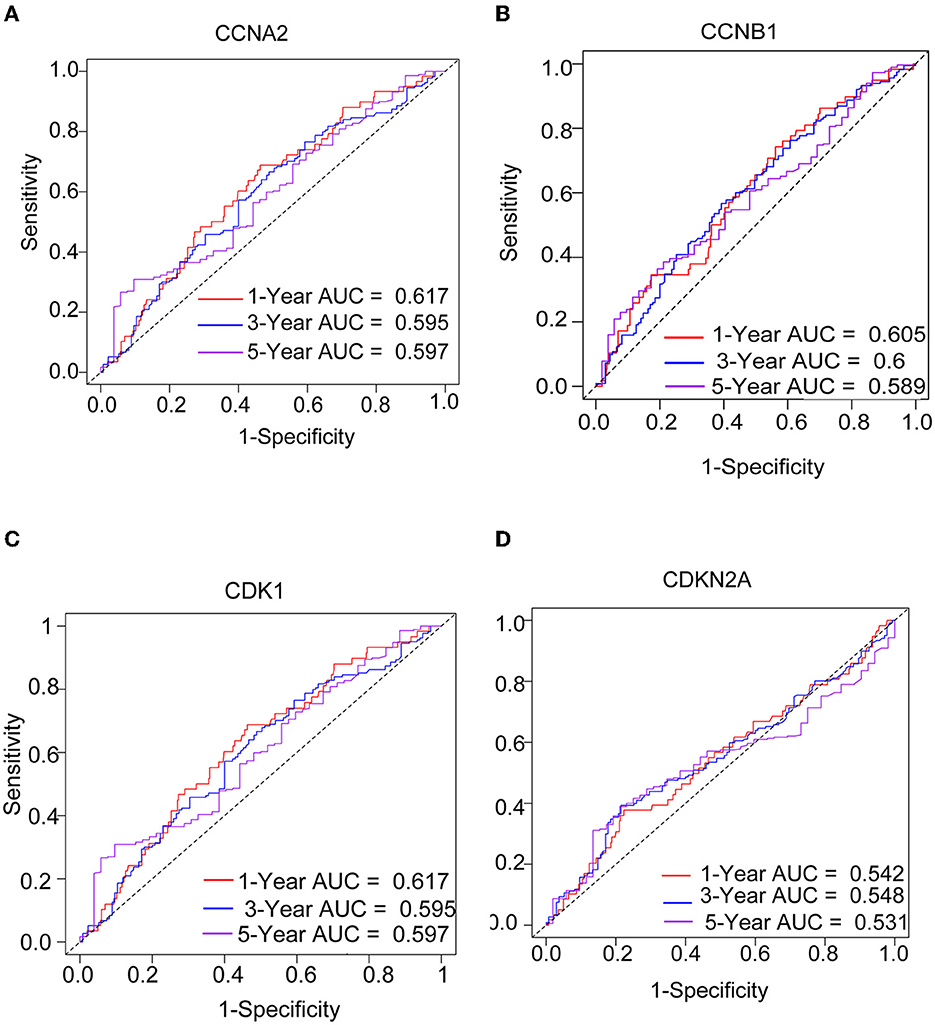
Figure 8. The ROC curves of four hub genes for predicting 1, 3, and 5-year mortality risk in the TCGA-LUAD dataset. (A) CCNA2. (B) CCNB1. (C) CDK1. (D) CDKN2A.
3.5. Immune infiltration analysis in LUAD and AIS
Immune infiltration is essential for the onset, prognosis, and treatment of numerous disorders. While the control group had higher levels of neutrophils, AIS patients had higher levels of resident memory CD4+ T cells, monocytes, and eosinophils (Figure 9A). As seen in the image, several immune cells are involved in the formation of LUAD (Figure 9B). In AIS patients, only CDK1 is associated with CD8+ T cells (Figure 10A). In LUAD patients, infiltration levels of resident memory CD4+ T cells, and activated dendritic cells were positively linked with CDKN2A overexpression. The immunological infiltration of resident memory CD4+ T cells, macrophage, activated dendritic cells, mast cells resting, activated NK cells and neutrophils were favorably connected with CDK1 and CCNA2 overexpression. However, it was not discovered that CCNB1 was connected to immune cells (Figure 10B).
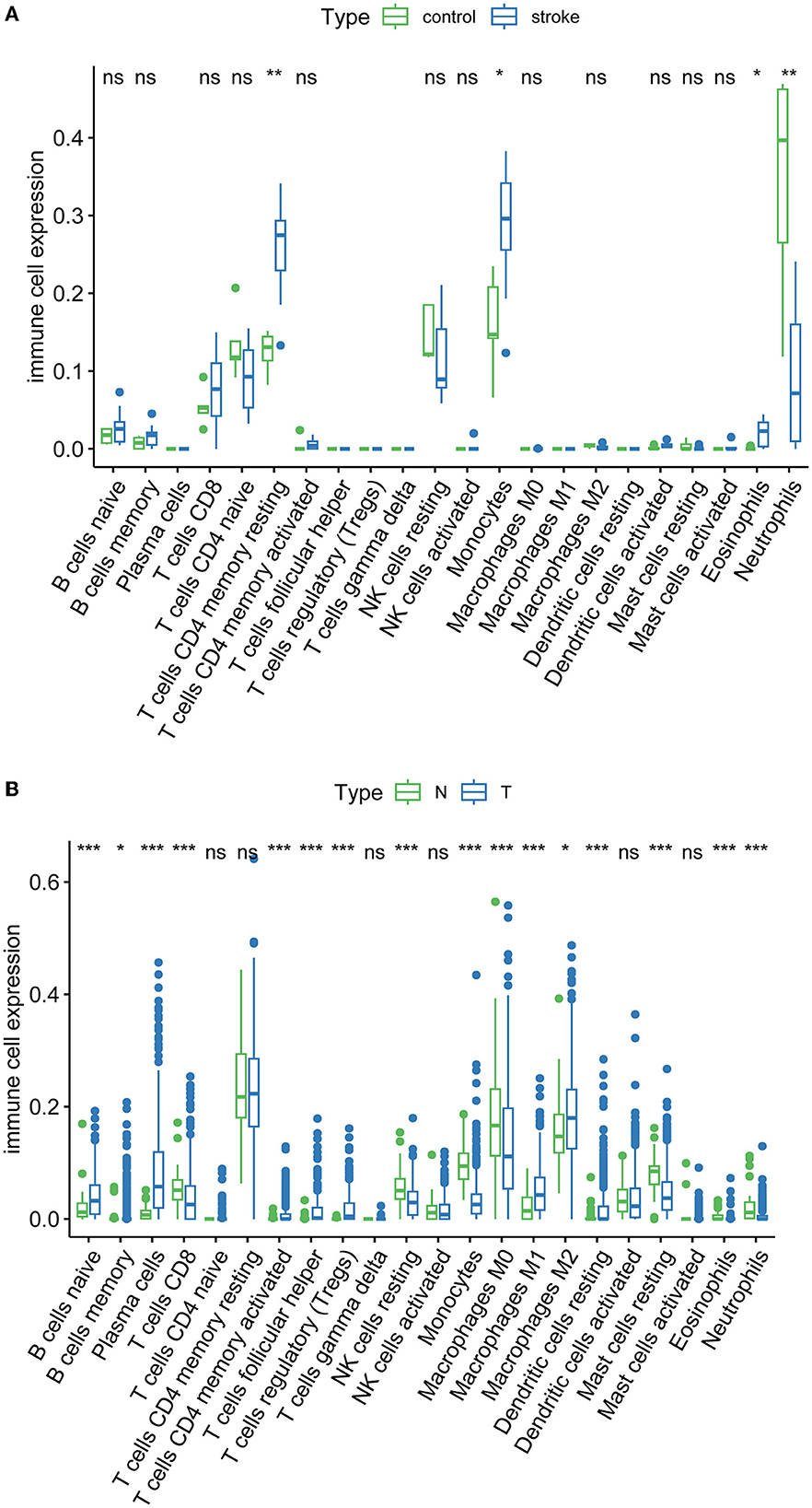
Figure 9. Immune infiltration of AIS and LUAD. (A) AIS. (B) LUAD (*, P < 0.05; **, P < 0.01; ***, P < 0.001; ns, not significant; N, normal; T, tumor).
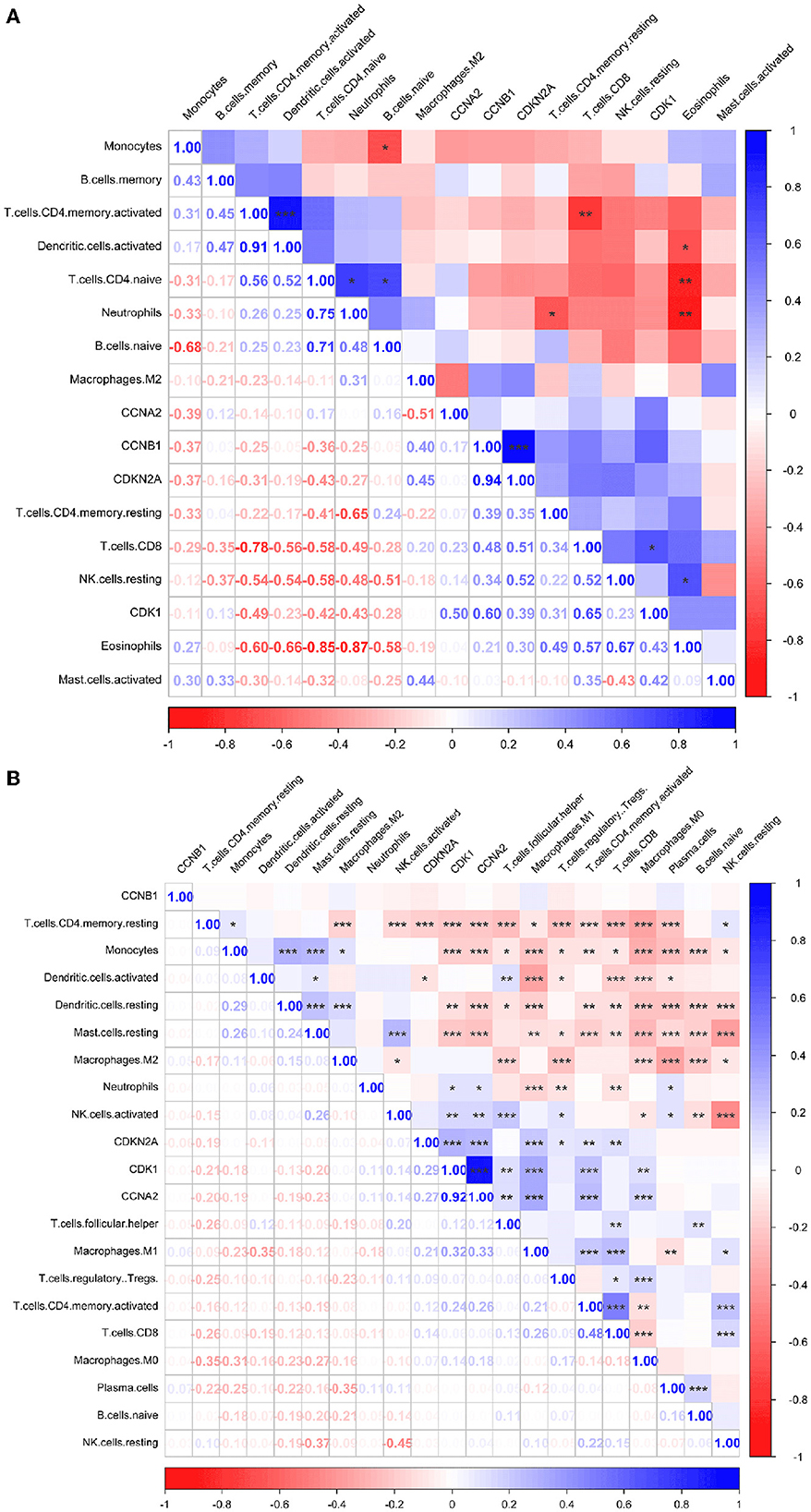
Figure 10. Immune correlation heatmaps of four hub genes in AIS and LUAD. (A) AIS. (B) LUAD (*, P < 0.05; **, P < 0.01; ***, P < 0.001).
3.6. Molecular docking of curcumin with hub genes
Potential medicines were identified based on transcriptome features in the DSigDB database of Enrichr. Curcumin is considered as a potential drug for AIS treatment and later analysis. Moreover, we predicted the binding mechanism of curcumin with four hub genes using molecular docking. It is commonly accepted that the likelihood of action increases with decreasing ligand and receptor binding energies. The binding energies of curcumin with four hub genes (CCNA2, CCNB1, CDK1, CDKN2A) were −6.999 kcal/mol, −5.87 kcal/mol, −7.1 kcal/mol, −5.366 kcal/ mol, respectively. These findings demonstrate the highly stable binding of curcumin to four hub genes (Figure 11).
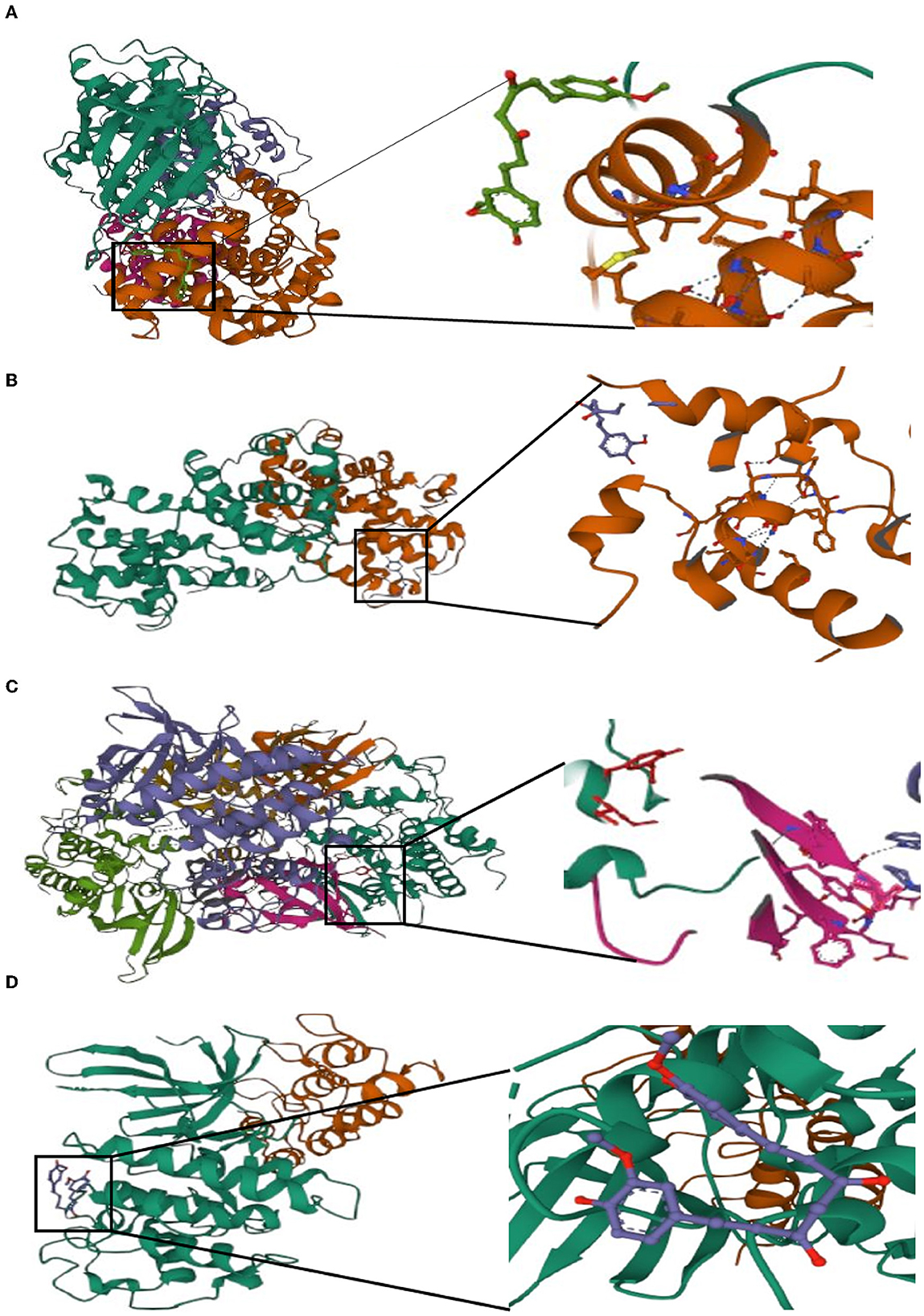
Figure 11. Molecular docking of curcumin with four hub genes. (A) CCNA2. (B) CCNB1. (C) CDK1. (D) CDKN2A.
3.7. DEGs of different etiologies of AIS
According to the analysis above, CCNA2, CCNB1, CDK1, CDKN2A are the hub genes for AIS and LUAD. Results of DEGs of AIS caused by atherosclerosis and cardiac embolism shown by heatmaps (Supplementary Figure 5).
4. Discussion
The leading cause of adult disability and the second leading cause of death globally, behind ischemic heart disease, is stroke (24). Nearly 85% of lung cancer cases are non-small cell lung cancer (NSCLC), with the most prevalent histological subtype, LUAD, having a high mortality and recurrence rate (25). Stroke patients have an increased incidence of cancer, including lung cancer (26). A previous clinical study indicated that AIS may be a unique precursor to LUAD. In patients with LUAD combined with AIS, LUAD occurs due to the occurrence of AIS. The prevalence of lung cancer in the stroke cohort was 5.3 per 1000 person-years (27, 28). Lung cancer patients are most likely to experience a stroke within one year after diagnosis. The risk of stroke occurring in lung cancer patients is more than two times higher than that in people without cancer (HR 2.40, 95% CI 1.53–3.78) (23, 29). However, the causal relationship, genetic mechanisms, and their interactions between LUAD and AIS are still unclear. It is crucial to study the biological processes that underlie AIS and LUAD. The cerebral atherosclerosis-related gene PITX2, RGS7, NKX2-5, NKX2-5, and ZFHX3 are involved in AIS. The genes of AIS brought on by cardiogenic embolism are TM4SF4-TM4SF1, EDNRA, HDAC9-TWIST1, and LINC01492 (30). Our study shows that the hub genes of AIS with LUAD are CCNA2, CCNB1, CDK1, and CDKN2A. Meanwhile, our findings suggest that LUAD-induced AIS is distinct from AIS produced by large artery atherosclerosis and cardiac embolism in terms of genes. These genes' prognostic and diagnostic capabilities in LUAD were examined, and their mRNA levels were significantly elevated in AIS and LUAD. Herein, we investigated the link between AIS and LUAD using our four hub genes and explored the underlying biological mechanisms shared between these two diseases.
GO, KEGG, and DO enrichment analyses were performed on the 372 DEGs that intersecting both datasets. DEGs are mainly enriched in nuclear division, organelle fission and mitotic nuclear division, and they are associated with the biological behavior of LUAD and the neuroprotective inhibition observed in AIS. The primary enriched pathways in KEGG are the cell cycle, cellular senescence, and the HIF-1 signaling pathway. The cellular senescence pathway was highlighted as the main contributor of this study, and the overexpression of four cellular senescence-related genes was closely related to AIS and LUAD. HIF-1 was found to have a positive connection with infarct size in AIS (31). However, HIF's impact on neuronal survival following a stroke is still debatable (32). HIF-1-α is associated with aggressiveness in lung cancer (33). Our investigation revealed that the DEGs were linked to many malignancies.
According to one definition, cellular senescence is an ongoing proliferative arrest brought on by various stressors. Furthermore, senescence and hyperplastic pathology are both related to a type of stress response known as cellular senescence (34). Numerous neurological diseases, including neurodegenerative conditions like Alzheimer's disease, Parkinson's disease, and stroke, are linked to cellular senescence (35). Cellular senescence of the cells happens as AIS, which develops and progresses (36). AIS and neurological damage are impacted by the senescence-associated secretory phenotype (SASP) (37). Cellular senescence is considered the new hallmark of cancer, as malignant and non-malignant tumor cells develop a SASP that stimulates cancer recurrence and metastasis (38). However, it was once believed that cellular senescence contributed to the prevention of tumors; therefore, the mechanism of cellular senescence in cancer is not welldefined and should be further explored (39). Recently, it was demonstrated that a high expression of cellular senescence genes correlates with a poor LUAD prognosis (40). This is in line with our study, where our four hub genes CCNA2, CCNB1, CDKN2A, and CDK1 were related to cellular senescence pathways. We investigated genes that were highly expressed in LUAD and that were linked to poor prognosis. It is crucial to deepen our understanding of how cellular senescence in AIS and LUAD functions, how it interacts with the immune system, and how it affects prognosis because the mechanism underlying these diseases has not yet been fully uncovered.
CCNB1 combines with CDK1 to form a complex that enables cells to enter the G2/M phase to promote mitosis (41, 42). Numerous malignancies have high CCNB1 expression (43), increase apoptosis and cell death via controlling the p53 signaling pathway (44), accumulate in the degenerating brain regions of stroke patients, and can participate in neuronal death (45). The regulation of the mitotic cell cycle is influenced by CDK1 (46). According to studies, CDK1 may contribute to stroke through an oxidative mode of damage (47) and may also be a potential biomarker for NSCLC (48). CDK1 is upregulated in patients with LUAD and accelerates tumor progression (49). CCNB1 and CDK1 enable the sustained proliferation of NSCLC by regulating the pRb protein (50). In our study, survival analysis showed that LUAD and AIS patients with overexpression of CCNA2, CCNB1, and CDK1 had a poor prognosis and that these genes can be used as predictors of prognosis. In addition, these genes have high diagnostic abilities in patients with LUAD and AIS and could later serve as biomarkers for predicting these two diseases.
The cell cycle is regulated by CDKN2A, a cyclin-dependent kinase inhibitor that encodes the p16 protein (51). CDKN2A is a ferroptosis and cuproptosis gene (52, 53). When iron-dependent lipid hydroperoxides build up to deadly amounts, controlled cell death known as ferroptosis occurs (54). Cuproptosis is another type in which excess copper leads to cell death by aggregation of mitochondrial proteins (55). According to reports, CDKN2A is a locus for AIS risk. It has been linked to an elevated risk of AIS in the Han Chinese population and in native West African men. Genetic variation at the CDKN2A locus also predicts stroke in hypertensive patients (56–58). Lung cancer is associated with genetic mutations in CDKN2A, such as genomic deletions (59). The high expression of CDKN2A in LUAD is correlated with a bad prognosis. High CDKN2A expression may be associated with increased immune cell numbers, immune checkpoint enhancement, and elevated chemokine levels (60). CDKN2A is often regarded as a tumor suppressor gene; however, hypermethylated CDKN2A may be responsible for poor cancer prognosis (61). These four hub genes were verified in the HPA database and discovered to be related to LUAD prognosis, indicating they are crucial to the onset and development of LUAD. Curcumin has been shown to have neuroprotective and neuroregenerative properties. It can also be utilized as a drug for the treatment of ischemic stroke (62). This is in line with our findings, which shows that curcumin has a strong affinity to four hub genes. Curcumin might be a potential therapeutic target for the therapy of AIS, according to our drug prediction and molecular docking results.
This research has several restrictions. First, this study only employed a few samples. Second, we did not confirm the hub genes discovered in this study based on data from earlier experiments. However, their expression levels were validated.
According to our research, CCNA2, CCNB1, CDKN2A, and CDK1 are significant components in AIS and LUAD. With multiple analyses, we explored AIS and LUAD pathogenesis as well as their common molecular mechanisms and observed high expression of these four hub genes, high diagnostic power in patients, and poor prognosis. Our results shed new light on improving AIS and LUAD prognosis. Further research into early intervention and treatment using our identified hub genes is warranted. Overall, this study identified CCNA2, CCNB1, CDKN2A, and CDK1 as hub genes involved in the cellular senescence pathway and may serve as diagnostic and prognostic indicators for AIS and LUAD.
Data availability statement
The original contributions presented in the study are included in the article/Supplementary material, further inquiries can be directed to the corresponding author.
Author contributions
LC and R-XQ conceived the study. R-XQ, YY, and J-FC contributed to the data analysis and interpretation of the results. L-JH, WX, Q-CQ, X-JL, X-YL, X-YH, and M-SX wrote the manuscript. LC, R-XQ, YY, J-FC, L-JH, WX, Q-CQ, X-JL, X-YL, X-YH, and M-SX contributed to its editing. All authors contributed to the article and approved the submitted version.
Funding
This study was supported by grants from the National Natural Science Foundation of China (82271371 and 82260367), the High-Level Medical Expert Training Program of Guangxi 139 Plan Funding, the Guangxi Medical and Health Appropriate Technology Development and Application Project (S2021107), the Clinical Research Climbing Program of the First Affiliated Hospital of Guangxi Medical University (YYZS2021002), and Advanced Innovation Teams and Xinghu Scholars Program of Guangxi Medical University, 2023 Guangxi Postgraduate Innovation Project (YCSW2023242).
Conflict of interest
The authors declare that the research was conducted in the absence of any commercial or financial relationships that could be construed as a potential conflict of interest.
Publisher's note
All claims expressed in this article are solely those of the authors and do not necessarily represent those of their affiliated organizations, or those of the publisher, the editors and the reviewers. Any product that may be evaluated in this article, or claim that may be made by its manufacturer, is not guaranteed or endorsed by the publisher.
Supplementary material
The Supplementary Material for this article can be found online at: https://www.frontiersin.org/articles/10.3389/fneur.2023.1119160/full#supplementary-material
References
1. Li R, Cheng X, Schwebel DC, Yang Y, Ning P, Cheng P, et al. Disability-adjusted life years associated with population ageing in China, 1990–2017. BMC Geriatr. (2021) 21:369. doi: 10.1186/s12877-021-02322-7
2. Barthels D, Das H. Current advances in ischemic stroke research and therapies. Biochimica et biophysica acta Mol Basis Dis. (2020) 1866:165260. doi: 10.1016/j.bbadis.2018.09.012
3. Tu WJ, Zhao Z, Yin P, Cao L, Zeng J, Chen H, et al. Estimated burden of stroke in China in 2020. JAMA Netw Open. (2023) 6:e231455. doi: 10.1001/jamanetworkopen.2023.1455
4. Chung JW, Park SH, Kim N, Kim WJ, Park JH, Ko Y, et al. Trial of ORG 10172 in Acute Stroke Treatment (TOAST) classification and vascular territory of ischemic stroke lesions diagnosed by diffusion-weighted imaging. J Am Heart Assoc. (2014) 3:4. doi: 10.1161/JAHA.114.001119
5. Feigin VL, Krishnamurthi RV, Parmar P, Norrving B, Mensah GA, Bennett DA, et al. Update on the global burden of ischemic and hemorrhagic stroke in 1990-2013: The GBD 2013 study. Neuroepidemiology. (2015) 45:161–76. doi: 10.1159/000441085
6. Kumar A, Misra S, Sagar R, Kumar P, Yadav AK, Talwar P, et al. Relationship between factor V Leiden Gene variant and risk of ischemic stroke: a case-control study. Ann Indian Acad Neurol. (2017) 20:284–8.
7. Iadecola C, Anrather J. The immunology of stroke: from mechanisms to translation. Nat Med. (2011) 17:796–808. doi: 10.1038/nm.2399
8. Iadecola C, Buckwalter MS, Anrather J. Immune responses to stroke: mechanisms, modulation, and therapeutic potential. J Clin Invest. (2020) 130:2777–88. doi: 10.1172/JCI135530
9. Campbell BC. Thrombolysis and thrombectomy for acute ischemic stroke: strengths and synergies. Semin Thromb Hemost. (2017) 43:185–90. doi: 10.1055/s-0036-1585078
10. Sung H, Ferlay J, Siegel RL, Laversanne M, Soerjomataram I, Jemal A, et al. Global cancer statistics 2020: GLOBOCAN estimates of incidence and mortality worldwide for 36 cancers in 185 countries. CA Cancer J Clin. (2021) 71:209–49. doi: 10.3322/caac.21660
11. Qi SA, Wu Q, Chen Z, Zhang W, Zhou Y, Mao K, et al. High-resolution metabolomic biomarkers for lung cancer diagnosis and prognosis. Sci Rep. (2021) 11:11805. doi: 10.1038/s41598-021-91276-2
12. Behera M, Owonikoko TK, Gal AA, Steuer CE, Kim S, Pillai RN, et al. Lung Adenocarcinoma Staging using the 2011 IASLC/ATS/ERS classification: a pooled analysis of adenocarcinoma in situ and minimally invasive adenocarcinoma. Clin Lung Cancer. (2016) 17:e57–64. doi: 10.1016/j.cllc.2016.03.009
13. de Sousa VML, Carvalho L. Heterogeneity in lung cancer. Pathobiol J Immunopathol Mol Cell Biol. (2018) 85:96–107. doi: 10.1159/000487440
14. Dai X, Lu L, Deng S, Meng J, Wan C, Huang J, et al. USP7 targeting modulates anti-tumor immune response by reprogramming tumor-associated macrophages in lung cancer. Theranostics. (2020) 10:9332–47. doi: 10.7150/thno.47137
15. Fan Y, Liu B, Chen F, Song Z, Han B, Meng Y, et al. Hepcidin upregulation in lung cancer: a potential therapeutic target associated with immune infiltration. Front Immunol. (2021) 12:612144. doi: 10.3389/fimmu.2021.612144
16. Garnis C, Buys TP, Lam WL. Genetic alteration and gene expression modulation during cancer progression. Mol Cancer. (2004) 3:9. doi: 10.1186/1476-4598-3-9
17. Sibilia M, Kroismayr R, Lichtenberger BM, Natarajan A, Hecking M, Holcmann M. The epidermal growth factor receptor: from development to tumorigenesis. Differentiation. (2007) 75:770–87. doi: 10.1111/j.1432-0436.2007.00238.x
18. Yang G, Wang W, Han S, Xu S, Liu H. Effect of microRNA-181b on the biological characteristics and clinical drug resistance of small-cell lung cancer by targeting angiotensin converting enzyme 2. Thoracic Cancer. (2022) 13:742–9. doi: 10.1111/1759-7714.14313
19. Salazar-Camelo RA, Moreno-Vargas EA, Cardona AF, Bayona-Ortiz HF. Ischemic stroke: a paradoxical manifestation of cancer. Crit Rev Oncol Hematol. (2021) 157:103181. doi: 10.1016/j.critrevonc.2020.103181
20. Chen PC, Muo CH, Lee YT Yu YH, Sung FC. Lung cancer and incidence of stroke: a population-based cohort study. Stroke. (2011) 42:3034–9. doi: 10.1161/STROKEAHA.111.615534
21. Yuan T, Wang J. [Clinical and Imaging Features of Acute Cerebral Infarction in Non-small Cell Lung Cancer Patients with Trousseau Syndrome]. Zhongguo Fei Ai Za Zhi. (2021) 24:13–8. doi: 10.3779/j.issn.1009-3419.2021.102.01
22. Li MJ, Yan SB, Chen G, Li GS, Yang Y, Wei T, et al. Upregulation of CCNB2 and its perspective mechanisms in cerebral ischemic stroke and all subtypes of lung cancer: a comprehensive study. Front Integr Neurosci. (2022) 16:854540. doi: 10.3389/fnint.2022.854540
23. Zhang J, Zhao J. Clinical characteristics and analysis of lung cancer- associated acute ischemic stroke. J Stroke Cerebrovascul Dis Offic J Nat Stroke Assoc. (2020) 29:105164. doi: 10.1016/j.jstrokecerebrovasdis.2020.105164
24. Koton S, Schneider AL, Rosamond WD, Shahar E, Sang Y, Gottesman RF, et al. Stroke incidence and mortality trends in US communities, 1987 to 2011. Jama. (2014) 312:259–68. doi: 10.1001/jama.2014.7692
25. Bajbouj K, Al-Ali A, Ramakrishnan RK, Saber-Ayad M, Hamid Q. Histone modification in NSCLC: molecular mechanisms and therapeutic targets. Int J Mol Sci. (2021) 22:21. doi: 10.3390/ijms222111701
26. Selvik HA, Thomassen L, Logallo N, Næss H. Prior cancer in patients with ischemic stroke: the Bergen NORSTROKE study. J Stroke Cerebrovascul Dis Offic J Nat Stroke Assoc. (2014) 23:919–25. doi: 10.1016/j.jstrokecerebrovasdis.2013.07.041
27. Lin J, Wu S, Xu R, Shi Q, Tian C, Cui F, et al. Clinical characteristics and risk factors of lung cancer-associated acute ischemic stroke. Biomed Res Int. (2019) 2019:6021037. doi: 10.1155/2019/6021037
28. Babore AD, Tybjerg AJ, Andersen KK, Olsen TS. Occult lung cancer manifesting within the first year after stroke. J Stroke Cerebrovascul Dis Offic J Nat Stroke Assoc. (2020) 29:105023. doi: 10.1016/j.jstrokecerebrovasdis.2020.105023
29. Florido R, Daya NR, Ndumele CE, Koton S, Russell SD, Prizment A, et al. Cardiovascular disease risk among cancer survivors: the atherosclerosis risk in communities (ARIC) study. J Am Coll Cardiol. (2022) 80:22–32. doi: 10.1016/j.jacc.2022.04.042
30. Ilinca A, Samuelsson S, Piccinelli P, Soller M, Kristoffersson U, Lindgren AG, et al. stroke gene panel for whole-exome sequencing. Euro J Hum Genetic EJHG. (2019) 27:317–24. doi: 10.1038/s41431-018-0274-4
31. Xue L, Chen H, Lu K, Huang J, Duan H, Zhao Y. Clinical significance of changes in serum neuroglobin and HIF-1α concentrations during the early-phase of acute ischemic stroke. J Neurol Sci. (2017) 375:52–7. doi: 10.1016/j.jns.2017.01.039
32. Barteczek P, Li L, Ernst AS, Böhler LI, Marti HH, Kunze R. Neuronal HIF-1α and HIF-2α deficiency improves neuronal survival and sensorimotor function in the early acute phase after ischemic stroke. J Cerebr Blood Flow Metabol Offic J Int Soc Cerebr Blood Flow Metabol. (2017) 37:291–306. doi: 10.1177/0271678X15624933
33. Hao S, Li F, Jiang P, Gao J. Effect of chronic intermittent hypoxia-induced HIF-1α/ATAD2 expression on lung cancer stemness. Cell Mol Biol Lett. (2022) 27:44. doi: 10.1186/s11658-022-00345-5
34. Sharpless NE, Sherr CJ. Forging a signature of in vivo senescence. Nat Rev Cancer. (2015) 15:397–408. doi: 10.1038/nrc3960
35. Wyss-Coray T. Ageing, neurodegeneration and brain rejuvenation. Nature. (2016) 539:180–6. doi: 10.1038/nature20411
36. Baixauli-Martín J, Aliena-Valero A, Castelló-Ruiz M, Burguete MC, López-Morales MA, Muñoz-Espín D, et al. Brain Cell Senescence: A New Therapeutic Target for the Acute Treatment of Ischemic Stroke. J Neuropathol Exp Neurol. (2022) 81:614–20. doi: 10.1093/jnen/nlac048
37. Torres-Querol C, Torres P, Vidal N, Portero-Otín M, Arque G, Purroy F. Acute ischemic stroke triggers a cellular senescence-associated secretory phenotype. Sci Rep. (2021) 11:15752. doi: 10.1038/s41598-021-95344-5
38. Faget DV, Ren Q, Stewart SA. Unmasking senescence: context-dependent effects of SASP in cancer. Nat Rev Cancer. (2019) 19:439–53. doi: 10.1038/s41568-019-0156-2
39. Hanahan D. Hallmarks of cancer: new dimensions. Cancer Discov. (2022) 12:31–46. doi: 10.1158/2159-8290.CD-21-1059
40. Lin W, Wang X, Wang Z, Shao F, Yang Y, Cao Z, et al. Comprehensive analysis uncovers prognostic and immunogenic characteristics of cellular senescence for lung adenocarcinoma. Front Cell Develop Biol. (2021) 9:780461. doi: 10.3389/fcell.2021.780461
41. Nam HJ, van Deursen JM. Cyclin B2 and p53 control proper timing of centrosome separation. Nat Cell Biol. (2014) 16:538–49. doi: 10.1038/ncb2952
42. Chiu HC, Huang WR, Liao TL, Chi PI, Nielsen BL, Liu JH, et al. Mechanistic insights into avian reovirus p17-modulated suppression of cell cycle CDK-cyclin complexes and enhancement of p53 and cyclin H interaction. J Biol Chem. (2018) 293:12542–62. doi: 10.1074/jbc.RA118.002341
43. Ding K, Li W, Zou Z, Zou X, Wang C. CCNB1 is a prognostic biomarker for ER+ breast cancer. Med Hypotheses. (2014) 83:359–64. doi: 10.1016/j.mehy.2014.06.013
44. Zhang H, Zhang X, Li X, Meng WB, Bai ZT, Rui SZ, et al. Effect of CCNB1 silencing on cell cycle, senescence, and apoptosis through the p53 signaling pathway in pancreatic cancer. J Cell Physiol. (2018) 234:619–31. doi: 10.1002/jcp.26816
45. Maestre C, Delgado-Esteban M, Gomez-Sanchez JC, Bolaños JP, Almeida A. Cdk5 phosphorylates Cdh1 and modulates cyclin B1 stability in excitotoxicity. EMBO J. (2008) 27:2736–45. doi: 10.1038/emboj.2008.195
46. Malumbres M, Barbacid M. Cell cycle, CDKs and cancer: a changing paradigm. Nat Rev Cancer. (2009) 9:153–66. doi: 10.1038/nrc2602
47. Cobelens PM, Kavelaars A, Heijnen CJ, Ribas C, Mayor F. Jr., Penela P. Hydrogen peroxide impairs GRK2 translation via a calpain-dependent and cdk1-mediated pathway. Cell Signall. (2007) 19:269–77. doi: 10.1016/j.cellsig.2006.06.009
48. Zhang L, Peng R, Sun Y, Wang J, Chong X, Zhang Z. Identification of key genes in non-small cell lung cancer by bioinformatics analysis. PeerJ. (2019) 7:e8215. doi: 10.7717/peerj.8215
49. Xue J, Song Y, Xu W, Zhu Y. The CDK1-Related lncRNA and CXCL8 mediated immune resistance in lung adenocarcinoma. Cells. (2022) 11(17). doi: 10.3390/cells11172688
50. Arora S, Singh P, Rahmani AH, Almatroodi SA, Dohare R, Syed MA. Unravelling the Role of miR-20b-5p, CCNB1, HMGA2 and E2F7 in development and progression of non-small cell lung cancer (NSCLC). Biology. (2020) 9(8). doi: 10.3390/biology9080201
51. Chan SH, Chiang J, Ngeow J. CDKN2A germline alterations and the relevance of genotype-phenotype associations in cancer predisposition. Hered Cancer Clin Pract. (2021) 19:21. doi: 10.1186/s13053-021-00178-x
52. Shi J, Wu P, Sheng L, Sun W, Zhang H. Ferroptosis-related gene signature predicts the prognosis of papillary thyroid carcinoma. Cancer Cell Int. (2021) 21:669. doi: 10.1186/s12935-021-02389-7
53. Tsvetkov P, Coy S, Petrova B, Dreishpoon M, Verma A, Abdusamad M, et al. Copper induces cell death by targeting lipoylated TCA cycle proteins. Science (New York, NY). (2022) 375:1254–61. doi: 10.1126/science.abf0529
54. Stockwell BR, Friedmann Angeli JP, Bayir H, Bush AI, Conrad M, Dixon SJ, et al. Ferroptosis: a regulated cell death nexus linking metabolism, redox biology, and disease. Cell. (2017) 171:273–85. doi: 10.1016/j.cell.2017.09.021
55. Kahlson MA, Dixon SJ. Copper-induced cell death. Science (New York, NY). (2022) 375:1231–2. doi: 10.1126/science.abo3959
56. Wahlstrand B, Orho-Melander M, Delling L, Kjeldsen S, Narkiewicz K, Almgren P, et al. The myocardial infarction associated CDKN2A/CDKN2B locus on chromosome 9p21 is associated with stroke independently of coronary events in patients with hypertension. J Hypertens. (2009) 27:769–73. doi: 10.1097/HJH.0b013e328326f7eb
57. Akinyemi R, Arnett DK, Tiwari HK, Ovbiagele B, Sarfo F, Srinivasasainagendra V, et al. Interleukin-6 (IL-6) rs1800796 and cyclin dependent kinase inhibitor (CDKN2A/CDKN2B) rs2383207 are associated with ischemic stroke in indigenous West African Men. J Neurol Sci. (2017) 379:229–35. doi: 10.1016/j.jns.2017.05.046
58. Hu WL Li SJ, Liu DT, Wang Y, Niu SQ, Yang XC, et al. Genetic variants on chromosome 9p21 and ischemic stroke in Chinese. Brain Res Bull. (2009) 79:431–5. doi: 10.1016/j.brainresbull.2009.04.001
59. Ulivi P, Urbini M, Petracci E, Canale M, Dubini A, Bartolini D, et al. Wide next-generation sequencing characterization of young adults non-small-cell lung cancer patients. Cancers. (2022) 14(10). doi: 10.3390/cancers14102352
60. Cheng T, Wu Y, Liu Z, Yu Y, Sun S, Guo M, et al. CDKN2A-mediated molecular subtypes characterize the hallmarks of tumor microenvironment and guide precision medicine in triple-negative breast cancer. Front Immunol. (2022) 13:970950. doi: 10.3389/fimmu.2022.970950
61. Xing X, Cai W, Shi H, Wang Y, Li M, Jiao J, et al. The prognostic value of CDKN2A hypermethylation in colorectal cancer: a meta-analysis. Br J Cancer. (2013) 108:2542–8. doi: 10.1038/bjc.2013.251
Keywords: lung adenocarcinoma, acute ischemic stroke, transcriptome, cellular senescence, bioinformatics
Citation: Qin R-X, Yang Y, Chen J-F, Huang L-J, Xu W, Qin Q-C, Liang X-J, Lai X-Y, Huang X-Y, Xie M-S and Chen L (2023) Transcriptomic analysis reveals the potential biological mechanism of AIS and lung adenocarcinoma. Front. Neurol. 14:1119160. doi: 10.3389/fneur.2023.1119160
Received: 08 December 2022; Accepted: 26 April 2023;
Published: 17 May 2023.
Edited by:
Wen-Jun Tu, Chinese Academy of Medical Sciences and Peking Union Medical College, ChinaReviewed by:
Lin Zhang, Renmin Hospital of Wuhan University, ChinaYuto Uchida, Johns Hopkins Medicine, United States
Copyright © 2023 Qin, Yang, Chen, Huang, Xu, Qin, Liang, Lai, Huang, Xie and Chen. This is an open-access article distributed under the terms of the Creative Commons Attribution License (CC BY). The use, distribution or reproduction in other forums is permitted, provided the original author(s) and the copyright owner(s) are credited and that the original publication in this journal is cited, in accordance with accepted academic practice. No use, distribution or reproduction is permitted which does not comply with these terms.
*Correspondence: Li Chen, chenliqfkk@163.com