- 1School of Medicine (School of Nursing), Nantong University, Nantong, China
- 2Department of Neurology, Nantong Third People's Hospital, Nantong University, Nantong, China
- 3Department of Gerontology, Nantong Third People's Hospital, Nantong University, Nantong, China
Introduction: Post-stroke fatigue (PSF) is a common complication in the patients with acute ischemic stroke (AIS). This prospective study aimed to investigate the relationship between red blood cell distribution width (RDW) at admission and PSF in the acute phase.
Methods: The AIS patients were enrolled in Nantong Third People's Hospital, consecutively. PSF in the acute phase was scored according to the Fatigue Severity Scale. Levels of RDW were measured at admission. The associations were analyzed using multivariate regression and restricted cubic splines (RCS).
Results: From April 2021 to March 2022, a total of 206 AIS patients (mean age, 69.3 ± 10.7 years; 52.9% men) were recruited. After the adjustment for potential confounding factors, RDW at admission remained the independent associated factor with PSF in the acute phase (OR [odds ratio], 1.635; 95% CI [confidence interval], 1.153–2.318; P = 0.006). The linear dose-response associations of RDW with PSF in the acute phase were found, based on the RCS model (P for non-linearity = 0.372; P for linearity = 0.037). These results remained significant in other models.
Conclusions: RDW at admission could serve as a novel biomarker of PSF in the acute phase of AIS.
Introduction
Stroke is one of the vital reasons for global disease burden (1–3). Acute ischemic stroke (AIS) accounts for the vast majority of stroke. Although there are many treatment options for ischemic stroke nowadays (4–6), the AIS patients may undergo several complications during their treatment and rehabilitation. Post-stroke fatigue (PSF), whose incidence range from 23 to 85%, is a common emotional complication after the onset of AIS (7–11). PSF could lead to poor recovery and prognosis (7–11). Therefore, it is meaningful and fundamental to explore the related factors to PSF.
Red blood cell distribution width (RDW), which could be obtained from routine blood testing, is an easily accessible biomarker. According to previous studies, RDW is able to reflect the variability in volumes of peripheral red blood cells and associated with inflammation (12, 13). In addition, RDW has been shown to be an effective biomarker for many diseases. For instance, RDW may serve as an undesirable prognostic factor in patients treated with hematopoietic stem cell transplantation (14). The levels of RDW might be associated with long-term all-cause mortality in the patients with acute myocardial infarction (15). Moreover, one recent study, which is carried out by Li Y et al., have found that elevated levels of RDW at admission may be able to predict depression after the onset of AIS (16).
Nevertheless, to our knowledge, there is no research about the relationship between the levels of RDW at admission and PSF in AIS patients. Hence, our prospective study was designed to assess the role of RDW on PSF in the acute phase.
Materials and methods
Study subjects
From April 2021 to March 2022, the AIS patients were enrolled in our study from the Nantong Third People's Hospital, consecutively and prospectively.
The inclusion criteria were listed as follows:
• Onset within 7 days;
• Age≥18 years;
• With sufficient cognitive ability;
• With sufficient knowledge of the Mandarin Chinese language and Nantong local dialect.
The exclusion criteria were listed as follows:
• Pre-stroke fatigue;
• Incomplete data;
• Refuse to participate in the research;
• Poor mental state.
This study was registered with China clinical trial registration center (Registration number: ChiCTR2100044165), approved by the ethics committee of Nantong Third People's hospital (Ethics number: EK2021008), and performed according to the principles of the Declaration of Helsinki.
Clinical assessments
The clinical assessments were completed in a separate conversation room. We collected general demographic questionnaires (age and sex), past medical history (hypertension, diabetes, cancer, coronary heart disease, atrial fibrillation, arthritis, previous stroke, alcohol abuse and tobacco use), clinical data (systolic blood pressure, diastolic blood pressure, stroke severity and anxiety severity) and laboratory parameters (fast blood glucose, total cholesterol, triglycerides, high density lipoprotein, low density lipoprotein, creatinine and RDW). Stroke severity was assessed by National Institute of Health stroke scale (NIHSS). Anxiety severity was assessed via Hamilton Anxiety Scale (HAMA). The levels of RDW at admission were measured within 24 h after admission.
The definition of PSF in the acute phase
PSF was assessed by the Fatigue Severity Scale (FSS) within 2 weeks after onset of ischemic stroke. FSS consists of 9 items, each item according to the patient's evaluation of fatigue severity will gradually transition the result to 1–7 points. The higher the score, the more severe PSF. We took the total score of 36 as the dividing line (7).
Statistical analysis
R software (Version 4.1.3; http://www.r-project.org) was used to conduct statistical analyses. Categorical variables are expressed as n (percentages). Normally distributed variables are expressed as the mean ± SD, and abnormally distributed continuous variables are expressed as medians (interquartile range [IQR]). The differences between PSF group and non-PSF group were identified with the Student's t-test, the Wilcoxon W-test, the chi-square test or Fisher's exact test as appropriate. The violin plot was utilized to present the distribution of RDW between the PSF group and the non-PSF group. We explored the relationship between RDW and PSF in different logistic regression models. Model 1 was unadjusted model. Model 2 was adjusted for age and sex. Model 3 was adjusted for age, sex, coronary heart disease and baseline NIHSS score. Model 4 was adjusted for age, sex, coronary heart disease, NIHSS score and HAMA score. What is more, we used restricted cubic splines (RCS) with three knots placed at the 10th, 50th, and 90th percentiles to evaluate the dose-response relationship of RDW with PSF in different models. P < 0.05 was considered statistically significant.
Results
From April 2021 to March 2022, we screened 233 AIS patients, and excluded 27 AIS patients as following reasons: Pre-stroke fatigue (n = 8); Incomplete data (n = 7); Refuse to participate in the research (n = 8); Poor mental state (n = 4) (Figure 1). Finally, a total of 206 AIS patients (mean age, 69.3 ± 10.7 years; 52.9% men) were included in the analysis.
Table 1 showed the baseline data of AIS patients stratified by PSF or non-PSF. In our study, the patients in the PSF group (n = 70) were more likely to have higher proportion of coronary heart disease (P = 0.026), elevated levels of NIHSS score (P = 0.036), HAMA score (P = 0.001) and RDW (P = 0.023). Figure 2 displayed the levels of RDW between the PSF groups and non-PSF groups (13.1% [12.5, 13.9%] vs. 12.8% [12.3, 13.4%], P = 0.023).
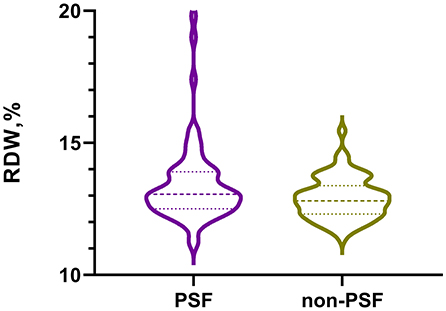
Figure 2. The violin plot in distribution of RDW between the PSF group and non-PSF group. RDW, red blood cell distribution width; PSF, post-stroke fatigue.
Figure 3 exhibited the results of logistic regression to explore the relationship between RDW and PSF in the acute phase. In the unadjusted model (model 1), RDW at admission might be related to PSF (OR [odds ratio], 1.545; 95% CI [confidence interval], 1.139–2.098; P = 0.005). After the adjustment for age and sex (model 2), RDW might also be the related factor to PSF (OR, 1.536; 95% CI, 1.135–2.080; P = 0.005). After the adjustment for age, sex, coronary heart disease and baseline NIHSS score (model 3), the OR of PSF for RDW was 1.517 (95% CI, 1.116–2.060, P = 0.008). What is more, in the model 4, which included age, sex, coronary heart disease, NIHSS score and HAMA score, RDW remained the independent associated factor with PSF (OR, 1.635; 95% CI, 1.153–2.318; P = 0.006).
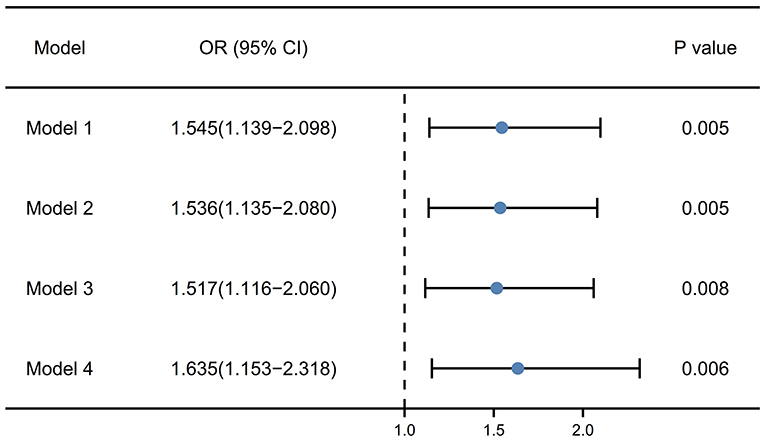
Figure 3. Multivariate logistic regression models for the association of RDW with PSF. Model 1, unadjusted model; Model 2, adjusted for age and sex; Model 3, adjusted for age, sex, coronary heart disease and baseline NIHSS score; Model 4, adjusted for age, sex, coronary heart disease, NIHSS score and HAMA score. RDW, red blood cell distribution width; PSF, post-stroke fatigue; NIHSS, National institute of health stroke scale; HAMA, Hamilton anxiety scale.
Figure 4 manifested the results of multivariable-adjusted spline regression models. In the model including age and sex, the linear dose-response associations of RDW at admission with PSF in the acute phase were found (P for non-linearity = 0.312; P for linearity = 0.019; Figure 4A). Furthermore, the linear dose-response associations of RDW with PSF in the acute phase remained significant in the model including age, sex, coronary heart disease and baseline NIHSS score (P for non-linearity = 0.351; P for linearity = 0.022; Figure 4B) and the model including age, sex, coronary heart disease, NIHSS score and HAMA score (P for non-linearity = 0.372; P for linearity = 0.037; Figure 4C).
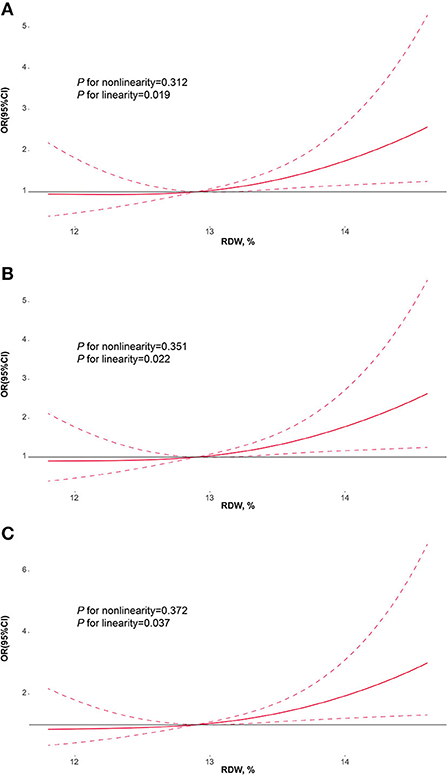
Figure 4. Restricted cubic spline regression models for the association of RDW with PSF. Adjusted odds ratios and 95% confidence intervals derived from restricted cubic spline regression, with knots placed at the 10th, 50th, and 90th percentiles of RDW. (A) adjusted for age and sex; (B) adjusted for age, sex, coronary heart disease and baseline NIHSS score; (C) adjusted for age, sex, coronary heart disease, NIHSS score and HAMA score. RDW, red blood cell distribution width; PSF, post-stroke fatigue; NIHSS, National institute of health stroke scale; HAMA, Hamilton anxiety scale.
Discussion
In this prospective observational study, we have found that RDW at admission could be one independent associated factor with PSF in the acute phase of AIS, according to the results of different logistic regression models. In addition, based on the RCS models, the linear dose-response associations of RDW with PSF in the acute phase were confirmed.
The incidence of PSF in the acute phase is 34.0 % in our study, which is in line with previous studies (7–11). This may be attributed to our rigorous and meticulous assessment about PSF during this prospective study. The incidence also indicated that approximately 1/3 of AIS patients might suffer from PSF in the acute phase. Therefore, the neurologists may be supposed to pay attention to the management of PSF in the acute phase.
RDW is a readily available laboratory parameter, which could reflect the variability in volumes of red blood cell. Higher levels of RDW mean greater variation in volumes. In normal Chinese population, the levels of RDW range from 11.0 to 16.0%, and may increase under pathological conditions. It is well known that inflammatory response plays a prominent role on the pathophysiology of cerebrovascular disease (17–22). RDW may be associated with C-reactive protein, interleukin −6 and other inflammatory biomarkers (23, 24). The research performed by Semba RD et al. manifested that serum selenium might be an independent predictor of RDW and mediate effects on RDW via interleukin-6 (25). These findings showed that RDW might be able to be an inflammatory biomarker and be involved in the pathophysiology of several inflammation-related diseases, for example, cerebrovascular diseases.
Previous clinical studies have revealed the role of RDW on cerebrovascular disease. Vayá A et al. found that RDW >14.0% might increase the risk of cryptogenic stroke (26). The results of one cross-sectional study, which enrolled 432 primary AIS patients, indicated that the AIS patients with carotid artery atherosclerosis could possess higher levels of RDW (27). RDW may also serve as an independent related factors to the prognostic outcomes in AIS patients treated with intravenous thrombolysis (28). Another research recruited the AIS patients without intravenous thrombolysis or endovascular treatment, and showed that elevated levels of RDW were related to increased risk of hemorrhagic transformation (29). A meta-analysis displayed that the baseline levels of RDW might be a predictor of stroke occurrence and outcome (30). What is more, high RDW levels may increase the risk of hemorrhagic transformation after intravenous thrombolysis in AIS patients (31). Nowadays, the levels of RDW were found to be linked to post-stroke depression (16), another common emotional complication after the onset of AIS. Although there are several studies focusing on the relationship between RDW and cerebrovascular disease, this study is the first prospective study that explore the role of RDW on PSF, and may assist neurologists with managing AIS patients.
However, there are still several shortcomings in our prospective observational study. First, we only collected the data about PSF in the acute phase now. Therefore, we are following up the PSF at 6 months in these AIS patients prospectively, and we will explore the relationship between RDW and PSF at 6 months in the future research. Second, the sample size of this study is relatively small. We aim to carry out the study with large sample to provide higher levels of evidence about the association of RDW with PSF. Third, RDW and other laboratory parameters may change during hospitalization, so it might be critical to monitor these parameters, dynamically. In addition, we have not utilized machine learning in this study and collected the data of other inflammatory biomarkers, such as interleukin-6 (IL-6) and tumor necrosis factor-α (TNF-α).
In short, this prospective observational study is the first study centering on the relationship between RDW at admission and PSF in the acute phase of AIS, as far as we know. Linear dose-response associations of RDW with PSF in the acute phase of AIS have been found. Consequently, RDW at admission may be a novel biomarker of PSF in the acute phase, which is helpful for neurologists. Nevertheless, the study with large sample is required in the future, and these conclusions need to be verified in other stroke centers.
Data availability statement
The original contributions presented in the study are included in the article, further inquiries can be directed to the corresponding author.
Ethics statement
The studies involving human participants were reviewed and approved by the Ethics Committee of Nantong Third People's hospital (Ethics number: EK2021008). The patients/participants provided their written informed consent to participate in this study.
Author contributions
MP wrote the manuscript and performed the statistical analyses. YuC collected the data and assisted with writing the manuscript. YaC, KF, HS, HH, WZ, HZ, and JJ collected the data. All authors contributed to the article and approved the submitted version.
Funding
This study was supported by Social and People's Livelihood Science and Technology Innovation Special Project in Nantong City (ms12021065).
Conflict of interest
The authors declare that the research was conducted in the absence of any commercial or financial relationships that could be construed as a potential conflict of interest.
Publisher's note
All claims expressed in this article are solely those of the authors and do not necessarily represent those of their affiliated organizations, or those of the publisher, the editors and the reviewers. Any product that may be evaluated in this article, or claim that may be made by its manufacturer, is not guaranteed or endorsed by the publisher.
References
1. Ma Q, Li R, Wang L, Yin P, Wang Y, Yan C, et al. Temporal trend and attributable risk factors of stroke burden in China, 1990-2019: an analysis for the Global Burden of Disease Study 2019. Lancet Public Health. (2021) 6:e897–906. doi: 10.1016/S2468-2667(21)00228-0
2. Prevalence i. and mortality of stroke in China: results from a nationwide population-based survey of 480 687 adults. Circulation. (2017) 135:759–71. doi: 10.1161/CIRCULATIONAHA.116.025250
3. Thom T, Haase N, Rosamond W, Howard VJ, Rumsfeld J, Manolio T, et al. Heart disease and stroke statistics−2006 update: a report from the American Heart Association Statistics Committee and Stroke Statistics Subcommittee. Circulation. (2006)113:e85–151. doi: 10.1161/CIRCULATIONAHA.105.171600
4. Wang Y, Meng X, Wang A, Xie X, Pan Y, Johnston SC, et al. Ticagrelor versus clopidogrel in CYP2C19 loss-of-function carriers with stroke or TIA. N Engl J Med. (2021) 385:2520–30. doi: 10.1056/NEJMoa2111749
5. Xiong Y, Yan R, Gu H, Wang S, Fisher M, Zhao X, et al. Intravenous thrombolysis in Chinese patients with mild acute ischemic stroke. Ann Transl Med. (2021) 9:767. doi: 10.21037/atm-21-40
6. Yang P, Zhang Y, Zhang L, Zhang Y, Treurniet KM, Chen W, et al. Endovascular thrombectomy with or without intravenous alteplase in acute stroke. N Engl J Med. (2020) 382:1981–93. doi: 10.1056/NEJMoa2001123
7. Wang J, Li F, Xiao L, Peng F, Sun W, Li M, et al. Depressed TSH level as a predictor of post-stroke fatigue in patients with acute ischemic stroke. Neurology. (2018) 91:e1971–78. doi: 10.1212/WNL.0000000000006534
8. Paciaroni M, Acciarresi M. Poststroke fatigue. Stroke. (2019) 50:1927–33. doi: 10.1161/STROKEAHA.119.023552
9. Sarfo FS, Berchie P, Singh A, Nichols M, Agyei-Frimpong M, Jenkins C, et al. Prevalence, trajectory, and predictors of poststroke fatigue among ghanaians. J Stroke Cerebrovasc Dis. (2019) 28:1353–61. doi: 10.1016/j.jstrokecerebrovasdis.2019.02.002
10. De Doncker W, Dantzer R, Ormstad H, Kuppuswamy A. Mechanisms of poststroke fatigue. J Neurol Neurosurg Psychiatry. (2018) 89:287–93. doi: 10.1136/jnnp-2017-316007
11. Alghamdi I, Ariti C, Williams A, Wood E, Hewitt J. Prevalence of fatigue after stroke: a systematic review and meta-analysis. Eur Stroke J. (2021) 6:319–32. doi: 10.1177/23969873211047681
12. Kim CH, Park JT, Kim EJ, Han JH, Han JS, Choi JY, et al. An increase in red blood cell distribution width from baseline predicts mortality in patients with severe sepsis or septic shock. Crit Care. (2013) 17:R282. doi: 10.1186/cc13145
13. Vayá A, Alis R, Hernández JL, Calvo J, Micó L, Romagnoli M, et al. RDW in patients with systemic lupus erythematosus. Influence of anaemia and inflammatory markers. Clin Hemorheol Microcirc. (2013) 54:333–9. doi: 10.3233/CH-131738
14. Jia X, Cheng S, Zhang L, Zheng Y, Zou H, Huang S, et al. Elevated red blood cell distribution width as a poor prognostic factor in patients with hematopoietic stem cell transplantation. Front Oncol. (2021) 10:565265. doi: 10.3389/fonc.2020.565265
15. Chen M, Liao L, Yan J, Lin FQ. Predictive value of red blood cell distribution width for 1-year all-cause mortality in critically Ill patients with acute myocardial infarction. Int J Gen Med. (2022)15:465–71. doi: 10.2147/IJGM.S345109
16. Li Y, Zhang M, Dong C, Xue M, Li J, Wu G. Elevated red blood cell distribution width levels at admission predicts depression after acute ischemic stroke: a 3-month follow-up study. Neuropsychiatr Dis Treat. (2022)18:695–704. doi: 10.2147/NDT.S351136
17. Sun H, Li S, Xu Z, Liu C, Gong P, Deng Q, et al. SNHG15 is a negative regulator of inflammation by mediating TRAF2 ubiquitination in stroke-induced immunosuppression. J Neuroinflammation. (2022) 19:1. doi: 10.1186/s12974-021-02372-z
18. Zhang Y, Xing Z, Zhou K, Jiang S. The predictive role of systemic inflammation response index (SIRI) in the prognosis of stroke patients. Clin Int Aging. (2021) 16:1997–2007. doi: 10.2147/CIA.S339221
19. Przykaza Ł. Understanding the connection between common stroke comorbidities, their associated inflammation, and the course of the cerebral Ischemia/reperfusion cascade. Front Immunol. (2021) 12:782569. doi: 10.3389/fimmu.2021.782569
20. Franx BAA, Van der Toorn A, Van Heijningen C, Vivien D, Bonnard T, Dijkhuizen RM. Molecular magnetic resonance imaging of vascular inflammation after recanalization in a rat ischemic stroke model. Stroke. (2021) 52:e788–91. doi: 10.1161/STROKEAHA.121.034910
21. Wu M, Zhang X, Chen J, Zha M, Yuan K, Huang K, et al. A score of low-grade inflammation for predicting stroke recurrence in patients with Ischemic stroke. J Inflamm Res. (2021) 14:4605–14. doi: 10.2147/JIR.S328383
22. Esenwa C, Cheng NT, Luna J, Willey J, Boehme AK, Kirchoff-Torres K, et al. Biomarkers of coagulation and inflammation in COVID-19-associated ischemic stroke. Stroke. (2021) 52:e706–09. doi: 10.1161/STROKEAHA.121.035045
23. Lappé JM, Horne BD, Shah SH, May HT, Muhlestein JB, Lappé DL, et al. Red cell distribution width, C-reactive protein, the complete blood count, and mortality in patients with coronary disease and a normal comparison population. Clin Chim Acta. (2011) 412:2094–9. doi: 10.1016/j.cca.2011.07.018
24. Montagnana M, Cervellin G, Meschi T, Lippi G. The role of red blood cell distribution width in cardiovascular and thrombotic disorders. Clin Chem Lab Med. (2011) 50:635–41. doi: 10.1515/cclm.2011.831
25. Semba RD, Patel KV, Ferrucci L, Sun K, Roy CN, Guralnik JM, et al. Serum antioxidants and inflammation predict red cell distribution width in older women: the Women's Health and Aging Study I. Clin Nutr. (2010) 29:600–4. doi: 10.1016/j.clnu.2010.03.001
26. Vayá A, Hernández V, Rivera L, Hernández JL, Lago A, España F, et al. Red blood cell distribution width in patients with cryptogenic stroke. Clin Appl Thromb Hemost. (2015) 21:241–5. doi: 10.1177/1076029614547262
27. Jia H, Li H, Zhang Y, Li C, Hu Y, Xia C. Association between red blood cell distribution width (RDW) and carotid artery atherosclerosis (CAS) in patients with primary ischemic stroke. Arch Gerontol Geriatr. (2015) 61:72–5. doi: 10.1016/j.archger.2015.04.005
28. Turcato G, Cappellari M, Follador L, Dilda A, Bonora A, Zannoni M, et al. Red blood cell distribution width is an independent predictor of outcome in patients undergoing thrombolysis for ischemic stroke. Semin Thromb Hemost. (2017) 43:30–5. doi: 10.1055/s-0036-1592165
29. Wang C, Wang L, Zhong D, Deng L, Qiu S, Li Y, et al. Association between red blood cell distribution width and hemorrhagic transformation in acute ischemic stroke patients. Cerebrovasc Dis. (2019) 48:193–9. doi: 10.1159/000504742
30. Song SY, Hua C, Dornbors III D, Kang RJ, Zhao XX, Du X, et al. Baseline red blood cell distribution width as a predictor of stroke occurrence and outcome: a comprehensive meta-analysis of 31 studies. Front Neurol. (2019) 10:1237. doi: 10.3389/fneur.2019.01237
Keywords: acute ischemic stroke, post-stroke fatigue, red blood cell distribution width, restricted cubic spline, biomarker
Citation: Peng M, Chen Y, Chen Y, Feng K, Shen H, Huang H, Zhao W, Zou H and Ji J (2022) The relationship between red blood cell distribution width at admission and post-stroke fatigue in the acute phase of acute ischemic stroke. Front. Neurol. 13:922823. doi: 10.3389/fneur.2022.922823
Received: 09 May 2022; Accepted: 06 July 2022;
Published: 29 July 2022.
Edited by:
Keith Pennypacker, University of Kentucky, United StatesCopyright © 2022 Peng, Chen, Chen, Feng, Shen, Huang, Zhao, Zou and Ji. This is an open-access article distributed under the terms of the Creative Commons Attribution License (CC BY). The use, distribution or reproduction in other forums is permitted, provided the original author(s) and the copyright owner(s) are credited and that the original publication in this journal is cited, in accordance with accepted academic practice. No use, distribution or reproduction is permitted which does not comply with these terms.
*Correspondence: Meidi Peng, cGVuZ21laWRpQG50dS5lZHUuY24=