- 1Department of Joint Sports Medicine, The Affiliated Taian City Central Hospital of Qingdao University, Taian, China
- 2Department of Pathology, The Second Affiliated Hospital of Shandong First Medical University, Taian, China
- 3Neurointensive Care Unit, The Second Affiliated Hospital of Shandong First Medical University, Taian, China
- 4Department of Orthopedics, The Second Affiliated Hospital of Shandong First Medical University, Taian, China
This study aimed to explore the potential molecular pathways and targets of Alzheimer's disease leading to osteoporosis using bioinformatics tools. The Alzheimer's and osteoporosis microarray gene expression data were retrieved from the Gene Expression Omnibus, and differentially expressed genes in the blood microenvironment related to Alzheimer's disease and osteoporosis were identified. The intersection of the three datasets (GSE97760, GSE168813, and GSE62402) was used to obtain 21 co-expressed targets in the peripheral blood samples in patients with Alzheimer's disease and osteoporosis. Based on the degree algorithm, the top 10 potential core target genes related to these diseases were identified, which included CLEC4D, PROK2, SIGLEC7, PDGFB, PTCRA, ECH1, etc. Two differentially expressed mRNAs, Prokineticin 2 (PROK2) and three colony-stimulating factor 3 (CSF3), were screened in the GSE62402 dataset associated with osteoporosis. Protein–protein rigid docking with ZDOCK revealed that PROK2 and CSF3 could form a stable protein docking model. The interaction of PROK2 and CSF3, core genes related to osteoporosis inflammation, plays an important role in the mechanism of osteoporosis in patients with Alzheimer's. Therefore, abnormalities or alterations in the inflammatory pathways in the peripheral blood samples of Alzheimer's patients may affect the course of osteoporosis.
Introduction
Osteoporosis (OP) is a bone metabolic disease that is common and highly prevalent in the elderly population (1). The prevalence of OP in the elderly increases with age (2). OP reduces bone strength and increases the risk of fracture in these patients (3, 4). Globally, osteoporotic fractures are an economic burden on society and the patients' families. They are also associated with high disability and mortality rates in elderly patients, which raise serious concerns about their health in today's aging society (5–8).
Alzheimer's disease (AD), which is yet another concern in the elderly, has been found to have a high incidence in the elderly along with OP. Previous studies have shown a prevalence of osteoporosis of 27% in patients with Alzheimer's disease, compared to 16% in residents without dementia (9). Beta-amyloid, APOE4, vitamin K, and vitamin D may be important proteins that interconnect AD and OP (10). Vitamin deficiency significantly increases AD risk. Interestingly, vitamin levels within a certain range positively correlate with cognitive performance (11–13). Also, alterations in vitamin D levels in the serum in middle-aged and elderly populations are associated with decreased bone mineral density (14). The AD mouse model, APP/PS1 transgenic mice, had significantly different bone microarchitecture and bone density parameters compared to wild-type mice and was more susceptible to OP (15). In vitro and in vivo studies in AD transgenic mouse models showed an enhanced amyloid beta (Aβ) peptide expression in bone tissue. Furthermore, an increase in Aβ peptide levels induces changes in bone mineral density, affecting the balance between bone formation and bone resorption, leading to OP (16). In addition, the Wnt/β-catenin signaling pathway plays an important role in AD and OP pathogenesis due to its role in inflammation (17, 18). Therefore, it is tempting to postulate a correlation between AD and OP. Patients with AD are cognitively impaired and prone to physical injuries. It is important to understand how AD regulates OP in patients to prevent OP occurrence and its treatment in patients with AD.
With the advancement in bioinformatics and high-throughput sequencing, it is now possible to screen the differentially expressed genes (DEGs) using microarray gene expression profiling (19–23). Publicly available databases and repositories that store information on gene expression, microarrays, and clinical samples can help understand the underlying mechanism of the disease and screen potential molecular targets quickly and efficiently before their use in clinical settings (24–26). This study aimed to investigate the molecular mechanism of OP in patients with AD using data retrieved from the Gene Expression Omnibus (GEO) database. The potential molecular pathways and biological processes associated with OP in patients with AD were explored using bioinformatics tools. Finally, we identified key targets for preventing and treating OP in patients with AD. This will provide valuable insights into understanding the pathogenesis and progression of OP in patients with AD.
Materials and methods
Target gene identification
The gene expression microarray data on “Alzheimer's disease” and “osteoporosis” were retrieved from the GEO database (https://www.ncbi.nlm.nih.gov/geo/). The data were screened using the following criteria: (i) keywords “Alzheimer's disease,” “osteoporosis,” (ii) peripheral blood, and (iii) human. The expression matrix data were corrected and normalized using the Bioconductor R package (R version 4.0.4). The differentially expressed mRNAs in the peripheral blood samples of patients with “AD” and “OP,” that is, the differentially expressed genes (DEGs) associated with AD and OP, were found in compared to normal healthy adults. The DEGs between the two groups were calculated using the linear models for the microarray data (limma package), with the screening criteria of P < 0.05 and absolute value of fold change ≥ 1.41 (|log2 FC| ≥ 0.50). We used the statistical tests built into the ggpubr package for statistical testing.
Screening and co-expression of differential genes in AD and OP and PPI network construction
Using the Venn R package, Venn graphs were created by intersecting AD-related and OP-related DEGs. The Search Tool for the Retrieval of Interacting Genes/Proteins database (https://string-db.org/) was used to construct the protein–protein interaction (PPI) network and generate PPI relationship data. The PPI network model was further visualized by Cytoscape 3.7.2. The PPI network was topologically analyzed according to degree values to screen for the core target proteins.
Gene ontology functional analysis and KEGG pathway enrichment
The clusterProfiler, an R package, was used to perform Gene Ontology (GO) and Kyoto Encyclopedia of Genes and Genomes (KEGG) enrichment analysis on AD-OP-related DEGs. The species was set to human for this analysis. The signaling pathways were mapped using the “Pathview: an R/Bioconductor package.”
Establishment of OP-inflammatory-related gene expression matrix
The gene expression matrix of the OP transcriptome profile was established with the inflammatory response-related genes extracted from the Gene Set Enrichment Analysis (GSEA) database as previous researches (27–29). The differentially expressed mRNAs between the OP group and normal healthy groups were calculated using the limma package. The “heatmap” package was used to construct maps of gene expression and cluster the DEGs.
Relative expression of core target genes
The microarray data of the OP-related gene expression matrix were retrieved from GEO, and the expression of core DEGs in each sample was derived based on the core targets obtained from the pre-screening. The “ggpubr package” was used to analyze the relative expression of the core targets in the OP expression data. P < 0.05 was considered statistically significant. A box plot of the relative expression of the core targets was plotted (R version 4.0.4).
GO and KEGG enrichment analysis of OP-inflammation-related genes
GO and KEGG pathway enrichment analysis of OP-inflammation-related genes were done using the Scatterplot3d: 3D graphics, clusterProfiler in R package software, and Perl software package.
Molecular docking to validate the interactions between inflammatory proteins
Rigid protein–protein docking (ZDOCK) was performed between inflammatory proteins to study the reciprocal relationships. The PDB format of the protein structural domain was downloaded from the Protein Data Bank PDB database (http://www.rcsb.org/). The protein structure was imported into Discovery Studio 2019 software to dehydrate and dephosphorylate the proteins. The upstream protein of the inflammatory pathway was set as the receptor protein, and the downstream protein was selected as the ligand–protein. The angular step size was set to 15°. The ZDOCK module was run to identify the docking site and calculate the ZDOCK Score. When molecular dynamics simulation (MDS) finds the docking site, the two form a stable docking (30–33). The results of protein–protein molecular docking are shown in 2D format.
Results
Screening for disease targets
Based on the keywords used and screening criteria set, nine patients with AD and 10 normal healthy individuals from the GSE97760 dataset retrieved from GEO were included in the study. A total of 7,370 differentially expressed mRNAs, of which 4,003 upregulated and 3,367 downregulated mRNAs, were obtained. In the GSE168813 dataset, five patients with AD and 10 normal healthy individuals were included in the study. In this dataset, 499 differentially expressed mRNAs were identified, of which 236 mRNAs were upregulated, and 263 mRNAs were downregulated. In the GSE62402 dataset, five OP patients and five normal healthy individuals were included in the study, and 110 differentially expressed mRNAs (94 upregulated and 16 downregulated mRNAs) were obtained. The heat map generated is shown in Figures 1A–C. The transcriptome differential expression data were represented by constructing a volcano map, as shown in Figures 1D–F.
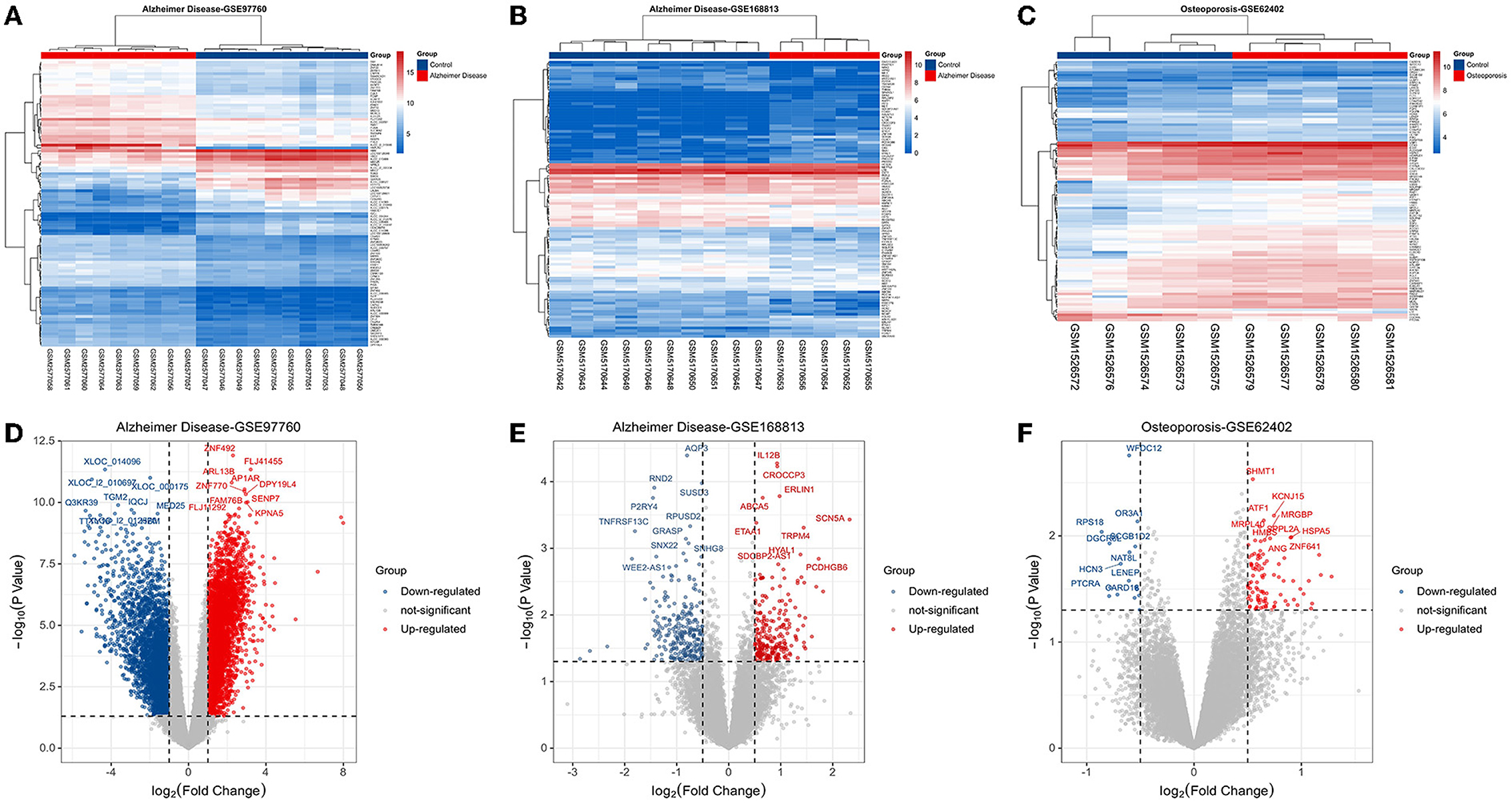
Figure 1. Disease target screening. (A) Heat map of differentially expressed genes in GSE97760; (B) heat map of differentially expressed genes in GSE168813; (C) heat map of differentially expressed genes in GSE62402; (D) volcano map of differentially expressed genes in GSE97760; (E) volcano map of differentially expressed genes in GSE168813; (F) volcano map of differentially expressed genes in GSE62402.
Detection of AD-OP-related DEGs in peripheral blood and construction of PPI networks
Twenty-one AD-OP-related DEGs were obtained from the intersection of the DEGs of the three microarray datasets (Figure 2). The AD-OP-target gene network was constructed by Cytoscape software (Figures 3A–C). Protein–protein interaction of the AD-OP-related DEGs was constructed using Cytoscape software. The top 10 potential core target proteins (Figure 3D), including CLEC4D, PROK2, SIGLEC7, PDGFB, PTCRA, and ECH1, were obtained using the CytoHubba plugin in Cytoscape software based on degree size screening (34).
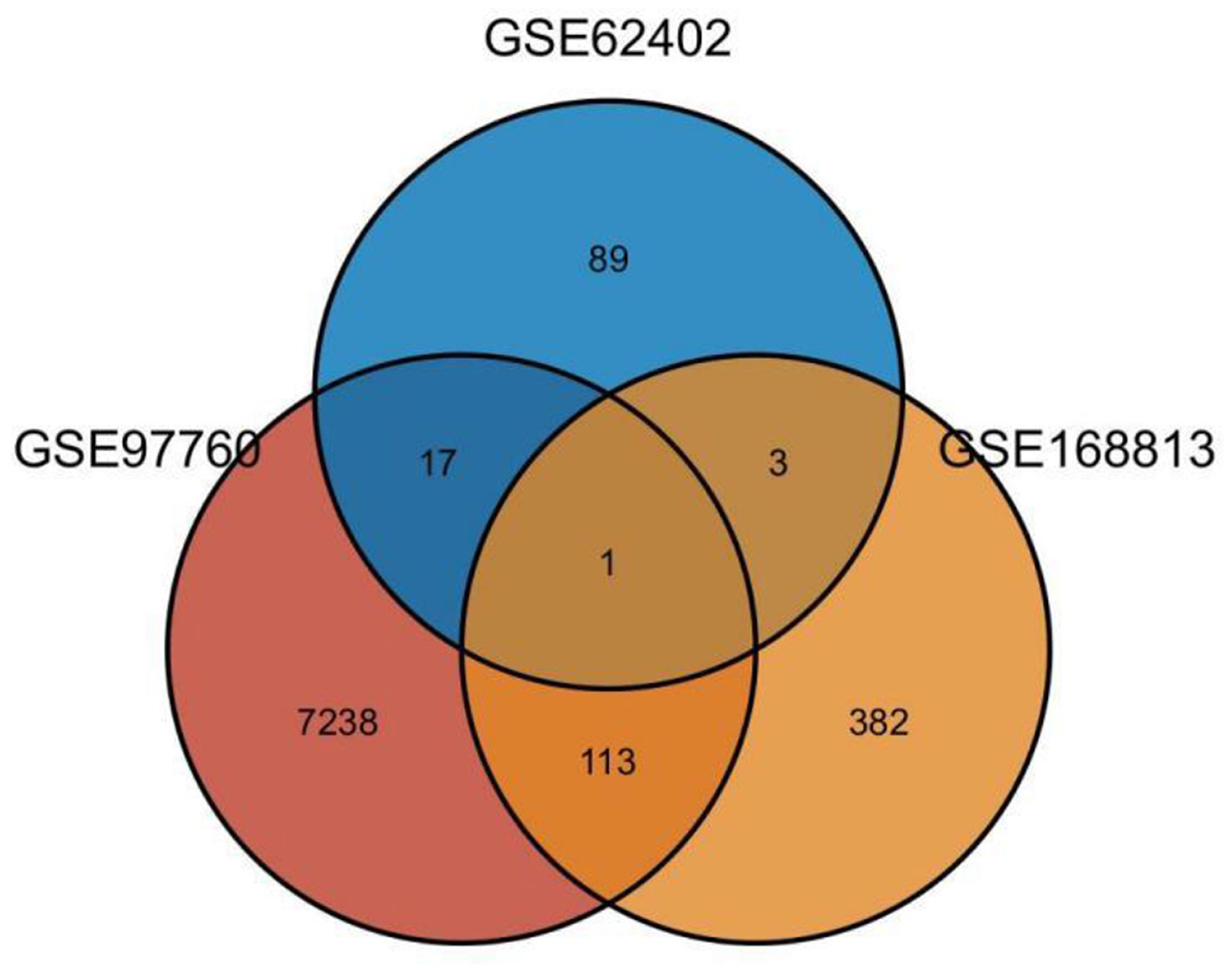
Figure 2. Venn diagram of differential co-expressed genes in Alzheimer's disease and osteoporosis in the blood microenvironment.
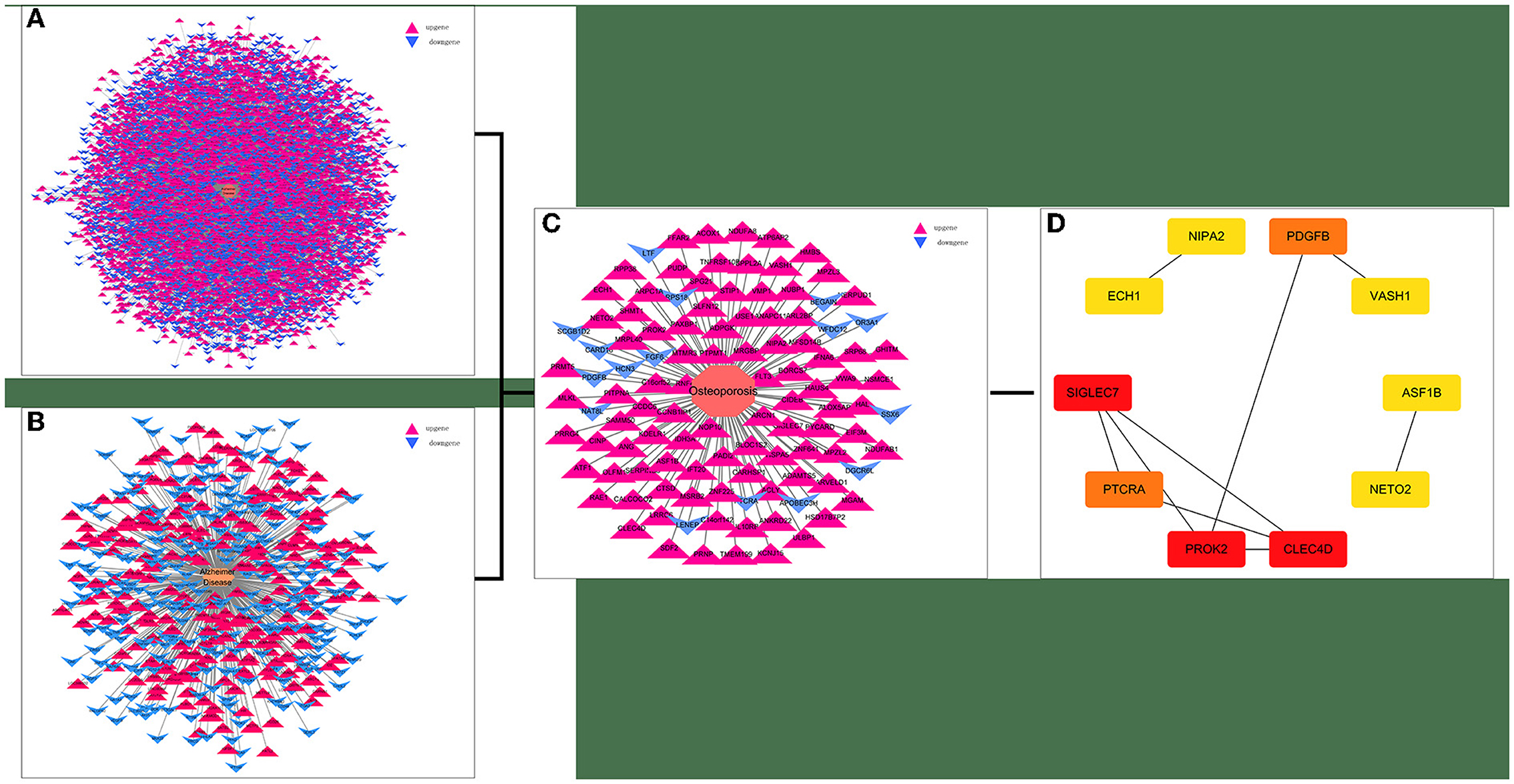
Figure 3. Screening for AD-OP-related DEGs in peripheral blood and construction of PPI networks. (A,B) GSE168813 differentially expressed gene interaction network; (C) GSE62402 differentially expressed gene interaction network; (D) top 10 potential core target genes based on degree values.
Results of the GO and KEGG enrichment analysis
The biological processes (BP) associated with the 21 AD-OP-related DEGs were regulation of calcium ion import, endothelial cell proliferation, and inositol phosphate-mediated signaling (Figure 4A). The related cell compositions (CC) mainly included BLOC-1 complex, eukaryotic 48S preinitiation complex, and eukaryotic translation initiation factor 3 complex (Figure 4B). The related molecular functions (MF) mainly enriched were glutamate receptor binding, superoxide-generating NADPH oxidase activator, and G protein-coupled glutamate receptor binding (Figure 4C). Figure 4D shows the GO enrichment features. KEGG pathway enrichment analysis shows that pathways like transcriptional dysregulation in cancer, ferroptosis, porphyrin metabolism, and other immune-related signaling pathways (Figure 5A) were associated with 21 AD-OP-related DEGs in peripheral blood. Furthermore, AD-OP-related DEGs in peripheral blood function were closely related to the ferroptosis signaling pathway (Figure 5B).
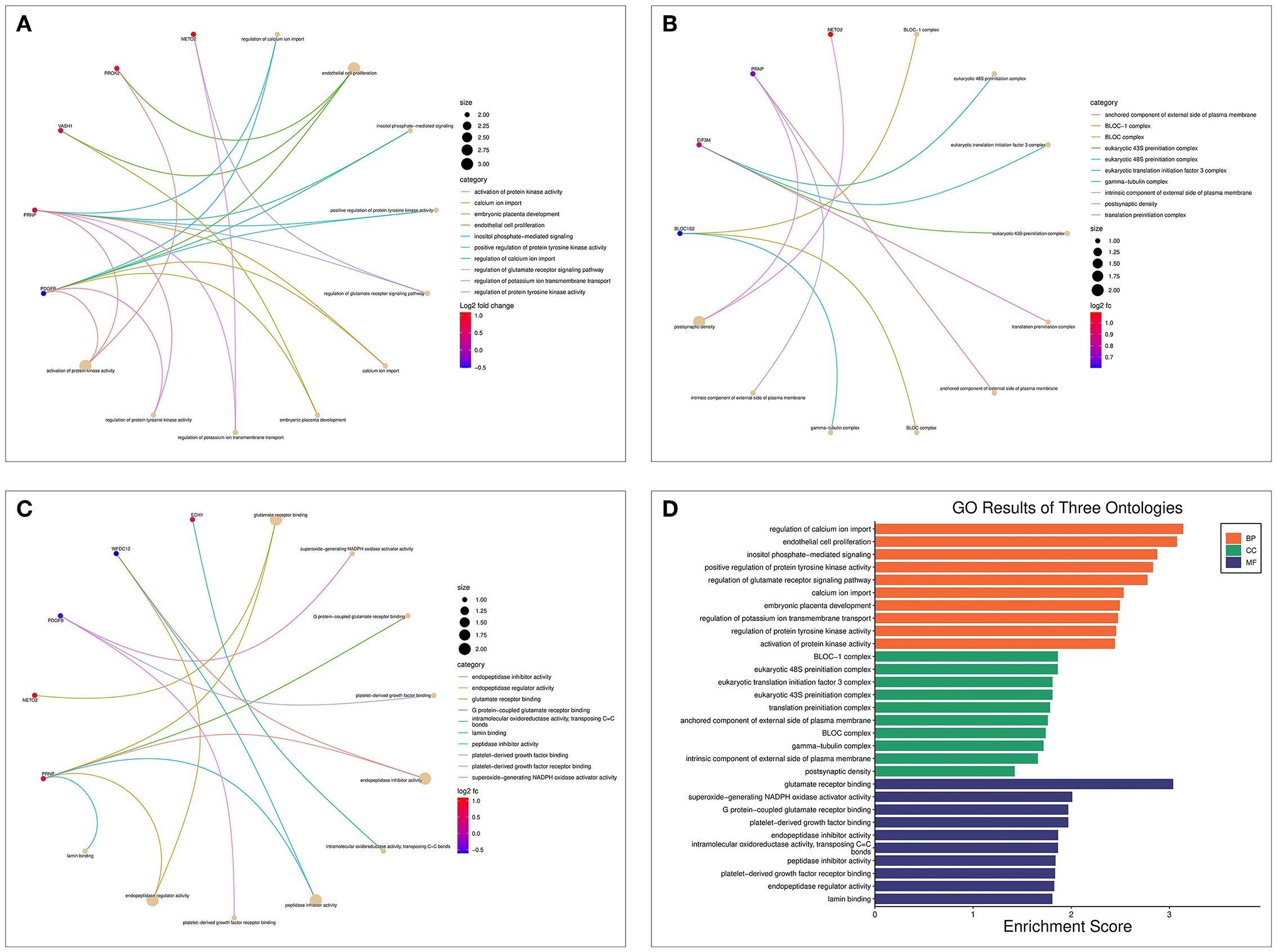
Figure 4. Results of the GO enrichment analysis for AD-OP-related DEGs. (A) Chord diagram of biological processes functional analysis; (B) chord diagram of cell component functional analysis; (C) chord diagram of molecular function functional analysis; (D) histogram of GO enrichment analysis.
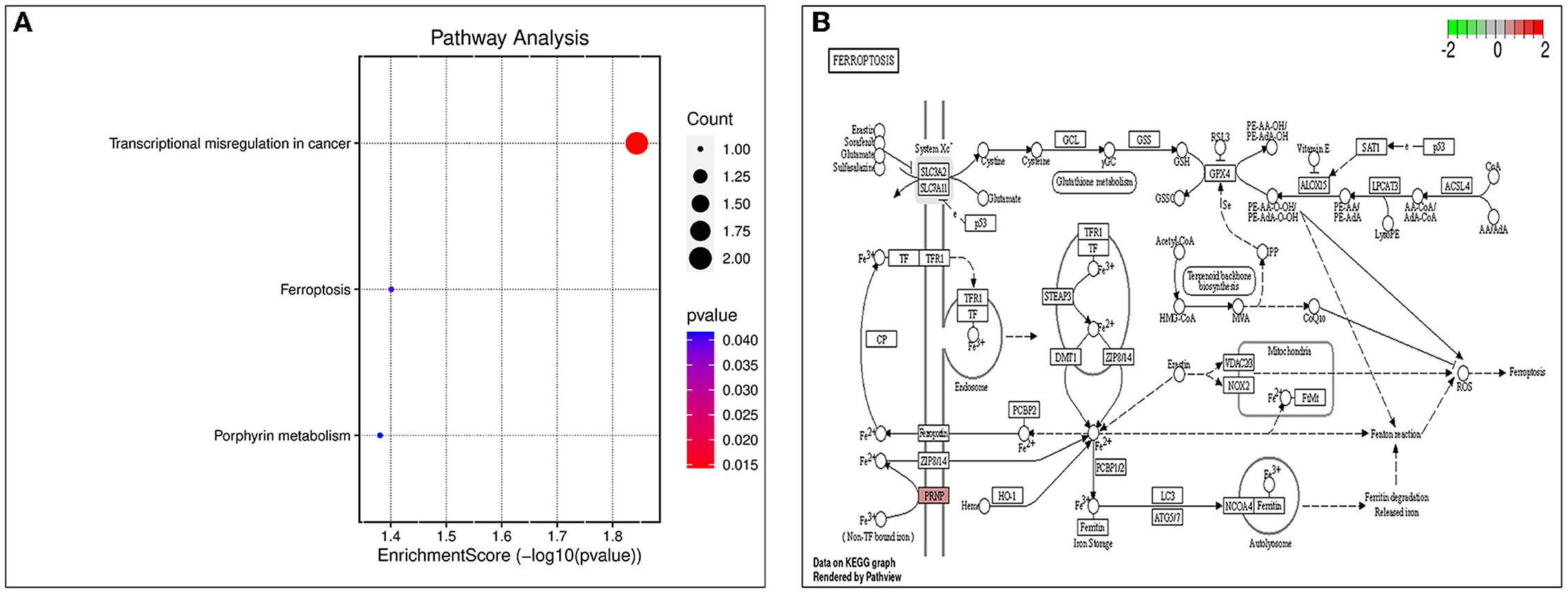
Figure 5. Results of KEGG enrichment analysis. (A) KEGG enrichment analysis bubble chart; (B) ferroptosis signaling pathway.
Establishment of OP-inflammatory response-related gene expression matrix
The gene set related to the inflammatory response was downloaded from GSEA. The OP-related GSE62402 dataset was retrieved from GEO based on the pre-set filters. R software was used to organize and analyze the metabolomics-related expression matrix. According to the screening criteria set earlier, two differentially expressed mRNAs were identified, PROK2 was upregulated, and CSF3 was downregulated. Figure 6 shows a heat map of the OP-inflammatory response-related gene expression matrix.
Relative expression of the core target genes associated with OP inflammation
The core OP-inflammation-related genes PROK2 and CSF3 were obtained by comprehensive differential expression analysis. The relative expression of PROK2 and CSF3 in OP was analyzed. The relevant expression profile of PROK2 and CSF3 in OP patients was downloaded from GEO, analyzed by the ggpubr package, and visualized using the box expression map (Figures 7A,B). The results showed that PROK2 was highly expressed in peripheral blood OP patients compared to normal healthy individuals and the difference was statistically significant (P < 0.01). Furthermore, compared to normal healthy individuals, there was a significant reduction in CSF3 expression in peripheral blood of OP patients (P < 0.01).
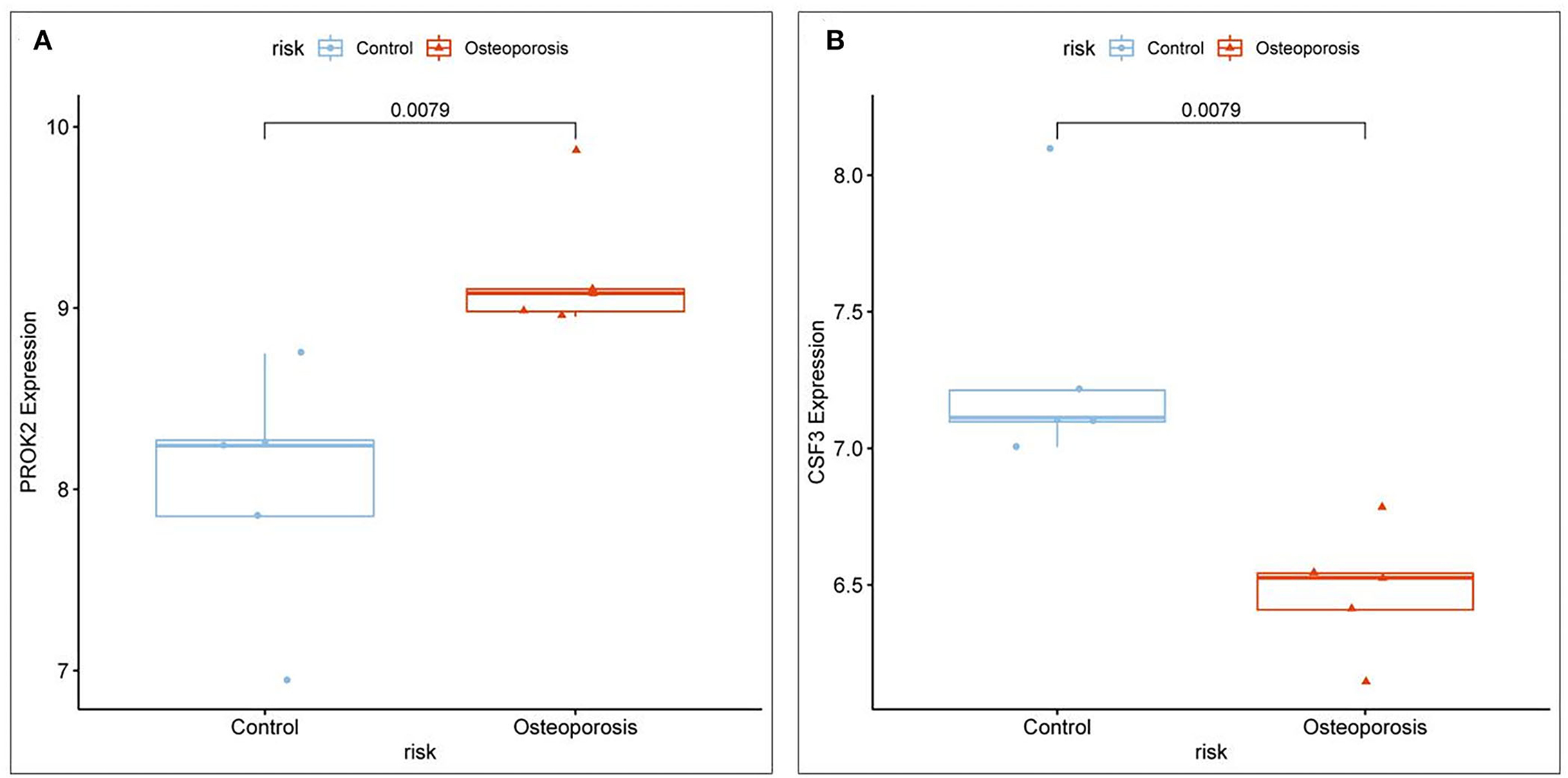
Figure 7. Relative expression levels of core targets involved in OP inflammation. (A) PROK2 relative expression; (B) CSF3 relative expression.
GO and KEGG enrichment analysis results of OP-inflammation-related genes
Enrichment analysis of the two OP-inflammatory response-related genes enriched BP, such as the regulation of actin cytoskeleton reorganization, smooth muscle contraction, and granulocyte differentiation. Their molecular functions enriched were growth factor receptor binding, growth factor activity, and cytokine activity (Figures 8A–D). The KEGG pathway enrichment analysis found that their functions are mainly associated with malaria, IL-17 signaling pathway, hematopoietic cell lineage, JAK-STAT signaling pathway, and COVID-19 (Figures 8E,F).
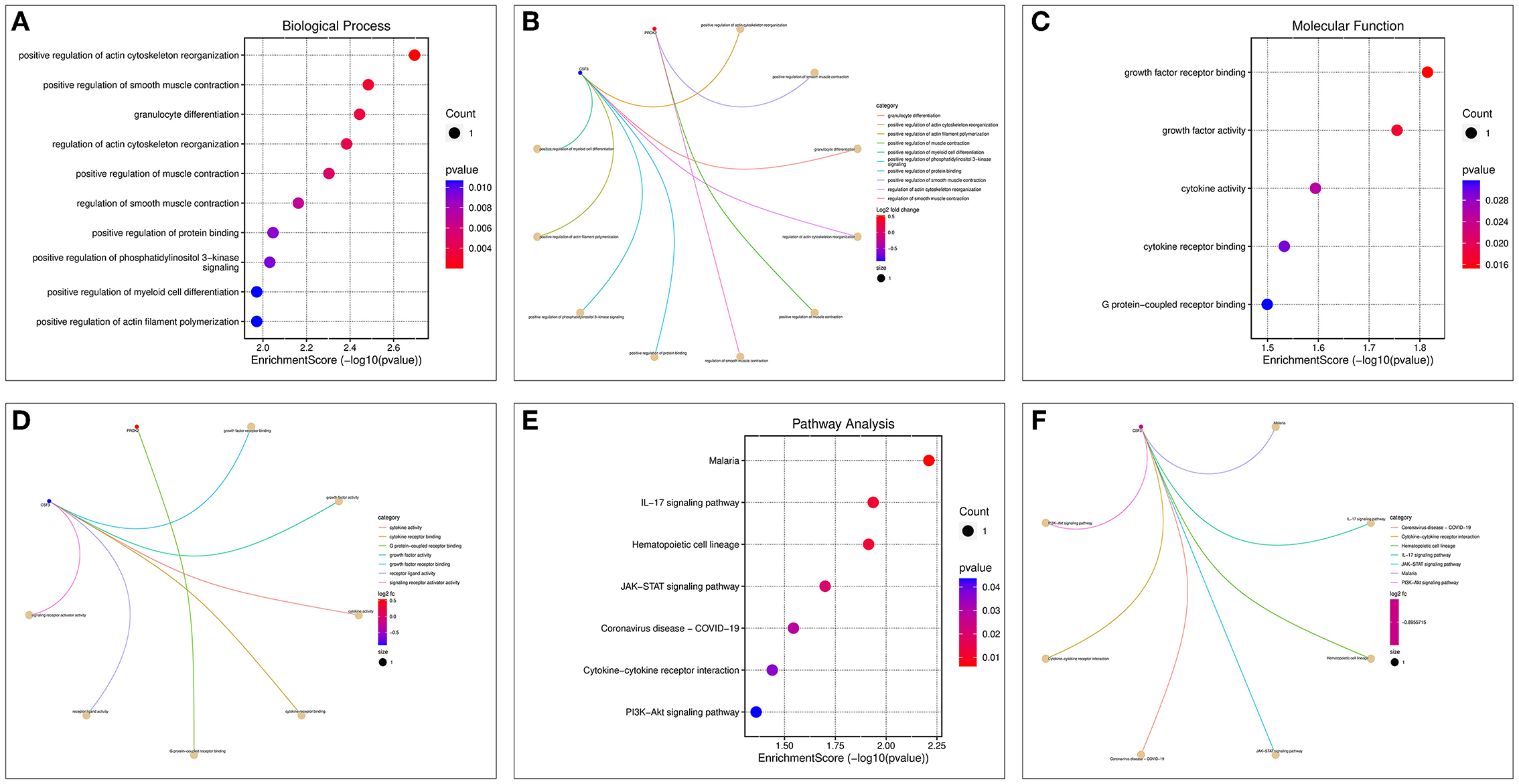
Figure 8. GO and KEGG enrichment analysis of OP-inflammation-related genes. (A) BP enrichment bubble diagram; (B) BP enrichment arc diagram; (C) MF enrichment bubble diagram; (D) MF enrichment arc diagram; (E) KEGG enrichment bubble diagram; (F) KEGG enrichment arc diagram.
Molecular docking of inflammatory proteins
The 3D structures of the 1IMT structural domain of PROK2 protein and the 2D9Q structural domain of CSF3 protein were downloaded from the PDB database and exported in PDB format. The ZDOCK module of Discovery Studio 2019 software was used to rigidly dock PROK2 protein to CSF3 protein. The ZDOCK Score values and their best pose interaction were calculated, as shown in Table 1. The ZDOCK Score of the 1IMT domain of PROK2 protein and the 2D9Q docking model of CSF3 protein was −85.085. The 1IMT domain of PROK2 proteins forms hydrogen bond links with amino acid sites such as B:ARG167:NH1—A:ASP109:OD1, B:ARG167:NH2—A:ASP112:OD1, B:ARG288:NH2—A:GLU19:OE1, A:HOH177:O—A:PRO65:O, and other amino acid sites, whereas A:LYS16:NZ—B:ASP197:O D1, A:LYS16:NZ—B:ASP200:OD1, B:ARG167:NH1—A:ASP112:OD2, B:ARG288:NH1—A:GLU19:OE2, A:LEU15—B:LEU291, and other amino acid sites form electrostatic interactions and water transport bonds. Comprehensive analysis revealed that proteins PROK2 and CSF3 formed a stable protein docking model. Figure 9 demonstrates two-dimensional molecular docking constructed using Discovery Studio 2019 software.
Discussion
Gene expression microarray datasets on Alzheimer's disease and osteoporosis patients' peripheral blood samples were retrieved from the GEO database. The results revealed that two dysregulated proteins, PROK2 and CSF3, were associated with the occurrence of OP in patients with AD. Using rigid protein–protein docking by ZDOCK confirmed that the two proteins form a stable protein docking model, suggesting that the interaction between the two proteins plays an important role in the occurrence of OP in patients with AD.
Prokineticin 2 (PROK2) is expressed throughout the central nervous system (35). As a new family of chemokine-like molecules, they are involved in various physiological and pathological processes, including nerve and blood vessel regeneration, pain, inflammation, and neuroinflammation (36–38). A study confirmed that PROK2 mediates harmful brain injuries (39). In AD, PROK2 maintains a state of neuroinflammation and causes neurotoxicity (35). Studies show the involvement of PROK2 in Aβ-induced toxicity, as Aβ peptides increase PROK2 expression in AD, representing a new class of pathological markers in AD animal models (40–42). Consistent with the previous studies, our results show that PROK2 expression was upregulated in patients with AD. Furthermore, the PROK2 expression was not only associated with inflammatory responses in the blood samples of patients with AD but also was a core gene associated with inflammation in OP.
Few studies have shown the PROK2 expression and functions in OP. Interestingly, previous studies have demonstrated the involvement of PROK2 in Aβ-mediated toxicity and have a positive correlation with Aβ peptides. It also alters the bone mineral density, which may affect the bone formation and resorption balance, leading to the development of OP (16, 42). Prokineticin receptor 2 (PROKR2) is the PROK2 and G protein-coupled receptor (GPCR). In addition, GPCRs affect bone metabolism by influencing the cytokines and signaling pathways that regulate osteoblasts (OB) and osteoclasts (OC) (43). In addition, PROK2 is also closely associated with gastrointestinal (GI) function and GI diseases (44). Previous studies have also shown that the OP incidences were significantly higher in patients with GI diseases (45, 46). Hence, we hypothesized that PROK2 plays an important role in the development of OP. In this study, we show for the first time the upregulation of PROK2 expression in OP. Furthermore, PROK2 was a core gene associated with OP inflammation and a common gene differentially expressed between AD and OP patients. Hence, PROK2 could be potentially used as a molecular marker for predicting the occurrence of OP in patients with AD.
CSF3 is a member of the colony-stimulating factor family. Together with its receptor CSF3R, CSF3 is involved in regulating sarcomere cell production, neutrophil function, etc. (47). A study has reported that neutrophil/lymphocyte ratio could be used in predicting the occurrence of OP (48). Zhang et al. demonstrated the expression of RANKL, the osteoclast differentiation factor on the surface of neutrophils. RANKL binds to the osteoclast differentiation factor receptor, RANK, which mediates osteoclast differentiation, thereby enhancing the osteoclast activity. This disrupts bone metabolism, which reduces bone mass (49). However, the relationship between CSF3 and OP has not been established.
In this study, the expression of CSF3 was downregulated in OP as a core gene related to OP inflammation. Bone resorption is enhanced during the chronic inflammatory response, reducing the bone formation and promoting OP (50). Previous studies show that CSF3 is an anti-inflammatory cytokine that clears bacterial pathogens and modulates the inflammatory response (51). Therefore, we postulate the involvement of CSF3 in inflammation-related biological processes in the progression of OP.
The core inflammation-related genes PROK2 and CSF3 involved in OP, identified in this study, were associated with the signal transducer and activator of the transcription (STAT) pathway. The STAT pathway induces astrocyte proliferation and is activated in AD animal models and humans. Previous studies have demonstrated that reactive astrocyte proliferation is a hallmark of the AD signaling pathway (52). STAT3 induces astrocyte proliferation and is activated in human AD and animal models, and reactive astrocyte proliferation is a hallmark of AD (52). During acute inflammation and septic inflammatory conditions, CSF3 mediates STAT3-dependent upregulation of neutrophil IL-4R (53). Interestingly, an increase in STAT3 phosphorylation was observed in cells stably expressing PROKR2, which is the receptor for PROK2 (54). In addition, the STAT signaling pathway plays an important role in the pathogenesis of OP and AD. Inhibiting STAT3 phosphorylation attenuates learning and causes memory deficits in AD animal models (55). Furthermore, the STAT3 signaling pathway is involved in the progression of OP by altering osteoblast bone metabolism (43, 56). Consistent with the previous studies, the KEGG pathway enrichment analysis revealed that both genes enriched the JAK-STAT signaling pathway and pathways associated with malaria, IL-17 signaling pathway, hematopoietic cell lineage, and COVID-19. GeneCards database (https://ga.genecards.org/#results) shows that both PROK2 and CSF3 were associated with the extracellular region. Furthermore, GO analysis revealed the involvement of PROK2 and CSF3 in protein binding and their association with VEGF. In this study, we identified the combined role of PROK2 and CSF3 in the pathogenesis of AD and OP. Our results reveal those alterations in the inflammatory response pathway in the peripheral blood of patients with AD may affect the occurrence and progression of OP. The docking results show that proteins PROK2 and CSF3 could form a stable protein docking model, thus confirming the previous bioinformatics results that the interaction between the PROK2 and CSF3 could be involved in the inflammatory-related response to OP in patients with AD.
In this study, using bioinformatics analysis, we demonstrated that the proteins PROK2 and CSF3 may be involved in inflammation-related processes in the development of OP in patients with AD and confirmed stable protein interactions between them by docking, thereby verifying the reliability of predictions made by bioinformatics analysis. However, the study has a few shortcomings. The primary technique used in this study was bioinformatics analysis. Hence, further experiments validating the interaction between PROK2 and CSF3 proteins are required. The results of our study predict the role of PROK2 and CSF3 protein binding in the pathogenesis of OP in patients with AD. However, the mechanism is still unclear and needs further exploration using appropriate experiments.
Conclusion
AD-related OP may be caused by the interaction between PROK2 and CSF3, two proteins related to OP inflammation. Accordingly, abnormalities/alterations in the inflammatory response in the peripheral blood of patients with AD could influence the progression of OP. Further exploration of targets for treating OP in patients with AD will be facilitated by our study.
Data availability statement
Publicly available datasets were analyzed in this study. This data can be found at: The gene micro-array data related to Alzheimer's disease and osteoporosis were downloaded from the GEO (gene expression omnibus) database (https://www.ncbi.nlm.nih.gov/geo/): GSE97760, GSE168813, and GSE62402 dataset.
Author contributions
AW, WZ, YZ, and NH: conceptualization, methodology, software, data curation, writing the original draft preparation, and validation. AW and WZ: visualization, investigation, writing, reviewing, and editing. AW: supervision. All authors contributed to the article and approved the submitted version.
Conflict of interest
The authors declare that the research was conducted in the absence of any commercial or financial relationships that could be construed as a potential conflict of interest.
Publisher's note
All claims expressed in this article are solely those of the authors and do not necessarily represent those of their affiliated organizations, or those of the publisher, the editors and the reviewers. Any product that may be evaluated in this article, or claim that may be made by its manufacturer, is not guaranteed or endorsed by the publisher.
Abbreviations
AD, Alzheimer's disease; OP, Osteoporosis; PPI, Protein–protein interaction; GO, Gene Ontology; KEGG, Kyoto Encyclopedia of Genes and Genomes; DEGs, Differentially expressed genes.
References
1. Li G, Thabane L, Papaioannou A, Ioannidis G, Levine MAH, Adachi JD. An overview of osteoporosis and frailty in the elderly. BMC Musculoskelet Disord. (2017) 18:46. doi: 10.1186/s12891-017-1403-x
2. Vandenbroucke A-M, Luyten F, Flamaing J, Gielen E. Pharmacological treatment of osteoporosis in the oldest old. CIA. (2017) 12:1065–77. doi: 10.2147/CIA.S131023
3. Li W, Zhang S, Liu J, Liu Y, Liang Q. Vitamin K2 stimulates Mc3T3-E1 osteoblast differentiation and mineralization through autophagy induction. Mol Med Report. (2019) 19:3676–84. doi: 10.3892/mmr.2019.10040
4. Agnew AM, Dominguez VM, Sciulli PW, Stout SD. Variability of in vivo linear microcrack accumulation in the cortex of elderly human ribs. Bone Rep. (2017) 6:60–3. doi: 10.1016/j.bonr.2017.02.004
5. Tella SH, Gallagher JC. Prevention and treatment of postmenopausal osteoporosis. J Steroid Biochem Mol Biol. (2014) 142:155–70. doi: 10.1016/j.jsbmb.2013.09.008
6. Hongo M, Miyakoshi N, Shimada Y, Sinaki M. Association of spinal curve deformity and back extensor strength in elderly women with osteoporosis in Japan and the United States. Osteoporos Int. (2012) 23:1029–34. doi: 10.1007/s00198-011-1624-z
7. Snellman G, Byberg L, Lemming EW, Melhus H, Gedeborg R, Mallmin H, et al. Long-term dietary vitamin d intake and risk of fracture and osteoporosis: a longitudinal cohort study of Swedish middle-aged and elderly women. J Clin Endocrinol Metab. (2014) 99:781–90. doi: 10.1210/jc.2013-1738
8. Yoo JE, Shin DW, Han K, Kim D, Yoon JW, Lee D-Y. Association of female reproductive factors with incidence of fracture among postmenopausal women in Korea. J Am Med Assoc Netw Open. (2021) 4:e2030405. doi: 10.1001/jamanetworkopen.2020.30405
9. Weller I. Hip fractures and Alzheimer's disease in elderly institutionalized Canadians. Ann Epidemiol. (2004) 14:319–24. doi: 10.1016/j.annepidem.2003.08.005
10. Frame G, Bretland KA, Dengler-Crish CM. Mechanistic complexities of bone loss in Alzheimer's disease: a review. Connect Tissue Res. (2020) 61:4–18. doi: 10.1080/03008207.2019.1624734
11. Balion C, Griffith LE, Strifler L, Henderson M, Patterson C, Heckman G, et al. Vitamin D, cognition, and dementia: a systematic review and meta-analysis. Neurology. (2012) 79:1397–405. doi: 10.1212/WNL.0b013e31826c197f
12. Littlejohns TJ, Henley WE, Lang IA, Annweiler C, Beauchet O, Chaves PHM, et al. Vitamin D and the risk of dementia and Alzheimer disease. Neurology. (2014) 83:920–8. doi: 10.1212/WNL.0000000000000755
13. Shen L, Ji H-F. Vitamin D deficiency is associated with increased risk of Alzheimer's disease and dementia: evidence from meta-analysis. Nutr J. (2015) 14:76. doi: 10.1186/s12937-015-0063-7
14. Wei Q, Chen Z, Tan X, Su H, Chen X, He W, et al. Relation of age, sex and bone mineral density to serum 25-hydroxyvitamin D levels in Chinese women and men: 25(OH)D in healthy adults. Orthop Surg. (2015) 7:343–9. doi: 10.1111/os.12206
15. Panaro MA, Corrado A, Benameur T, Paolo CF, Cici D, Porro C. The emerging role of curcumin in the modulation of TLR-4 signaling pathway: focus on neuroprotective and anti-rheumatic properties. Int J Mol Sci. (2020) 21:2299. doi: 10.3390/ijms21072299
16. Kelly RR, Sidles SJ, LaRue AC. Effects of neurological disorders on bone health. Front Psychol. (2020) 11:612366. doi: 10.3389/fpsyg.2020.612366
17. Jia L, Piña-Crespo J, Li Y. Restoring Wnt/β-catenin signaling is a promising therapeutic strategy for Alzheimer's disease. Mol Brain. (2019) 12:104. doi: 10.1186/s13041-019-0525-5
18. Wang F, Li C, Shao J, Ma J. Sevoflurane induces inflammation of microglia in hippocampus of neonatal rats by inhibiting Wnt/β-Catenin/CaMKIV pathway. J Pharmacol Sci. (2021) 146:105–15. doi: 10.1016/j.jphs.2021.02.004
19. Feng X, Ding Y, Zhou M, Song N, Ding Y. Integrative analysis of exosomal miR-452 and miR-4713 downregulating NPY1R for the prevention of childhood obesity. Dis Markers. (2022) 2022:1–12. doi: 10.1155/2022/2843353
20. Huang H, Jiang H, Liu J, Chen J, Qiu L, Wang J, et al. Facial nerve monitoring under different levels of neuromuscular blockade with Cisatracurium besilate in parotid tumour surgery. Biomed Res Int. (2021) 2021:5655061. doi: 10.1155/2021/5655061
21. Won H-H, Kim SR, Bang OY, Lee S-C, Huh W, Ko J-W, et al. Differentially expressed genes in human peripheral blood as potential markers for statin response. J Mol Med. (2012) 90:201–11. doi: 10.1007/s00109-011-0818-3
22. Kleinrouweler CE, Van Uitert M, Moerland PD, Ris-Stalpers C, Van Der Post JAM, Afink GB. Differentially expressed genes in the pre-eclamptic placenta: a systematic review and meta-analysis. PLoS ONE. (2013) 8:e68991. doi: 10.1371/journal.pone.0068991
23. Chen Y, Sun Y, Luo Z, Chen X, Wang Y, Qi B, et al. Exercise Modifies the Transcriptional regulatory features of monocytes in Alzheimer's patients: a multi-omics integration analysis based on single cell technology. Front Aging Neurosci. (2022) 14:881488. doi: 10.3389/fnagi.2022.881488
24. Andreu-Perez J, Poon CCY, Merrifield RD, Wong STC, Yang G-Z. Big data for health. IEEE J Biomed Health Inform. (2015) 19:1193–208. doi: 10.1109/JBHI.2015.2450362
25. Dong S, Huang F, Zhang H, Chen Q. Overexpression of BUB1B, CCNA2, CDC20, and CDK1 in tumor tissues predicts poor survival in pancreatic ductal adenocarcinoma. Biosci Rep. (2019) 39:BSR20182306. doi: 10.1042/BSR20182306
26. Chen L, Yuan L, Wang Y, Wang G, Zhu Y, Cao R, et al. Co-expression network analysis identified FCER1G in association with progression and prognosis in human clear cell renal cell carcinoma. Int J Biol Sci. (2017) 13:1361–72. doi: 10.7150/ijbs.21657
27. Wu L, Su C, Yang C, Liu J, Ye Y. TBX3 regulates the transcription of VEGFA to promote osteoblasts proliferation and microvascular regeneration. PeerJ. (2022) 10:e13722. doi: 10.7717/peerj.13722
28. Xu K, Wu C, Wang Z, Wang H, Yin F, Li W, et al. Family gene expression as prognostic biomarkers for Alzheimer's disease and primary liver cancer. Comput Math Methods Med. (2021) 2021:1–15. doi: 10.1155/2021/3422393
29. Subramanian A, Tamayo P, Mootha VK, Mukherjee S, Ebert BL, Gillette MA, et al. Gene set enrichment analysis: a knowledge-based approach for interpreting genome-wide expression profiles. Proc Natl Acad Sci USA. (2005) 102:15545–50. doi: 10.1073/pnas.0506580102
30. Hildebrand PW, Rose AS, Tiemann JKS. Bringing molecular dynamics simulation data into view. Trends Biochem Sci. (2019) 44:902–13. doi: 10.1016/j.tibs.2019.06.004
31. Lu L, Kang X, Yi B, Jiang C, Yan X, Chen B, et al. Exploring the mechanism of Yiqi Qingre Ziyin method in regulating neuropeptide expression for the treatment of atrophic rhinitis. Dis Markers. (2022) 2022:1–12. doi: 10.1155/2022/4416637
32. Chen Y, Luo Z, Lin J, Qi B, Sun Y, Li F, et al. Exploring the potential mechanisms of melilotus officinalis (l) pall in chronic muscle repair patterns using single cell receptor-ligand marker analysis and molecular dynamics simulations. Dis Markers. (2022) 2022:1–11. doi: 10.1155/2022/9082576
33. Hollingsworth SA, Dror RO. Molecular dynamics simulation for all. Neuron. (2018) 99:1129–43. doi: 10.1016/j.neuron.2018.08.011
34. Chin C-H, Chen S-H, Wu H-H, Ho C-W, Ko M-T, Lin C-Y. cytoHubba: identifying hub objects and sub-networks from complex interactome. BMC Syst Biol. (2014) 8:S11. doi: 10.1186/1752-0509-8-S4-S11
35. Zuena AR, Casolini P, Lattanzi R, Maftei D. Chemokines in Alzheimer's disease: new insights into prokineticins, chemokine-like proteins. Front Pharmacol. (2019) 10:622. doi: 10.3389/fphar.2019.00622
36. Zinni M, Zuena AR, Marconi V, Petrella C, Fusco I, Giuli C, et al. Maternal exposure to low levels of corticosterone during lactation protects adult rat progeny against TNBS-induced colitis: a study on GR-mediated anti-inflammatory effect and prokineticin system. PLoS ONE. (2017) 12:e0173484. doi: 10.1371/journal.pone.0173484
37. Negri L, Ferrara N. The prokineticins: neuromodulators and mediators of inflammation and myeloid cell-dependent angiogenesis. Physiol Rev. (2018) 98:1055–82. doi: 10.1152/physrev.00012.2017
38. Negri L, Maftei D. Targeting the prokineticin system to control chronic pain and inflammation. Curr Med Chem. (2018) 25:3883–94. doi: 10.2174/0929867324666170713102514
39. Cheng MY, Lee AG, Culbertson C, Sun G, Talati RK, Manley NC, et al. Prokineticin 2 is an endangering mediator of cerebral ischemic injury. Proc Natl Acad Sci USA. (2012) 109:5475–80. doi: 10.1073/pnas.1113363109
40. Severini C, Lattanzi R, Maftei D, Marconi V, Ciotti MT, Petrocchi Passeri P, et al. Bv8/prokineticin 2 is involved in Aβ-induced neurotoxicity. Sci Rep. (2015) 5:15301. doi: 10.1038/srep15301
41. Caioli S, Severini C, Ciotti T, Florenzano F, Pimpinella D, Petrocchi Passeri P, et al. Prokineticin system modulation as a new target to counteract the amyloid beta toxicity induced by glutamatergic alterations in an in vitro model of Alzheimer's disease. Neuropharmacology. (2017) 116:82–97. doi: 10.1016/j.neuropharm.2016.12.012
42. Maftei D, Ratano P, Fusco I, Marconi V, Squillace S, Negri L, et al. The prokineticin receptor antagonist PC1 rescues memory impairment induced by β amyloid administration through the modulation of prokineticin system. Neuropharmacology. (2019) 158:107739. doi: 10.1016/j.neuropharm.2019.107739
43. Luo J, Sun P, Siwko S, Liu M, Xiao J. The role of GPCRs in bone diseases and dysfunctions. Bone Res. (2019) 7:19. doi: 10.1038/s41413-019-0059-6
44. Kurebayashi H, Goi T, Shimada M, Tagai N, Naruse T, Nakazawa T, et al. Prokineticin 2 (PROK2) is an important factor for angiogenesis in colorectal cancer. Oncotarget. (2015) 6:26242–51. doi: 10.18632/oncotarget.4385
45. Locantore P, Del Gatto V, Gelli S, Paragliola RM, Pontecorvi A. The interplay between immune system and microbiota in osteoporosis. Mediators Inflamm. (2020) 2020:1–8. doi: 10.1155/2020/3686749
46. Stuckey BGA, Mahoney LA, Dragovic S, Brown SJ. Celiac disease and bone health: is there a gap in the management of postmenopausal osteoporosis? Climacteric. (2020) 23:559–65. doi: 10.1080/13697137.2020.1816957
47. Zhang H, Coblentz C, Watanabe-Smith K, Means S, Means J, Maxson JE, et al. Gain-of-function mutations in granulocyte colony–stimulating factor receptor (CSF3R) reveal distinct mechanisms of CSF3R activation. J Biol Chem. (2018) 293:7387–96. doi: 10.1074/jbc.RA118.002417
48. Gao K, Zhu W, Liu W, Ma D, Li H, Yu W, et al. The predictive role of monocyte-to-lymphocyte ratio in osteoporosis patient. Medicine. (2019) 98:e16793. doi: 10.1097/MD.0000000000016793
49. Zhang P, Pan L, Luo Z, Zhao H, Cai S. Interrelationship of circulating matrix metalloproteinase-9, TNF-α, and OPG/RANK/RANKL systems in COPD patients with osteoporosis. J Chronic Obst Pulmonary Dis. (2013) 10:650–6. doi: 10.3109/15412555.2013.813928
50. Sato K, Suematsu A, Okamoto K, Yamaguchi A, Morishita Y, Kadono Y, et al. Th17 functions as an osteoclastogenic helper T cell subset that links T cell activation and bone destruction. J Exp Med. (2006) 203:2673–82. doi: 10.1084/jem.20061775
51. Yeganegi M, Leung CG, Martins A, Kim SO, Reid G, Challis JRG, et al. Lactobacillus rhamnosus GR-1 stimulates colony-stimulating factor 3 (Granulocyte) (CSF3) output in placental trophoblast cells in a fetal sex-dependent manner1. Biol Reprod. (2011) 84:18–25. doi: 10.1095/biolreprod.110.085167
52. Reichenbach N, Delekate A, Plescher M, Schmitt F, Krauss S, Blank N, et al. Inhibition of Stat3-mediated astrogliosis ameliorates pathology in an Alzheimer's disease model. EMBO Mol Med. (2019) 11:e9665. doi: 10.15252/emmm.201809665
53. Panda SK, Wigerblad G, Jiang L, Jiménez-Andrade Y, Iyer VS, Shen Y, et al. IL-4 controls activated neutrophil FcγR2b expression and migration into inflamed joints. Proc Natl Acad Sci USA. (2020) 117:3103–13. doi: 10.1073/pnas.1914186117
54. Lattanzi R, Maftei D, Vincenzi M, Fullone MR, Miele R. Identification and characterization of a new splicing variant of prokineticin 2. Life. (2022) 12:248. doi: 10.3390/life12020248
55. Choi M, Kim H, Yang E-J, Kim H-S. Inhibition of STAT3 phosphorylation attenuates impairments in learning and memory in 5XFAD mice, an animal model of Alzheimer's disease. J Pharmacol Sci. (2020) 143:290–9. doi: 10.1016/j.jphs.2020.05.009
Keywords: Alzheimer's disease, osteoporosis, PROK2, CSF3, bio-informatics analysis, biomarkers, neurovascular
Citation: Zhang W, Zhang Y, Hu N and Wang A (2022) Alzheimer's disease-associated inflammatory pathways might contribute to osteoporosis through the interaction between PROK2 and CSF3. Front. Neurol. 13:990779. doi: 10.3389/fneur.2022.990779
Received: 10 July 2022; Accepted: 03 August 2022;
Published: 20 September 2022.
Edited by:
Jun Xu, Capital Medical University, ChinaReviewed by:
Xiaofan Jiang, Fourth Military Medical University, ChinaJin Huang, Aviation General Hospital of China Medical University, China
Chen Chen, The First Affiliated Hospital of Soochow University, China
Copyright © 2022 Zhang, Zhang, Hu and Wang. This is an open-access article distributed under the terms of the Creative Commons Attribution License (CC BY). The use, distribution or reproduction in other forums is permitted, provided the original author(s) and the copyright owner(s) are credited and that the original publication in this journal is cited, in accordance with accepted academic practice. No use, distribution or reproduction is permitted which does not comply with these terms.
*Correspondence: Anying Wang, d2FuZ2FueWluZzAwOCYjeDAwMDQwOzE2My5jb20=