- 1Department of Neurology, National Clinical Research Center for Geriatric Diseases, Xuanwu Hospital of Capital Medical University, Beijing, China
- 2Institute of Genetics and Developmental Biology, Chinese Academy of Sciences, Beijing, China
- 3Department of Neurobiology, National Clinical Research Center for Geriatric Diseases, Xuanwu Hospital of Capital Medical University, Beijing, China
- 4Department of Neurology, Fujian Key Laboratory of Molecular Neurology, Fujian Medical University Union Hospital, Institute of Neuroscience, Fujian Medical University, Fuzhou, China
- 5The Xinjiekou Community Health Service Center, Beijing, China
- 6The Qinglonghu Community Health Service Center, Beijing, China
- 7Department of Geriatric Disease, Peking University Shenzhen Hospital, Shenzhen, China
Objectives: To develop and validate a predictive nomogram for idiopathic rapid eye movement (REM) sleep behavior disorder (RBD) in a community population in Beijing, China.
Methods: Based on the validated RBD questionnaire-Hong Kong (RBDQ-HK), we identified 78 individuals with possible RBD (pRBD) in 1,030 community residents from two communities in Beijing. The least absolute shrinkage and selection operator (LASSO) regression was applied to identify candidate features and develop the nomogram. Internal validation was performed using bootstrap resampling. The discrimination of the nomogram was evaluated using the area under the curve (AUC) of the receiver operating characteristic (ROC) curve, and the predictive accuracy was assessed via a calibration curve. Decision curve analysis (DCA) was performed to evaluate the clinical value of the model.
Results: From 31 potential predictors, 7 variables were identified as the independent predictive factors and assembled into the nomogram: family history of Parkinson's disease (PD) or dementia [odds ratio (OR), 4.59; 95% confidence interval (CI), 1.35–14.45; p = 0.011], smoking (OR, 3.24; 95% CI, 1.84–5.81; p < 0.001), physical activity (≥4 times/week) (OR, 0.23; 95% CI, 0.12–0.42; p < 0.001), exposure to pesticides (OR, 3.73; 95%CI, 2.08–6.65; p < 0.001), constipation (OR, 6.25; 95% CI, 3.58–11.07; p < 0.001), depression (OR, 3.66; 95% CI, 1.96–6.75; p < 0.001), and daytime somnolence (OR, 3.28; 95% CI, 1.65–6.38; p = 0.001). The nomogram displayed good discrimination, with original AUC of 0.885 (95% CI, 0.845–0.925), while the bias-corrected concordance index (C-index) with 1,000 bootstraps was 0.876. The calibration curve and DCA indicated the high accuracy and clinical usefulness of the nomogram.
Conclusions: This study proposed an effective nomogram with potential application in the individualized prediction for pRBD.
Introduction
Rapid eye movement (REM) sleep behavior disorder (RBD) is a parasomnia characterized by loss of normal muscle atonia during the REM sleep, presenting with complex behaviors associated with a nightmare (1). The gold standard diagnostic criterion of RBD is video-polysomnography (vPSG), with a video demonstration of complex motor behaviors accompanied by an excess of muscle tone and/or phasic muscle twitching during REM sleep (2). The clinical features of RBD could be variable with a spectrum of dream-enacting behavioral expression from simple acts, such as talking in sleep and shouting to complex body movements that include punching, kicking, and falling out of bed (3), which can lead to significant injuries. These severe symptoms usually prompt patients to seek medical aid.
Idiopathic RBD (iRBD) is by far the strongest risk factor for prodromal synucleinopathies (4), a group of neurodegenerative disorders, such as Parkinson's disease (PD), multiple system atrophy (MSA), and dementia with Lewy bodies (DLB) (5–8). They all have a long prodromal stage in which symptoms of neurodegeneration are perceptible, but the full clinical disease has not developed yet (9). More than 80% of individuals with iRBD will develop one of these neurodegenerative diseases within 20 years of onset of iRBD (6, 10). Because of the strong relationship between iRBD and neurodegenerative diseases, identification of risk factors for iRBD and early detection of individuals who are likely to develop iRBD is of great importance and may aid in delivering the proper intervention and minimizing its injurious potential. Furthermore, patients with RBD can be ideal candidates for screening new neuroprotective methods and a better understanding of the progression and pathophysiology of synucleinopathies from their presymptomatic phases. Some large population-based studies reported the potential risk factors for iRBD (11–15), such as socioeconomic status, male sex, lower education, head injury, pesticide exposure, cigarette smoking, alcohol use, carbon monoxide (CO) poisoning, a family history of PD or dementia, psychological distress, and so on. Considering that vPSG is time and resource consuming and can only be feasible in medical centers, most of the studies used questionnaires as screening tools for iRBD diagnosis.
Although many predictive factors for iRBD have been proposed, none of them has been integrated into a prediction model. The nomogram has been accepted as a reliable tool to create a simple intuitive graph of a statistical predictive model that quantifies the risk of a clinical event (16, 17). Nomograms enable specific individual risk scores by numerical estimation of the possibility of an event that is tailored to the situation of an individual. The use of nomograms is facilitated by user-friendly graphical interfaces for generating the estimates during clinical encounters to inform clinical decision-making (17). The aim of this study was to develop and validate a nomogram for predicting the risk of possible RBD (pRBD), based on two elderly community cohorts in Beijing, China.
Methods
Study Design and Data Source
The study was approved by the Medical Ethics Committee of Xuanwu Hospital of Capital Medical University. Informed consent was obtained from all subjects in the study. The present study used a random sampling method to select a community cohort of Beijing residents aged ≥50 years from April 2019 to September 2021. Individuals were selected from 7 community units of one urban district (Xicheng district) and 13 community units of a suburb district (Fangshan district). In total, 1,300 persons were invited, of whom 232 were not eligible, mainly because inclusion criteria were not met (n = 133), the persons moved before recruitment (n = 39), mobile phone was not connected (n = 55), or deceased (n = 5), resulting in a final source population of 1,068 persons. Among them, there were 38 individuals who missed data for some critical assessments and, thus, were excluded from the study (Supplementary Figure S1). The response rate of the study was 96.44%. The individuals were excluded from the study using the following criteria: (i) subjects with PD-related motor symptoms (bradykinesia, tremor, postural instability, and rigidity); (ii) diagnosed with dementia or PD and other neurodegenerative diseases; (iii) subjects with malignant tumors or other serious systemic diseases. All the subjects were assessed for demographic information, history of chronic diseases, medication use, lifestyle behaviors, and environmental exposures. Subjects were also assessed for motor and non-motor symptoms of PD, such as RBD status, using scales (additional information was given in Supplementary Material). All the assessments were performed via face-to-face interviews by clinical investigators with unified training.
Candidate Predictors
Based on the previously published literature (11–15), the following 31 potential predictive variables were selected for prediction: demographic variables (age, sex, and education level), body mass index (BMI), family history of PD or dementia, medical history (hypertension, diabetes, coronary heart disease, hyperlipidemia, stroke, head injury with unconsciousness, CO poisoning with unconsciousness, and general anesthesia), medications (such as aspirin, statins, calcium antagonist, and antidiabetics), non-motor symptoms of PD [olfactory dysfunction, constipation, cognitive impairment, depression, daytime somnolence, and scale for outcomes in PD-autonomic (SCOPA-AUT) scores], lifestyle (smoking, drinking of alcohol, tea, coffee, and physical activity), and environmental exposures (pesticides, organic solvents, and heavy metals).
Outcome Measure
The main outcome was the diagnosis of pRBD using the validated RBD Questionnaire–Hong Kong (RBDQ-HK) (Supplementary Table S1). The RBDQ-HK is a self-administered questionnaire comprising 13 questions related to various clinical features of RBD, which are rated on scales of lifetime occurrence and recent 1-year frequency (18). The questionnaire consists of factor 1 (Q1–Q5, and Q13, dream-related factor) and factor 2 (Q6–Q12, behavioral manifestations factor). The best cutoff score for the overall RBDQ-HK questionnaire was found to be at 18/19, which had good sensitivity (82.2%) and specificity (86.9%) in a large PSG-based study (18). The RBDQ-HK overall scale had a sensitivity of 85% and specificity of 81% in another validation study in East China, which included patients with PD or obstructive sleep apnea (19). Therefore, a score ≥19 for the overall scale of RBDQ-HK was considered to be pRBD status in the study, without further verification by PSG.
Statistical Analysis
All statistical analyses were performed with the R software (version 4.1.1; R Foundation for Statistical Computing Vienna, Austria; http://www.R-project.org/). Categorical variables were expressed as frequencies and percentages, while continuous variables were described as medians and interquartile ranges (IQRs). Mann-Whitney U tests (for continuous variables with skewness distributions) and the chi-square test (for categorical variables) were utilized to compare the differences in characteristics between groups. The R package “glmnet” was used to perform the least absolute shrinkage and selection operator (LASSO) regression and the R package “rms” to establish the nomogram and calibration curve. The R package “pROC,” “plotROC,” and “rmda” were applied to generate the receiver operating characteristic (ROC) and decision curve analysis (DCA). All tests were two-tailed and p < 0.05 was defined as statistically significant (original data and analysis code were given in Supplementary Material).
Variable Selection and Establishment of a Predictive Model
The least absolute shrinkage and selection operator (LASSO) regression with 10-fold cross-validation was used for the most useful predictive factors from the initial dataset to address multiple cross-related covariates and reduce the risk of overfitting the data (20). It adds an L1 norm as a penalty in the calculation of the minimum residual sum of squares (RSS). As the lambda gets large, some coefficients can be accurately shrunk to zero (20, 21). We then selected the lambda of 1 standard for which the cross-validation error is the smallest. Multivariable logistic regression analysis was performed to develop a predictive model of pRBD risk based on the predictors selected by LASSO. A nomogram was created based on the results of multivariable analyses. Predictors whose p-values were <0.05 were included.
Model Performance
Model performance is divided into two main categories: discriminative ability and model calibration. The discriminative ability assesses whether the model is able to differentiate between patients with a favorable and an unfavorable outcome. It is expressed by the area under the curve (AUC) of a ROC curve (22). Model calibration assesses to what extent predicted values agree with the observed outcomes. It was assessed using a calibration plot, in which predicted probabilities are plotted against observed outcome frequencies (23). For a well-calibrated model, the predictions should fall on a 45-degree diagonal line. Overall concordance between predicted and observed outcomes was tested using the Hosmer-Lemeshow test (23). A p > 0.05 was considered as well calibrated.
Model Validation
Internal validation evaluating the stability of a prediction model to random changes in sample composition was performed by the bootstrap resampling, in which regression models were fitted in 1,000 bootstrap replicates, drawn with replacement from the development sample (24). The model was refitted in each bootstrap replicate and tested on the original sample to estimate optimism in model performance (17). Specifically, a bias-corrected concordance index (C-index) was estimated using a 1,000-sample bootstrap to calculate the discrimination of the model.
Clinical Utility of Nomogram
The clinical usefulness of the nomogram was determined by DCA by quantifying the net benefit to the subjects under different threshold probabilities (25).
Results
Clinical and Demographic Characteristics
Table 1 summarizes the demographic and clinical data of the subjects. The median age of the subjects was 68 years (IQR, 64–72 years). Men accounted for 39.22% and women accounted for 60.78%, and most had received junior to middle school education. After screening using the RBDQ-HK scale, a total of 78 subjects were positive for pRBD. Among all the subjects, 2.04% had a family history of PD or dementia, 57.57% had hypertension, 27.57% had diabetes, 12.62% had coronary heart disease, 6.89% had a stroke, and 38.16% had hyperlipidemia.
Subjects with pRBD were more probable to report a family history of PD or dementia than subjects without pRBD and were more likely to be smokers or alcohol drinkers when compared with subjects without pRBD. Subjects with pRBD had a lower frequency of physical activity than those without. We found a significantly higher occurrence of exposure to pesticides in subjects with pRBD than in those without. We also found an increased prevalence of constipation, depression, and daytime somnolence among subjects with pRBD. On the SCOPA-AUT scores, subjects with pRBD had a higher score than those without. No statistically significant differences were found in other factors between the subjects with and without pRBD.
Variable Selection and Model Construction
A LASSO regression model with 10-fold cross-validation was employed to select predictive variables among the preliminarily screened factors (Table 1). A total of 31 variables assessed at baseline were included in the LASSO regression. After LASSO regression selection (Figure 1), 7 variables remained to be non-zero coefficients that minimized the overall Lambda and were confirmed as the potentially optimal variables for predicting pRBD, such as a family history of PD or dementia, smoking, physical activity, exposure to pesticides, constipation, depression, and daytime somnolence.
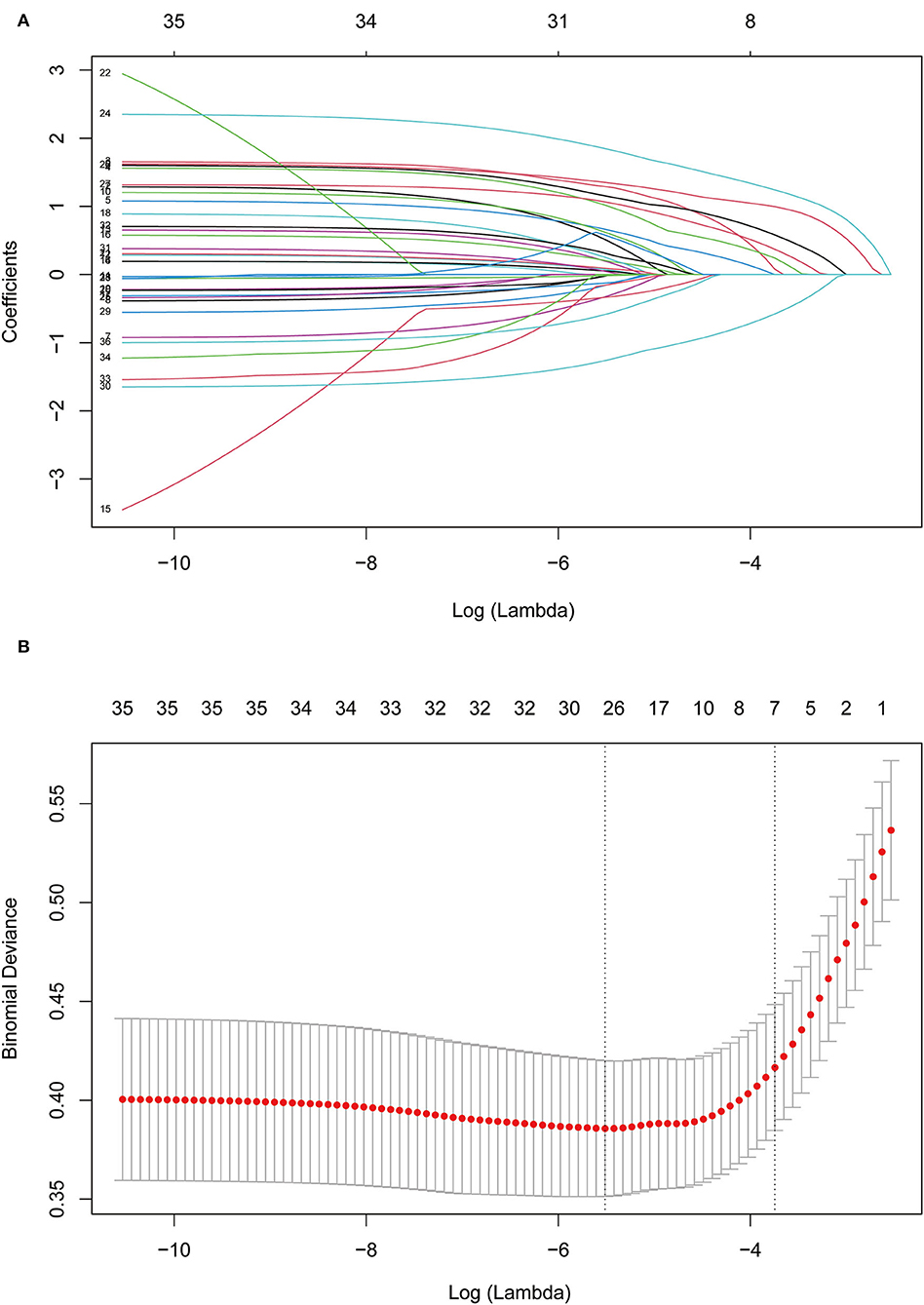
Figure 1. Feature selection using Lasso regression. (A) Lasso coefficient profiles of the candidate features. (B) The selection of optimal parameters (lambda) by 10-fold cross-validation. The left and right dotted vertical lines, respectively, represented the optimal lambda values when using the minimum error criterion and one standard error (1-SE) of the minimum criterion.
Inclusion of these 7 variables in a multivariate logistic regression model resulted in all the 7 variables that were independently statistically significant predictors for pRBD. These variables included family history of PD or dementia [odds ratio (OR), 4.59; 95% confidence interval (CI), 1.35–14.45; p = 0.011], smoking (OR, 3.24; 95% CI, 1.84–5.81; p < 0.001), physical activity (≥4 times/week) (OR, 0.23; 95% CI, 0.12–0.42; p < 0.001), exposure to pesticides (OR, 3.73; 95% CI, 2.08–6.65; p < 0.001), constipation (OR, 6.25; 95% CI, 3.58–11.07; p < 0.001), depression (OR, 3.66; 95% CI, 1.96–6.75; p < 0.001), and daytime somnolence (OR, 3.28; 95% CI, 1.65–6.38; p = 0.001; Table 2). Using these variables, the nomogram was constructed.
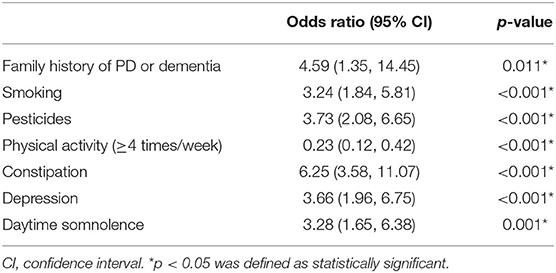
Table 2. Parameters selected for multivariate logistic regression models for predicting possible REM sleep behavior disorder (pRBD).
Performance of the Nomograms and Bootstrap Internal Validation
The nomogram is generated and shown in Figure 2. In the nomogram, each value of a variable corresponds to a score, and the corresponding scores for the 7 variables included in the model were summed to achieve a total score for an individual. The total score of an individual was then projected onto a total point scale to obtain the probability of risk of pRBD. The nomogram had high discrimination, with an AUC of 0.885 (95% CI, 0.845–0.925; Figure 3). The optimism-corrected C-index obtained from bootstrap resampling with 1,000 iterations was 0.876, suggesting good internal validation. In addition, the Hosmer-Lemeshow test indicated that the model calibrated well (p = 0.980). A calibration curve with 500 bootstrap resamples is presented in Figure 4, which showed that the pRBD probabilities predicted by the nomogram agreed well with the observed probabilities (p = 0.956).
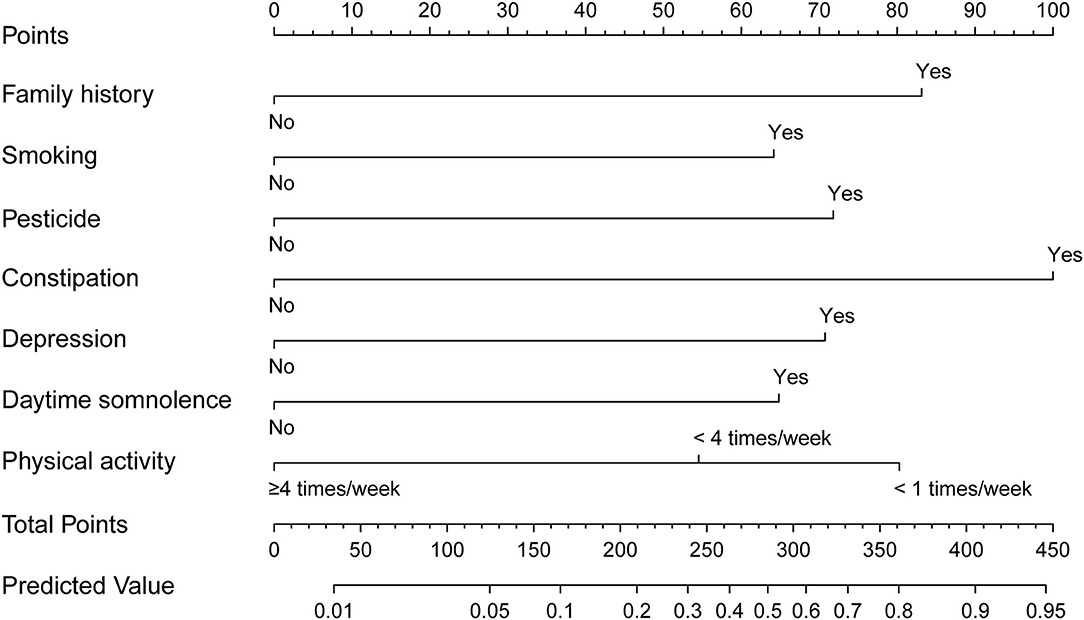
Figure 2. Nomogram to estimate the probability of possible rapid eye movement sleep behavior disorder (pRBD). Find the predictor points on the uppermost point scale that correspond to each subject variable and add them up. The total points projected to the bottom scale indicate the probability of pRBD.
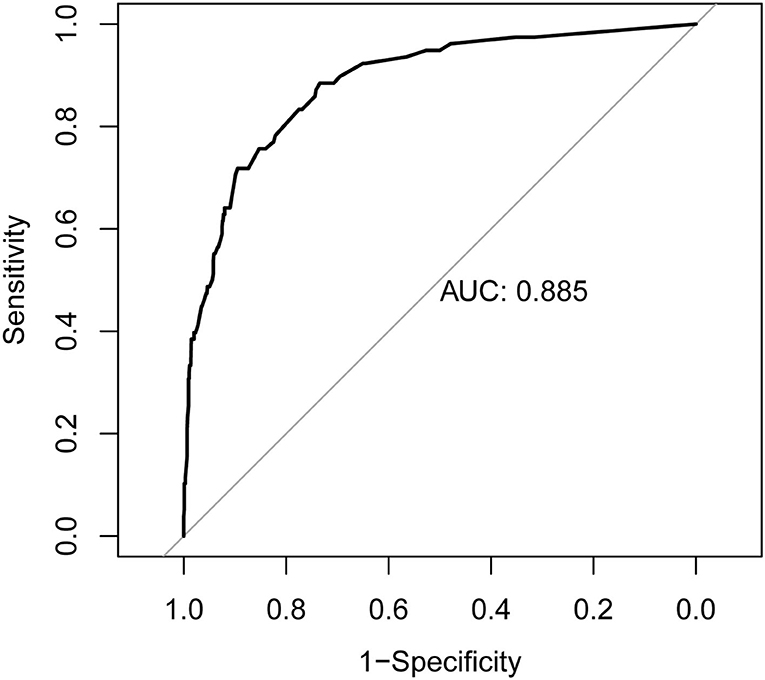
Figure 3. Receiver operating characteristic (ROC) curve of the nomogram. The nomogram had good discriminative power with area under ROC curve of 0.885 (95% CI, 0.845–0.925).
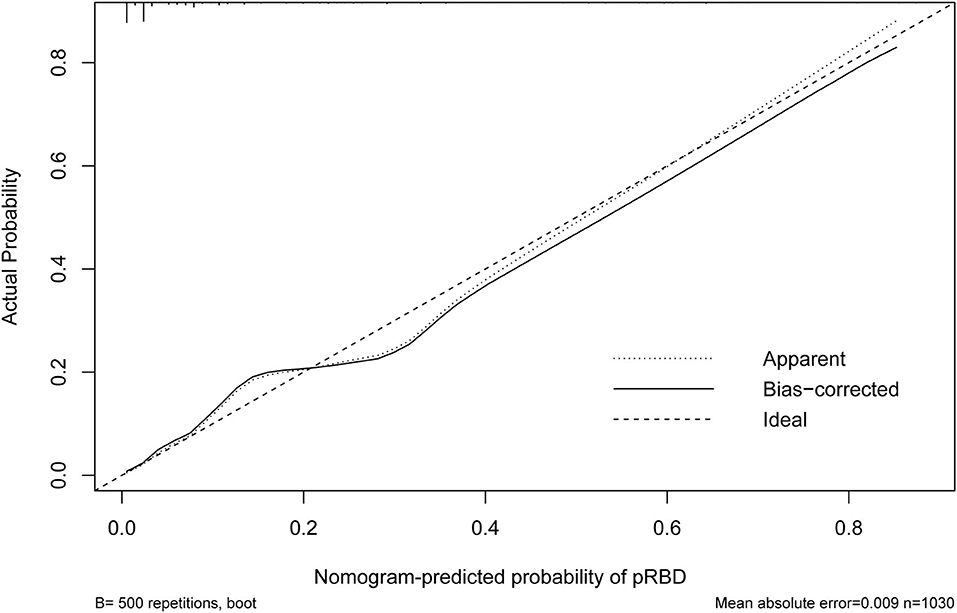
Figure 4. Calibration curve of the nomogram. The X-axis represented the predicted possible rapid eye movement sleep behavior disorder (pRBD) risk. The Y-axis represented the actual diagnosed pRBD. The diagonal dotted line meant a perfect prediction by an ideal model. The short-dashed line represented the apparent prediction of nomogram, and the solid line was bias-corrected by bootstrapping (B = 500 repetitions), indicating observed nomogram performance.
Clinical Utility of Nomogram
The DCA for the nomogram is presented in Figure 5. DCA illustrated that the nomogram model has an obvious net benefit for most of the probabilities, especially in threshold probabilities of 10–85%.
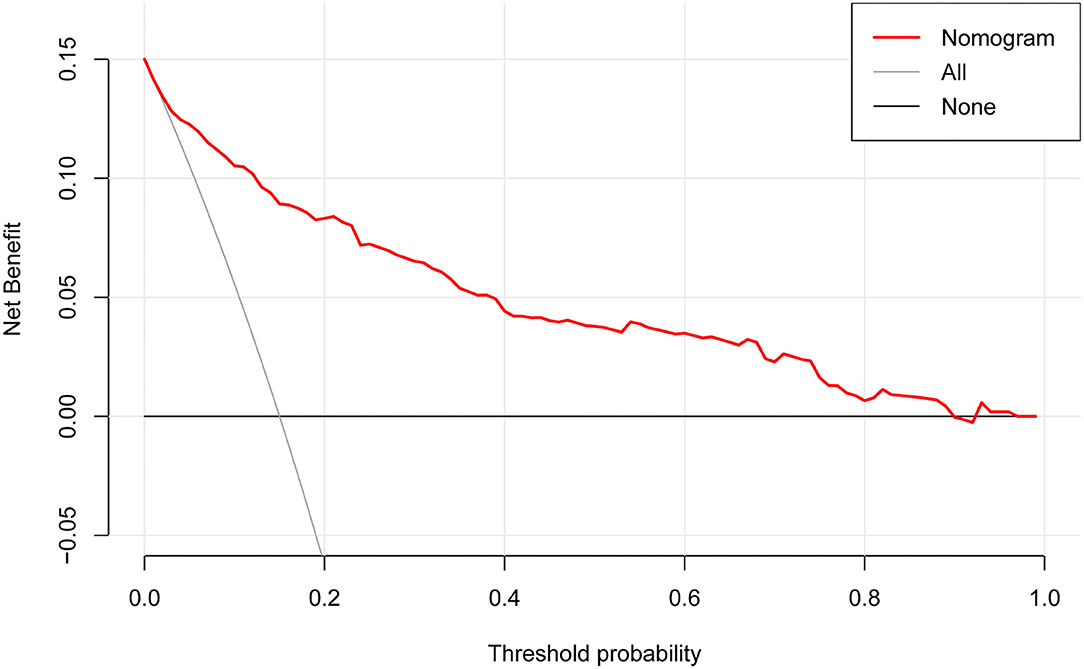
Figure 5. Decision curve analysis for the nomogram. The X-axis showed the threshold probability. The Y-axis measured the net benefit. The red solid line represented the nomogram. The gray solid line represented the assumption that all subjects were possible rapid eye movement sleep behavior disorder (pRBD). The black solid line represents the assumption that no subjects were pRBD.
Discussion
The current study has developed a nomogram for predicting pRBD in the community population. It is well discriminated and calibrated for the personal prediction and facilitates individualized treatment. This will improve clinical decisions for clinicians and help patients to get more net benefits. To our knowledge, this study is the first to develop a nomogram for predicting risk for individuals with pRBD. The nomogram visualized the predictive model and made clinical use convenient.
Different from the traditional multivariate logistic regression analysis method, this study adopted the LASSO regression analysis, which performed well in reducing the data dimensionality and decreasing multicollinearity between variables, and it has emerged as a powerful tool under the theory of bias-variance tradeoff in the variable selection (20, 21). Compared with a single predictive factor, which has limited predictive value and is easily affected by multiple factors, the nomogram (a combination of predictive factors) has a tendency for the prediction of higher sensitivity and specificity.
In this study, we found that genetic factors, lifestyles, and environmental exposures are strong predictors of pRBD, which are mostly consistent with previous studies but may differ from those previously defined for neurodegenerative synucleinopathies (11–15, 26). However, the mechanisms underlying these factors in RBD remain largely unclear. We found that a family history of PD or dementia was associated with RBD but this has not been widely reported. Studies have suggested the clustering of the RBD features and increased PD risk and dementia in the iRBD family members (27, 28) and a large population-based study found participants with pRBD were more likely to report a family history of parkinsonism/dementia than controls (11). Genetic studies established that the glucocerebrosidase (GBA) mutations (29) and TMEM175 variant (30) have been associated with both PD and RBD. However, the PD-associated leucine-rich repeat kinase 2 (LRRK2) mutations (31) and microtubule-associated protein tau (MAPT) H2/H1 haplotypes (32) were not associated with RBD, and neither was the AD-related Apolipoprotein E (APOE) ε4 allele (33), suggesting that the genetics of RBD only partially overlaps with PD and DLB. In lifestyles, unlike PD, RBD risk was positively associated with smoking but not with coffee intake, as confirmed in several large epidemiologic studies (14, 15, 26, 34). The explanations for such paradox epidemiological correlations were unclear, but smoking may selectively protect substantia nigra but not other structures involved in synucleinopathies (35), while coffee was infrequently used in the Chinese population. Moreover, a potential protective role for physical activity (≥4 times/week) has been suggested by our study. A recent study suggested that physical inactivity serves as an early and robust prodromal marker of the conversion of iRBD into clinically diagnosed synucleinopathies (36). Further, pesticide exposure is a well-known risk factor for PD and has also been associated with a higher likelihood of RBD in the current and previous studies (26).
We also identified a strong relationship between pRBD and several other non-motor symptoms of PD. There was a positive association between RBD and comorbid depression (37, 38). However, there has been controversy on the role of antidepressants and RBD features, since antidepressants may both treat prodromal depression and trigger early RBD by increasing REM muscle tone and dreaming activity (39). A previous study used the hyperechoic examination of the raphe of the brain stem and substantia nigra to predict the depression in iRBD with a sensitivity of 23.1% and a specificity of 97.1% (40). This finding indicated that serotonergic dorsal raphe dysfunction may be involved in the pathophysiological process of depression in iRBD (40). Our finding that constipation had the strongest relevance with pRBD is consistent with the biopsy studies showing α-synuclein immunostaining in colons of patients with iRBD (41) and with the Braak's hypothesis that α-synuclein pathology may begin with the enteric nervous system (42). Moreover, there has been controversy in the association between RBD and daytime somnolence (36, 43–46), possibly due to the relatively short time period for following up the process of neurodegeneration. In this regard, further prospective studies with a larger sample size, longer follow-up period, and periodic assessment are warranted to determine the association of daytime somnolence with RBD.
Idiopathic RBD, which represents a premonitory symptom of impending neurodegeneration, offers a precious time window, so early identification of pRBD is important to allow neuroprotective and early management strategies to be administered prior to the neurodegenerative diseases. In this study, we used the screened predictors to construct and validate the nomogram model. We found and validated that a combination of family history of PD or dementia, smoking, pesticide exposure, and several non-motor symptoms are useful for predicting pRBD. Since it combines simple and easy-to-collect variables that can easily be gathered in the clinic and communities, the model provides an accurate, non-invasive, low-cost, and rapid method for screening high-risk individuals for pRBD and neurodegenerative disorders. In addition, due to the strength and utility of this nomogram and the easy way to implement, the nomogram is likely to be useful in large population-based primary screening studies.
There are several limitations of this study. First, a major limitation is that the diagnosis of pRBD was completely based on a self-report questionnaire but not the formal PSG confirmation (11–13, 47, 48). As noted in all epidemiological studies, there was a high prevalence of false-positive screen cases as judged by the discrepancy between pRBD and iRBD prevalence. In fact, the prevalence of pRBD (i.e., 7.57%) in our study was similar to those of other population-based studies (i.e., 3.48–7.70%) (49–52) but was higher than those in studies with PSG confirmation (i.e., 0.68–1.15%) (15, 51, 53). Thus, the association of the risk factors in pRBD could only be an approximate correlation and preliminary work for vPSG confirmed iRBD. Considering time and resource consumption, population-based studies on iRBD using PSG are scarce. Therefore, a two-phase screening method that includes an initial questionnaire-based screening for a large population and a PSG-based confirmation for screened positive individuals has been suggested to be an ideal approach (38). Second, the construction of the nomogram based on a cross-sectional study and a relatively small sample size, thus further larger scale studies are needed for validation. We are referring to further video-polysomnographic confirmation and will continue to build the cohort with an expanded sample size. Third, only internal validation was performed. The data for nomogram development and validation are entirely from the community population in Beijing, which could potentially limit the generalizability of the nomogram in other Chinese areas. Last, the success of the model will also rely on the comprehensiveness of the inclusion of the potential risk factors. Therefore, more factors need to be included in future analysis.
In conclusion, the nomogram we developed can successfully predict pRBD risk for elderly subjects. Future studies with larger numbers of participants will be useful for updating and validating this nomogram to improve predictions of pRBD risk among the community population.
Data Availability Statement
The raw data supporting the conclusions of this article will be made available by the authors, without undue reservation.
Ethics Statement
The studies involving human participants were reviewed and approved by the Medical Ethics Committee of Xuanwu Hospital of Capital Medical University ([2020]060). The patients/participants provided their written informed consent to participate in this study.
Author Contributions
HL designed the study, analyzed the data, interpreted the results, and wrote the manuscript. X-YL, JH, WL (4th author), FX, JZ, RH, HW, LC, JY, XL, YS, and WL (15th author) collected the data. XW, ZW, RK, YL, and JX supervised the study. YD and QY designed the study and supervised the study. CW designed the study, supervised the study, and revised the manuscript. All authors contributed to the article and approved the submitted version.
Funding
This study was supported by grants from the National Natural Science Foundation (81771212 and 82171412), the Ministry of Science and Technology (2016YFC1306000), and the Special Fund from Key Laboratory of Neurodegenerative Diseases, Ministry of Education of China (PXM2019_026283_ 000002).
Conflict of Interest
The authors declare that the research was conducted in the absence of any commercial or financial relationships that could be construed as a potential conflict of interest.
Publisher's Note
All claims expressed in this article are solely those of the authors and do not necessarily represent those of their affiliated organizations, or those of the publisher, the editors and the reviewers. Any product that may be evaluated in this article, or claim that may be made by its manufacturer, is not guaranteed or endorsed by the publisher.
Acknowledgments
The authors are grateful to the participating patients for their involvement.
Supplementary Material
The Supplementary Material for this article can be found online at: https://www.frontiersin.org/articles/10.3389/fneur.2022.903721/full#supplementary-material
Abbreviations
REM, rapid eye movement; RBD, REM sleep behavior disorder; iRBD, idiopathic RBD; pRBD, possible REM sleep behavior disorder; PSG, polysomnography; vPSG, video-polysomnography; RBDQ-HK, RBD Questionnaire–Hong Kong; PD, Parkinson's disease; DLB, dementia with Lewy bodies; MSA, multiple system atrophy; BMI, body mass index; CI, confidence interval; CO, carbon monoxide; OR, odds ratio; IQRs, interquartile ranges; RSS, residual sum of squares; LASSO, least absolute shrinkage and selection operator; ROC, receiver operating characteristic; AUC, the area under ROC curve; C-index, Concordance index; DCA, decision curve analysis; SCOPA-AUT, scale for outcomes in PD-autonomic.
References
1. Schenck CH, Bundlie SR, Patterson AL, Mahowald MW. Rapid eye movement sleep behavior disorder. A treatable parasomnia affecting older adults. JAMA. (1987) 257:1786–9. doi: 10.1001/jama.257.13.1786
2. Sateia MJ. International classification of sleep disorders-third edition: highlights and modifications. Chest. (2014) 146:1387–94. doi: 10.1378/chest.14-0970
3. Frauscher B, Gschliesser V, Brandauer E, Ulmer H, Peralta CM, Müller J, et al. Video analysis of motor events in REM sleep behavior disorder. Mov Disord. (2007) 22:1464–70. doi: 10.1002/mds.21561
4. Postuma RB, Berg D, Stern M, Poewe W, Olanow CW, Oertel W, et al. MDS research criteria for prodromal Parkinson's disease. Mov Disord. (2015) 30:1600–11. doi: 10.1002/mds.26431
5. Postuma RB, Gagnon JF, Vendette M, Fantini ML, Massicotte-Marquez J, Montplaisir J. Quantifying the risk of neurodegenerative disease in idiopathic REM sleep behavior disorder. Neurology. (2009) 72:1296–300. doi: 10.1212/01.wnl.0000340980.19702.6e
6. Schenck CH, Boeve BF, Mahowald MW. Delayed emergence of a parkinsonian disorder or dementia in 81% of older men initially diagnosed with idiopathic rapid eye movement sleep behavior disorder: a 16-year update on a previously reported series. Sleep Med. (2013) 14:744–8. doi: 10.1016/j.sleep.2012.10.009
7. Claassen DO, Josephs KA, Ahlskog JE, Silber MH, Tippmann-Peikert M, Boeve BF, et al. Sleep behavior disorder preceding other aspects of synucleinopathies by up to half a century. Neurology. (2010) 75:494–9. doi: 10.1212/WNL.0b013e3181ec7fac
8. Iranzo A, Fernández-Arcos A, Tolosa E, Serradell M, Molinuevo JL, Valldeoriola F, et al. Neurodegenerative disorder risk in idiopathic REM sleep behavior disorder: study in 174 patients. PLoS ONE. (2014) 9:e89741. doi: 10.1371/journal.pone.0089741
9. McKeith IG, Ferman TJ, Thomas AJ, Blanc F, Boeve BF, Fujishiro H, et al. Research criteria for the diagnosis of prodromal dementia with Lewy bodies. Neurology. (2020) 94:743–55. doi: 10.1212/WNL.0000000000009323
10. Postuma RB, Iranzo A, Hu M, Högl B, Boeve BF, Manni R, et al. Risk and predictors of dementia and parkinsonism in idiopathic REM sleep behaviour disorder: a multicentre study. Brain. (2019) 142:744–59. doi: 10.1093/brain/awz030
11. Zhang H, Gu Z, Yao C, Cai Y, Li Y, Mao W, et al. Risk factors for possible REM sleep behavior disorders: a community-based study in Beijing. Neurology. (2020) 95:e2214–e24. doi: 10.1212/WNL.0000000000010610
12. Wong JC, Li J, Pavlova M, Chen S, Wu A, Wu S, et al. Risk factors for probable REM sleep behavior disorder: a community-based study. Neurology. (2016) 86:1306–12. doi: 10.1212/WNL.0000000000002414
13. Ma JF, Qiao Y, Gao X, Liang L, Liu XL Li DH, et al. A community-based study of risk factors for probable rapid eye movement sleep behavior disorder. Sleep Med. (2017) 30:71–6. doi: 10.1016/j.sleep.2016.06.027
14. Yao C, Fereshtehnejad SM, Keezer MR, Wolfson C, Pelletier A, Postuma RB. Risk factors for possible REM sleep behavior disorder: a CLSA population-based cohort study. Neurology. (2018) 92:e475–85. doi: 10.1212/WNL.0000000000006849
15. Haba-Rubio J, Frauscher B, Marques-Vidal P, Toriel J, Tobback N, Andries D, et al. Prevalence and determinants of rapid eye movement sleep behavior disorder in the general population. Sleep. (2018) 41:zsx197. doi: 10.1093/sleep/zsx197
16. Moons KG, Altman DG, Reitsma JB, Ioannidis JP, Macaskill P, Steyerberg EW, et al. Transparent reporting of a multivariable prediction model for individual prognosis or diagnosis (TRIPOD): explanation and elaboration. Ann Intern Med. (2015) 162:W1–73. doi: 10.7326/M14-0698
17. Iasonos A, Schrag D, Raj GV, Panageas KS. How to build and interpret a nomogram for cancer prognosis. J Clin Oncol. (2008) 26:1364–70. doi: 10.1200/JCO.2007.12.9791
18. Li SX, Wing YK, Lam SP, Zhang J, Yu MW. Validation of a new REM sleep behavior disorder questionnaire (RBDQ-HK). Sleep Med. (2010) 11:43–8. doi: 10.1016/j.sleep.2009.06.008
19. Shen SS, Shen Y, Xiong KP, Chen J, Mao CJ, Huang JY, et al. Validation study of REM sleep behavior disorder questionnaire-Hong Kong (RBDQ-HK) in east China. Sleep Med. (2014) 15:952–8. doi: 10.1016/j.sleep.2014.03.020
20. Friedman J, Hastie T, Tibshirani R. Regularization paths for generalized linear models via coordinate descent. J Stat Softw. (2010) 33:1–22. doi: 10.18637/jss.v033.i01
21. Tibshirani R. The lasso method for variable selection in the Cox model. Stat Med. (1997) 16:385–95. doi: 10.1002/(SICI)1097-0258(19970228)16:4<385::AID-SIM380>3.0.CO;2-3
22. Harrell FE, Jr., Lee KL, Califf RM, Pryor DB, Rosati RA. Regression modelling strategies for improved prognostic prediction. Stat Med. (1984) 3:143–52. doi: 10.1002/sim.4780030207
23. Kramer AA, Zimmerman JE. Assessing the calibration of mortality benchmarks in critical care: the Hosmer-Lemeshow test revisited. Crit Care Med. (2007) 35:2052–6. doi: 10.1097/01.CCM.0000275267.64078.B0
24. Iba K, Shinozaki T, Maruo K, Noma H. Re-evaluation of the comparative effectiveness of bootstrap-based optimism correction methods in the development of multivariable clinical prediction models. BMC Med Res Methodol. (2021) 21:9. doi: 10.1186/s12874-020-01201-w
25. Vickers AJ, Elkin EB. Decision curve analysis: a novel method for evaluating prediction models. Med Decis Making. (2006) 26:565–74. doi: 10.1177/0272989X06295361
26. Postuma RB, Montplaisir JY, Pelletier A, Dauvilliers Y, Oertel W, Iranzo A, et al. Environmental risk factors for REM sleep behavior disorder: a multicenter case-control study. Neurology. (2012) 79:428–34. doi: 10.1212/WNL.0b013e31825dd383
27. Dauvilliers Y, Postuma RB, Ferini-Strambi L, Arnulf I, Högl B, Manni R, et al. Family history of idiopathic REM behavior disorder: a multicenter case-control study. Neurology. (2013) 80:2233–35. doi: 10.1212/WNL.0b013e318296e967
28. Liu Y, Zhang J, Lam SP, Zhou J, Yu MWM, Li SX, et al. A case-control-family study of idiopathic rapid eye movement sleep behavior disorder. Ann Neurol. (2019) 85:582–92. doi: 10.1002/ana.25435
29. Gan-Or Z, Mirelman A, Postuma RB, Arnulf I, Bar-Shira A, Dauvilliers Y, et al. GBA mutations are associated with rapid eye movement sleep behavior disorder. Ann Clin Transl Neurol. (2015) 2:941–5. doi: 10.1002/acn3.228
30. Krohn L, Öztürk TN, Vanderperre B, Ouled Amar Bencheikh B, Ruskey JA, Laurent SB, et al. Genetic, structural, and functional evidence link TMEM175 to synucleinopathies. Ann Neurol. (2020) 87:139–53. doi: 10.1002/ana.25629
31. Ouled Amar Bencheikh B, Ruskey JA, Arnulf I, Dauvilliers Y, Monaca CC, et al. LRRK2 protective haplotype and full sequencing study in REM sleep behavior disorder. Parkinsonism Relat Disord. (2018) 52:98–101. doi: 10.1016/j.parkreldis.2018.03.019
32. Li J, Ruskey JA, Arnulf I, Dauvilliers Y, Hu MTM, Högl B, et al. Full sequencing and haplotype analysis of MAPT in Parkinson's disease and rapid eye movement sleep behavior disorder. Mov Disord. (2018) 33:1016–20. doi: 10.1002/mds.27385
33. Gan-Or Z, Montplaisir JY, Ross JP, Poirier J, Warby SC, Arnulf I, et al. The dementia-associated APOE ε4 allele is not associated with rapid eye movement sleep behavior disorder. Neurobiol Aging. (2017) 49:218.e13–.e15. doi: 10.1016/j.neurobiolaging.2016.10.002
34. Ascherio A, Schwarzschild MA. The epidemiology of Parkinson's disease: risk factors and prevention. Lancet Neurol. (2016) 15:1257–72. doi: 10.1016/S1474-4422(16)30230-7
35. Postuma RB, Iranzo A, Hogl B, Arnulf I, Ferini-Strambi L, Manni R, et al. Risk factors for neurodegeneration in idiopathic rapid eye movement sleep behavior disorder: a multicenter study. Ann Neurol. (2015) 77:830–9. doi: 10.1002/ana.24385
36. Feng H, Chen L, Liu Y, Chen X, Wang J, Yu MWM, et al. Rest-activity pattern alterations in idiopathic REM sleep behavior disorder. Ann Neurol. (2020) 88:817–29. doi: 10.1002/ana.25853
37. Frauscher B, Jennum P, Ju YE, Postuma RB, Arnulf I, Cochen De Cock V, et al. Comorbidity and medication in REM sleep behavior disorder: a multicenter case-control study. Neurology. (2014) 82:1076–9. doi: 10.1212/WNL.0000000000000247
38. Mok V, Wing YK. Prevalence and correlates of REM sleep behaviour disorder in patients with major depressive disorder: a two-phase study. J Neurol Neurosurg Psychiatry. (2021) jnnp-2021-327460. doi: 10.1136/jnnp-2021-327460. [Epub ahead of print].
39. Arnaldi D, Antelmi E, St Louis EK, Postuma RB, Arnulf I. Idiopathic REM sleep behavior disorder and neurodegenerative risk: to tell or not to tell to the patient? How to minimize the risk? Sleep Med Rev. (2017) 36:82–95. doi: 10.1016/j.smrv.2016.11.002
40. Vilas D, Iranzo A, Pont-Sunyer C, Serradell M, Gaig C, Santamaria J, et al. Brainstem raphe and substantia nigra echogenicity in idiopathic REM sleep behavior disorder with comorbid depression. J Neurol. (2015) 262:1665–72. doi: 10.1007/s00415-015-7745-0
41. Sprenger FS, Stefanova N, Gelpi E, Seppi K, Navarro-Otano J, Offner F, et al. Enteric nervous system α-synuclein immunoreactivity in idiopathic REM sleep behavior disorder. Neurology. (2015) 85:1761–8. doi: 10.1212/WNL.0000000000002126
42. Braak H, Del Tredici K, Rüb U, de Vos RA, Jansen Steur EN, Braak E. Staging of brain pathology related to sporadic Parkinson's disease. Neurobiol Aging. (2003) 24:197–211. doi: 10.1016/S0197-4580(02)00065-9
43. Zhou J, Zhang J, Lam SP, Chan JW, Mok V, Chan A, et al. Excessive daytime sleepiness predicts neurodegeneration in idiopathic REM sleep behavior disorder. Sleep. (2017) 40. doi: 10.1093/sleep/zsx041
44. Iranzo A, Serradell M, Santamaria J. Excessive daytime sleepiness does not predict neurodegeneration in idiopathic REM sleep behavior disorder. Sleep. (2017) 40. doi: 10.1093/sleep/zsx161
45. Arnulf I, Neutel D, Herlin B, Golmard JL, Leu-Semenescu S, Cochen de Cock V, et al. Sleepiness in idiopathic REM sleep behavior disorder and parkinson disease. Sleep. (2015) 8:1529–35. doi: 10.5665/sleep.5040
46. Postuma RB, Gagnon JF, Pelletier A, Montplaisir JY. Insomnia and somnolence in idiopathic RBD: a prospective cohort study. NPJ Parkinsons Dis. (2017) 3:9. doi: 10.1038/s41531-017-0011-7
47. Pujol M, Pujol J, Alonso T, Fuentes A, Pallerola M, Freixenet J, et al. Idiopathic REM sleep behavior disorder in the elderly Spanish community: a primary care center study with a two-stage design using video-polysomnography. Sleep Med. (2017) 40:116–21. doi: 10.1016/j.sleep.2017.07.021
48. Fernández-Arcos A, Iranzo A, Serradell M, Gaig C, Santamaria J. The clinical phenotype of idiopathic rapid eye movement sleep behavior disorder at presentation: a study in 203 consecutive patients. Sleep. (2016) 39:121–32. doi: 10.5665/sleep.5332
49. Boot BP, Boeve BF, Roberts RO, Ferman TJ, Geda YE, Pankratz VS, et al. Probable rapid eye movement sleep behavior disorder increases risk for mild cognitive impairment and Parkinson disease: a population-based study. Ann Neurol. (2012) 71:49–56. doi: 10.1002/ana.22655
50. Mahlknecht P, Seppi K, Frauscher B, Kiechl S, Willeit J, Stockner H, et al. Probable RBD and association with neurodegenerative disease markers: a population-based study. Mov Disord. (2015) 30:1417–21. doi: 10.1002/mds.26350
51. Cicero CE, Giuliano L, Luna J, Zappia M, Preux PM, Nicoletti A. Prevalence of idiopathic REM behavior disorder: a systematic review and meta-analysis. Sleep. (2021) 44:zsaa294. doi: 10.1093/sleep/zsaa294
52. Cicero CE, Giuliano L, Sgroi R, Squillaci R, Terravecchia C, Vancheri E, et al. Prevalence of isolated RBD in the city of Catania, Italy: a population-based study. J Clin Sleep Med. (2021) 17:2241–48. doi: 10.5664/jcsm.9416
Keywords: REM sleep behavior disorder (RBD), LASSO, nomogram, decision curve analysis (DCA), predictive model
Citation: Lai H, Li X-Y, Hu J, Li W, Xu F, Zhu J, He R, Weng H, Chen L, Yu J, Li X, Song Y, Wang X, Wang Z, Li W, Kang R, Li Y, Xu J, Deng Y, Ye Q and Wang C (2022) Development and Validation of a Predictive Nomogram for Possible REM Sleep Behavior Disorders. Front. Neurol. 13:903721. doi: 10.3389/fneur.2022.903721
Received: 24 March 2022; Accepted: 26 May 2022;
Published: 29 June 2022.
Edited by:
Erik K. St. Louis, Mayo Clinic, United StatesReviewed by:
Yun Kwok Wing, The Chinese University of Hong Kong, ChinaFeng Jiang, Fudan University, China
Chuyan Wu, Nanjing Medical University, China
Pingping Ning, Sichuan University, China
Copyright © 2022 Lai, Li, Hu, Li, Xu, Zhu, He, Weng, Chen, Yu, Li, Song, Wang, Wang, Li, Kang, Li, Xu, Deng, Ye and Wang. This is an open-access article distributed under the terms of the Creative Commons Attribution License (CC BY). The use, distribution or reproduction in other forums is permitted, provided the original author(s) and the copyright owner(s) are credited and that the original publication in this journal is cited, in accordance with accepted academic practice. No use, distribution or reproduction is permitted which does not comply with these terms.
*Correspondence: Chaodong Wang, cdongwang01@126.com; Qinyong Ye, unionqyye@163.com; Yuanfei Deng, dengyf5666@hotmail.com
†These authors have contributed equally to this work and share last authorship