- 1Department of Radiology, General Hospital of Northern Theater Command, Shenyang, China
- 2Department of Orthopedics, Chinese PLA General Hospital, Beijing, China
- 3Department of Radiology, Changhai Hospital, Shanghai, China
Machine learning (ML) has been proposed for lesion segmentation in acute ischemic stroke (AIS). This study aimed to provide a systematic review and meta-analysis of the overall performance of current ML algorithms for final infarct prediction from baseline imaging. We made a comprehensive literature search on eligible studies developing ML models for core infarcted tissue estimation on admission CT or MRI in AIS patients. Eleven studies meeting the inclusion criteria were included in the quantitative analysis. Study characteristics, model methodology, and predictive performance of the included studies were extracted. A meta-analysis was conducted on the dice similarity coefficient (DSC) score by using a random-effects model to assess the overall predictive performance. Study heterogeneity was assessed by Cochrane Q and Higgins I2 tests. The pooled DSC score of the included ML models was 0.50 (95% CI 0.39–0.61), with high heterogeneity observed across studies (I2 96.5%, p < 0.001). Sensitivity analyses using the one-study removed method showed the adjusted overall DSC score ranged from 0.47 to 0.52. Subgroup analyses indicated that the DL-based models outperformed the conventional ML classifiers with the best performance observed in DL algorithms combined with CT data. Despite the presence of heterogeneity, current ML-based approaches for final infarct prediction showed moderate but promising performance. Before well integrated into clinical stroke workflow, future investigations are suggested to train ML models on large-scale, multi-vendor data, validate on external cohorts and adopt formalized reporting standards for improving model accuracy and robustness.
Introduction
Stroke is a life-threatening disease accounting for approximately 10% of all deaths and presenting an estimated lifetime risk of 25% worldwide (1). Recanalization of the occluded vessels is the only effective treatment to restore blood flow and prevent neural functional deterioration. Early studies suggested 4.5 and 6 h as the time window for intravenous thrombolysis (IVT) and endovascular thrombectomy (EVT) from symptoms onset (2–4). Recent advances in endovascular approaches have broadened the boundaries of eligible patient selection and expanded the time window to 24 h by using advanced neuroimaging (5, 6).
Currently, acute stroke imaging allows estimating the ischemic core and penumbra by predefined imaging thresholds. An apparent diffusion coefficient threshold between 600 and 625 × 10−3 mm2/s remains a robust parameter for infarct core estimation, and a decreased relative cerebral blood flow (rCBF) threshold of <30% has been extensively used to quantify final infarct size for CT-based method. The mismatch between infarct core and perfusion deficit identified by time to maximum of the residue function (Tmax) with a delay >6s provides a delineation of tissue at risk (7, 8). Despite the easy application of using single-valued thresholds to predict ischemic tissue outcome, conventional thresholds derived from approximate linear statistic models would probably fail to capture the heterogeneity of stroke lesion development from baseline imaging. Moreover, thresholds based on a single imaging modality disregarded the complementary effect of multimodal imaging, thus limiting the reliability in delineating infarct lesions.
Recent advances in machine learning (ML) offer promising applications in medical imaging by learning informative features and patterns from structured input data. It also drives the emergence of deep learning (DL) subfield, which has shown impressive results in medical image processing without prior selection for relevant features (9, 10). Given the suboptimal performance of the conventional thresholding methods, initial studies attempted to apply ML and DL-based approaches and showed clear advantages for more precise prediction of the final infarct lesion from baseline imaging (11–17). These promising results inspired investigators to propose novel model methodologies by improving algorithm architectures, combining multi-modality input parameters, and applying in different clinical scenarios.
Although studies on this topic are growing, there is a lack of studies that review the general applications of the state-of-the-art ML-based approaches in ischemic core estimation. Therefore, we conducted this systematic review and meta-analysis to provide an overview of the potential advantages and remaining challenges of ML-based model methodologies for final infarct lesion prediction from acute stroke imaging, evaluate the overall performance of existing approaches, and provide suggestions for future research to potentially aid in acute ischemic stroke (AIS) management.
Methods
This systematic review and meta-analysis was performed following the Preferred Reporting Items for Systematic Reviews and Meta-Analyses (PRISMA) statement (18).
Literature Search and Study Selection
We comprehensively searched PubMed, EMBASE, Cochrane Library, Science Direct, Springer, and IEEE Xplore Digital Library databases from inception to May 31, 2022, with the following keywords: “machine learning”, “deep learning”, “neural network”, “stroke”, “cerebrovascular event”, “cerebral infarct”, “computed tomography”, “magnetic resonance imaging”. Studies that developed ML algorithms for predicting the final infarct lesion from baseline acute stroke imaging were included. Eligible studies satisfying the following inclusion criteria were included in the meta-analysis: (1) study cohort was AIS patients; (2) study described ML algorithms for predicting ischemic core tissue from baseline CT or MR imaging; (3) reference standard (i.e., ground truth) was true infarct lesion segmented on follow-up imaging; (4) prediction performance was reported as dice similarity coefficient (DSC) score; (5) imaging sets for algorithm training and test were clearly defined; (6) published articles with full text; and (7) English language articles. Review articles, conference abstracts, letters, case reports including fewer than 10 patients, and non-human research were excluded. If studies came from the same cohort or compared different algorithms on the same dataset, we only retained the article with the largest sample size or the best-performing algorithm in the quantitative synthesis in case sample duplicate or overlapping would affect the overall pooled effect size.
One investigator (XW) read the titles and abstracts of all records. After preliminary screening, potentially eligible articles were shortlisted. Two investigators (XW and YF) independently read the full-text articles to assess eligibility, with disagreements resolved by discussion and consensus.
Data Extraction and Quality Assessment
Two investigators (XW and YF) independently extracted data from the included studies using a predefined data extraction sheet. Disagreements were re-evaluated and determined by a third investigator (NZ). The extracted data included: (1) first author and year; (2) source of the dataset; (3) sample size including the total patient number and numbers of the training, validation, and test sets; (4) model methodology, including algorithm types, input parameters and standard reference; (5) predictive performance, including the primary performance metric of DSC score and secondary metrics of area under the receiver operating characteristic curve (AUC), sensitivity, specificity, accuracy, precision, recall and volume error between the prediction result and the standard reference.
To assess the quality of ML-based diagnostic accuracy studies, Collins and Moons initially introduced a modified version of the Transparent Reporting of a Multivariable Prediction Model for Individual Prognosis or Diagnosis statement specific to machine learning (TRIPOD-ML) (19). However, the TRIPOD-ML guideline was complicated and covered a broad range of ML applications. The Radiology editorial board has developed a list of nine key considerations to improve the soundness and applicability of artificial intelligence research in diagnostic imaging (20). We adapted these items as quality assessment criteria in our study. Two investigators (XW and YF) independently evaluated the risks of bias using this questionnaire, with disagreements resolved by discussion and consensus.
Statistical Assessment
We estimated the overall performance of the ML models by using the DSC score, a commonly used volume-based performance metric for target segmentation. The DSC score represents the overlap between the prediction segmentation and the standard reference, ranging from 0 (indicates no overlap) to 1 (indicates complete overlap). For effect size calculation in the meta-analysis, the mean DSC score with standard deviation (SD) or 95% confidence interval (CI) was required. When study reported the DSC score as median and interquartile range (IQR), the mean and SD was converted using a quantile estimating method described by Wan et al. (21). The sample mean () and SD (S) were estimated as follows, where q1 referred to the first quartile, m referred to the median, and q3 referred to the third quartile.
A random-effects model meta-analysis was performed, and forest plots were generated to depict the effect size of individual studies and overall performance. The heterogeneity across the included studies was assessed using the Cochrane Q and Higgins I2 tests, where the p-value <0.05 in Cochrane Q test and Higgins I2-value > 75% indicated significant heterogeneity (22). Due to the high heterogeneity observed in this study, sensitivity analysis using the one-study removed method was conducted to explain the heterogeneity of the results. Subgroup analyses were performed according to algorithm types (conventional ML classifiers and deep neural networks) and imaging modality for model input (CT and MR data). Publication bias was examined by creating a funnel plot and Egger's bias test (23). Statistical analyses were performed using the STATA 17.0 statistical package (StataCorp, Stata Statistical Software). Two-sided p-value <0.05 was considered statistically significant.
Results
A total of 3,298 publications were initially identified through database searching. After removing 281 duplicate records, the remaining 3,017 publications were screened preliminarily. Based on title and abstract, 2,870 articles were excluded, and 147 articles were assessed for eligibility by two investigators independently. After full-text review, 38 studies were included in the systematic review. Among them, 11 studies that met the inclusion criteria and provided sufficient quantitative data were included in the meta-analysis, and 27 studies were excluded for the following reasons: 14 studies proposed ML models trained and tested on duplicate datasets, 6 studies didn't clearly define the training and testing sets, 7 studies didn't report a complete DSC score with standard deviation or interquartile range. The literature search flow diagram is presented in Figure 1.
Study Characteristics and Model Methodology: A Systematic Review
Based on full-text evaluation, 38 studies were included in the systematic review. Study characteristics, model methodology, and predictive performance are summarized in Table 1. Of the 38 included studies, 12 studies were based on single-center datasets (11, 12, 24, 25, 30, 34, 38, 41, 45–47, 52), and 26 studies were conducted on multicenter datasets, including 9 on registered clinical trials [13–17, 34–37] and 13 on two publicly available databases (Ischemic Stroke Lesion Segmentation [ISLES] challenges 2017 and 2018, http://www.isles-challenge.org) (27–29, 32, 37, 39, 40, 42, 44, 49–51, 53). All except two studies (45, 48) reported validation methods for the proposed model including using an independent test set, k-fold cross-validation, and leave-one-out cross-validation. External validation was only performed by one study (13).

Table 1. Study characteristics, model methodology, and predictive performance of the included studies.
Thirteen studies adopted conventional ML algorithms including k-nearest neighbor classification (24), general linear regression (47), random forest (13, 15, 25, 34, 36, 38, 41, 48) and gradient boosting (11, 26, 36) classifiers. Twenty-five studies proposed DL-based approaches consisting of artificial neural network (ANN) (31) and various types of convolutional neural network (CNN) with some of the noteworthy popular architectures, including 2D and 3D U-Net (12, 16, 17, 27, 28, 39, 40, 43, 49, 50), residual network (ResNet) (12, 29, 37, 50), recurrent residual U-Net (R2U-Net) (52) and DeepMedic (32). Four studies applied modifications of the common rectified linear unit (ReLU) activation function for non-linear transformation after each convolution operation, including parametric ReLU, noisy ReLU, and leaky ReLU activation (32, 33, 40, 51). Given the class imbalance issue, 6 studies used hybrid loss function methods for target lesion segmentation (12, 16, 17, 29, 42, 52). Four studies introduced optimization strategies such as data augmentation for the training procedure (28, 29, 37, 39). The reference standard for model training was actual infarct lesion manually or semi-automatically segmented on follow-up CT or MR images with a wide-range time interval from 1 h to 90 days from baseline imaging.
Eleven studies used CT perfusion source data and parametric maps as model input for core infarct estimation (12, 13, 29, 31, 33, 42, 44, 47, 50, 53, 54), including one study generating a synthesized pseudo-DWI map based on CTP parametric maps (42). Five studies used source images and features derived from non-contrast CT (41, 52) and CT angiography (15, 45, 46). Twenty-two studies adopted different combinations of MRI sequences including T1WI, T2WI, diffusion and perfusion for infarct core prediction (11, 14, 16, 17, 24–28, 30, 32, 34–40, 43, 48, 49, 51). In addition to imaging data, 6 studies added clinical information into the model inputs, such as stroke severity quantified by the National Institutes of Health Stroke Scale [NIHSS] and modified Rankin Scale [mRS] scores, recanalization status assessed by modified Thrombolysis in Cerebral Infarction [mTICI] score and time variants of onset-to-imaging time and onset-to-treatment time (12, 23, 26, 41, 43, 45, 46).
Outcome measures were heterogeneous across studies. As infarct core estimation is a prediction segmentation task, model performance was commonly evaluated using the DSC score in all except 7 studies (14, 24, 26, 30, 41, 45, 46). Other metrics such as AUC, sensitivity, and specificity for classification results, accuracy, precision and recall for detection results, and a clinically intuitive metric of volume error were also employed and summarized in Table 1.
Performance for Core Infarcted Tissue Prediction: A Meta-Analysis
Eleven studies were included in the meta-analysis (11, 13, 16, 25, 31, 38, 40, 43, 50, 52, 54). Methodological quality assessment of the included studies is shown in Table 2. Three studies clearly defined all three image sets of training, validation, and test (11, 16, 31). Only one study determined model performance using an external test set (13). Imaging data from one study were collected from four major manufacturers (44), five studies reported using single-vendor data (11, 25, 38, 40, 54), and others remained unknown (13, 16, 31, 43, 52). Although all the included studies clearly defined the validation methods, the relationship between the number of training images and model performance (i.e., sample size estimation) was not carefully evaluated. All studies described the data pre-processing procedure, trained their models using acceptable reference standards, and demonstrated the predictive performance assessed by multiple performance metrics. Algorithms from two studies were partially publicly available via the website of GitHub (11, 40).
The overall performance of 11 predictive models is presented in Figure 2. The pooled DSC score was 0.50 (95% CI 0.39–0.61). The value of Cochrane Q test p < 0.001 and Higgins I2 of 96.5%, indicating high heterogeneity across the included studies. We conducted a sensitivity analysis by removing one study at each step (Figure 3). The adjusted overall DSC score ranged from 0.47 (95% CI 0.41–0.53) after removing the study by Zhu et al. (54) to 0.52 (95% CI 0.41–0.63) after removing the study by McKinley et al. (25). Publication bias assessed by graphic funnel plot showed an asymmetrical shape, and not all studies were plotted within the area under the curve of the pseudo-95% CI, indicating the potential publication bias among included studies (Figure 4). Egger's test showed no statistically significant publication bias (p = 0.565).
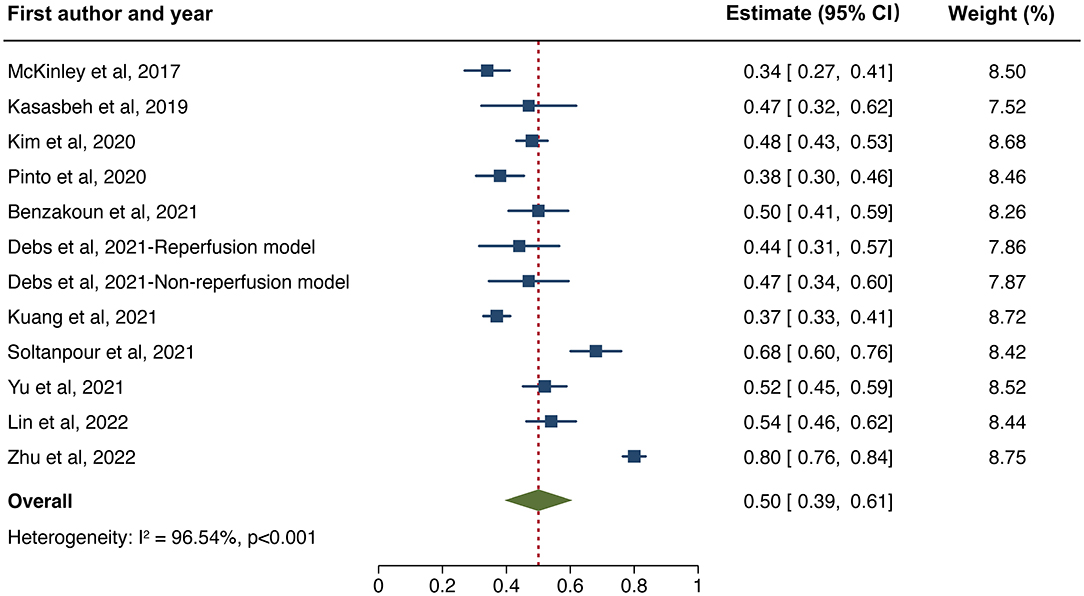
Figure 2. Forest plot of the included studies that assessed the performance of infarct tissue outcome prediction. Forest plot shows that the dice similarity coefficient (DSC) representing the performance of the machine learning-based approaches for final infarct prediction centers around 0.50 with a 95% confidence interval (CI) ranging from 0.39 to 0.61.
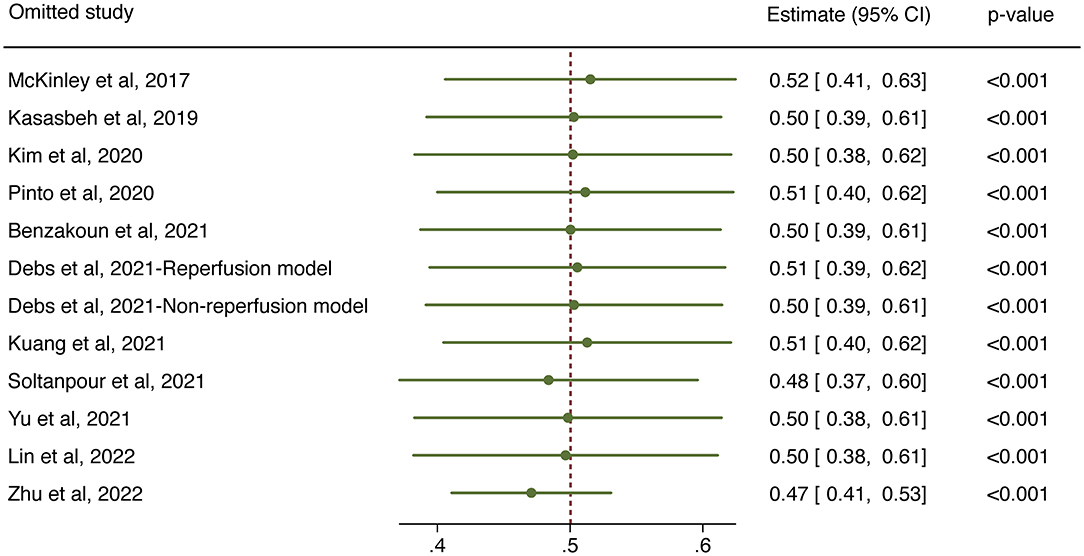
Figure 3. Sensitivity analysis for the overall predictive performance using one-study-removed method.
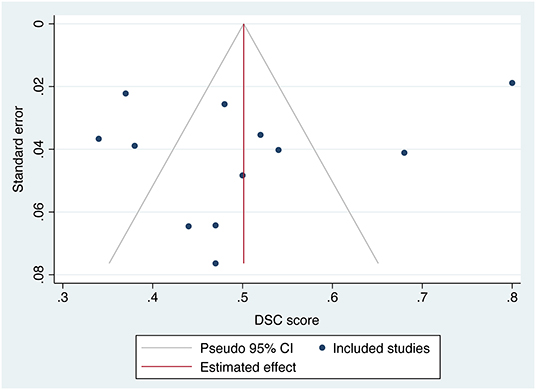
Figure 4. Funnel plot of the included studies. The effect size of mean dice similarity coefficient (DSC) score was displayed on the horizontal axis. Standard error was plotted on the vertical axis.
We made further subgroup analyses by algorithm types and imaging modality. Forest plot for each subgroup is depicted in Figure 5. For studies applying conventional ML classifiers, the pooled DSC score was 0.44 (95%CI 0.34–0.54) for models inputting MR data (11, 25, 38) and 0.37 (95%CI 0.33–0.41) for a single model using CT data as a reference (13). For studies developing DL-based approaches, the pooled DSC was 0.45 (95%CI 0.38–0.53) for models inputting MR data (16, 40, 43) and 0.63 (95%CI 0.48–0.78) when using CT data (31, 50, 52, 54). Sustained high heterogeneity was observed in the subgroup of DL models combined with CT data (Higgins I2 93.9%, p < 0.001). Sensitivity analysis revealed that after removing one study (54), the adjusted pooled DSC score was 0.59 (95%CI 0.54–0.64), with a downward trend of heterogeneity (Higgins I2 of 77.3%, p = 0.012).
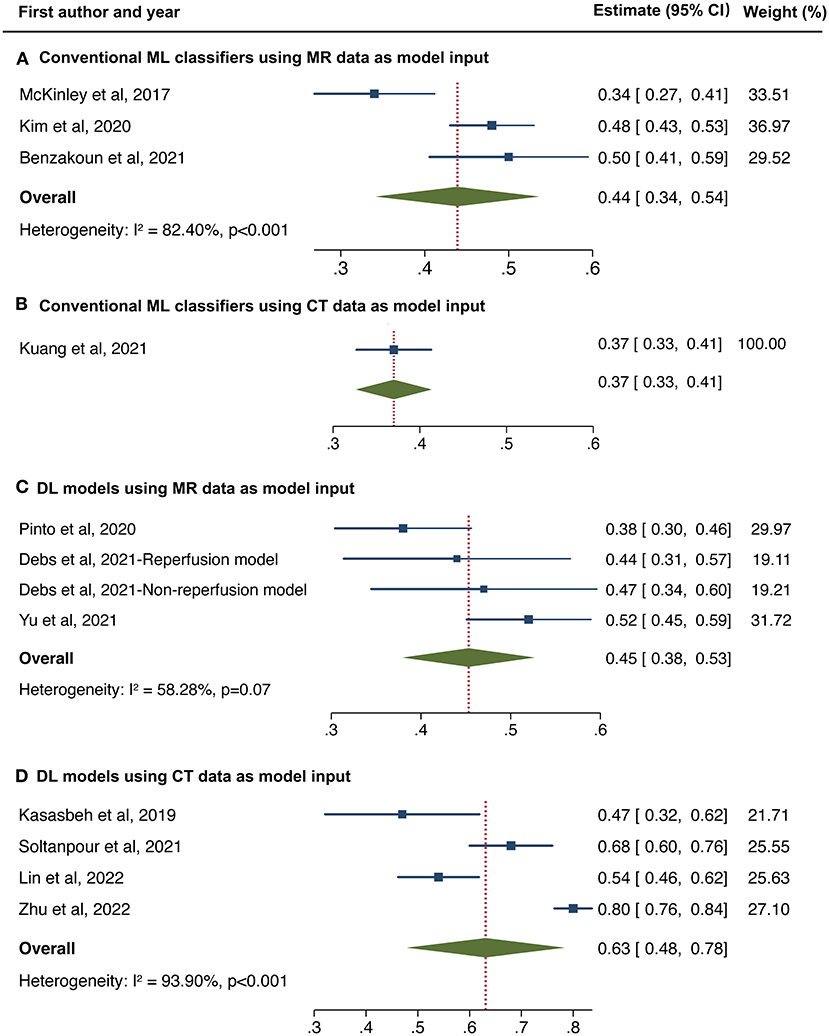
Figure 5. Forest plot of subgroup analyses in conventional machine learning (ML) classifiers using MR data (A) and CT data (B) as model input, and deep learning models using MR data (C) and CT data (D) as model input, respectively.
Discussion
In this study, we reviewed the performance of ML-based approaches for final infarct lesion prediction from acute stroke imaging. The overall predictive performance of ML algorithms was moderate with a pooled DSC score of 0.50 (95% CI 0.39–0.61, Higgins I2 = 96.5%, p < 0.001). Subgroup analyses indicated that the DL-based models outperformed the conventional ML classifiers with the best performance observed in DL algorithms combined with pre-processing CT data. Although high heterogeneity was present across studies, current ML algorithms still showed promising performance for ischemic tissue outcome prediction from baseline imaging.
Estimating the final infarct lesion from baseline imaging is complex due to the heterogeneity of lesion shape, location, and progression. The aim of ML applications is to exact the maximum amount of predictive power from the available multi-modality imaging information, where conventional thresholding methods seem inadequate (10). A few studies validated their proposed ML-based approaches compared to conventional thresholds for core infarcted tissue delineation and showed significant improvement in measurement results using ML-based methods (11, 13–15, 17). For instance, when training an attention-gated U-Net with baseline MR diffusion and perfusion parameters, the prediction model outperformed the ADC <620 × 10−6 mm2/s threshold, with more precise segmentation (DSC score 0.53 vs. 0.45), higher discriminating power (AUC 0.92 vs. 0.71) and smaller volume error (median 9 vs. 12 ml) (14). Such strategies take advantage of the data-processing ability of ML algorithms to provide rapid and reliable assessment, which is promising to support clinical management.
Conventional ML classifiers included linear regression and decision trees. Grosser et al. compared the performance of 3 classical ML algorithms for infarct core estimate and revealed that decision trees (random forest and gradient boosting) performed better than linear regression model (36), indicating the necessity of using non-linear algorithms for stroke prediction segmentation. In the 2017 ISLES challenge, where uniform pre-processing data for model training and test were provided, almost all top-ranking teams employed DL algorithms instead of ML classifiers (28). Our finding was consistent with the results of ISLES challenge 2017, which indicated the advantages of using DL algorithms for final infarct prediction. However, a recent study held contradictory results that a U-net model performed less well than two decision tree classifiers (DSC score 0.48 vs. 0.53 and 0.51) (11). A possible reason was the relatively small sample size for fully training a DL algorithm. The inherent data-dependent characteristic of DL algorithms meant that once trained on sufficient data, the model performance would continue to improve, while classical ML approaches tend toward stability. Moreover, most well-performed DL models were customized on the baseline architectures. Innovative modifications of algorithm architectures and training strategies would further improve the predictive performance of DL models.
Most of the previous works have chosen MR images for model training, given the high tissue contrast and the sensitivity of MR diffusion for infarct core detection. Our study revealed that the DSC score of MR-input models stabilized at around 0.45, either using ML classifiers or DL models. In clinical practice, CT is more widely available for acute stroke triage, detecting large vessel occlusion, and selecting candidates for revascularization (8, 55). Studies developing models training on CT perfusion data appeared late yet achieved comparable or better performance than MR-input models (12, 29, 31, 33, 42, 44, 47, 50, 52, 53). One study employing a random forest classifier using features extracted from multi-phase CT angiography presented less satisfactory performance with a DSC score of 0.22 (15). However, a more recent study based on the R2U-RNet algorithm using non-contrast CT data with intensity normalization and histogram equalization showed promising performance with a DSC score of 0.54 (52). Theoretically, different imaging modalities and parameters provide complementary information, and thus the combination of multimodal imaging data with reasonable pre-processing would enhance the overall predictive performance. In addition, several approaches incorporated clinical data such as stroke severity, reperfusion status, and time variants (13, 28, 33, 34, 48). Multi-dimensional input information consisting of imaging and non-imaging data is expected to establish better prediction models, which is a direction of future research.
In our study, we have chosen the DSC score as the primary performance metric, a commonly used volume-based metric containing lesion size and location information for target segmentation. Other segmentation metrics, such as Jaccard index was less reported in this research field, and Hausdorff distance and surface distance were distance-based metrics that were less optimal for final infarct lesion prediction (56). Although ROC is more familiar in diagnostic accuracy studies, its efficacy has been challenged for class imbalance tissue, such as infarct core prediction. Large numbers of “healthy” voxels would lead the AUC values to a high level and reduce its discriminating power. From a clinical standpoint, we included the volume error as a secondary performance metric, which enabled intuitive assessment of the size differences between prediction results and reference standards, as the estimate of core infarct volume was critical to identify eligible patients who would benefit from treatment in the late time window.
Although ML-based approaches provided promising results for final infarct lesion prediction, there is still no wide acceptance and implementation in clinical practice. Most of the proposed models were trained on datasets with small sample size, which was deemed insufficient to train an ML algorithm (especially a DL algorithm), leading to an overall moderate predictive performance. Many studies validated using the k-fold cross-validation method to provide an unbiased evaluation with small sample size. However, the real predictive performance would be overestimated without an independent external validation (57). Another limitation was data heterogeneity, as models trained on single-center cohort using single-vendor data would reduce the model generalizability. One study validating their approach on an external cohort indicated less satisfactory performance with a median DSC score of 0.39 (13). There is an emerging trend to build up large multi-vendor, multi-institution diagnostic datasets with initial implementation on chest X-ray data (56). A similar dataset for stroke lesion segmentation would be helpful. In addition, a standardized methodologic procedure is also warranted including the definition of the clinical cohort, imaging protocols, reference standard, model training and validation process, and clinical evaluation of model performance.
Our study has several limitations. First, the heterogeneity was high across studies due to the differences in the study cohorts, algorithm types, and input parameters. We made sensitivity analyses and found no obvious deviation of the adjusted effect size from the main effect size. We also conducted subgroup analyses to explain the heterogeneity and found a downward trend of heterogeneity in the subgroup analyses. However, re-evaluation of the overall model performance is needed as more relevant, intensive studies accumulate. Second, as an emerging field of artificial intelligence in imaging, there was no consensus on the reporting standards. Therefore, 13 studies were excluded before the meta-analysis because of lacking definition of image sets or results of DSC scores, which might result in an incomplete assessment of available studies. Third, although the publication bias examined by Egger's test was not significant, the funnel plot showed an asymmetrical shape. We excluded 14 studies due to dataset duplicates or overlapping to avoid affecting the overall pooled effect size. It might contribute to the risk of publication bias.
Conclusion
In this study, we conducted a systematic review and meta-analysis of current studies using ML algorithms for infarct core prediction. Despite the heterogeneity across studies, the overall performance of ML-based predictive methods is moderate but promising, with better predictive performance presented in the DL-based approaches. However, before well integrated into clinical stroke workflow, future studies are suggested to train ML-based approaches on large-scale, multi-vendor data, validate on external cohorts and adopt formalized reporting standards for improving model accuracy and robustness.
Data Availability Statement
The original contributions presented in the study are included in the article/supplementary material, further inquiries can be directed to the corresponding author/s.
Author Contributions
XW conducted the literature search. XW, YF, and NZ participated in study selection and data collection. XW and YF analyzed the data and drafted the manuscript. NZ, JL, YD, and BY revised the manuscript for important intellectual content. BY designed the review protocol and he was the study guarantor. All authors approved the final version of the manuscript.
Funding
This study was supported by grant 2019-BS-267 from the Project of Scientific Research Foundation for the Ph.D. of Liaoning Province, and by grant 20-205-4-086 from the Project of Natural Science Foundation of Shenyang.
Conflict of Interest
The authors declare that the research was conducted in the absence of any commercial or financial relationships that could be construed as a potential conflict of interest.
Publisher's Note
All claims expressed in this article are solely those of the authors and do not necessarily represent those of their affiliated organizations, or those of the publisher, the editors and the reviewers. Any product that may be evaluated in this article, or claim that may be made by its manufacturer, is not guaranteed or endorsed by the publisher.
References
1. Feigin VL, Nguyen G, Cercy K, Johnson CO, Alam T, Parmar PG, et al. Global, regional, and country-specific lifetime risks of stroke, 1990 and 2016. N Engl J Med. (2018) 379:2429–37. doi: 10.1056/NEJMoa1804492
2. Hacke W, Kaste M, Bluhmki E, Brozman M, Dávalos A, Guidetti D, et al. Thrombolysis with alteplase 3 to 45 hours after acute ischemic stroke. N Engl J Med. (2008) 359:1317–29. doi: 10.1056/NEJMoa0804656
3. Wahlgren N, Ahmed N, Dávalos A, Hacke W, Millán M, Muir K, et al. Thrombolysis with alteplase 3-45 h after acute ischaemic stroke (SITS-ISTR): an observational study. Lancet. (2008) 372:1303–9. doi: 10.1016/S0140-6736(08)61339-2
4. Berkhemer OA, Fransen PS, Beumer D, van den Berg LA, Lingsma HF, Yoo AJ, et al. A randomized trial of intraarterial treatment for acute ischemic stroke. N Engl J Med. (2015) 372:11–20.
5. Nogueira RG, Jadhav AP, Haussen DC, Bonafe A, Budzik RF, Bhuva P, et al. Thrombectomy 6 to 24 hours after stroke with a mismatch between deficit and infarct. N Engl J Med. (2018) 378:11–21.
6. Albers GW, Marks MP, Kemp S, Christensen S, Tsai JP, Ortega-Gutierrez S, et al. Thrombectomy for stroke at 6 to 16 hours with selection by perfusion imaging. N Engl J Med. (2018) 378:708–18. doi: 10.1056/NEJMoa1713973
7. Demeestere J, Wouters A, Christensen S, Lemmens R, Lansberg MG. Review of perfusion imaging in acute ischemic stroke: from time to tissue. Stroke. (2020) 51:1017–24. doi: 10.1161/STROKEAHA.119.028337
8. de Oliveira EP, Fiebach JB, Vagal A, Schaefer PW, Aviv RI. Controversies in imaging of patients with acute ischemic stroke: AJR expert panel narrative review. AJR Am J Roentgenol. (2021) 217:1027–37. doi: 10.2214/AJR.21.25846
9. Choy G, Khalilzadeh O, Michalski M, Do S, Samir AE, Pianykh OS, et al. Current applications and future impact of machine learning in radiology. Radiology. (2018) 288:318–28. doi: 10.1148/radiol.2018171820
10. Chavva IR, Crawford AL, Mazurek MH, Yuen MM, Prabhat AM, Payabvash S, et al. Deep learning applications for acute stroke management. Ann Neurol. (2022).
11. Benzakoun J, Charron S, Turc G, Hassen WB, Legrand L, Boulouis G, et al. Tissue outcome prediction in hyperacute ischemic stroke: comparison of machine learning models. J Cereb Blood Flow Metab. (2021) 41:3085–96. doi: 10.1177/0271678X211024371
12. He Y, Luo Z, Zhou Y, Xue R, Li J, Hu H, et al. U-net models based on computed tomography perfusion predict tissue outcome in patients with different reperfusion patterns. Transl Stroke Res. (2022). doi: 10.1007/s12975-022-00986-w
13. Kuang H, Qiu W, Boers AM, Brown S, Muir K, Majoie C, et al. Computed tomography perfusion-based machine learning model better predicts follow-up infarction in patients with acute ischemic stroke. Stroke. (2021) 52:223–31. doi: 10.1161/STROKEAHA.120.030092
14. Nielsen A, Hansen MB, Tietze A, Mouridsen K. Prediction of tissue outcome and assessment of treatment effect in acute ischemic stroke using deep learning. Stroke. (2018) 49:1394–401. doi: 10.1161/STROKEAHA.117.019740
15. Qiu W, Kuang H, Ospel JM, Hill MD, Demchuk AM, Goyal M, et al. Automated prediction of ischemic brain tissue fate from multiphase computed tomographic angiography in patients with acute ischemic stroke using machine learning. J Stroke. (2021) 23:234–43. doi: 10.5853/jos.2020.05064
16. Yu Y, Xie Y, Thamm T, Gong E, Ouyang J, Christensen S, et al. Tissue at risk and ischemic core estimation using deep learning in acute stroke. AJNR Am J Neuroradiol. (2021) 42:1030–7. doi: 10.3174/ajnr.A7081
17. Yu Y, Xie Y, Thamm T, Gong E, Ouyang J, Huang C, et al. Use of deep learning to predict final ischemic stroke lesions from initial magnetic resonance imaging. JAMA Netw Open. (2020) 3:e200772. doi: 10.1001/jamanetworkopen.2020.0772
18. Liberati A, Altman DG, Tetzlaff J, Mulrow C, Gøtzsche PC, Ioannidis JP, et al. The PRISMA statement for reporting systematic reviews and meta-analyses of studies that evaluate healthcare interventions: explanation and elaboration. BMJ. (2009) 339:b2700. doi: 10.1136/bmj.b2700
19. Collins GS, Moons KGM. Reporting of artificial intelligence prediction models. Lancet. (2019) 393:1577–9. doi: 10.1016/S0140-6736(19)30037-6
20. Bluemke DA, Moy L, Bredella MA, Ertl-Wagner BB, Fowler KJ, Goh VJ, et al. Assessing radiology research on artificial intelligence: a brief guide for authors, reviewers, and readers from the Radiology editorial board. Radiology. (2020) 294:487–9. doi: 10.1148/radiol.2019192515
21. Wan X, Wang W, Liu J, Tong T. Estimating the sample mean and standard deviation from the sample size, median, range and/or interquartile range. BMC Med Res Methodol. (2014) 14:135. doi: 10.1186/1471-2288-14-135
22. Higgins JP, Thompson SG, Deeks JJ, Altman DG. Measuring inconsistency in meta-analyses. BMJ. (2003) 327:557–60. doi: 10.1136/bmj.327.7414.557
23. Egger M, Davey Smith G, Schneider M, Minder C. Bias in meta-analysis detected by a simple, graphical test. BMJ. (1997) 315:629–34. doi: 10.1136/bmj.315.7109.629
24. Gottrup C, Thomsen K, Locht P, Wu O, Sorensen AG, Koroshetz WJ, et al. Applying instance-based techniques to prediction of final outcome in acute stroke. Artif Intell Med. (2005) 33:223–36. doi: 10.1016/j.artmed.2004.06.003
25. McKinley R, Häni L, Gralla J, El-Koussy M, Bauer S, Arnold M, et al. Fully automated stroke tissue estimation using random forest classifiers (FASTER). J Cereb Blood Flow Metab. (2017) 37:2728–41. doi: 10.1177/0271678X16674221
26. Livne M, Boldsen JK, Mikkelsen IK, Fiebach JB, Sobesky J, Mouridsen K. Boosted tree model reforms multimodal magnetic resonance imaging infarct prediction in acute stroke. Stroke. (2018) 49:912–8. doi: 10.1161/STROKEAHA.117.019440
27. Pinto A, McKinley R, Alves V, Wiest R, Silva CA, Reyes M. Stroke lesion outcome prediction based on MRI imaging combined with clinical information. Front Neurol. (2018) 9:1060. doi: 10.3389/fneur.2018.01060
28. Winzeck S, Hakim A, McKinley R, Pinto J, Alves V, Silva C, et al. ISLES 2016 and 2017-benchmarking ischemic stroke lesion outcome prediction based on multispectral MRI. Front Neurol. (2018) 9:679.
29. Clèrigues A, Valverde S, Bernal J, Freixenet J, Oliver A, Lladó X. Acute ischemic stroke lesion core segmentation in CT perfusion images using fully convolutional neural networks. Comput Biol Med. (2019) 115:103487. doi: 10.1016/j.compbiomed.2019.103487
30. Ho KC, Scalzo F, Sarma KV, Speier W, El-Saden S, Arnold C. Predicting ischemic stroke tissue fate using a deep convolutional neural network on source magnetic resonance perfusion images. J Med Imaging. (2019) 6:026001. doi: 10.1117/1.JMI.6.2.026001
31. Kasasbeh AS, Christensen S, Parsons MW, Campbell B, Albers GW, Lansberg MG. Artificial neural network computer tomography perfusion prediction of ischemic core. Stroke. (2019) 50:1578–81. doi: 10.1161/STROKEAHA.118.022649
32. Pérez Malla CU, Valdés Hernández MDC, Rachmadi MF, Komura T. Evaluation of enhanced learning techniques for segmenting ischaemic stroke lesions in brain magnetic resonance perfusion images using a convolutional neural network scheme. Front Neuroinform. (2019) 13:33. doi: 10.3389/fninf.2019.00033
33. Robben D, Boers AMM, Marquering HA, Langezaal L, Roos Y, van Oostenbrugge RJ, et al. Prediction of final infarct volume from native CT perfusion and treatment parameters using deep learning. Med Image Anal. (2020) 59:101589. doi: 10.1016/j.media.2019.101589
34. Winder AJ, Siemonsen S, Flottmann F, Thomalla G, Fiehler J, Forkert ND. Technical considerations of multi-parametric tissue outcome prediction methods in acute ischemic stroke patients. Sci Rep. (2019) 9:13208. doi: 10.1038/s41598-019-49460-y
35. Grosser M, Gellißen S, Borchert P, Sedlacik J, Nawabi J, Fiehler J, et al. Localized prediction of tissue outcome in acute ischemic stroke patients using diffusion- and perfusion-weighted MRI datasets. PLoS ONE. (2020) 15:e0241917. doi: 10.1371/journal.pone.0241917
36. Grosser M, Gellißen S, Borchert P, Sedlacik J, Nawabi J, Fiehler J, et al. Improved multi-parametric prediction of tissue outcome in acute ischemic stroke patients using spatial features. PLoS ONE. (2020) 15:e0228113. doi: 10.1371/journal.pone.0228113
37. Hu X, Luo W, Hu J, Guo S, Huang W, Scott MR, et al. Brain SegNet: 3D local refinement network for brain lesion segmentation. BMC Med Imaging. (2020) 20:17. doi: 10.1186/s12880-020-0409-2
38. Kim YC, Kim HJ, Chung JW, Kim IG, Seong MJ, Kim KH, et al. Novel estimation of penumbra zone based on infarct growth using machine learning techniques in acute ischemic stroke. J Clin Med. (2020) 9:1977. doi: 10.3390/jcm9061977
39. Kumar A, Upadhyay N, Ghosal P, Chowdhury T, Das D, Mukherjee A, et al. CSNet: A new DeepNet framework for ischemic stroke lesion segmentation. Comput Methods Programs Biomed. (2020) 193:105524. doi: 10.1016/j.cmpb.2020.105524
40. Pinto A, Pereira S, Meier R, Wiest R, Alves V, Reyes M, et al. Combining unsupervised and supervised learning for predicting the final stroke lesion. Med Image Anal. (2021) 69:101888. doi: 10.1016/j.media.2020.101888
41. Qiu W, Kuang H, Teleg E, Ospel JM, Sohn SI, Almekhlafi M, et al. Machine learning for detecting early infarction in acute stroke with non-contrast-enhanced CT. Radiology. (2020) 294:638–44. doi: 10.1148/radiol.2020191193
42. Wang G, Song T, Dong Q, Cui M, Huang N, Zhang S. Automatic ischemic stroke lesion segmentation from computed tomography perfusion images by image synthesis and attention-based deep neural networks. Med Image Anal. (2020) 65:101787. doi: 10.1016/j.media.2020.101787
43. Debs N, Cho TH, Rousseau D, Berthezène Y, Buisson M, Eker O, et al. Impact of the reperfusion status for predicting the final stroke infarct using deep learning. Neuroimage Clin. (2021) 29:102548. doi: 10.1016/j.nicl.2020.102548
44. Hakim A, Christensen S, Winzeck S, Lansberg MG, Parsons MW, Lucas C, et al. Predicting infarct core from computed tomography perfusion in acute ischemia with machine learning: lessons from the ISLES challenge. Stroke. (2021) 52:2328–37. doi: 10.1161/STROKEAHA.120.030696
45. Hokkinen L, Mäkelä T, Savolainen S, Kangasniemi M. Evaluation of a CTA-based convolutional neural network for infarct volume prediction in anterior cerebral circulation ischaemic stroke. Eur Radiol Exp. (2021) 5:25. doi: 10.1186/s41747-021-00225-1
46. Hokkinen L, Mäkelä T, Savolainen S, Kangasniemi M. Computed tomography angiography-based deep learning method for treatment selection and infarct volume prediction in anterior cerebral circulation large vessel occlusion. Acta Radiol Open. (2021) 10:20584601211060347. doi: 10.1177/20584601211060347
47. Klug J, Dirren E, Preti MG, Machi P, Kleinschmidt A, Vargas MI, et al. Integrating regional perfusion CT information to improve prediction of infarction after stroke. J Cereb Blood Flow Metab. (2021) 41:502–10. doi: 10.1177/0271678X20924549
48. Modrau B, Winder A, Hjort N, Johansen MN, Andersen G, Fiehler J, et al. Machine learning-based prediction of brain tissue infarction in patients with acute ischemic stroke treated with theophylline as an add-on to thrombolytic therapy: a randomized clinical trial subgroup analysis. Front Neurol. (2021) 12:613029. doi: 10.3389/fneur.2021.613029
49. Pinto A, Amorim J, Hakim A, Alves V, Reyes M, Silva CA. Prediction of stroke lesion at 90-day follow-up by fusing raw DSC-MRI with parametric maps using deep learning. IEEE Access. (2021) 9:26260–70. doi: 10.1109/ACCESS.2021.3058297
50. Soltanpour M, Greiner R, Boulanger P, Buck B. Improvement of automatic ischemic stroke lesion segmentation in CT perfusion maps using a learned deep neural network. Comput Biol Med. (2021) 137:104849. doi: 10.1016/j.compbiomed.2021.104849
51. Vupputuri A, Gupta A, Ghosh N MCA-DN. Multi-path convolution leveraged attention deep network for salvageable tissue detection in ischemic stroke from multi-parametric MRI. Comput Biol Med. (2021) 136:104724. doi: 10.1016/j.compbiomed.2021.104724
52. Lin SY, Chiang PL, Chen PW, Cheng LH, Chen MH, Chang PC, et al. Toward automated segmentation for acute ischemic stroke using non-contrast computed tomography. Int J Comput Assist Radiol Surg. (2022) 17:661–71. doi: 10.1007/s11548-022-02570-x
53. Shi T, Jiang H, Zheng B. C2MA-Net: Cross-modal cross-attention network for acute ischemic stroke lesion segmentation based on CT perfusion scans. IEEE Trans Biomed Eng. (2022) 69:108–18. doi: 10.1109/TBME.2021.3087612
54. Zhu H, Chen Y, Tang T, Ma G, Zhou J, Zhang J, et al. ISP-Net: Fusing features to predict ischemic stroke infarct core on CT perfusion maps. Comput Methods Programs Biomed. (2022) 215:106630. doi: 10.1016/j.cmpb.2022.106630
55. Powers WJ, Rabinstein AA, Ackerson T, Adeoye OM, Bambakidis NC, Becker K, et al. 2018 Guidelines for the early management of patients with acute ischemic stroke: a guideline for healthcare professionals from the American Heart Association/American Stroke Association. Stroke. (2018) 49:e46–e110. doi: 10.1161/STR.0000000000000158
56. Lin H, Xiao H, Dong L, Teo KB, Zou W, Cai J, et al. Deep learning for automatic target volume segmentation in radiation therapy: a review. Quant Imaging Med Surg. (2021) 11:4847–58. doi: 10.21037/qims-21-168
Keywords: ischemic stroke, machine learning, deep learning, computed tomography, magnetic resonance imaging, meta-analysis
Citation: Wang X, Fan Y, Zhang N, Li J, Duan Y and Yang B (2022) Performance of Machine Learning for Tissue Outcome Prediction in Acute Ischemic Stroke: A Systematic Review and Meta-Analysis. Front. Neurol. 13:910259. doi: 10.3389/fneur.2022.910259
Received: 01 April 2022; Accepted: 20 June 2022;
Published: 08 July 2022.
Edited by:
Olivier Detante, Centre Hospitalier Universitaire de Grenoble, FranceReviewed by:
Warda Syeda, University of Melbourne, AustraliaBatyrkhan Omarov, Al-Farabi Kazakh National University, Kazakhstan
Samiappan Dhanalakshmi, SRM Institute of Science and Technology, India
Copyright © 2022 Wang, Fan, Zhang, Li, Duan and Yang. This is an open-access article distributed under the terms of the Creative Commons Attribution License (CC BY). The use, distribution or reproduction in other forums is permitted, provided the original author(s) and the copyright owner(s) are credited and that the original publication in this journal is cited, in accordance with accepted academic practice. No use, distribution or reproduction is permitted which does not comply with these terms.
*Correspondence: Benqiang Yang, YnF5YW5nODg4JiN4MDAwNDA7c2luYS5jb20=
†These authors have contributed equally to this work and share first authorship