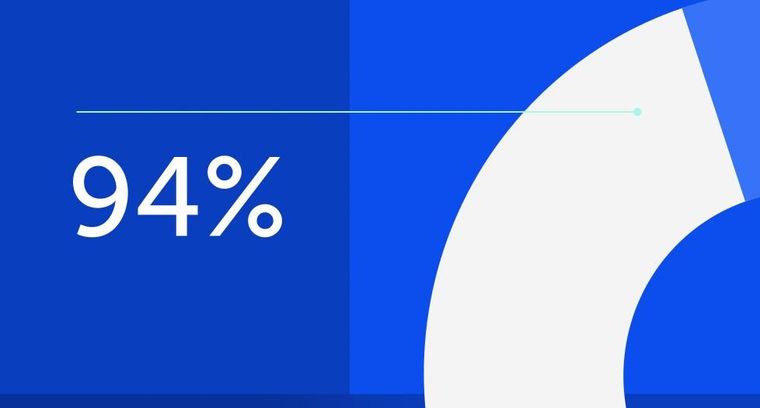
94% of researchers rate our articles as excellent or good
Learn more about the work of our research integrity team to safeguard the quality of each article we publish.
Find out more
ORIGINAL RESEARCH article
Front. Neurol., 28 July 2022
Sec. Neurotrauma
Volume 13 - 2022 | https://doi.org/10.3389/fneur.2022.949091
Background: Hyperglycemia is an independent risk factor for the poor prognosis in patients with traumatic brain injury (TBI), and stress-induced impaired insulin function is the major factor of hyperglycemia in non-diabetic patients with TBI. Several types of research suggested that insulin resistance (IR) is related to the poor prognosis of neurocritical ill patients; here we focused on the role of IR in non-diabetic patients after TBI.
Methods: We performed a prospective observational study with the approval of the Ethics Committee of our institute. IR was accessed via the update Homeostasis Model Assessment (HOMA2) of IR, a computer-calculated index by glucose and insulin level. HOMA2 ≥ 1.4 was considered as the threshold of IR according to the previous studies. The glycemic variability (GV) indices were calculated by fingertip blood glucose concentration at an interval of 2 h within 24 h to explore the relationship between IR and GV. The outcome was the 6-month neurological outcome evaluated with the Glasgow outcome scale.
Results: A total of 85 patients with isolated moderate-to-severe TBI (admission GCS ≤ 12) were finally included in our study, 34 (40%) were diagnosed with IR with HOMA2 ≥ 1.4. After propensity score matching (PSM), 22 patients in IR group were matched to 34 patients in non-IR group. Patients with IR suffered increased systemic glycemic variation after isolated moderate-to-severe TBI. IR was a significant factor for the poor prognosis after TBI (OR = 3.25, 95% CI 1.03–10.31, p = 0.041).
Conclusions: The IR estimated by HOMA2 was associated with greater GV and an unfavorable outcome after isolated moderate-to-severe TBI. Ameliorating impaired insulin sensitivity may be a potential therapeutic strategy for the management of TBI patients.
As suggested by the study of the International Mission for Prognosis and Clinical Trial design in TBI (IMPACT), hyperglycemia is widely recognized as a significant prognostic factor of poor prognosis after brain injuries (1). The previous studies further confirmed that glycemic normalization by insulin therapy significantly improves the prognosis of patients with traumatic brain injury (TBI), although there is still controversy over intensive and conventional insulin therapy (2, 3).
Diabetes is one of the most important factors of hyperglycemia and leads to poor prognosis after TBI (4). However, hyperglycemia is also common in non-diabetic TBI patients and is suggested to have a greater impact on poor prognosis with over 50–60% increased mortality rate (5, 6). Moreover, the adverse effect of hyperglycemia in non-diabetic patients is more significant than that in diabetic patients after TBI (6).
Stress-induced impaired insulin function is one of the major factors of hyperglycemia in TBI patients besides diabetes (7). Insulin and insulin signaling have been found to play important roles in both peripheral and central functions (8, 9). Impaired insulin sensitivity may lead to greater glycemic variation and metabolic dysfunction, which was significantly associated with poorer functional outcome in neurocritical ill patients (10–12). Insulin resistance (IR) is also suggested to be associated with a variety of neurological disorders by its effect on the central nervous system (CNS) (13–15).
Several types of research suggested that IR is related to the poor prognosis of neurocritical ill patients (16, 17). Mowery et al. reported IR is still associated with poor prognosis despite tight glucose control in critically ill patients (18). However, there is no study focused on the role of IR in non-diabetic patients after TBI. In this single-center prospective observational study, we explored the incidence of IR and whether greater glycemic variability and poor prognosis after isolated moderate-to-severe TBI are related to IR in non-diabetic patients. We hypothesized IR was associated with increased systemic glycemic variation and would be a significant factor of poor prognosis among non-diabetic patients with TBI.
This was a prospective pilot observational study to explore the incidence of IR and whether IR was associated with increased systemic glycemic variation and poor prognosis in non-diabetic patients with isolated moderate-to-severe TBI (Figure 1). Approval was obtained from the Ethics Committee of Jiangyin Hospital Affiliated to Nantong University before initiation of the study (No. 2016012).
Patients who met the following criteria were consecutively recruited to our study: (1) patients with admission Glasgow Outcome Scale (GCS) score ≤ 12; (2) patients with no history of diabetes; (3) patients with normal BMI, 18.5 ≤ BMI < 24; (4) patients with isolated TBI, Abbreviated Injury Scale score ≤ 1 except the head. Candidates with severe chronic diseases or malignant tumors, under the age of 18, and with admission HbA1c ≥ 6.5% were excluded. Informed consent was signed by close relatives of all enrolled patients. Body Mass Index (BMI) was calculated according to the patient's height and weight before injury. The level of glycated hemoglobin (measured by HbA1c) was detected at admission, and further analyzed between groups.
For each study participant, fasting blood glucose (FBG) and fasting insulin (FIns) were collected in the first 24 h after admission. FBG and Fins were used to estimate IR via Homeostasis Model Assessment (19) (HOMA, University of Oxford, Oxon, UK). In our study, the updated HOMA, HOMA2 (HOMA2 Calculator software version 2.2.3) was used to estimate IR of the participants, and the calculator is available at: https://www.dtu.ox.ac.uk. The accepted input range for HOMA2 Calculator was shown as below: plasma glucose 3.5–25.0 mmol/L and plasma insulin 20–400 pmol/L. Candidates with unaccepted FBG and FIns values for HOMA2 Calculator were also excluded. According to previous studies, IR was defined as HOMA2 ≥ 1.4 (20).
During the first 24 h after admission, the fingertip blood glucose concentrations were recorded every 2 h for the calculation of glycemic variability (GV) Indices, mean fingertip blood glucose (FtBG), blood glucose standard deviation (SD), coefficient of variation (CV), and mean amplitude of glucose excursions (MAGE) (21, 22). MAGE is one of the most preferred indices in the quantitative evaluation of the short-term within-day GV (23). The GV Indices were calculated using EasyGV (©) software (available free for non-commercial use at www.easygv.co.uk) (24). CV was calculated by dividing SD by the corresponding mean FtBG. The calculating formula of MAGE is shown below:
λ = glucose changes from peak to nadir, x = number of valid observations, and v = 1 SD of mean glucose for a 24-h period.
Other data regarding sex, GCS, causes of injury, and the IMPACT core variables (age, GCS-Motor score, and pupillary reactivity) (1) were also collected at admission. The causes of injury include road traffic accident, ground level fall, fall from height, and others (e.g., violence and the bruise injury caused by heavy object). The worst Marshall CT score (25) in 24 h after admission was also collected. The GCS score at admission was used to classify the severity of TBI, and GCS-M score was used in the final statistical analysis.
All patients of our study were admitted to 18-bed neurological intensive care unit (NICU). Treatments for study patients were under the direction of evidence-based protocols and management guidelines, such as ICP control strategy, ventilator management, sedation management, indications of surgical operations, nutritional support, stress ulcer prophylaxis, deep venous thrombosis prophylaxis, and antibiotic prophylaxis and therapy (26). In our study, we conducted conventional glycemic targets aimed at keeping glucose levels range of 6–10 mmol/L.
The outcome was the 6-month neurological outcome evaluated with the Glasgow outcome scale (GOS): (1) death; (2) persistent vegetative state, severe damage with a prolonged state of unresponsiveness, and a lack of higher mental functions; (3) severe disability, severe injury with a permanent need for help with daily living; (4) moderate disability, no need for assistance in everyday life, employment is possible but may require special equipment; (5) low disability, light damage with minor neurological and psychological deficits. GOS 4–5 is defined as a favorable prognosis, while GOS 1–3 as an unfavorable prognosis.
Normally distributed continuous variables were reported by mean and SD, and compared by Student's t test. Non-normally distributed continuous variables were presented by the median and interquartile ranges (IQR), and compared using the Mann–Whitney U test. The analyzed categorical variables between groups were conducted by the chi-squared test. A propensity score matching (PSM) procedure was used to balance the co-variable factors of the IR and non-IR groups. SPSS version 26.0 (IBM Corporation, Armonk, NY, USA) was used to perform the statistical analyzes at α = 0.05. The PSM procedure was conducted by SPSS with R 3.5 Plug-in for Statistics.
A total of 101 consecutive patients with isolated moderate-to-severe TBI (admission GCS ≤ 12) who had no history of diabetes were admitted to our 18-bed NICU. A total of 13 patients were excluded for their admission HbA1c ≥ 6.5%. A total of 4 patients were excluded for the unqualified glucose or insulin level to calculate HOMA2. Finally, 85 patients were completely investigated. Among the included patients, 34 (40%) were diagnosed with IR with HOMA2 ≥ 1.4, while 51 (60%) were with HOMA2 < 1.4 after TBI (Figure 1).
The analysis of characteristics suggested statistically significant differences in gender, FBG level, GCS-Motor score, and pupillary reactivity between the IR and non-IR groups. There were no differences in age, the level of HbA1c, causes of injury and Marshall CT score between the two groups. The nearest neighbor PSM was performed in a 1:2 ratio for gender, FBG level, GCS-Motor score, and pupillary reactivity, with a caliper value of 0.2 for exact matching. After PSM analysis for the co-variables, 22 patients in IR group were matched to 34 patients in non-IR group. All baseline characteristics were well balanced between the two groups in the post-PSM analysis (Table 1).
Table 1. Baseline data comparison between the two groups before and after propensity score matching (PSM).
Before PSM, mean FtBG, SD, and MAGE in patients with HOMA2 ≥ 1.4 was higher than that in patients with HOMA2 < 1.4, while no statistically significant association was found between IR and CV. In the analysis between the propensity-matched groups, higher MAGE was still associated with IR after TBI (p = 0.031) (Table 2).
Table 2. The comparison of Glycemic Variability Indices between the two groups before and after PSM.
The poor prognosis after TBI was significantly more frequent in the IR group as compared to the non-IR group (50.00 vs. 23.53%, p = 0.041) in the propensity-matched groups. IR was a significant factor for the poor prognosis of non-diabetic patients with isolated moderate-to-severe TBI (OR = 3.25, 95% CI 1.03–10.31) (Table 3).
Table 3. Comparative analysis of Glasgow outcome scale (GOS) between the two groups before and after PSM.
We enrolled 85 non-diabetic patients with isolated moderate-to-severe TBI in this 1-year case cohort. Approximately 40% (34/85) of the cohort patients suffered IR with HOMA2 ≥ 1.4. A PSM procedure was used to balance the co-variable factors of the IR and non-IR groups, and the further analysis suggested that IR was associated with increased systemic glycemic variation and a poor prognosis after isolated moderate-to-severe TBI among non-diabetic patients.
Insulin is one of the main treatments for ICU clinicians to treat hyperglycemia of critically ill patients, which is partly similar to the treatment of diabetes. The evaluation of IR is one of the important procedures in the diagnosis and treatment of diabetes. However, the concern of IR is always lacking in non-diabetic hyperglycemia. Clinicians are always confused about the assessments of insulin sensitivity. It is the main reason that restricts ICU clinicians to evaluate insulin sensitivity in non-diabetic patients (27). Though several methods have been used to evaluate the insulin sensitivity, there are still no standardized methods and reference values (27). Hyperinsulinemic-euglycemic clamp (HIEC) is referred to as the gold standard for insulin sensitivity assessment, but the complex procedure limits its use in critically ill patients (28). Indirect indices of IR in fasting state are another insulin sensitivity parameter. HOMA is one of the representative indices (19). With the advantages of simple, economy and almost harmless to patients, HOMA are widely applied in epidemiological investigation, large-scale clinical trials, clinical investigation (29, 30). Several studies have verified the good correlation between estimates of IR derived from HOMA and HIEC (19, 31, 32).
In our study, estimates of insulin sensitivity were assessed by HOMA2 Calculator. IR was defined as HOMA2 ≥ 1.4. Mowery et al. explore IR among the ventilated, critically ill surgical patients with TBI through an adapting multiplier, which is based on the hypoglycemic efficiency of insulin (16). However, the adapting multiplier is a retrospective value, and nutritional support interfered with the dosage of insulin during the insulin therapy procedure, which limited its use. Recently, several researchers used triglyceride-glucose index (TyG) as a simple insulin sensitivity parameter (33). TyG was reported to have a high correlation with HOMA (34, 35). TyG was also suggested to be superior to HOMA in several IR-related diseases (36–38), there was still a study showing that HOMA has advantages yet (39). Considering that IR in acute critical illness may not immediately respond to changes in TyG, it seems more reasonable for TyG to study IR in chronic diseases. It is worthy to study the association of TyG and chronic IR after TBI.
The incidence of IR in our cohort was about 40%, which was significantly higher than the incidence of hyperglycemia in our study (fasting blood glucose ≥ 11.1 mmol/L) and that reported in several studies on TBI (5, 40, 41). Although there are no relevant research reports, it is rational that part of TBI patients with IR may only presented abnormal fasting blood glucose. In our study, only 23.53% patients with HOMA2 ≥ 1.4 were with fasting blood glucose higher than 11.1 mmol/L, and 88.24% ones were with fasting blood glucose higher than 6.1 mmol/L (data do not show). However, this was only the result of a small sample analysis with HOMA2. It is necessary to expand the variety of insulin sensitivity methods and the research sample to reach a comprehensive perspective on the incidence of impaired insulin sensitivity after TBI.
In our study, after balancing the co-variables, our data suggested that poor prognosis after TBI was significantly more frequent in the IR group as compared to the non-IR group (50.00 vs. 23.53%, p = 0.041). There are other studies reporting IR lower the likelihood of neurological improvement in neurocritical illness both laboratory and clinic. Xin et al. (42) induced IR in an animal model with severe TBI and found that IR was associated with higher modified neurological severity scores after severe TBI. A meta-analysis on acute cerebral ischemia suggested that a higher HOMA index was associated with a higher risk of neurological deterioration (17). Mowery et al. reported that IR indexed by an adapting multiplier was associated with in-hospital mortality after TBI (16).
Insulin resistance can contribute to the poor prognosis of TBI in many ways. Patients with IR presented increased systemic glycemic variation (higher MAGE). An observational study included 5,567 critical ill patients; suggested increased glycemic variability was independently associated with increased mortality among patients with HbA1c <6.5% (43). Another large observational study included 11,812 TBI patients found that greater GV was associated with greater mortality (44). Greater GV after IR were associated with dysregulated energy metabolism, aggravated oxidative stress and exacerbated inflammatory responses, and may lead to poor prognosis (9, 10, 12). In addition, the novel and important effect of insulin signaling on CNS has been widely studied (45). Insulin signaling was participated in neuronal survival and synaptic plasticity (46), while impaired brain insulin signaling was associated with exacerbated neuroinflammation (47), aggravated glutamate excitotoxicity (48), decreased synaptosomal insulin responsiveness (49).
The effects of IR on the prognosis of TBI are mainly due to the impairment of normal insulin action on modulating peripheral metabolism and protecting brain function. It is well studied that insulin signaling cascade promotes glucose and lipid metabolism, protein synthesis, and cell/neuron survival through activation of phosphatidylinositol-3-kinase/Akt pathway (PI3K/Akt) and mitogen-activated protein kinases/Ras pathway (MAPK/Ras) both in the brain and in the periphery (8, 50). IR after TBI leads to dysfunction in neurogenesis, brain function, and whole-body energy balance and metabolism (13).
Therefore, therapy on insulin signaling may be beneficial both in glycemic normalization and neuroprotective effects among patients with TBI (51, 52). Evidences suggested that brain insulin signaling regulates the homeostasis of peripheral energy metabolism through the autonomic nervous system and hypothalamic–pituitary axis (8, 50). The incretins Glucagon-like peptide 1 (GLP-1) and glucose-dependent insulinotropic peptide (GIP), which were suggested to be efficacy in restoring insulin sensitivity (53), have shown potential in reducing GV in patients with Type 2 Diabetes (54, 55). The neuroprotective effect of insulin administration has been reported in vivo (56) and in animal models (50, 51, 57) by alleviating glutamate excitotoxicity and reducing inflammatory response. Insulin-like growth factor I (another ligand of insulin receptor signaling) (58, 59), Thiazolidinedione (TZD, known as insulin sensitizing drug) (60, 61), and the incretins GLP-1 and GIP (62, 63) were all reported efficacy in preventing apoptosis, oxidative stress, and neuroinflammation and improving neurological deficit after TBI in animal model. A TZD drug, Pioglitazone, was reported to be associated with a lower risk of recurrent ischemic events in a multicenter, double-blind trial involving non-diabetic patients with IR (64). In addition, several incretin-based therapies have also been approved in Clinical Trials (63). These results make ameliorating IR as a potential approach to TBI treatment, yet which needs concerted efforts to conduct more comprehensive research.
There are several limitations in our study. First, our study is a single-center observational study with relatively strict enrollment. Our study population provided us the opportunity to explore the impact of IR on the prognosis after isolated TBI in a small sample, yet the findings of our study cohort cannot be extended to TBI patients with severe multiple injuries. The small sample size also limits our further analysis on the subgroup that HOMA and FBG are not well correlated. The insight into this subgroup may lead to a more comprehensive perspective on the IR and hyperglycemia after TBI and their effects on the prognosis after TBI. We will focus on this unique subgroup in future studies. Second, because of the applicability of the HOMA2 calculator to glucose and insulin concentrations, four cases were excluded in our study, which may bias the results. In further HOMA studies, multiple sampling to avoid single test error and unusable sample value may be recommended (30). The comprehensive application of over one simple insulin sensitivity index should be considered to better evaluate the insulin sensitivity of the patients. In addition, the impaired insulin sensitivity is not constant (65), and the dynamic assessment of insulin sensitivity and a metabolic follow-up may be more helpful for the TBI treatment. Last but not least, we have only discussed the benefits of insulin sensitization therapy based on our findings, and in follow-up studies, we will design trials to investigate the exact effect of insulin sensitization therapy on TBI.
IR estimated by HOMA2 was associated with greater GV and an unfavorable outcome after isolated moderate-to-severe TBI among non-diabetic patients. Ameliorating impaired insulin sensitivity may be a potential approach to TBI treatment.
The raw data supporting the conclusions of this article will be made available by the authors, without undue reservation.
The studies involving human participants were reviewed and approved by the Ethics Committee of Jiangyin Hospital Affiliated to Nantong University. The patients/participants provided their written informed consent to participate in this study.
CC, HG, and WW were involved in the design of study. CC wrote the manuscript. CC and HW collected all the data and involved in the statistical analysis. HG and WW evaluated the results and revised the manuscript. All authors contributed to the article and approved the submitted version.
HG was supported by the Taihu Lake Talent Program (2021) of Wuxi City.
The authors thank the research groups at the University of Oxford for the availability of HOMA2 calculator and EasyGV software.
The authors declare that the research was conducted in the absence of any commercial or financial relationships that could be construed as a potential conflict of interest.
All claims expressed in this article are solely those of the authors and do not necessarily represent those of their affiliated organizations, or those of the publisher, the editors and the reviewers. Any product that may be evaluated in this article, or claim that may be made by its manufacturer, is not guaranteed or endorsed by the publisher.
1. Steyerberg EW, Mushkudiani N, Perel P, Butcher I, Lu J, McHugh GS, et al. Predicting outcome after traumatic brain injury: development and international validation of prognostic scores based on admission characteristics. PLoS Med. (2008) 5:e165. doi: 10.1371/journal.pmed.0050165
2. Hermanides J, Plummer MP, Finnis M, Deane AM, Coles JP, Menon DK. Glycaemic control targets after traumatic brain injury: a systematic review and meta-analysis. Crit Care. (2018) 22:11. doi: 10.1186/s13054-017-1883-y
3. NICE-SUGAR Study Investigators for the Australian and New Zealand Intensive Care Society Clinical Trials Group and the Canadian Critical Care Trials Group, Finfer S, Chittock D, Li Y, Foster D, Dhingra V, et al. Intensive vs. conventional glucose control in critically ill patients with traumatic brain injury: long-term follow-up of a subgroup of patients from the NICE-SUGAR study. Intensive Care Med. (2015) 41:1037–47. doi: 10.1007/s00134-015-3757-6
4. Chun-Chia T, Ying-Cheng H, Po-Hsun T, Ping KY, Ting-Wei C, Cheng-Chi L, et al. Impact of diabetic hyperglycemia on clinical outcomes in patients with diabetes mellitus following traumatic brain injury. Turk Neurosurg. (2021) [Epub ahead of print]. doi: 10.5137/1019-5149.JTN.33659-21.3
5. Rau C-S, Wu S-C, Chen Y-C, Chien P-C, Hsieh H-Y, Kuo P-J, et al. Stress-induced hyperglycemia, but not diabetic hyperglycemia, is associated with higher mortality in patients with isolated moderate and severe traumatic brain injury: analysis of a propensity score-matched population. Int J Environ Res Public Health. (2017) 14:E1340. doi: 10.3390/ijerph14111340
6. Tsai Y-C, Wu S-C, Hsieh T-M, Liu H-T, Huang C-Y, Chou S-E, et al. Association of stress-induced hyperglycemia and diabetic hyperglycemia with mortality in patients with traumatic brain injury: analysis of a propensity score-matched population. Int J Environ Res Public Health. (2020) 17:E4266. doi: 10.3390/ijerph17124266
7. Shi J, Dong B, Mao Y, Guan W, Cao J, Zhu R, et al. Review: traumatic brain injury and hyperglycemia, a potentially modifiable risk factor. Oncotarget. (2016) 7:71052–61. doi: 10.18632/oncotarget.11958
8. Agrawal R, Reno CM, Sharma S, Christensen C, Huang Y, Fisher SJ. Insulin action in the brain regulates both central and peripheral functions. Am J Physiol Endocrinol Metab. (2021) 321:E156–63. doi: 10.1152/ajpendo.00642.2020
9. Johan Groeneveld AB, Beishuizen A, Visser FC. Insulin: a wonder drug in the critically ill? Crit Care. (2002) 6:102–5. doi: 10.1186/cc1463
10. Matsushima K, Peng M, Velasco C, Schaefer E, Diaz-Arrastia R, Frankel H. Glucose variability negatively impacts long-term functional outcome in patients with traumatic brain injury. J Crit Care. (2012) 27:125–31. doi: 10.1016/j.jcrc.2011.08.012
11. Deng J, Li L, Cao F, Wang F, Wang H, Shi H, et al. Systemic glycemic variation predicts mortality of acute ischemic stroke after mechanical thrombectomy: a prospective study using continuous glucose monitoring. Front Neurol. (2022) 13:817033. doi: 10.3389/fneur.2022.817033
12. Palaiodimou L, Lioutas V.-A, Lambadiari V, Theodorou A, Themistocleous M, Aponte L, et al. Glycemic variability of acute stroke patients and clinical outcomes: a continuous glucose monitoring study. Ther Adv Neurol Disord. (2021) 14:17562864211045876. doi: 10.1177/17562864211045876
13. Shaughness M, Acs D, Brabazon F, Hockenbury N, Byrnes KR. Role of insulin in neurotrauma and neurodegeneration: a review. Front Neurosci. (2020) 14:547175. doi: 10.3389/fnins.2020.547175
14. Neth BJ, Craft S. Insulin resistance and alzheimer's disease: bioenergetic linkages. Front Aging Neurosci. (2017) 9:345. doi: 10.3389/fnagi.2017.00345
15. Hamer JA, Testani D, Mansur RB, Lee Y, Subramaniapillai M, McIntyre RS. Brain insulin resistance: a treatment target for cognitive impairment and anhedonia in depression. Exp Neurol. (2019) 315:1–8. doi: 10.1016/j.expneurol.2019.01.016
16. Mowery NT, Gunter OL, Guillamondegui O, Dossett LA, Dortch MJ, Morris JA, et al. Stress insulin resistance is a marker for mortality in traumatic brain injury. J Trauma. (2009) 66:145–51. doi: 10.1097/TA.0b013e3181938c5e
17. Wu A, Li Y, Liu R, Qi D, Yu G, Yan X, et al. Predictive value of insulin resistance as determined by homeostasis model assessment in acute ischemic stroke patients: a systematic review and meta-analysis. Horm Metab Res. (2021) 53:746–51. doi: 10.1055/a-1648-7767
18. Mowery NT, Dortch MJ, Dossett LA, Norris PR, Diaz JJ, Morris JA, et al. Insulin resistance despite tight glucose control is associated with mortality in critically ill surgical patients. J Intensive Care Med. (2009) 24:242–51. doi: 10.1177/0885066609335663
19. Matthews DR, Hosker JP, Rudenski AS, Naylor BA, Treacher DF, Turner RC. Homeostasis model assessment: insulin resistance and beta-cell function from fasting plasma glucose and insulin concentrations in man. Diabetologia. (1985) 28:412–9. doi: 10.1007/BF00280883
20. Mojiminiyi OA, Abdella NA. Effect of homeostasis model assessment computational method on the definition and associations of insulin resistance. Clin Chem Lab Med. (2010) 48:1629–34. doi: 10.1515/CCLM.2010.303
21. Service FJ, Molnar GD, Rosevear JW, Ackerman E, Gatewood LC, Taylor WF. Mean amplitude of glycemic excursions, a measure of diabetic instability. Diabetes. (1970) 19:644–55. doi: 10.2337/diab.19.9.644
22. Breyton A-E, Lambert-Porcheron S, Laville M, Vinoy S, Nazare J-A. CGMS and glycemic variability, relevance in clinical research to evaluate interventions in T2D, a literature review. Front Endocrinol. (2021) 12:666008. doi: 10.3389/fendo.2021.666008
23. New and Improved Methods to Characterize Glycemic Variability Using Continuous Glucose Monitoring. Available online at: https://pubmed.ncbi.nlm.nih.gov/19764834/ (accessed May 11, 2022).
24. Hill NR, Oliver NS, Choudhary P, Levy JC, Hindmarsh P, Matthews DR. Normal reference range for mean tissue glucose and glycemic variability derived from continuous glucose monitoring for subjects without diabetes in different ethnic groups. Diabetes Technol Ther. (2011) 13:921–8. doi: 10.1089/dia.2010.0247
25. Marshall LF, Marshall SB, Klauber MR, Van Berkum Clark M, Eisenberg H, Jane JA, et al. The diagnosis of head injury requires a classification based on computed axial tomography. J Neurotrauma. (1992) 9:S287–92.
26. Carney N, Totten AM, O'Reilly C, Ullman JS, Hawryluk GWJ, Bell MJ, et al. Guidelines for the management of severe traumatic brain injury, fourth edition. Neurosurgery. (2017) 80:6–15. doi: 10.1227/NEU.0000000000001432
27. Group (preparatory), C.D.S.I.R.S. Expert guidance on insulin resistance assessment methods and applications. Chin J Diabetes Mellit. (2018) 10:377–385. doi: 10.3760/cma.j.issn.1674-5809.2018.06.001
28. Defronzo RA, Tobin JD, Andres R. Glucose clamp technique: a method for quantifying insulin secretion and resistance. Am J Physiol. (1979) 237:E214–23. doi: 10.1152/ajpendo.1979.237.3.E214
29. Minh HV, Tien HA, Sinh CT, Thang DC, Chen C-H, Tay JC, et al. Assessment of Preferred methods to measure insulin resistance in Asian patients with hypertension. J Clin Hypertens. (2021) 23:529–37. doi: 10.1111/jch.14155
30. Wallace TM, Levy JC, Matthews DR. Use and abuse of HOMA modeling. Diabetes Care. (2004) 27:1487–95. doi: 10.2337/diacare.27.6.1487
31. Bonora E, Targher G, Alberiche M, Bonadonna RC, Saggiani F, Zenere MB, et al. Homeostasis model assessment closely mirrors the glucose clamp technique in the assessment of insulin sensitivity: studies in subjects with various degrees of glucose tolerance and insulin sensitivity. Diabetes Care. (2000) 23:57–63 doi: 10.2337/diacare.23.1.57
32. Emoto M, Nishizawa Y, Maekawa K, Hiura Y, Kanda H, Kawagishi T, et al. Homeostasis model assessment as a clinical index of insulin resistance in type 2 diabetic patients treated with sulfonylureas. Diabetes Care. (1999) 22:818–22. doi: 10.2337/diacare.22.5.818
33. Mazidi M, Katsiki N, Kengne AP, Mikhailidis DP, Banach M. Adiposity mediates the association between whole grain consumption, glucose homeostasis and insulin resistance: findings from the US NHANES. Lipids Health Dis. (2018) 17:219. doi: 10.1186/s12944-018-0805-6
34. Gesteiro E, Bastida S, Barrios L, Sánchez-Muniz FJ. The triglyceride-glucose index, an insulin resistance marker in newborns? Eur J Pediatr. (2018) 177:513–20. doi: 10.1007/s00431-018-3088-z
35. Dikaiakou E, Vlachopapadopoulou EA, Paschou SA, Athanasouli F, Panagiotopoulos I, Kafetzi M, et al. Triglycerides-Glucose (TyG) index is a sensitive marker of insulin resistance in Greek children and adolescents. Endocrine. (2020) 70:58–64. doi: 10.1007/s12020-020-02374-6
36. Son D-H, Lee HS, Lee Y-J, Lee J-H, Han J-H. Comparison of triglyceride-glucose index and HOMA-IR for predicting prevalence and incidence of metabolic syndrome. Nutr Metab Cardiovasc Dis. (2022) 32:596–604. doi: 10.1016/j.numecd.2021.11.017
37. Nam K-W, Kwon H-M, Jeong H-Y, Park J-H, Kwon H, Jeong S-M. High triglyceride-glucose index is associated with subclinical cerebral small vessel disease in a healthy population: a cross-sectional study. Cardiovasc Diabetol. (2020) 19:53. doi: 10.1186/s12933-020-01031-6
38. Thai PV, Tien HA, Van Minh H, Valensi P. Triglyceride glucose index for the detection of asymptomatic coronary artery stenosis in patients with type 2 diabetes. Cardiovasc Diabetol. (2020) 19:137. doi: 10.1186/s12933-020-01108-2
39. Gu Q, Meng J, Hu X, Ge J, Wang SJ, Liu XZ. Isolated systolic hypertension and insulin resistance assessment tools in young and middle-aged chinese men with normal fasting glucose: a cross-sectional study. Sci Rep. (2022) 12:758. doi: 10.1038/s41598-021-04763-x
40. Bosarge PL, Shoultz TH, Griffin RL, Kerby JD. Stress-induced hyperglycemia is associated with higher mortality in severe traumatic brain injury. J Trauma Acute Care Surg. (2015) 79:289–94. doi: 10.1097/TA.0000000000000716
41. Chen C-H, Hsieh Y-W, Huang J-F, Hsu C-P, Chung C-Y, Chen C-C. Predictors of In-hospital mortality for road traffic accident-related severe traumatic brain injury. J Pers Med. (2021) 11:1339. doi: 10.3390/jpm11121339
42. Kang X, Liu Y, Yuan T, Jiang N-N, Dong Y-B, Wang J-W, et al. Early care of acute hyperglycemia benefits the outcome of traumatic brain injury in rats. Brain Res. (2016) 1650:112–7. doi: 10.1016/j.brainres.2016.08.038
43. Krinsley JS, Rule P, Pappy L, Ahmed A, Huley-Rodrigues C, Prevedello D, et al. The interaction of acute and chronic glycemia on the relationship of hyperglycemia, hypoglycemia, and glucose variability to mortality in the critically ill. Crit Care Med. (2020) 48:1744–51. doi: 10.1097/CCM.0000000000004599
44. Pappacena S, Bailey M, Cabrini L, Landoni G, Udy A, Pilcher DV, et al. Early dysglycemia and mortality in traumatic brain injury and subarachnoid hemorrhage. Minerva Anestesiol. (2019) 85:830–9. doi: 10.23736/S0375-9393.19.13307-X
45. Maciejczyk M, Zebrowska E, Chabowski A. Insulin resistance and oxidative stress in the brain: what's new? Int J Mol Sci. (2019) 20:E874. doi: 10.3390/ijms20040874
46. Bilotta F, Lauretta MP, Tewari A, Haque M, Hara N, Uchino H, et al. Insulin and the brain: a sweet relationship with intensive care. J Intensive Care Med. (2017) 32:48–58. doi: 10.1177/0885066615594341
47. Karelina K, Sarac B, Freeman LM, Gaier KR, Weil ZM. Traumatic brain injury and obesity induce persistent central insulin resistance. Eur J Neurosci. (2016) 43:1034–43. doi: 10.1111/ejn.13194
48. Pomytkin I, Krasil'nikova I, Bakaeva Z, Surin A, Pinelis V. Excitotoxic glutamate causes neuronal insulin resistance by inhibiting insulin Receptor/Akt/MTOR pathway. Mol Brain. (2019) 12:112. doi: 10.1186/s13041-019-0533-5
49. Franklin W, Krishnan B, Taglialatela G. Chronic synaptic insulin resistance after traumatic brain injury abolishes insulin protection from amyloid beta and tau oligomer-induced synaptic dysfunction. Sci Rep. (2019) 9:8228. doi: 10.1038/s41598-019-44635-z
50. Kleinridders A, Ferris HA, Cai W, Kahn CR. Insulin action in brain regulates systemic metabolism and brain function. Diabetes. (2014) 63:2232–43. doi: 10.2337/db14-0568
51. Brabazon F, Wilson CM, Jaiswal S, Reed J, Frey WH, Byrnes KR. Intranasal insulin treatment of an experimental model of moderate traumatic brain injury. J Cereb Blood Flow Metab. (2017) 37:3203–18. doi: 10.1177/0271678X16685106
52. Beirami E, Oryan S, Seyedhosseini Tamijani SM, Ahmadiani A, Dargahi L. Intranasal insulin treatment alleviates methamphetamine induced anxiety-like behavior and neuroinflammation. Neurosci Lett. (2017) 660:122–9. doi: 10.1016/j.neulet.2017.09.026
53. Li Z, Zhu Y, Li C, Tang Y, Jiang Z, Yang M, et al. Liraglutide ameliorates palmitate-induced insulin resistance through inhibiting the IRS-1 serine phosphorylation in mouse skeletal muscle cells. J Endocrinol Invest. (2018) 41:1097–102. doi: 10.1007/s40618-018-0836-x
54. FLAT-SUGAR trial investigators. Glucose variability in a 26-week randomized comparison of mealtime treatment with rapid-acting insulin vs. GLP-1 agonist in participants with type 2 diabetes at high cardiovascular risk. Diabetes Care. (2016) 39:973–81. doi: 10.2337/dc15-2782
55. Lee H, Park S-E, Kim E-Y. Glycemic variability impacted by SGLT2 inhibitors and GLP 1 agonists in patients with diabetes mellitus: a systematic review and meta-analysis. J Clin Med. (2021) 10:4078. doi: 10.3390/jcm10184078
56. Krasil'nikova I, Surin A, Sorokina E, Fisenko A, Boyarkin D, Balyasin M, et al. Insulin protects cortical neurons against glutamate excitotoxicity. Front Neurosci. (2019) 13:1027. doi: 10.3389/fnins.2019.01027
57. Rajasekar N, Nath C, Kanif K, Shukla R. intranasal insulin administration ameliorates streptozotocin (ICV)-induced insulin receptor dysfunction, neuroinflammation, amyloidogenesis, and memory impairment in rats. Molr Neurobiol. (2017) 54:6507–22. doi: 10.1007/s12035-016-0169-8
58. Herrera ML, Bandín S, Champarini LG, Hereñú CB, Bellini MJ. Intramuscular insulin-like growth factor-1 gene therapy modulates reactive microglia after traumatic brain injury. Brain Res Bull. (2021) 175:196–204. doi: 10.1016/j.brainresbull.2021.07.023
59. Montivero AJ, Ghersi MS, Silvero CMJ, Artur de la Villarmois E, Catalan-Figueroa J, Herrera M, et al. Early IGF-1 Gene Therapy Prevented Oxidative Stress and Cognitive Deficits Induced by Traumatic Brain Injury. Front Pharmacol. (2021) 12:672392 doi: 10.3389/fphar.2021.672392
60. Weil Z.M, Karelina K, Whitehead B, Velazquez-Cruz R, Oliverio R, Pinti M, Nwafor D.C, Nicholson S, Fitzgerald J.A, Hollander J, et al. Mild traumatic brain injury increases vulnerability to cerebral ischemia in mice. Exp Neurol. (2021) 342:113765 doi: 10.1016/j.expneurol.2021.113765
61. Liu H, Rose ME, Culver S, Ma X, Dixon CE, Graham SH. Rosiglitazone attenuates inflammation and CA3 neuronal loss following traumatic brain injury in rats. Biochem Biophys Res Commun. (2016) 472:648–55. doi: 10.1016/j.bbrc.2016.03.003
62. Bader M, Li Y, Tweedie D, Shlobin NA, Bernstein A, Rubovitch V, et al. Neuroprotective Effects and Treatment Potential of Incretin Mimetics in a Murine Model of Mild Traumatic Brain Injury. Front Cell Dev Biol. (2019) 7:356 doi: 10.3389/fcell.2019.00356
63. Glotfelty E.J, Delgado T, Tovar-Y-Romo L.B, Luo Y, Hoffer B, Olson L, Karlsson T, Mattson M.P, Harvey B, Tweedie D, et al. Incretin mimetics as rational candidates for the treatment of traumatic brain injury. ACS Pharmacol Transl Sci. (2019) 2:66–91. doi: 10.1021/acsptsci.9b00003
64. Kernan WN, Viscoli CM, Furie KL, Young LH, Inzucchi SE, Gorman M, et al. Pioglitazone after ischemic stroke or transient ischemic attack. N Engl J Med. (2016) 374:1321–31. doi: 10.1056/NEJMoa1506930
65. Morimoto A, Tatsumi Y, Soyano F, Miyamatsu N, Sonoda N, Godai K, et al. Increase in Homeostasis Model Assessment of Insulin Resistance (HOMA-IR) had a strong impact on the development of type 2 diabetes in japanese individuals with impaired insulin secretion: the saku study. PLoS ONE. (2014) 9:e105827. doi: 10.1371/journal.pone.0105827
Keywords: traumatic brain injury, insulin resistance, HOMA2, hyperglycemia, glycemic variability, prognosis
Citation: Cao C, Wang H, Gao H and Wu W (2022) Insulin resistance is associated with an unfavorable outcome among non-diabetic patients with isolated moderate-to-severe traumatic brain injury – A propensity score-matched study. Front. Neurol. 13:949091. doi: 10.3389/fneur.2022.949091
Received: 20 May 2022; Accepted: 04 July 2022;
Published: 28 July 2022.
Edited by:
Changiz Taghibiglou, University of Saskatchewan, CanadaReviewed by:
Zachary M. Weil, West Virginia University, United StatesCopyright © 2022 Cao, Wang, Gao and Wu. This is an open-access article distributed under the terms of the Creative Commons Attribution License (CC BY). The use, distribution or reproduction in other forums is permitted, provided the original author(s) and the copyright owner(s) are credited and that the original publication in this journal is cited, in accordance with accepted academic practice. No use, distribution or reproduction is permitted which does not comply with these terms.
*Correspondence: Wei Wu, ZHJfd3V3ZWk4NTJAMTYzLmNvbQ==; Heng Gao, ZHJfZ2FvaGVuZ0AxNjMuY29t
Disclaimer: All claims expressed in this article are solely those of the authors and do not necessarily represent those of their affiliated organizations, or those of the publisher, the editors and the reviewers. Any product that may be evaluated in this article or claim that may be made by its manufacturer is not guaranteed or endorsed by the publisher.
Research integrity at Frontiers
Learn more about the work of our research integrity team to safeguard the quality of each article we publish.