- 1Department of Radiology, Guizhou Provincial People's Hospital, Guiyang, China
- 2Department of Oncology, Xichang People's Hospital, Liangshan High-Tech Tumor Hospital, Xichang, China
- 3Department of Gynaecology and Obstetrics, Zhejiang Xinda Hospital, Huzhou, China
Bioinformatics tools are used to create a clinical prediction model for cervical cancer metastasis and to investigate the neurovascular-related genes that are involved in brain metastasis of cervical cancer. One hundred eighteen patients with cervical cancer were divided into two groups based on the presence or absence of metastases, and the clinical data and imaging findings of the two groups were compared retrospectively. The nomogram-based model was successfully constructed by taking into account four clinical characteristics (age, stage, N, and T) as well as one imaging characteristic (original_glszm_GrayLevelVariance Rad-score). In patients with cervical cancer, headaches and vomiting were more often reported in the brain metastasis group than in the other metastasis groups. According to the TCGA data, mRNA differential gene expression analysis of patients with cervical cancer revealed an increase in the expression of neurovascular-related gene Adrenoceptor Beta 1 (ADRB1) in the brain metastasis group. An analysis of the correlation between imaging features and ADRB1 expression revealed that ADRB1 expression was significantly higher in the low Rad-score group compared with the high Rad-score group (P = 0.025). Therefore, ADRB1 expression in cervical cancer was correlated with imaging features and was associated as a risk factor for cerebral neurovascular metastases. This study developed a nomogram prediction model for cervical cancer metastasis using age, stage, N, T and original_glszm_GrayLevelVariance. As a risk factor associated with the development of cerebral neurovascular metastases of cervical cancer, ADRB1 expression was significantly higher in brain metastases from cervical cancer.
Introduction
The fourth most prevalent cancer among women worldwide is cervical cancer, a common gynecologic malignancy (1). Approximately 570,000 new cases of cervical cancer were reported in 2018, representing 3.15% of all malignant tumor cases. Furthermore, approximately 310,000 cervical cancer-related deaths were reported in 2018, representing 3.26% of all malignant tumor deaths (1, 2). Cancer metastasis is a major cause of poor prognosis in cervical cancer (3–6). The five-year survival rate for cervical cancer patients with metastasis is only 30–60%, which is significantly lower than that for those without metastasis (7, 8). Cervical cancer metastasis involves complex molecular mechanisms (9). The lack of effective measures to prevent or treat cervical cancer metastasis is attributable to the fact that the mechanism of cervical cancer metastasis remains unclear.
Cervical cancer metastasis occurs mainly via the lymphatic route (10). The most common sites of distant metastases are the lungs, bones, and liver, in order of priority (11). It is rare for cervical cancer to spread to the central nervous system, and very little research has been conducted on this subject (12, 13). When diagnosed with brain metastases, cervical cancer patients typically have a poor prognosis; the median survival period from the time of diagnosis until death is 2–3 months (13). As of now, there is no reliable way to predict early whether cervical cancer will spread to the neurovascular system.
Known as an emerging method of image analysis, radiomics can extract quantitative features from medical images that describe biological information, such as tumor characteristics and heterogeneity, which can serve to guide clinical planning (14). In patients with esophageal cancer, radiomics also proved to be effective for predicting T and N stages as well as lymph node metastasis (15–17). Based on the results of the radiomics study, the model can improve the diagnostic accuracy of differentiating benign and malignant cartilage tumors, as well as benign and low-grade malignant chondrosarcomas (18). An analysis conducted by Zhao et al. of diffusion-weighted images of osteosarcoma was retrospectively screened for four clinical features and eight imaging features, and a combined feature model was constructed for both characteristics, displaying good performance (19). Thus, it is possible to assess a tumor and predict its progression by combining imaging and clinical data. Moreover, imaging analysis is useful in determining the malignant and benign classification and staging of ovarian tumors, monitoring treatment outcomes in patients with metastatic ovarian tumors earlier in the course of treatment, and providing high-precision survival assessment for patients with ovarian tumors (20, 21). Consequently, radiomics is an important tool for predicting tumorigenesis, metastasis, and prognosis.
Radiomics has made significant progress in recent years when it comes to detecting and localizing brain metastases, making a differential diagnosis with other primary brain tumors, and determining the prognosis of the patient. An algorithm based on multi-sequence magnetic resonance imaging (MRI) for example, is capable of automatically detecting and segmenting brain metastases with great precision (22). The best results of glioblastoma multiforme and metastasis differentiation can be obtained by applying an Support Vector Machine (SVM) classifier based on enhanced T1-weighted images (T1W1) radiomics (23). Furthermore, the enhanced T1W1 imaging feature – “zone percentage” is an independent prognostic factor for local tumor control in non-small cell lung cancer brain metastases (24). Researchers from Shen et al. performed a retrospective study using computed tomography (CT) images of patients with bones metastases from lung adenocarcinoma who had genetically confirmed epithelial growth factor receptor (EGFR) mutation status. Three features were associated with EGFR mutation status (25). Yu et al. demonstrated that high-throughput MRI imaging features extracted from conventional T2 fluid attenuation inversion recovery (T2-FLAIR) images were highly correlated with isocitrate dehydrogenase genotype 1 (IDH1) (26). Furthermore, the conventional sequence-based fusion imaging model constructed by Jiang et al. accurately predicted the methylation status of O6-methylguanine-DNA methyltransferase (MGMT) in low-grade gliomas (27). Hence, imaging features have a potential superior predictive efficacy in predicting tumor genotypes (28).
Several studies have reported that neurovascular factors are involved in the development and metastasis of several malignancies (29, 30). The adrenergic receptor gene, a neurovascular-related factor, encodes the adrenergic receptor.
β-adrenergic receptor blockers have the ability to reduce the risk of head and neck cancer, as well as esophageal, gastric, colorectal, and prostate cancer (31, 32). Catecholamines promote ADRB2-dependent pancreatic ductal adenocarcinoma and neurotrophic factor secretion, which is critical to tumor development (33). The induction of angiogenesis by brain endothelial cells was shown to be crucial for the early survival of brain metastatic cells (34). Further, patients with lung cancer with a positive VEGFC expression were significantly more likely to develop brain metastases than those with a negative VEGFC expression (35). Accordingly, neurovascular-related genes are involved in brain metastasis in cervical cancer, and it may be possible to predict neurovascular-related genes that are associated with brain metastasis by imaging features.
In recent years, bioinformatics has gained significant attention in several fields for its role in exploring disease mechanisms (36–41). The objective of this study was to identify early risk factors and potential targets from an imaging and genomics perspective for patients with brain metastases from cervical cancer in order to improve prognoses. By combining radiomics data and clinical information, a prediction model reflecting the risk of cervical cancer metastasis was constructed in this study that screened neurovascular genes with brain metastases related to cervical cancer.
Methods
Data Collection
Patients treated at the Guizhou Provincial People's Hospital between January 2010 and January 2021 for CT examinations and a biopsy or surgical pathology that confirmed cervical cancer were included in the study. Inclusion criteria were as follows: I. an accurate pathological diagnosis; II. archived CT examination data; III. comprehensive medical and follow-up records. The following exclusion criteria were applied: I. incomplete clinical information; II. concurrent presence of primary malignant tumors at other sites; III. inadequate image quality. A total of 118 patients with cervical cancer participated in the study. The study was approved by the Ethics Committee of Guizhou Provincial People's Hospital.
Acquisition and Differential Testing of Neurovascular-Related Gene Expression Data
From the TCGA database, clinical information and transcript information were downloaded for two patients with brain metastases and 15 patients with extracerebral metastases from cervical cancer. The RNA transcription data were log-transformed and de-batched. In total, 469 genes related to neural or vascular function were identified through a literature review using PubMed, Web of Science, Google Scholar, and GeneCards databases. These genes regulate the interaction between nerves and blood vessels. The R package “limma” (version 3.50) was used to conduct gene expression analyses of groups with brain metastases and those with extracerebral metastases. Genes with false discovery rate < 0.05 and |log2 FoldChange| >1 were filtered for subsequent analysis.
Image Processing and Radiomics Feature Extraction
GE 16-row spiral CT scanners were used to acquire the imaging data of all patients. All patients were injected with 500 ml of pantethine (Xi'an Hanfeng Pharmaceutical Co., Ltd.), using a 5 mm needle, 30 min before scanning. CT images of patients were read and processed in Digital Imaging and Communications in Medicine (DICOM) format. ITK-SNAP (version 3.8.0; https://www.itksnap.org) was used for image segmentation, whereas the region of interest (ROI) was manually outlined, layer by layer, along the tumor edge. Finally, the segmented images were subjected to radiomics feature extraction using the Pyradiomics (version 3.0.1) package. Spearman's correlation analysis was used to assess the correlation between the radiomics features. For radiomics features with correlation coefficients > 0.85, only one was retained, thus minimizing redundant features.
Logistic Regression Analyses
Clinical and imaging characteristics were subjected to univariate logistics regression analysis, and characteristic variables with P < 0.05 were retained. Based on previous studies, we constructed a nomogram prediction model (40, 42–44). The retained imaging and clinical characteristics were included in the multivariate logistics regression analysis together, and characteristics with P < 0.1 were retained. The odds ratio (OR) and 95% confidence interval (95% CI) for each variable was determined separately. The receiver operating characteristic curve (ROC) was used to assess the sensitivity and specificity of continuous variables for predicting tumor metastasis.
Nomogram Construction and Clinical Application Validation
A multivariate logistics model was developed using the retained clinical and imaging characteristics. The entire dataset was divided into training and test sets in the ratio of 7:3, and the predictive performance of the model was quantitatively evaluated using the area under the curve (AUC) of the ROC. Decision curve analysis (DCA) calculated the net yield at different threshold probabilities to assess the utility and safety of the clinical prediction model. To facilitate clinical use, “RMS” (version 6.2) was used to visualize this combined multivariate model by constructing a nomogram. Predictors in the nomogram included select clinical and radiomics features. Calibration curves were used to assess the concurrence between predicted probabilities and actual outcomes.
Gene Set Enrichment Analysis
GSEA was performed to identify target neurovascular-related gene pathways using the RNA transcript dataset of patients with cervical cancer from TCGA database (45). The R package cluster profile (version 4.0) was used for GSEA analysis (46). The reference gene set “c2.cp.v7.2.symbols.GMT [Curated]” (MSigDB Collections, https://www.gsea-msigdb.org/gsea/msigdb/) was used for functional pathway annotation. The significant difference was adjusted to P < 0.05 as the cut-off criterion.
Quantitative Reverse Transcription Polymerase Chain Reaction
Patient tumor tissue samples were analyzed using qRT-PCR to explore the relationship between imaging features and gene expression. TRIzol reagent (Invitrogen) kits were used to extract total RNAs from the samples. According to the manufacturer's instructions, stem-loop antisense primer mix and AMV transcriptase (TaKaRa, China) kits were used to reverse transcribe these total RNAs into cDNAs. Finally, qRT-PCR was performed in the ABI 7500 real-time PCR system (Applied Biosystems, CA, USA) using an SYBR QPCR kit (Toyobo, Osaka, Japan). The primer sequences used are as follows: for ADRB1, forward: 5′-TCTACGTGCCCCTGTGCAT-3′ and reverse: 5′-TCGATCTTCTTCACCTGCTTCTG-3′; for GAPDH, forward: 5′-AAGAAGGTGGTGAAGCAGGC-3′ and reverse: 5′-TCCACCACCCAGTTGCTGTA-3′; for GAPDH, forward: 5′-AAGAAGGTGGTGAAGCAGGC-3′ and reverse: 5′-TCCACCACCCAGTTGCTGTA-3′ (47). GAPDH was used as a reference to normalize the same gene expressions between samples. The 2−ΔΔCt method was used to calculate the relative expression of the target gene. Gene expression was further normalized between samples before subsequent analysis.
Statistical Analysis
Demographic variables were divided into categorical and continuous variables, with Pearson's chi-square or Fisher's exact tests being used for categorical variables and Wilcoxon rank sum tests for continuous variables. Statistical analysis was performed using R software (version 4.0.2), and a two-tailed P < 0.05 was considered a statistically significant difference.
Results
Demographic Characteristics and Imaging Features of the Patients
The flow chart of patient inclusion and data sources in this study are shown in Figure 1. The clinical characteristics and imaging variables of 118 patients with cervical cancer are presented in Table 1. Older patients showed a higher incidence of tumor metastasis than younger patients (p = 0.022). Additionally, a statistical difference was observed in stage, metastasis, T, N, surgery and radiation between patients with and without metastasis (P < 0.05). Patients with metastasis had a higher stage, larger T and N grades and lower surgery and radiation therapy rates than those without metastasis. Analyses of univariate regression and correlation were performed on the features extracted from the enhanced CT images, identifying three most critical variables, namely original_glszm_GrayLevelVariance, original_gldm_SmallDependenceHighGrayLevelEmphasis and original_glszm_SmallAreaLowGrayLevelEmphasis. The correlation heatmap of these three features is shown in Figure 2A. Based on the above analysis, we identified the main imaging features of metastases from cervical cancer.
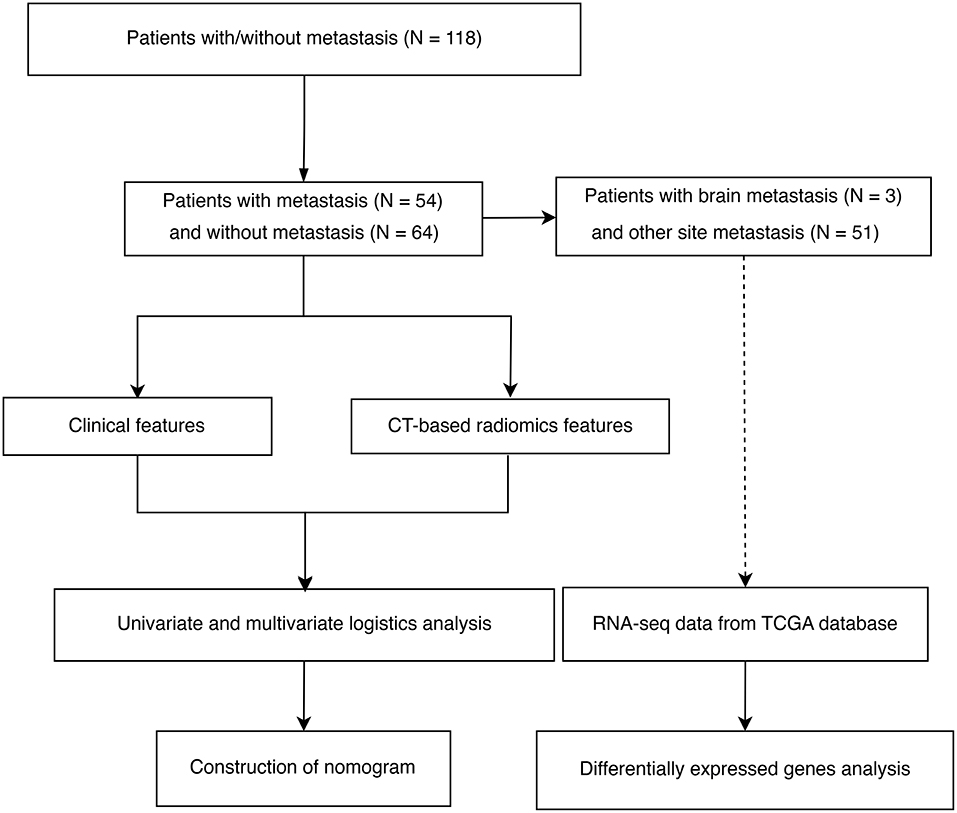
Figure 1. Flowchart showing the inclusion criteria and study process of selecting patients with cervical cancer.
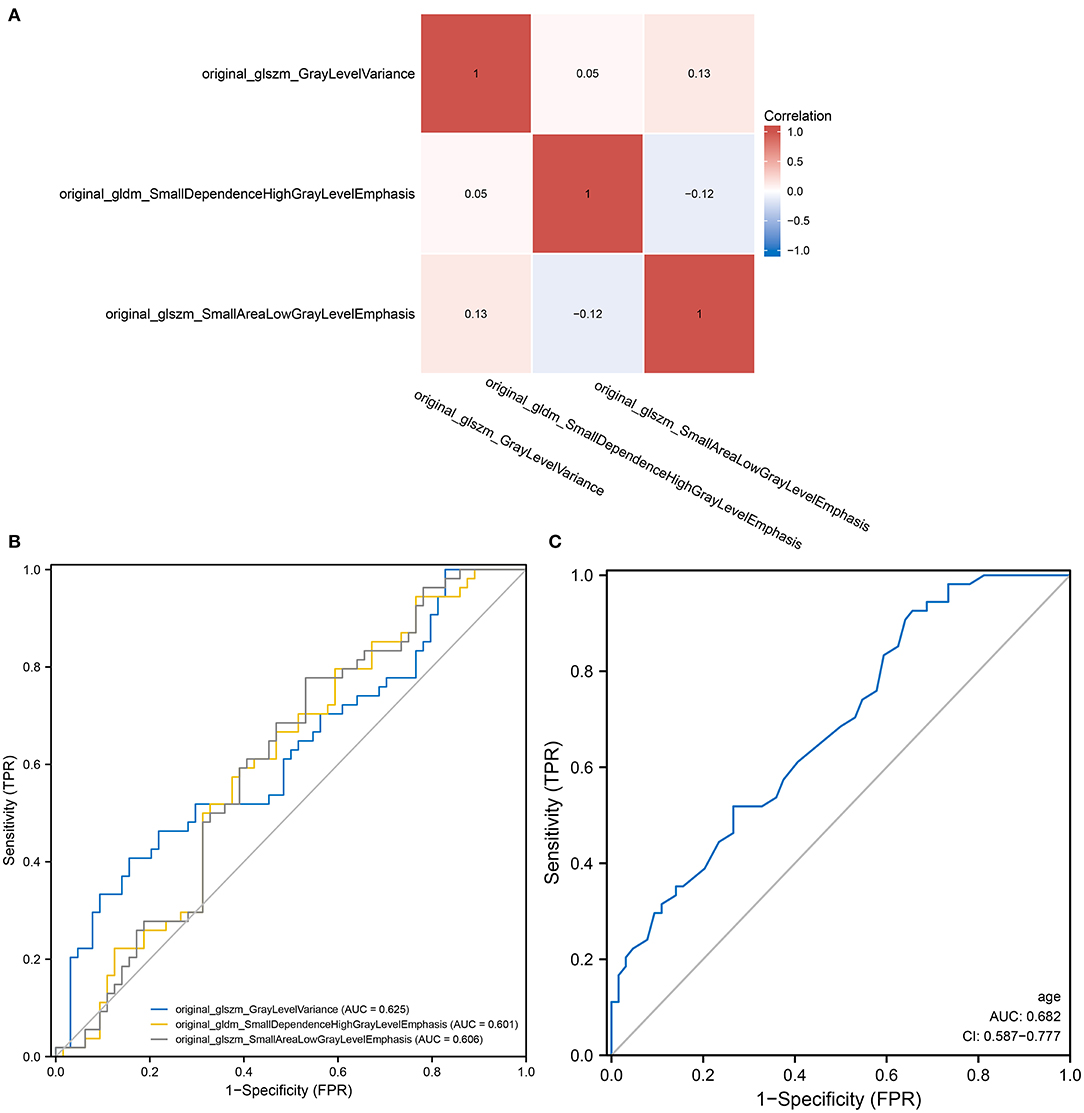
Figure 2. Radiomics and the predictive power of age in predicting tumor metastasis. (A) A heat map demonstrating low correlation between the three screened radiomics features; (B) A heat map demonstrating low correlation between the three screened radiomics features; (C) A ROC curve showing the ability of univariate logistic regression models of age to predict tumor metastasis.
Variable Screening for Univariate and Multivariate Logistic Regression Analysis
Logistic models are commonly used to screen for risk factors for clinical outcomes. The predictors associated with tumor metastasis based on univariate and multivariate logistic regression models are shown in Table 2. Based on the filtering conditions of univariate analysis, age, stage, N, T, surgery, radiation, original_glszm_GrayLevelVariance, original_gldm_SmallDependenceHighGrayLevelEmphasis and original_glszm_SmallAreaLowGrayLevelEmphasis were able to predict tumor metastasis in patients with cervical cancer (P < 0.05). The AUCs for these three radiomics features were 0.625, 0.601, and 0.606 according to the univariate analysis, which means these features can serve as good predictors of cervical cancer (Figure 2B). Further multivariate logistic regression analysis showed that age, stage and N were independent predictors of tumor metastasis (P < 0.05). According to Figure 2C, the AUC on the prediction model constructed based on age was 0.682 (0.587–0.777). Based on predefined filtering conditions, age, stage, N, surgery, and original_glszm_GrayLevelVariance have been incorporated into a multivariate logistic regression model in order to construct a clinical prediction model. In the training, validation, and overall groups, the AUCs of this predictive model were, respectively 0.922, 0.833, and 0.910 (Figure 3A). Accordingly, the predictive efficacy and accuracy of the model was enhanced by the inclusion of clinical and imaging factors together. Based on DCA of the training, validation and overall groups shown in Figure 3B, the model appears to be user-friendly and has a wide range of clinical applicability. Figure 3C depicts the weights assigned to each variable in the model, with the imaging features having the greatest weight (Table 3).
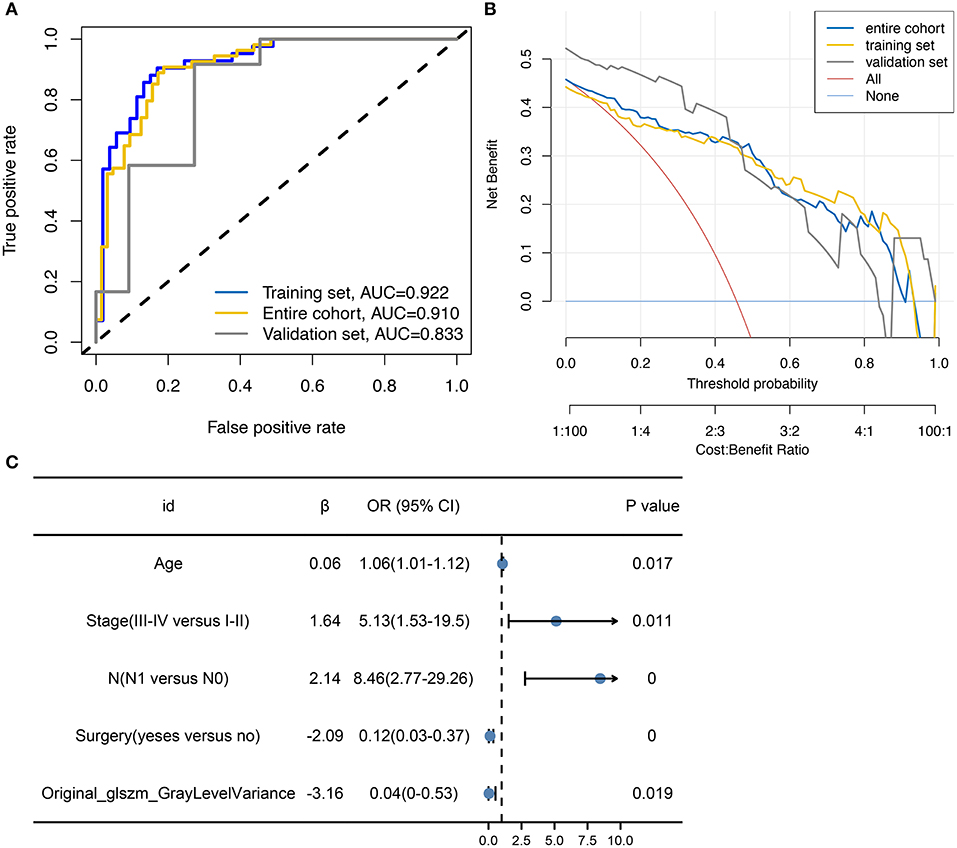
Figure 3. Construction of multifactor models with variable weights. (A) ROC curve showing the predictive performance of models based on clinical data and imaging features. (B) DCA showing the wide range of clinical utility and safety associated with the built prediction model. (C) Forest plot displaying the relative weights and values of the variables included in the model.
Nomogram Construction for Predicting Tumor Metastasis
Based on the regression coefficients of each variable in the constructed multivariate logistic regression model, the nomogram of the clinical prediction model was plotted (Figure 4A). The nomogram consists of a series of selected variables and a score corresponding to each featured variable. By calculating the total score of all features in the nomogram, the likelihood of tumor metastasis can be determined. It is evident in Figure 4B that there is a high degree of consistency between the predicted and true values for patients in the multivariate prediction model. Principal component analysis (PCA) reveals that the variables incorporated in the model show significant differences between patients with cervical cancer with and without metastasis (Figure 4C). These results suggest that this cervical cancer metastatic prediction model performs well.
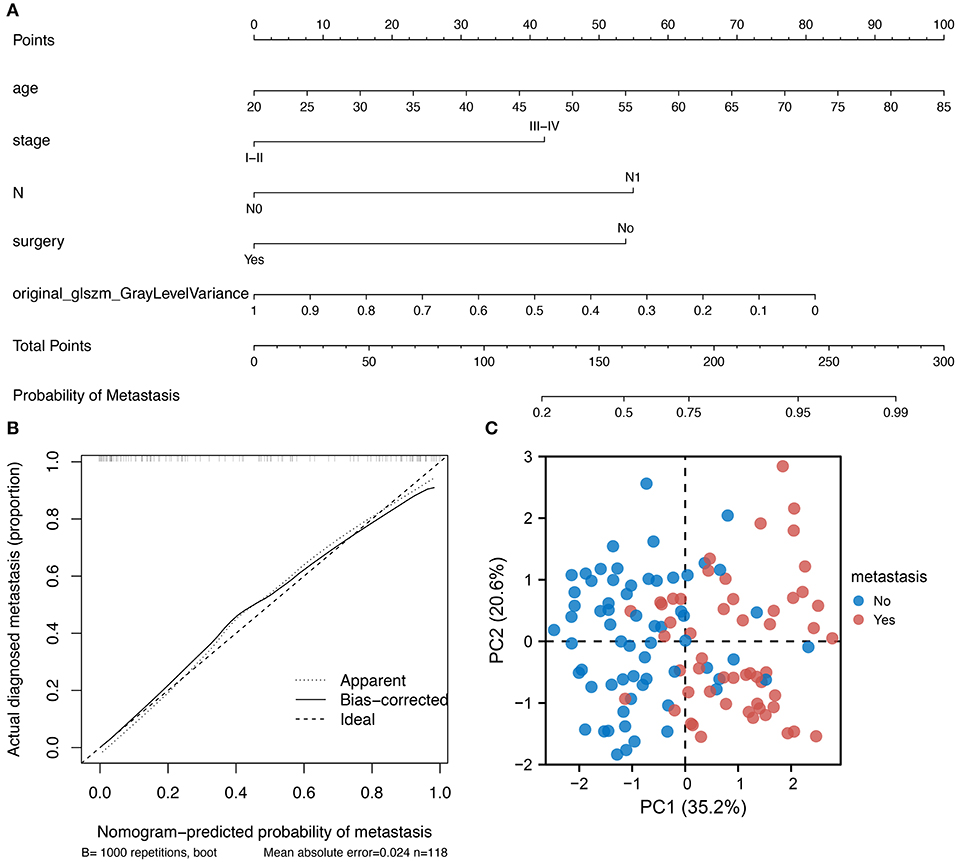
Figure 4. Nomogram development and clinical validation. (A) A nomogram was constructed using the multi-factor logistic regression model; (B) Calibration plot showing a good fit between the true and predicted values; (C) PCA showing the variables incorporated into the model could distinguish between cervical cancer patients with metastasis.
Comparison of the Brain and Non-brain Metastasis Groups
An examination of clinical differences between patients with brain metastases and those with non-brain metastases was performed to determine the relationship between metastases, neurological and vascular effects. Among the brain metastases group, the incidence of headache and vomiting was 100 and 66.7%, respectively (Figure 5A), whereas among the metastases group, the incidence of headache and vomiting was 7.8 and 17.6%, respectively (Figure 5B), with a statistically significant difference between the two groups (p < 0.05). In addition to the increased intracranial pressure due to intracranial occupancy, headache can also be associated with neurological and vascular disorders. Additionally, associated neuropeptide or vasoactive factor release can also result in vomiting in addition to gastrointestinal dysfunction. Consequently, the 469 genes related to neurological and vascular conditions were analyzed.
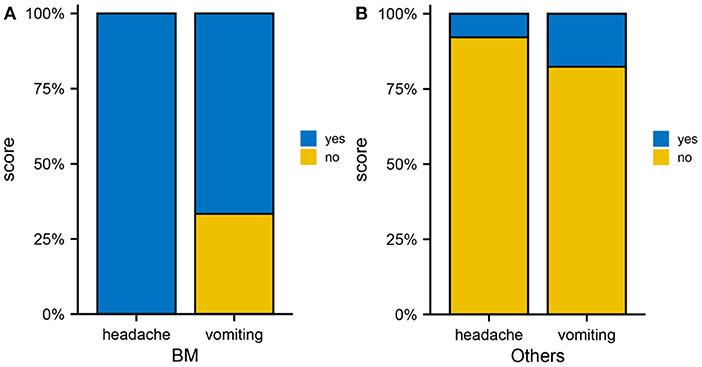
Figure 5. Brain metastases from cervical cancer. (A) Occurrence rate of headache as well as vomiting in the brain metastasis group. (B) Occurrence rate of headache and vomiting in the non-brain metastasis group.
Variance Analysis in the TCGA Database
RNA transcription data were obtained from the TCGA database for three patients with cervical cancer who had brain metastases and 14 patients with cervical cancer who did not have brain metastases. Differential expression analysis was performed on the 469 genes related to neurological function, which were obtained from the literature review and GeneCards database. Five genes (CHGB, CACNA1B, ADRB1, PLCG1 and TSPO) were found to be statistically significant between the two groups (Figure 6A). After ranking the differential genes by significance and investigating the function of each gene, ADRB1 was identified as a gene that could be involved in nerves and blood vessels. ADRB1 expression was significantly different in brain metastasis compared to non-brain metastasis groups (Figure 6B). In the tumor brain metastasis group, ADRB1 expression was significantly higher than at other metastasis-site group (p = 0.003). In order to further identify the role of ADRB1 in cervical cancer brain metastasis, the GSEA analysis of RNA transcription data from patients with cervical cancer revealed that the T cell receptor complex pathway was upregulated, while the iron-sulfur cluster assembly pathway was downregulated (Figure 6C). This suggests the potential role of ADRB1 in immune regulation and altered mitochondrial respiratory function in response to hypoxia (48).
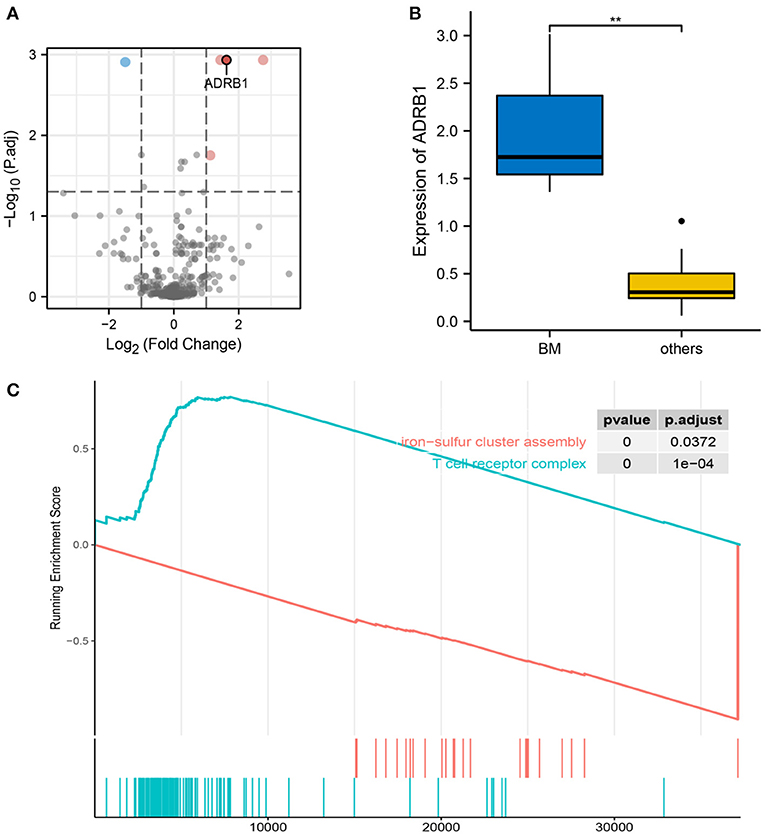
Figure 6. Characteristics of ADRB1. (A) Volcano plot showing differentially expressed genes between the brain metastasis and the extracerebral metastasis groups, and ADRB1 was upregulated in the brain metastasis group. (B) Differences in ADRB1 expression between the brain metastasis and non-brain metastasis groups. (C) GSEA showing the upregulation of the T cell receptor complex pathway in the ADRB1-enhanced group and the downregulation of the iron-sulfur cluster assembly pathway.
Radiomics Features Can Predict ADRB1 Expression
A correlation analysis was conducted between original_glszm_GrayLevelVariance and ADRB1 expression in 60 patients to further examine the predictability of the imaging feature in the constructed model. The correlation analysis revealed a negative correlation between the radiomics feature original_glszm_GrayLevelVariance and ADRB1 expression (Figure 7A, Spearman r = −0.46, p < 0.001). Patients were further divided into high Rad-score and low Rad-score groups according to the value variation of the radiomics feature original_glszm_GrayLevelVariance. The difference in ADRB1 expression between the high- and low-Rad-score groups is statistically significant (Figure 7B). Expression of ADRB1 was higher in the low-Rad-score group than the high-Rad-score group (p = 0.025). In light of this, the radiomics feature original_glszm_GrayLevelVariance in the model is likely to be able to predict ADRB1 expression and, thereby, brain metastasis in cervical cancer. Hence, ADRB1 expression is associated with radiomics features and is considered a risk factor for cerebral neurovascular metastasis of cervical cancer.
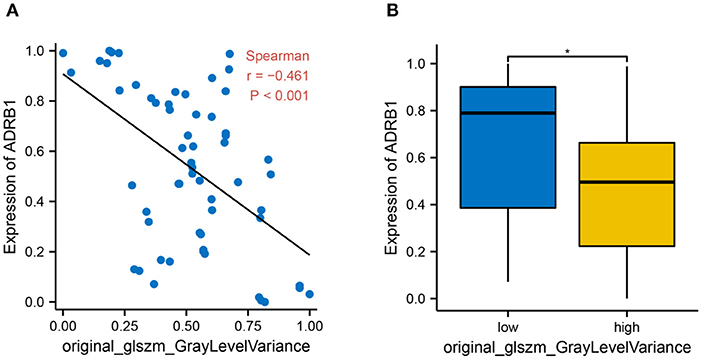
Figure 7. The relationship between histological features and ADRB1 expression. (A) A correlation analysis found a negative relationship between the imaging feature original_glszm_GrayLevelVariance and ARDB1 expression (Spearman r = −0.46, P < 0.001). (B) Patients were divided into high Rad-score and low Rad-score groups based on the value of the histological feature original_glszm_GrayLevelVariance; * p < 0.05.
Discussion
The clinical and radiomic features of 118 patients with cervical cancer (with or without tumor metastasis) were retrospectively analyzed. Univariate and multivariate logistic regression analyses screened four clinical features (age, stage, N and T) and one radiomics feature (original_glszm_GrayLevelVariance) associated with cervical cancer metastasis. These features were used to construct clinical prediction models. ADRB1 was found to be related to radiomic features and was considered as a risk factor associated with the risk of cerebral neurovascular metastasis in cervical cancer.
Radiomics is an emerging field that involves the transformation of various types of images into high-dimensional image feature data, providing a more comprehensive description of tumor tissue heterogeneity (49, 50). In a study, the prediction of cervical cancer metastasis was modeled using multiparametric MRI imaging and seven clinical and pathological characteristics, demonstrating that multisequence MRI features serve as non-invasive biomarkers for the preoperative assessment of cervical cancer lymph node status. Furthermore, these characteristics can have an impact on treatment decisions for patients with cervical cancer at an early stage (51). Wu et al. constructed a radiomics model and apparent diffusion coefficients (ADCs) of 189 patients, which was able to predict the development of lymph node metastases in cervical cancer with an AUC of 0.847 (52). Similarly to previous MR-based studies of cervical cancer, this study also observed a better predictability of cervical cancer metastasis using radiomics (53). Based on the original_glszm_GrayLevelVariance feature of enhanced CT images in conjunction with clinical features, the current study developed a clinical prediction model for cervical cancer metastasis. It was demonstrated that the prediction model had good predictive efficacy, a high clinical usefulness and a wide safety range, which successfully differentiated between women with cervical cancer who had metastasized and those who did not. Recent studies have shown that radiomics can be used to investigate the association between histological features and gene mutations by mining high-throughput radiomics features from conventional CT images (54). Similarly, radiomics can also predict genes associated with cervical cancer metastasis.
ADRB1, a β1 adrenergic receptor, is a member of the G protein-coupled receptor family, serving as adrenergic receptors in myocardiocytes (55). The β-adrenergic receptor signaling pathway has also been found to be closely associated with tumor proliferation, apoptosis and microenvironment regulation (56–59). According to previous studies, ADRB family genes play a crucial role in regulating the interaction between the sympathetic drive and the immune system (60). According to previous studies, ADRB family genes play a crucial role in regulating the interaction between the sympathetic drive and the immune system. ADRB1 is involved in the regulation of tumor development in the lungs, breast and prostate (61–63). Furthermore, ADRB1 genes may act synergistically with FOXA1 and ADRB2 genes to drive tumor formation and development (62). It is also closely associated with metastasis and recurrence of oral squamous cell carcinoma (64). Similarly, this study identified ADRB1 as a potential oncogene involved in the neurovascular metastasis of cervical cancer. Consistent with previous studies, GSEA revealed that the T cell receptor complex pathway was upregulated, whereas the iron-sulfur cluster assembly pathway was downregulated in the ADRB1 group, indicating the role of ADRB1 in immune regulation and altered mitochondrial respiratory function in response to hypoxia. Wang et al. observed that the high expression of ADRB1 could mediate and maintain a normal immune response by promoting the activity of B cells and dendritic cells (61). Immune infiltration contributes significantly to tumor progression (65). Thus, ADRB1 serves as an immunomodulator in the process of cerebral neurovascular metastasis in cervical cancer.
Lymph node status in early-stage cervical cancer was found to be associated with the age of the patient (66). Ki et al. report the mean age of diagnosis in patients with cervical cancer metastasis as 55 years (67). Advanced age is a remarkable factor affecting the prognosis of patients with stage IV cervical cancer, with patients ≥70 years of age having the worst prognosis (68). Similar to previous studies, the age of patients with cervical cancer in the non-metastatic group was found to be significantly lower than those with metastasis. Moreover, age turned out to be an independent risk factor for cervical cancer metastasis. Additionally, patients with cervical cancer who had tumor diameters >4 cm were significantly more likely to develop metastasis than those who had tumor diameters <4 cm (69). The incidence of cervical cancer metastasis increases with the progression of the tumor stage (70). Similarly, pelvic lymph node metastasis in patients with cervical cancer could lead to a higher rate of distant metastasis (71).
Neurovascular factors are thought to contribute to headache and vomiting. The incidence of headache and vomiting was higher in patients with cervical cancer in the brain metastasis group when compared to those with other sites of metastasis. Further bioinformatic analysis revealed that the expression of neurovascular-related gene ADRB1 was elevated in the tumor brain metastasis group. However, the current study has certain limitations: (1) The clinical aspect of this study was retrospective, with possible selection bias and a small sample size. Therefore, further multi-center studies should expand the sample size; (2) The risk factors for brain metastasis in cervical cancer screened in this study were based on bioinformatic analyses, which require further clinical validation; (3) RNA transcription data were downloaded from the TCGA database for two patients with brain metastases and 15 patients with extracerebral metastases from cervical cancer, which may be subject to selection bias due to the limited sample size; (4) Additionally, further experiments to validate the function of ADRB1 in cervical cancer brain metastasis are required.
Conclusion
Based on four clinical features and one radiomic feature, a nomogram-based cervical cancer metastasis prediction model has been developed. A radiomics feature is considered essential for predicting tumor gene expression. ADRB1 gene expression was significantly higher in cervical cancer brain metastasis than in other metastasis sites. Therefore, features of radiomics that are related to ADRB1 may be associated with the risk of cerebral neurovascular metastases in cervical cancer.
Data Availability Statement
The datasets presented in this study can be found in online repositories. The names of the repository/repositories and accession number(s) can be found in the article/supplementary material.
Ethics Statement
The studies involving human participants were reviewed and approved by Institutional Ethics Review Board of the Guizhou Provincial People' s Hospital. The patients/participants provided their written informed consent to participate in this study.
Author Contributions
XZ, SX, and JW reviewed the manuscript, analysis, and analyzed the results. XZ drafted and performed the data curation. All authors read and approved the final manuscript.
Conflict of Interest
The authors declare that the research was conducted in the absence of any commercial or financial relationships that could be construed as a potential conflict of interest.
Publisher's Note
All claims expressed in this article are solely those of the authors and do not necessarily represent those of their affiliated organizations, or those of the publisher, the editors and the reviewers. Any product that may be evaluated in this article, or claim that may be made by its manufacturer, is not guaranteed or endorsed by the publisher.
Acknowledgments
The authors wish to thank all the patients and staff who participated in this study. Thanks to Bullet Edits Limited for language editing and proofreading of the manuscript.
References
1. Vu M, Yu J, Awolude OA, Chuang L. Cervical cancer worldwide. Curr Probl Cancer. (2018) 42:457–65. doi: 10.1016/j.currproblcancer.2018.06.003
2. Arbyn M, Weiderpass E, Bruni L, de Sanjosé S, Saraiya M, Ferlay J, et al. Estimates of incidence and mortality of cervical cancer in 2018: a worldwide analysis. Lancet Global Health. (2020) 8:e191–203. doi: 10.1016/S2214-109X(19)30482-6
3. Chen S, Gao C, Wu Y, Huang Z. Identification of Prognostic miRNA signature and lymph node metastasis-related key genes in cervical cancer. Front Pharmacol. (2020) 11:544. doi: 10.3389/fphar.2020.00544
4. Zhong Y, Lu Q, Qiu W, Luo Y. LINC00636 promotes lymph node metastasis and cervical cancer through targeting NM23. Biosci Rep. (2020) 40:BSR20200367. doi: 10.1042/BSR20200367
5. Gong Y, Wan J-H, Zou W, Lian G-Y, Qin J-L, Wang Q-M. MiR-29a inhibits invasion and metastasis of cervical cancer via modulating methylation of tumor suppressor SOCS1. Future Oncol. (2019) 15:1729–44. doi: 10.2217/fon-2018-0497
6. Shi X, Wang J, Dai S, Qin L, Zhou J, Chen Y. Apolipoprotein C1 (APOC1): a novel diagnostic and prognostic biomarker for cervical cancer. OTT. Volume. (2020) 13:12881–91. doi: 10.2147/OTT.S280690
7. Wang P, Zhai G, Bai Y. Values of miR-34a and miR-218 expression in the diagnosis of cervical cancer and the prediction of prognosis. Oncol Lett. (2018) 15:3580–5. doi: 10.3892/ol.2018.7791
8. Hisamatsu T, Mabuchi S, Yoshino K, Fujita M, Enomoto T, Hamasaki T. Kimura T. Prediction of progression-free survival and response to paclitaxel plus carboplatin in patients with recurrent or advanced cervical cancer. Int J Gynecol Cancer. (2012) 22:623–9. doi: 10.1097/IGC.0b013e3182473277
9. Zhou J-Y, Zheng S-R, Liu J, Shi R, Yu H-L, Wei M. MiR-519d facilitates the progression and metastasis of cervical cancer through direct targeting Smad7. Cancer Cell Int. (2016) 16:21. doi: 10.1186/s12935-016-0298-1
10. Peng J, Liu X, Li C, Gao M, Wang H. Sema4C modulates the migration of primary tumor-associated lymphatic endothelial cells via an ERK-mediated pathway. Exp Ther Med. (2021) 22:1102. doi: 10.3892/etm.2021.10535
11. Qian C, Liu H, Feng Y, Meng S, Wang D, Nie M, et al. Clinical characteristics and risk of second primary lung cancer after cervical cancer: a population-based study. PLoS ONE. (2020) 15:e0231807. doi: 10.1371/journal.pone.0231807
12. Piura E, Piura B. Brain metastases from cervical carcinoma: overview of pertinent literature. Eur J Gynaecol Oncol. (2012) 33:567–73. doi: 10.5402/2012/581749
13. Cormio G, Pellegrino A, Landoni F, Regallo M, Zanetta G, Colombo A, et al. Brain metastases from cervical carcinoma. Tumori. (1996) 82:394–6. doi: 10.1177/030089169608200420
14. Lambin P, Leijenaar RTH, Deist TM, Peerlings J, de Jong EEC, van Timmeren J, et al. Radiomics: the bridge between medical imaging and personalized medicine. Nat Rev Clin Oncol. (2017) 14:749–62. doi: 10.1038/nrclinonc.2017.141
15. Liu S, Zheng H, Pan X, Chen L, Shi M, Guan Y, et al. Texture analysis of CT imaging for assessment of esophageal squamous cancer aggressiveness. J Thorac Dis. (2017) 9:4724–32. doi: 10.21037/jtd.2017.06.46
16. Shen C, Liu Z, Wang Z, Guo J, Zhang H, Wang Y, et al. Building CT radiomics based nomogram for preoperative esophageal cancer patients lymph node metastasis prediction. Transl Oncol. (2018) 11:815–24. doi: 10.1016/j.tranon.2018.04.005
17. Qu J, Shen C, Qin J, Wang Z, Liu Z, Guo J, et al. The MR radiomic signature can predict preoperative lymph node metastasis in patients with esophageal cancer. Eur Radiol. (2019) 29:906–14. doi: 10.1007/s00330-018-5583-z
18. Fritz B, Müller DA, Sutter R, Wurnig MC, Wagner MW, Pfirrmann CWA, et al. Magnetic resonance imaging–based grading of cartilaginous bone tumors: added value of quantitative texture analysis. Invest Radiol. (2018) 53:663–72. doi: 10.1097/RLI.0000000000000486
19. Zhao S, Su Y, Duan J, Qiu Q, Ge X, Wang A, et al. Radiomics signature extracted from diffusion-weighted magnetic resonance imaging predicts outcomes in osteosarcoma. J Bone Oncol. (2019) 19:100263. doi: 10.1016/j.jbo.2019.100263
20. Zhang H, Mao Y, Chen X, Wu G, Liu X, Zhang P, et al. Magnetic resonance imaging radiomics in categorizing ovarian masses and predicting clinical outcome: a preliminary study. Eur Radiol. (2019) 29:3358–71. doi: 10.1007/s00330-019-06124-9
21. Kyriazi S, Collins DJ, Messiou C, Pennert K, Davidson RL, Giles SL, et al. Metastatic ovarian and primary peritoneal cancer: assessing chemotherapy response with diffusion-weighted MR imaging—value of histogram analysis of apparent diffusion coefficients. Radiology. (2011) 261:182–92. doi: 10.1148/radiol.11110577
22. Grøvik E, Yi D, Iv M, Tong E, Rubin D, Zaharchuk G. Deep learning enables automatic detection and segmentation of brain metastases on multisequence MRI. J Magn Reson Imaging. (2020) 51:175–82. doi: 10.1002/jmri.26766
23. Artzi M, Bressler I, Ben Bashat D. Differentiation between glioblastoma, brain metastasis and subtypes using radiomics analysis: radiomics classification of brain tumors. J Magn Reson Imaging. (2019) 50:519–28. doi: 10.1002/jmri.26643
24. Huang C-Y, Lee C-C, Yang H-C, Lin C-J, Wu H-M, Chung W-Y, et al. Radiomics as prognostic factor in brain metastases treated with Gamma Knife radiosurgery. J Neurooncol. (2020) 146:439–49. doi: 10.1007/s11060-019-03343-4
25. Shen T, Liu L, Li W, Fu P, Xu K, Jiang Y, et al. imaging-based histogram features for prediction of EGFR mutation status of bone metastases in patients with primary lung adenocarcinoma. Cancer Imaging. (2019) 19:34. doi: 10.1186/s40644-019-0221-9
26. Yu J, Shi Z, Lian Y, Li Z, Liu T, Gao Y, et al. Noninvasive IDH1 mutation estimation based on a quantitative radiomics approach for grade II glioma. Eur Radiol. (2017) 27:3509–22. doi: 10.1007/s00330-016-4653-3
27. Jiang C, Kong Z, Liu S, Feng S, Zhang Y, Zhu R, et al. Fusion radiomics features from conventional MRI predict MGMT promoter methylation status in lower grade gliomas. Eur J Radiol. (2019) 121:108714. doi: 10.1016/j.ejrad.2019.108714
28. Aerts HJWL. The potential of radiomic-based phenotyping in precision medicine: a review. JAMA Oncol. (2016) 2:1636. doi: 10.1001/jamaoncol.2016.2631
29. Xu K, Wu C, Wang Z, Wang H, Yin F, Li W, et al. Family gene expression as prognostic biomarkers for Alzheimer's disease and primary liver cancer. Comput Math Methods Med. (2021) 2021:1–15. doi: 10.1155/2021/3422393
30. Deng C, Guo H, Yan D, Liang T, Ye X, Li Z. Pancancer analysis of neurovascular-related NRP family genes as potential prognostic biomarkers of bladder urothelial carcinoma. Biomed Res Int. (2021) 2021:5546612. doi: 10.1155/2021/5546612
31. Chang A, Yeung S, Thakkar A, Huang KM, Liu MM, Kanassatega R-S, et al. Prevention of skin carcinogenesis by the β-blocker carvedilol. Cancer Prev Res. (2015) 8:27–36. doi: 10.1158/1940-6207.CAPR-14-0193
32. Chang P-Y, Huang W-Y, Lin C-L, Huang T-C, Wu Y-Y, Chen J-H, et al. Propranolol reduces cancer risk: a population-based cohort study. Medicine. (2015) 94:e1097. doi: 10.1097/MD.0000000000001097
33. Renz BW, Takahashi R, Tanaka T, Macchini M, Hayakawa Y, Dantes Z, et al. β2 Adrenergic-neurotrophin feedforward loop promotes pancreatic cancer. Cancer Cell. (2018) 33:75–90.e7. doi: 10.1016/j.ccell.2018.10.010
34. Chen G, Liu X, Wang Z, Liu F. Vascular endothelial growth factor C: the predicator of early recurrence in patients with N2 non-small-cell lung cancer. Eur J Cardiothorac Surg. (2010) 37:546–51. doi: 10.1016/j.ejcts.2009.08.005
35. Xing F, Liu Y, Sharma S, Wu K, Chan MD, Lo H-W, et al. Activation of the c-Met pathway mobilizes an inflammatory network in the brain microenvironment to promote brain metastasis of breast cancer. Cancer Res. (2016) 76:4970–80. doi: 10.1158/0008-5472.CAN-15-3541
36. Martínez-Rodríguez F, Limones-González JE, Mendoza-Almanza B, Esparza-Ibarra EL, Gallegos-Flores PI, Ayala-Luján JL, et al. understanding cervical cancer through proteomics. Cells. (2021) 10:1854. doi: 10.3390/cells10081854
37. Sun Y, Sun X, Liu S, Liu L, Chen J. The overlap between regeneration and fibrosis in injured skeletal muscle is regulated by phosphatidylinositol 3-kinase/Akt signaling pathway - a bioinformatic analysis based on lncRNA microarray. Gene. (2018) 672:79–87. doi: 10.1016/j.gene.2018.06.001
38. Luo Z, Sun Y, Qi B, Lin J, Chen Y, Xu Y, Chen J. Human bone marrow mesenchymal stem cell-derived extracellular vesicles inhibit shoulder stiffness via let-7a/Tgfbr1 axis. Bioact Mater. (2022) 17:344–59. doi: 10.1016/j.bioactmat.2022.01.016
39. Huang J, Liang X, Cai Z. A Potential ceRNA network for neurological damage in preterm infants. Biomed Res Int. (2021) 2021:2628824. doi: 10.1155/2021/2628824
40. Shi Q, Yan X, Wang J, Zhang X. Collagen family genes associated with risk of recurrence after radiation therapy for vestibular schwannoma and pan-cancer analysis. Dis Markers. (2021) 2021:1–15. doi: 10.1155/2021/7897994
41. Tang G-Y, Yu P, Zhang C, Deng H-Y, Lu M-X, Le J-H. The neuropeptide-related HERC5/TAC1 interactions may be associated with the dysregulation of lncRNA GAS5 expression in gestational diabetes mellitus exosomes. Dis Markers. (2022) 2022:1–12. doi: 10.1155/2022/8075285
42. Balachandran VP, Gonen M, Smith JJ, DeMatteo RP. Nomograms in oncology: more than meets the eye. Lancet Oncol. (2015) 16:e173–80. doi: 10.1016/S1470-2045(14)71116-7
43. Ying C, Guo C, Wang Z, Chen Y, Sun J, Qi X, et al. Prediction modeling based on the hospital for special surgery (HSS) knee score for poor postoperative functional prognosis of elderly patients with patellar fractures. Biomed Res Int. (2021) 2021:6620504. doi: 10.1155/2021/6620504
44. Sun C, Ma S, Chen Y, Kim NH, Kailas S, Wang Y, et al. Diagnostic value, prognostic value, and immune infiltration of LOX family members in liver cancer: bioinformatic analysis. Front Oncol. (2022) 12:843880. doi: 10.3389/fonc.2022.843880
45. Subramanian A, Tamayo P, Mootha VK, Mukherjee S, Ebert BL, Gillette MA, et al. Gene set enrichment analysis: a knowledge-based approach for interpreting genome-wide expression profiles. Proc Natl Acad Sci USA. (2005) 102:15545–50. doi: 10.1073/pnas.0506580102
46. Yu G, Wang L-G, Han Y, He Q-Y. clusterProfiler: an R package for comparing biological themes among gene clusters. OMICS. (2012) 16:284–7. doi: 10.1089/omi.2011.0118
47. Ranasinghe AM. How does glucose insulin potassium improve hemodynamic performance? evidence for altered expression of beta-adrenoreceptor and calcium handling genes. Circulation. (2006) 114(1 Suppl):I239–44. doi: 10.1161/CIRCULATIONAHA.105.000760
48. Chan SY, Zhang Y-Y, Hemann C, Mahoney CE, Zweier JL, Loscalzo J. MicroRNA-210 controls mitochondrial metabolism during hypoxia by repressing the iron-sulfur cluster assembly proteins ISCU1/2. Cell Metab. (2009) 10:273–84. doi: 10.1016/j.cmet.2009.08.015
49. Mayerhoefer ME, Materka A, Langs G, Häggström I, Szczypiński P, Gibbs P, et al. Introduction to radiomics. J Nucl Med. (2020) 61:488–95. doi: 10.2967/jnumed.118.222893
50. Lee S-H, Park H, Ko ES. Radiomics in breast imaging from techniques to clinical applications: a review. Korean J Radiol. (2020) 21:779. doi: 10.3348/kjr.2019.0855
51. Kan Y, Dong D, Zhang Y, Jiang W, Zhao N, Han L, et al. Radiomic signature as a predictive factor for lymph node metastasis in early-stage cervical cancer: radiomic signature of LNM in cervical cancer. J Magn Reson Imaging. (2019) 49:304–10. doi: 10.1002/jmri.26209
52. Wu Q, Wang S, Chen X, Wang Y, Dong L, Liu Z, et al. Radiomics analysis of magnetic resonance imaging improves diagnostic performance of lymph node metastasis in patients with cervical cancer. Radiother Oncol. (2019) 138:141–8. doi: 10.1016/j.radonc.2019.04.035
53. Li Z, Li H, Wang S, Dong D, Yin F, Chen A, et al. MR-based radiomics nomogram of cervical cancer in prediction of the lymph-vascular space invasion preoperatively: preoperative prediction of LVSI. J Magn Reson Imaging. (2019) 49:1420–6. doi: 10.1002/jmri.26531
54. Gillies RJ, Kinahan PE, Hricak H. Radiomics: images are more than pictures, they are data. Radiology. (2016) 278:563–77. doi: 10.1148/radiol.2015151169
55. Kelley EF, Snyder EM, Johnson BD. Influence of Beta-1 adrenergic receptor genotype on cardiovascular response to exercise in healthy subjects. Cardiol Res. (2018) 9:343–9. doi: 10.14740/cr785
56. Wang F, Liu H, Wang F, Xu R, Wang P, Tang F, et al. Propranolol suppresses the proliferation and induces the apoptosis of liver cancer cells. Mol Med Rep. (2018) 17:5213–21. doi: 10.3892/mmr.2018.8476
57. He J-J, Zhang W-H, Liu S-L, Chen Y-F, Liao C-X, Shen Q-Q, et al. Activation of β-adrenergic receptor promotes cellular proliferation in human glioblastoma. Oncol Lett. (2017) 14:3846–52. doi: 10.3892/ol.2017.6653
58. Calvani M, Pelon F, Comito G, Taddei ML, Moretti S, Innocenti S, et al. Norepinephrine promotes tumor microenvironment reactivity through β3-adrenoreceptors during melanoma progression. Oncotarget. (2015) 6:4615–32. doi: 10.18632/oncotarget.2652
59. Wrobel LJ, Bod L, Lengagne R, Kato M, Prévost-Blondel A, Gal F-AL. Propranolol induces a favourable shift of anti-tumor immunity in a murine spontaneous model of melanoma. Oncotarget. (2016) 7:77825–37. doi: 10.18632/oncotarget.12833
60. Ahmari N, Schmidt JT, Krane GA, Malphurs W, Cunningham BE, Owen JL, et al. Loss of bone marrow adrenergic beta 1 and 2 receptors modifies transcriptional networks, reduces circulating inflammatory factors, and regulates blood pressure. Physiol Genomics. (2016) 48:526–36. doi: 10.1152/physiolgenomics.00039.2016
61. Wang J, Zhang X, Li J, Ma X, Feng F, Liu L, et al. ADRB1 was identified as a potential biomarker for breast cancer by the co-analysis of tumor mutational burden and immune infiltration. Aging. (2021) 13:351–63. doi: 10.18632/aging.104204
62. Lehrer S, Rheinstein PH. The ADRB1 (Adrenoceptor Beta 1) and ADRB2 genes significantly co-express with commonly mutated genes in prostate cancer. Discov Med. (2020) 30:163–71.
63. Li C, Chen W, Zhang M, Zhang C, Cao B, Dong B, et al. Modulatory effects of Xihuang Pill on lung cancer treatment by an integrative approach. Biomed Pharmacother. (2020) 130:110533. doi: 10.1016/j.biopha.2020.110533
64. Dong H, Liao X-X, Mai H-M, Zhou N, Wang D-Y, Peng J-B, et al. Expression of beta adrenergic receptor in oral squamous cell carcinoma and its significance to the prognosis. Int J Clin Exp Pathol. (2017) 10:10431–40.
65. Lois H. New advances in immunotherapy for prostate cancer. Life Res. (2019) 2:152. doi: 10.53388/life2019-1025-302
66. Pallavi VR, Devi KU, Mukherjee G, Ramesh C, Bafna UD. Relationship between lymph node metastases and histopathological parameters in carcinoma cervix: a multivariate analysis. J Obstet Gynaecol. (2012) 32:78–80. doi: 10.3109/01443615.2011.625455
67. Ki EY, Lee KH, Park JS, Hur SY. A clinicopathological review of pulmonary metastasis from uterine cervical cancer. Cancer Res Treat. (2016) 48:266–72. doi: 10.4143/crt.2014.206
68. Zhang J, Qin L, Chen H-M, Hsu H-C, Chuang C-C, Chen D, et al. Overall survival, locoregional recurrence, and distant metastasis of definitive concurrent chemoradiotherapy for cervical squamous cell carcinoma and adenocarcinoma: before and after propensity score matching analysis of a cohort study. Am J Cancer Res. (2020) 10:1808–20. doi: 10.2139/ssrn.3582834
69. Blomberg K, Ternestedt B-M, Törnberg S, Tishelman C. How do women who choose not to participate in population-based cervical cancer screening reason about their decision? Psychooncology. (2008) 17:561–9. doi: 10.1002/pon.1270
70. Imachi M, Tsukamoto N, Matsuyama T, Nakano H. Pulmonary metastasis from carcinoma of the uterine cervix. Gynecol Oncol. (1989) 33:189–92. doi: 10.1016/0090-8258(89)90549-0
Keywords: cervical cancer, brain metastasis, neurovascular, clinical prediction model, radiomics, ADRB1, biomarkers
Citation: Zheng X, Xu S and Wu J (2022) Cervical Cancer Imaging Features Associated With ADRB1 as a Risk Factor for Cerebral Neurovascular Metastases. Front. Neurol. 13:905761. doi: 10.3389/fneur.2022.905761
Received: 27 March 2022; Accepted: 20 June 2022;
Published: 12 July 2022.
Edited by:
Yuzhen Xu, Tongji University, ChinaReviewed by:
Jie Yang, Affiliated Hospital of Shandong University of Traditional Chinese Medicine, ChinaSu-Ding Fei, Ningbo College of Health Sciences, China
Copyright © 2022 Zheng, Xu and Wu. This is an open-access article distributed under the terms of the Creative Commons Attribution License (CC BY). The use, distribution or reproduction in other forums is permitted, provided the original author(s) and the copyright owner(s) are credited and that the original publication in this journal is cited, in accordance with accepted academic practice. No use, distribution or reproduction is permitted which does not comply with these terms.
*Correspondence: JiaYing Wu, dGluZWVAMjFjbi5jb20=
†These authors have contributed equally to this work