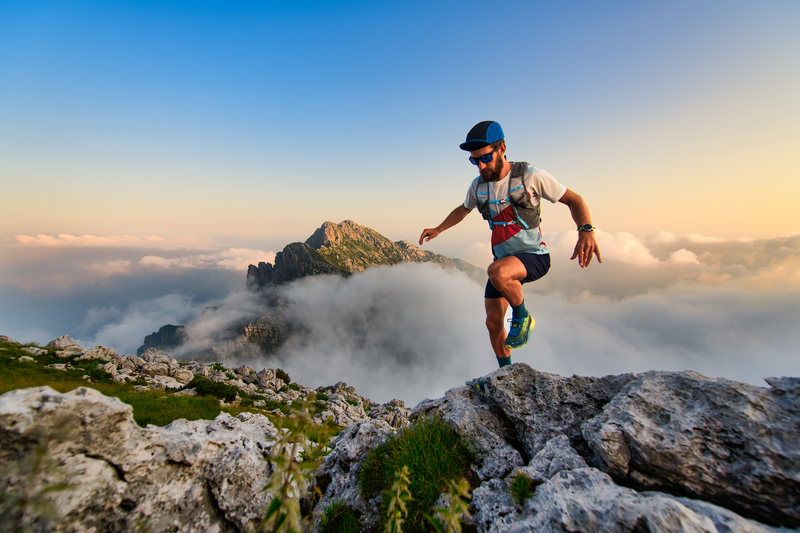
94% of researchers rate our articles as excellent or good
Learn more about the work of our research integrity team to safeguard the quality of each article we publish.
Find out more
ORIGINAL RESEARCH article
Front. Neurol. , 26 July 2022
Sec. Stroke
Volume 13 - 2022 | https://doi.org/10.3389/fneur.2022.894742
This article is part of the Research Topic Sickle Cell Disease View all 14 articles
Chronic anemia is commonly observed in patients with hemoglobinopathies, mainly represented by disorders of altered hemoglobin (Hb) structure (sickle cell disease, SCD) and impaired Hb synthesis (e.g. thalassemia syndromes, non-SCD anemia). Both hemoglobinopathies have been associated with white matter (WM) alterations. Novel structural MRI research in our laboratory demonstrated that WM volume was diffusely lower in deep, watershed areas proportional to anemia severity. Furthermore, diffusion tensor imaging analysis has provided evidence that WM microstructure is disrupted proportionally to Hb level and oxygen saturation. SCD patients have been widely studied and demonstrate lower fractional anisotropy (FA) in the corticospinal tract and cerebellum across the internal capsule and corpus callosum. In the present study, we compared 19 SCD and 15 non-SCD anemia patients with a wide range of Hb values allowing the characterization of the effects of chronic anemia in isolation of sickle Hb. We performed a tensor analysis to quantify FA changes in WM connectivity in chronic anemic patients. We calculated the volumetric mean of FA along the pathway of tracks connecting two regions of interest defined by BrainSuite's BCI-DNI atlas. In general, we found lower FA values in anemic patients; indicating the loss of coherence in the main diffusion direction that potentially indicates WM injury. We saw a positive correlation between FA and hemoglobin in these same regions, suggesting that decreased WM microstructural integrity FA is highly driven by chronic hypoxia. The only connection that did not follow this pattern was the connectivity within the left middle-inferior temporal gyrus. Interestingly, more reductions in FA were observed in non-SCD patients (mainly along with intrahemispheric WM bundles and watershed areas) than the SCD patients (mainly interhemispheric).
Chronic anemia (CA) is a condition in which the number of erythrocytes or hemoglobin (Hb) concentration is lower than expected and incapable of meeting physiological needs (1). Worldwide, the prevalence of anemia is very high, affecting around 1.93 billion people and causing more significant disability than asthma, diabetes, and cardiovascular disease combined (2). Tissue oxygen consumption is heterogeneous and organ-specific. The brain is one of the organs with higher metabolic demand that receives preferential blood flow under acute circumstances (3). As a result, neurons are specifically sensitive to hypoxia (4). CA causes reduced oxygenation in the brain, leading to hypoxia, neuroinflammation, and white matter (WM) remodeling (5).
CA is a standard clinical feature seen in patients with hemoglobinopathies (6), mainly represented by qualitative disorders in Hb structure (e.g., sickle cell disease, SCD) and quantitative disorders of Hb synthesis (e.g., thalassemia syndromes, non-SCD) (7). Hemoglobinopathies have been associated with gray matter (GM) (8–11) and WM alterations (12–17), cerebral vasculopathies (18–20), and changes in cerebral blood flow (21–26), thus serving as a model for the cerebral changes caused by CA (15, 17).
Studying SCD and non-SCD patients with a wide range of Hb values and genetic predisposition to anemia simultaneously allows the characterization of the effects of CA in isolation from sickle Hb (17, 22). In this context, novel structural magnetic resonance imaging (MRI) research done in our laboratory, where SCD and non-SCD patients were simultaneously analyzed, demonstrated that WM volume was diffusely lower in deep, watershed areas proportional to anemia severity regardless of Hb genotype (15). This relationship between anemia and WM volume was confirmed in a repeated analysis with a restricted population consisting of patients with beta-thalassemia (27).
We hypothesized that CA causes similar damaging effects and changes in structural connectivity of WM in patients with hemoglobinopathies. Furthermore, the damage is driven by hyperemia and not by the intrinsic pathophysiology of these hemoglobinopathies. To characterize alterations in the WM, we performed a diffusion tensor imaging (DTI) analysis and quantified the average fractional anisotropy (FA, overall directionality of water diffusion) along the pathways connecting every pair of regions of interest (ROIs) defined by an anatomical atlas. This approach covers all the WM bundles in the brain and not merely the main association fascicles (28).
Diffusion MRI (dMRI) is used to study in-vivo WM microstructure and allows quantitative characterization of healthy and diseased tissue. The most widely used dMRI technique is DTI, despite various limitations. Its derived metrics like FA are potential biomarkers of brain abnormalities in patients with neurodegenerative diseases. For example, DTI analysis already provided evidence of the relationship between WM microstructure and markers of anemia severity, such as oxygen saturation level and Hb value (13). Additionally, SCD patients have shown lower FA in the corticospinal tract and cerebellum (13), across the internal capsule (14), the corpus callosum (14, 16, 29, 30), and in the deep WM (30). Decrement of FA was also observed in major WM tracts in CA patients, regardless of the anemia subtype, and correlated significantly with the neurocognitive decline observed in the CA population (29).
All participants in this study were part of a larger project on Sickle Cell Disease at Children's Hospital Los Angeles that its Institutional Review Board approved. Each participant was recruited with informed consent. We collected MRI data and blood tests on patients, including non-SCD, SCD, and healthy controls matched by sex and age. The accepted age range was between 10 to 50 years old. Eligibility criteria included patients with SCD diagnosis (Hb SS, Hb SC, Hb Sβ0, and Hb Sβ+genotypes), patients with chronic anemia diagnosis (beta-thalassemia major, beta-thalassemia intermedia, hemoglobin H-constant spring, congenital dyserythropoietic anemia, spherocytosis anemia, and autoimmune hemolytic anemia) and healthy controls (mainly recruited from family members of SCD patients to match race and ethnicity between groups). The exclusion criteria disqualified those patients with previous overt stroke, acute chest syndrome, pain crisis hospitalization (within one month), and pregnant candidates. Similarly, individuals with prior history of neurologic insults, developmental delay, or chronic medical conditions that require regular medical care or medications and pregnant candidates would be ineligible as healthy controls.
We followed the standard guidelines and regulations for MRI safety and exclusion criteria. On the same day, each participant completed the MRI examination without sedating medications, and we collected vital signs and blood samples.
To account for the similarities and differences in CA pathophysiology, all participants enrolled in our study underwent a thorough examination of their blood samples. Complete blood count, reticulocyte total, and quantitative Hb electrophoresis percentages of Hb S, Hb A, Hb A2, hemoglobin F, etc.) were analyzed in the clinical laboratory. Additional surrogates for hemolysis like lactate dehydrogenase (LDH) and plasma-free Hb levels were also quantified (Table 1).
The MRI data were acquired on a 3T Philips Achieva scanner using an 8-channel head coil for each participant. The structural 3D T1-weighted (T1-w) sequence specification was TR/TE = 8.3/3.8 ms, SENSE = 2, and isotropic voxel size of 1 mm3. In addition, a single-shell dMRI sequence was acquired with TR/TE = 6,700/86 ms; isotropic voxel size of 2.5 mm3; 30 diffusion-encoding directions at b-value = 1,000 s/mm2 and one b-value = 0 s/mm2 using a single-shot echo-planar imaging sequence.
The Brain extraction and parcellation from the T1-weighted (T1-w) images were processed with BrainSuite (brainsuite.org, v19b). Specifically, BrainSuite's Cortical Surface Extraction (CSE) tool was used to perform skull stripping (31), tissue classification, including partial volume fraction of voxels identified as WM, GM, and CSF (32), topological corrections (33), and delineation of the inner/outer cortex. In addition, BrainSuite's Surface Volume Registration (SVReg) tool (34, 35) performed anatomical co-registration to the BCI-DNI anatomical atlas (35), and brain segmentation.
The dMRI data were corrected for localized geometric distortions to enable accurate multi-modal analysis. Each subject's motion and eddy current-induced distortions were corrected with FSL's eddy module (36, 37). Using BrainSuite's Diffusion Pipeline (BDP), we registered the dMRI to the T1-w data, followed by susceptibility-distortion correction based on the inverse contrast normalization (38).
Using the well-known tensor equation, we calculated the fractional anisotropy maps in BDP. To render more accurate tractography in the WM, we also computed in BDP the orientation density functions (ODFs). In particular, the ensemble average propagator response function optimized (ERFO) uses machine learning and linear estimation theory to optimize ODF accuracy for arbitrary q-space sampling schemes. It has shown advantages over other methods (39). Furthermore, ERFO can model single-shell (and multi-shell) data and has the capacity of rendering crossing fibers with the most negligible false positives (40).
Whole-brain deterministic fiber tracking, based on quantitative anisotropy (41), and visualization were performed with the DSI Studio tractography package (http://dsi-studio.labsolver.org). The sections of tracks entering cortical GM or subcortical regions were excluded to avoid partial volume effects. Afterward, detail connectivity analysis of fiber bundles connecting two ROIs (previously labeled on the T1-w structural images) was implemented with the TractConnect Matlab package (https://neuroimage.usc.edu/neuro/Resources/TractConnect). Specifically, TractConnect uses filtered tracks connecting two ROIs to define a volumetric white matter surface (WMS) and projects it into the FA maps (Figure 1). Then FA values within WMSs were averaged.
Figure 1. FA analysis in WMS based on the coregistration of the parceled T1-w with dMRI maps. This coregistration allows mapping the connectivity tracks between two ROIs to the FA map. The exact process is repeated to create a connectivity matrix for each subject.
For each individual, the average FA values were used as the elements of the connectivity matrix. 88 ROIs from the BCI-DNI atlas were used: 66 cortical regions, 14 subcortical, corpora quadrigemina, mammillary bodies, brainstem, and cerebellum.
Overall, this modeling method offers higher sensitivity and specificity to detect not only regional differences in WM microstructure (like voxel-wise analysis would do) but along the connectivity pathways, and it is robust to some of the commonly criticized featured of DTI ((42, 43)): the inability to render crossing fibers and to define connectivity between ROIs by streamlining counting. The first was overcome by using ERFO to model diffusion ODFs and the latter by defining the WMSs and characterizing these “connections” with the mean FA value.
For each element of the connectivity matrix (upper triangular part), Figure 1, the FA differences between groups were modeled using multiple linear regression analysis after controlling for logarithm of age (log-age), sex, and group. The logarithm of age was used because brain maturational effects are nonlinear with age in adolescents and young adults (44, 45). Finally, the results were also corrected for multiple comparisons using the False Discovery Rate (FDR) to adjust the correspondent p-values (46) with a 20% acceptance rate.
A similar analysis was performed using a permutation analysis using Manly's method (47, 48), which was also FDR corrected (49) with the same threshold. Given the complexity of the data, it was not possible to guarantee all the assumptions of linear modeling. Consequently, we also chose to model the WMSs using nonparametric permutation analysis. Overlapping between the two methods provided an additional confidence level in our results.
For completeness, we tested the possible contribution of changes in FA caused by monthly transfusions and LDH values in patients. For this, we only ran a multiple linear regression analysis controlling also for log-age, sex, and group. All the statistical analyses were calculated using the R statistical package (50).
In this analysis we considered 19 clinically asymptomatic SCD patients (age = 22.4 ± 7.8 years; Hb = 10.1 ± 2.1 g/dL; F = 9 patients), 15 non-SCD anemic patients (age = 22.4 ± 4.8 years; Hb = 10 ± 2.8 g/dL; F = 8 patients) and 23 control subjects (age = 21.3 ± 6 years; Hb = 13.3 ± 1.2 g/dL; F = 14 individuals). The age range for all the participants was 11.2 to 35.8 years. All demographics are reported in Table 1.
The breakdown of the race (and ethnicity) for control subjects was 17 African-American (non-Hispanic) and 5 White (Hispanic) individuals. SCD patients included 17 African-American (non-Hispanic) and 2 White (Hispanic) patients. The non-SCD group consisted of 8 Asian (non-Hispanic), 5 White (non-Hispanic), and 2 White (Hispanic) patients.
For the SCD group, the genotypes were 12 Hb SS and 7 Hb SC patients. Because of the specific matches between control and SCD, 9 of the control subjects were identified with sickle cell trait having hemoglobin AS (Hb AS). Previous work in our laboratory suggests that the Hb AS subtype does not alter normal cerebral blood flow (CBF) regulation and balance of oxygen supply and demand Field (22), making Hb AS carriers good candidates for control subjects.
The specific anemias in the non-SCD group consisted of 7 patients with beta-thalassemia major, 3 beta-thalassemia intermedia, 2 hemoglobin H-constant spring, 1 congenital dyserythropoietic anemia, 1 spherocytosis anemia and 1 autoimmune hemolytic anemia.
Of the CA patients, 8 non-SCD (7 beta-thalassemia major and 1 congenital dyserythropoietic anemia) and 5 SCD (Hb SS patients) were on monthly transfusions. The rest of the non-transfused SS patients were prescribed hydroxyurea and had a mean hemoglobin F fraction of 18%. One patient with SC was also taking hydroxyurea. At Children's Hospital Los Angeles, it is recommended to treat all pediatric patients, of all SCD genotypes, nine months and older with hydroxyurea unless they have been placed on chronic transfusion (51, 52), as indicated by NIH guidelines (53). Furthermore, as of 2,000, all SCD patients at our facility have received access to the transcranial Doppler screening (51–54).
Laboratory and clinical markers are shown in Table 1. Hemoglobin (p = 0.96) and hematocrit (p = 0.10) levels were not statistically different between CA groups, but both had statistically significant lower values compared with healthy control (non-SCD, SCD: p ≤ 0.01). Furthermore, the SCD population showed significantly higher levels of reticulocytes (p ≤ 0.01) and LDH (p ≤ 0.01) compared to both non-SCD and control, suggesting increased intravascular hemolysis. However, plasma-free Hb was not different between CA types (p = 0.99). SCD patients had mildly increased white cell counts with respect to control subjects (p ≤ 0.01) but not relative to the non-SCD anemic patients (p = 0.11). In the case of Hb electrophoresis, Hb S was highest for SCD patients. Still, our control also exhibited a smaller percentage of Hb S because of the inclusion of sickle trait subjects. SCD patients demonstrated the highest hemoglobin F (Hb F) concentration, with intermediate levels observed in non-SCD patients. Most control subjects had no Hb F, but one subject had 11.7% Hb F.
Overall, no statistically significant WMSs were found when comparing SCD with non-SCD patients. 10 WMSs in CTL vs. non-SCD and 5 WMSs in CTL vs. SCD analysis showed significant differences in both multiple linear regression and permutation analysis (Table 2). *FA indicates the group mean FA after controlling for log-age and sex using the multiple linear regression. The point-biserial correlation coefficient, , shows the direction and strength of the relationship between *FA and the status of being anemic or not. A negative value depicts higher *FA in control individuals than CA patients. All WMSs reported in Table 2 showed this behavior except for one (left middle and inferior temporal gyrus) in healthy controls vs. non-SCD comparison. The *FA unpaired two-samples t-test statistic is also displayed for completeness, which agrees with the multiple linear regression analysis.
When Hb was included in the mathematical models as a covariate, all the *FA differences reported in Table 2 were no longer statistically significant. This suggests that many of the effects reported are driven by the Hb differences between healthy controls and CA patients. To further study the relationship between *FA and Hb, we calculated the Pearson correlation coefficient, , and their respective p-value, , for the WMSs reported in Table 2. *FA was significantly correlated with Hb levels in 8 out of the 10 WMSs in the population consisting of control and non-SCD analysis and in 3 out of the 5 WMSs in the control and SCD population. Consequently, by calculating , the proportion of variance in *FA explained by Hb, we observed that in control and non-SCD for the WMSs reported in Table 2, Hb accounts for up to 26% (right thalamus and right amygdala) of the variance in *FA, and up to 21% (left thalamus and left parahippocampal gyrus) in the case of controls with SCD patients.
The spatial locations of the WMSs listed in Table 2 are 3D-rendered in the left and right hemispheres of a representative subject (Figure 2). In these same WMSs, we saw a positive correlation of *FA with Hb. Significant results were bilateral and generally symmetrical across hemispheres. Interestingly, more WMSs survived for the non-SCD (mainly intrahemispheric and along with watershed areas) than for the SCD (mainly interhemispheric) group compared with healthy controls.
Figure 2. 3D-rendering of left and right hemispheres of a representative subject, the white matter surfaces (WMSs) where FA was controlled for age (log transformed), sex and group and it was statistically significant in both statistical models (linear regression and permutation analysis) after FDR correction. The specific regions of interest are listed on Table 2. Top Row: green WMSs, comparison of healthy controls (CTL) with non-sickle cell anemia (non-SCD). Bottom row: red WMSs, comparison of CTL with sickle cell anemia (SCD).
Table 3 shows the results of the multiple linear regression when adding in the model transfusion status. For non-SCD patients, 4 out of 10 WMSs displayed in Table 2 were still statistically significant, and for SCD patients, all the WMSs reported in Table 2 still appeared. When including LDH as a marker of hemolysis in the mathematical model, 6 out of 10 WMSs were statistically significant for non-SCD and 4 of 5 WMSs for SCD patients.
We observed potential microstructural differences along WMSs in both groups of patients with CA with and without SCD compared to controls. These results predominantly showed lower FA values in CA patients, indicating the loss of coherence in the main diffusion direction, which could indicate WM injury. Lower FA was highly associated with decreasing Hb levels revealing that the decreased microstructural integrity found in CA patients is highly driven by chronic hypoxia.
Previous work in CA has shown that the whole brain increases cerebral blood flow (CBF) to compensate for the loss of oxygen-carrying capacity (21, 24, 55–57). This offset in CBF preserves total resting oxygen delivery to the whole brain (21, 22, 55, 58), such that the correspondent oxygen extraction fraction (OEF) from the cerebral cortex seems to be normal or even reduced (26, 59–62). Although resting oxygen delivery is preserved in the cortex, the cerebral vascular reserve is diminished, proportional to the resting hyperemia (23, 25), potentially leaving the brain vulnerable to acute insults such as nighttime hypoxia, acute anemia, and fever. However, while there have been some reports of reduced cortical and subcortical GM volumes (9, 63, 64) in patients not receiving chronic transfusion or hydroxyurea treatment, total GM volume appears preserved (65) in clinically asymptomatic SCD patients (adolescents and young adults) treated with either therapy suggesting that cortical volume loss may not manifest until later in life.
However, regions of deep WM do not seem to have the same metabolic stability during periods of ischemic stress. OEF is increased in the deep watershed areas (56), colocalizing with WM injury patterns (18, 58, 66). Chai et al. (58) showed that independently of disease state, CBF and oxygen delivery to regions of deep WM and border zone regions are considerably smaller than those measured elsewhere within the WM and GM. Furthermore, Wang et al. (30) showed that elevated CBF can be associated with normal-appearing (i.e., infarct-free) WM disruption. Inadequate resting oxygen delivery in the WM is further compounded by blunted cerebrovascular reserve (25).
Thus, chronic hypoperfusion plays a role in the development of the entire WM damage phenotype, including hyperintensities on the T2 FLAIR (17, 18, 58, 66, 67), reduced WM volume (15, 27, 68) or changes in diffusion metrics (12–14, 16, 29, 30).
Based on this consideration, the damage observed in non-SCD patients compared with controls followed an intuitive pattern located primarily in the frontal-parietal WM watershed areas (Table 2). Watershed areas are regions in the brain that sit in-between major cerebral arterial territories and are the most susceptible to hypoxic-ischemic damage when a supply-demand mismatch occurs in the cerebrovascular supply (18, 58, 69).
While most of the affected connections were unilateral, WMSs observed with lower FA in non-SCD patients appeared similarly distributed between the two hemispheres (Figure 2). These results are consistent with the spatial patterns of lower WM volume associated with the severity of anemia diffusely across frontal, parietal lobes, and temporal lobes especially in these watershed areas (15, 68).
Given that most of the WMSs survived when controlling for a hemolysis marker (Table 3), the results are also aligned to a model of global hypoxia that will usually cause diffuse, bilateral brain injury as seen in patients in drowning accidents, cardiac arrest, or bilateral carotid stenosis, in contrast to more localized and asymmetric injury patterns caused by embolic stroke (70). Therefore, we suggest that the injury pattern in WM microstructure of non-SCD patients can indicate global chronic hypoxia driven by anemia's effect on the brain's hemodynamics. A possible explanation is that the vascular architecture providing blood perfusion to WM areas is the long-penetrating medullary arteries with poor collateralization. Consequently, WM is especially vulnerable to hyperintensities development under focal ischemic events or periods of acute stress (71).
For SCD patients and healthy controls, three out of five connections crossed to the contralateral side. Interhemispheric involvement is consistent with previous results from our laboratory showing lower FA in the corpus callosum in CA patients (higher burden on SCD) (29). There are similar observations on SCD in studies performed in Tanzania (16), the United Kingdom (14), and the United States (30). Kawadler et al. (13) also showed associations between microstructural properties in the corpus callosum with daytime oxygen saturation and Hb levels in SCD patients, indicating that hypoxia related at least in part to low hemoglobin in SCD patients drives the WM injury patterns.
Previous DTI studies in SCD patients have also reported widespread FA decrease in the WM (12–14, 30). Surprisingly, we did not observe this extent of systematic FA derangements. This difference possibly reflects the variability in disease expression in our SCD cohort compared to previous reports; 7 subjects had SC genotype, and 5 of the 12 SS patients were receiving chronic transfusions. While SC and chronically transfused patients develop WM hyperintensities, the phenotype of their WM disease is less severe than nontransfused SS and Sβ0 (72), and may even result from different mechanisms. Furthermore, the mean hemoglobin F fractions among the nontransfused SS patients was 18%, suggesting good response to hydroxyurea. Our non-SCD and SCD cohorts were matched for hemoglobin level, so one could reasonably have expected a similar spectrum of disease. Nevertheless, this is a cross-sectional study of young adults, and there is a possibility that exposure to severely reduced arterial oxygen content prior to treatment irreversibly affected brain microstructure during brain development in transfusion-dependent non-SCD patients.
Additionally, Table 3 shows almost no contribution from monthly transfusions or LDH in the WMSs found in SCD patients. Possibly, the distribution of WMS in SCD and non-SCD looks substantially different due to uncontrolled confounders, such as chronic pain reported extensively as a burden for SCD patients (73, 74).
In childhood, SCD patients might have an intermittent pain phenotype. Around 50% of the cases evolve as a chronic pain syndrome in adulthood, with periods of lower and higher pain correlated with the ongoing vaso-occlusion (75). Table 2 shows a significant involvement of the orbito-frontal gyrus, which has been implicated in the modulation of chronic pain (76–78) and pain-related emotions (79). Furthermore, functional imaging studies have shown that regions like the thalamus and the parahippocampal gyrus, also depicted in Table 2, belong to the functional pain network (80, 81). In particular, the thalamus has been identified as a central region that processes pain (82). Anemia, by itself, is a robust biomarker of disease severity in SCD, so it is not surprising that hemoglobin levels correlate with FA in pain circuits.
Several research groups have also shown neurocognitive decline in patients with CA, suggesting a possible and early involvement of the brain even in the absence of overt strokes (83, 84). Many significant WMSs were in the prefrontal cortex (Figure 2), where WM abnormality has been associated with negative effects on neurocognitive function in CA patients (14, 15, 29). Specifically, Chai et al. reported that lower FA in the corpus callosum was associated with lower scores across nine neurocognitive measures. At the same time, Stotesbury et al. (14) found that white matter microstructural properties were associated with processing speed, where FA was the strongest predictor. Additional work in our laboratory has previously demonstrated that lower WM volume predicted low matrix reasoning scores, a measure of executive function, in CA patients and identified changes in resting-state fMRI activity in the orbitofrontal and subcallosal gyri (11). Altogether, microstructural injury patterns indicated in CA patients driven by low Hb levels may have cognitive consequences.
The small sample size limited our study. All participants were part of a larger project on Sickle Cell Disease at Children's Hospital Los Angeles that involved various MRI protocols (75 minutes of total scan time) and was not limited to the dMRI sequence (4 minutes). Our current approach allowed us to include all WM tracks, but it required to have high-quality structural and diffusion data, limiting us to a smaller sample size than described in Chai et al. (29). While significant sex differences have been previously indicated in the study of CA, we could not fully resolve sex disease-specific differences. The use of data from multiple cohorts of CA, whose intrinsic pathophysiology is different, weakens statistical power in the short term but opens the possibility to differentiate and characterize the unique damage induced by individual hemoglobinopathies. In addition, the control subjects were mainly recruited from family members of SCD patients, and they do not necessarily represent the non-SCD population. Consequently, the statistical differences that we found in non-SCD patients (even when controlling for log-age and sex) could be affected by random effects.
Although our contemporaneous hematological investigations are a strength of this study, we did not have previous hemoglobin or any oxygen saturation values. The use of chronic blood transfusion therapy in some of our patients potentially represents a limitation on our findings because no single hemoglobin level characterizes the hypoxic exposure. Furthermore, SCD patients are often placed on chronic transfusions later in life (than non-SCD patients) and their current hemoglobin values do not reflect their lifetime hypoxic exposure. Chronic transfusion is also a complicated therapeutic yielding improvement in erythrocyte deformability and oxygen-carrying capacity but increased viscosity in the microcirculation can potentially worsen the blood flow and oxygen delivery (85). Given our sample size, it would be tough to accurately separate the rheologic and oxygen-carrying capacity effects of red blood cells. Furthermore, the inclusion of transfusion status in the model weakens the statistical power by adding an additional degree of freedom. In addition, we were not able to assess any additional effect of low oxygen saturation on arterial oxygen content and therefore hypoxic exposure.
The constraints associated with using single-shell diffusion images and simple tensor modeling are well documented in the literature, and urge caution to draw firm conclusions from a single tensor metric like FA. This work tried to address some of these limitations by using ERFO ODFs to correctly render crossing fibers and creating WMSs to avoid characterizing connectivity by streamlining counting. However, we recognize that the information provided by FA is limited and that other methods like diffusion kurtosis imaging and neurite orientation dispersion have proven to be more robust to some of the pitfalls of DTI and could provide a more biological explanation of our current observations.
To characterize the effects of CA in white matter, mean FA along the WMSs (surface connecting two ROIs) of chronic anemic patients with sickle and non-sickle anemias were compared with healthy controls. This grouping allowed the isolation of sickle hemoglobin effects in our analysis. Both CA cohorts showed localized FA differences along the WMSs of patients compared with controls but did not show differences between them. However, non-SCD patients manifested bigger systematic FA derangements in the watershed areas that were bilateral and spatially symmetrical. These results suggested that the broad spectrum of variability in disease expression in our sickle cell anemia cohort and uncontrolled confounders of mesostructure integrity affected our ability to detect widespread WM abnormalities as proposed in the literature. Nevertheless, finding interhemispheric WMSs affected in SCD aligns with previous literature reports showing decreased FA in the corpus callosum in CA patients. Recognizing both the differences and the similitudes between CA patients and the affliction that anemia causes in white matter may help develop earlier and more generalized interventions to help overcome the anemia burden.
The datasets presented in this article are not readily available because the complete data set from this trial will be made available upon reasonable request and with an approved data sharing agreement. Requests to access the datasets should be directed to JW, Sldvb2RAY2hsYS51c2MuZWR1.
The studies involving human participants were reviewed and approved by the Institutional Review Board at Children's Hospital Los Angeles in the United States. Written informed consent to participate in this study was provided by the participants' legal guardian/next of kin.
CG-Z, RL, and JW conceived and designed the study. SC, CV, BX, JS, and JW were involved in data acquisition and administrative duties. CG-Z, SC, CV, BX, JS, AJ, RL, and JW contributed to statistical analysis, manuscript writing, and designing figures. AJ, RL, and JW lead the first author's activities and decisions during the research. JW is the last author of this manuscript. All authors contributed to the article and approved the submitted version.
This work was supported by National Heart, Lung, and Blood Institute (Grants 1U01-HL-117718-01, 1R01-HL136484-01A1, and 1F31NS106828). The National Center for Research (5UL1-TR000130-05) through the Clinical Translational Science Institute at Children's Hospital Los Angeles. A Research Career Development Fellowship supports Chau Vu from Saban Research Institute at Children's Hospital Los Angeles. Additionally, Philips Healthcare provided support for protocol development and applications engineering on a support-in-kind basis.
We want to thank Dr. Matthew T. Borzage for the valuable discussions in the analysis of data and the paper's organization. In addition, we thank Noel Arugay, Dr. Adam Bush, and Dr. Xin Miao for their support with MRI data collection and Silvie Suriany and Honglei Liu for the bloodwork analysis. We also acknowledge Mr. Bertin Valdez and Obdulio Carreras for coordinating the patient study visits and Dr. Tom Hofstra, Dr. Jackie Bascom, Susan Carson, Trish Peterson, and Debbie Harris from the CHLA Hematology Division for their assistance with patient recruitment, and Benita Tamrazi, MD, for her help with radiological readings.
Author JW is a Consultant for BluebirdBio, Celgene, Apopharma, WorldcareClinical, and BiomeInformatics.
The remaining authors declare that the research was conducted in the absence of any commercial or financial relationships that could be construed as a potential conflict of interest.
All claims expressed in this article are solely those of the authors and do not necessarily represent those of their affiliated organizations, or those of the publisher, the editors and the reviewers. Any product that may be evaluated in this article, or claim that may be made by its manufacturer, is not guaranteed or endorsed by the publisher.
1. Chaparro CM, Suchdev PS. Anemia epidemiology, pathophysiology, and etiology in low- and middle-income countries. Ann N Y Acad Sci. (2019) 1450:15–31. doi: 10.1111/nyas.14092
2. Kassebaum NJ, GBD Anemia Collaborators. The global burden of anemia. Hematol Oncol Clin North Am. (2016) 30:247–308. doi: 10.1016/j.hoc.2015.11.002
3. Tsui AKY, Marsden PA, Mazer CD, Sled JG, Lee KM, Henkelman RM, et al. Differential HIF and NOS responses to acute anemia: defining organ-specific hemoglobin thresholds for tissue hypoxia. Am J Physiol Regul Integr Comp Physiol. (2014) 307:13–25. doi: 10.1152/ajpregu.00411.2013
4. Watts ME, Pocock R, Claudianos C. Brain energy and oxygen metabolism: emerging role in normal function and disease. Front Mol Neurosci. (2018) 11. doi: 10.3389/fnmol.2018.00216
5. Wolters FJ, Zonneveld HI, Licher S, Cremers LGM, Ikram MK, Koudstaal PJ, et al. Hemoglobin and anemia in relation to dementia risk and accompanying changes on brain MRI. Neurology. (2019) 93:917–26. doi: 10.1212/WNL.0000000000008003
6. Angastiniotis M, Eleftheriou A, Galanello R, Harteveld CL, Petrou M, Traeger-Synodinos J, et al. Chapter 2 - epidemiology of hemoglobinopathies. In: Old J, editor. Prevention of Thalassaemias and Other Haemoglobin Disorders: Volume 1: Principles. Nicosia: Thalassaemia International Federation (2013).
7. Wahed A, Dasgupta A. Chapter 4 - hemoglobinopathies and thalassemias. In: Wahed A, Dasgupta A, editors. Hematology and Coagulation. San Diego: Elsevier (2015). p. 55–80.
8. Metafratzi Z, Argyropoulou MI, Kiortsis DN, Tsampoulas C, Chaliassos N, Efremidis SC. T-2 relaxation rate of basal ganglia and cortex in patients with beta-thalassaemia major. Br J Radiol. (2001) 74:407–10. doi: 10.1259/bjr.74.881.740407
9. Kawadler JM, Clayden JD, Kirkham FJ, Cox TC, Saunders DE, Clark CA. Subcortical and cerebellar volumetric deficits in paediatric sickle cell anaemia. Br J Haematol. (2013) 163:373–6. doi: 10.1111/bjh.12496
10. Case M, Zhang HS, Mundahl J, Datta Y, Nelson S, Gupta K, et al. Characterization of functional brain activity and connectivity using EEG and fMRI in patients with sickle cell disease. Neuroimage Clin. (2017) 14:1–17. doi: 10.1016/j.nicl.2016.12.024
11. Coloigner J, Kim Y, Bush A, Choi S, Balderrama MC, Coates TD, et al. Contrasting resting-state fMRI abnormalities from sickle and non-sickle anemia. PLoS ONE. (2017) 12:e0184860. doi: 10.1371/journal.pone.0184860
12. Sun B, Brown RC, Hayes L, Burns TG, Huamani J, Bearden DJ, et al. White matter damage in asymptomatic patients with sickle cell anemia: screening with diffusion tensor imaging. Am J Neuroradiol. (2012) 33:2043–9. doi: 10.3174/ajnr.A3135
13. Kawadler JM, Kirkham FJ, Clayden JD, Hollocks MJ, Seymour EL, Edey R, et al. White matter damage relates to oxygen saturation in children with sickle cell anemia without silent cerebral infarcts. Stroke. (2015) 46:1793–9. doi: 10.1161/STROKEAHA.115.008721
14. Stotesbury H, Kirkham FJ, Kolbel M, Balfour P, Clayden JD, Sahota S, et al. White matter integrity and processing speed in sickle cell anemia. Neurology. (2018) 90:E2042–50. doi: 10.1212/WNL.0000000000005644
15. Choi S, O'Neil SH, Joshi AA, Li J, Bush AM, Coates TD, et al. Anemia predicts lower white matter volume and cognitive performance in sickle and non-sickle cell anemia syndrome. Am J Hematol. (2019) 94:1055–65. doi: 10.1002/ajh.25570
16. Jacob M, Stotesbury H, Kawadler JM, Lapadaire W, Saunders DE, Sangeda RZ, et al. White matter integrity in tanzanian children with sickle cell anemia a diffusion tensor imaging study. Stroke. (2020) 51:1166–73. doi: 10.1161/STROKEAHA.119.027097
17. Fields ME, Mirro AE, Binkley MM, Guilliams KP, Lewis JB, Fellah S, et al. Cerebral oxygen metabolic stress is increased in children with sickle cell anemia compared to anemic controls. Am J Hematol. (2022) 97:682–90. doi: 10.1002/ajh.26485
18. Guilliams KP, Fields ME, Ragan DK, Chen Y, Eldeniz C, Hulbert ML, et al. Large-vessel vasculopathy in children with Sickle Cell Disease: a magnetic resonance imaging study of infarct topography and focal atrophy. Pediatr Neurol. (2016) 69:49–57. doi: 10.1016/j.pediatrneurol.2016.11.005
19. Usmani A, Machado RF. Vascular complications of sickle cell disease. Clin Hemorheol Microcirc. (2018) 68:205–21. doi: 10.3233/CH-189008
20. Russo AG, Ponticorvo S, Tartaglione I, Caiazza M, Roberti D, Elefante A, et al. No increased cerebrovascular involvement in adult beta-thalassemia by advanced MRI analyses. Blood Cells Mol Dis. (2019) 78:9–13. doi: 10.1016/j.bcmd.2019.05.001
21. Gevers S, Nederveen AJ, Fijnvandraat K, van den Berg SM, van Ooij P, Heijtel DF, et al. Arterial spin labeling measurement of cerebral perfusion in children with sickle cell disease. J Magn Reson Imaging. (2012) 35:779–87. doi: 10.1002/jmri.23505
22. Borzage MT, Bush AM, Choi S, Nederveen AJ, Vaclavu L, Coates TD, et al. Predictors of cerebral blood flow in patients with and without anemia. J Appl Physiol. (2016) 120:976–81. doi: 10.1152/japplphysiol.00994.2015
23. Kosinski PD, Croal PL, Leung J, Williams S, Odame I, Hare GMT, et al. The severity of anaemia depletes cerebrovascular dilatory reserve in children with sickle cell disease: a quantitative magnetic resonance imaging study. Br J Haematol. (2017) 176:280–7. doi: 10.1111/bjh.14424
24. Guilliams KP, Fields ME, Ragan DK, Eldeniz C, Binkley MM, Chen Y, et al. Red cell exchange transfusions lower cerebral blood flow and oxygen extraction fraction in pediatric sickle cell anemia. Blood. (2018) 131:1012–21. doi: 10.1182/blood-2017-06-789842
25. Afzali-Hashemi L, Baas KPA, Schrantee A, Coolen BF, van Osch MJP, Spann SM, et al. Impairment of cerebrovascular hemodynamics in patients with severe and milder forms of sickle cell disease. Front Physiol. (2021) 12:645205. doi: 10.3389/fphys.2021.645205
26. Juttukonda MR, Donahue MJ, Waddle SL, Davis LT, Lee CA, Patel NJ, et al. Reduced oxygen extraction efficiency in sickle cell anemia patients with evidence of cerebral capillary shunting. J Cereb Blood Flow Metab. (2021) 41:546–60. doi: 10.1177/0271678X20913123
27. Choi S, Leahy RM, Wood JC. Lower white matter volume in beta-thalassemia associated with anemia and cognitive performance. Am J Hematol. (2020) 95:E144–6. doi: 10.1002/ajh.25787
28. Mandonnet E, Sarubbo S, Petit L. The nomenclature of human white matter association pathways: proposal for a systematic taxonomic anatomical classification. Front Neuroanat. (2018) 12. doi: 10.3389/fnana.2018.00094
29. Chai Y, Ji CR, Coloigner J, Choi S, Balderrama M, Vu C, et al. Tract-specific analysis and neurocognitive functioning in sickle cell patients without history of overt stroke. Brain Behav. (2021) 11:e01978. doi: 10.1002/brb3.1978
30. Wang Y, Fellah S, Fields ME, Guilliams KP, Binkley MM, Eldeniz C, et al. Cerebral oxygen metabolic stress, microstructural injury, and infarction in adults with sickle cell disease. Neurology. (2021) 97:e902–12. doi: 10.1212/WNL.0000000000012404
31. Sandor S, Leahy R. Surface-based labeling of cortical anatomy using a deformable atlas. IEEE Trans Med Imaging. (1997) 16:41–54. doi: 10.1109/42.552054
32. Shattuck DW, Sandor-Leahy SR, Schaper KA, Rottenberg DA, Leahy RM. Magnetic resonance image tissue classification using a partial volume model. Neuroimage. (2001) 13:856–76. doi: 10.1006/nimg.2000.0730
33. Shattuck DW, Leahy RM. Automated graph-based analysis and correction of cortical volume topology. IEEE Trans Med Imaging. (2001) 20:1167–77. doi: 10.1109/42.963819
34. Joshi AA, Shattuck DW, Thompson PM, Leahy RM. Surface-constrained volumetric brain registration using harmonic mappings. IEEE Trans Med Imaging. (2007) 26:1657–69. doi: 10.1109/TMI.2007.901432
35. Joshi AA, Choi S, Liu Y, Chong M, Sonkar G, Gonzalez-Martinez J, et al. A hybrid high-resolution anatomical MRI atlas with sub-parcellation of cortical gyri using resting fMRI. bioRxiv. (2021) 2020.2009.2012.294322. doi: 10.1101/2020.09.12.294322
36. Andersson JLR, Graham MS, Zsoldos E, Sotiropoulos SN. Incorporating outlier detection and replacement into a non-parametric framework for movement and distortion correction of diffusion MR images. Neuroimage. (2016) 141:556–72. doi: 10.1016/j.neuroimage.2016.06.058
37. Andersson JLR, Sotiropoulos SN. An integrated approach to correction for off-resonance effects and subject movement in diffusion MR imaging. Neuroimage. (2016) 125:1063–78. doi: 10.1016/j.neuroimage.2015.10.019
38. Bhushan C, Haldar JP, Choi S, Joshi AA, Shattuck DW, Leahy RM. Co-registration and distortion correction of diffusion and anatomical images based on inverse contrast normalization. Neuroimage. (2015) 115:269–80. doi: 10.1016/j.neuroimage.2015.03.050
39. Varadarajan D, Haldar JP. A theoretical signal processing framework for linear diffusion MRI: Implications for parameter estimation and experiment design. Neuroimage. (2017) 161:206–18. doi: 10.1016/j.neuroimage.2017.08.048
40. Varadarajan D, Haldar JP. Towards optimal linear estimation of orientation distribution functions with arbitrarily sampled diffusion MRI data. 2018 IEEE 15th International Symposium on Biomedical Imaging (Isbi 2018), Washington, DC (2018). p. 743–6.
41. Yeh FC, Verstynen TD, Wang YB, Fernandez-Miranda JC, Tseng WYI. Deterministic diffusion fiber tracking improved by quantitative anisotropy. PLoS ONE. (2013) 8:e80713. doi: 10.1371/journal.pone.0080713
42. Jones DK, Knosche TR, Turner R. White matter integrity, fiber count, and other fallacies: The do's and don'ts of diffusion MRI. Neuroimage (2013) 73:239–54. doi: 10.1016/j.neuroimage.2012.06.081
43. Soares JM, Marques P, Alves V, Sousa N. A hitchhiker's guide to diffusion tensor imaging. Front Neurosci. (2013) 7:31. doi: 10.3389/fnins.2013.00031
44. Lebel C, Beaulieu C. Longitudinal development of human brain wiring continues from childhood into adulthood. J Neurosci. (2011) 31:10937–947. doi: 10.1523/jneurosci.5302-10.2011
45. Chang YS, Owen JP, Pojman NJ, Thieu T, Bukshpun P, Wakahiro MLJ, et al. White matter changes of neurite density and fiber orientation dispersion during human brain maturation. PLoS ONE (2015) 10:e0123656. doi: 10.1371/journal.pone.0123656
46. Yekutieli D, Benjamini Y. Resampling-based false discovery rate controlling multiple test procedures for correlated test statistics. J Stat Plan Inference. (1999) 82:171–96. doi: 10.1016/S0378-3758(99)00041-5
47. Manly BFJ. Randomization and regression methods for testing for associations with geographical, environmental and biological distances between populations. Res Popul Ecol. (1986) 28:201–18. doi: 10.1007/BF02515450
48. Manly BFJ. Randomization, Bootstrap and Monte Carlo Methods in Biology. New York, NY: Chapman and Hall (2007).
49. Winkler AM, Ridgway GR, Webster MA, Smith SM, Nichols TE. Permutation inference for the general linear model. Neuroimage. (2014) 92:381–97. doi: 10.1016/j.neuroimage.2014.01.060
50. R Core Team. R: A Language and Environment for Statistical Computing. Vienna: R Foundation for Statistical Computing (2020).
51. Steinberg MH. Management of sickle cell disease. N Eng J Med. (1999) 340:1021–30. doi: 10.1056/NEJM199904013401307
52. Kavanagh PL, Sprinz PG, Vinci SR, Bauchner H, Wang CJ. Management of children with sickle cell disease: a comprehensive review of the literature. Pediatrics. (2011) 128:e1552–74. doi: 10.1542/peds.2010-3686
53. Yawn BP, Buchanan GR, Afenyi-Annan AN, Ballas SK, Hassell KL, James AH, et al. Management of sickle cell disease: summary of the 2014 evidence-based report by expert panel members. JAMA. (2014) 312:1033–48. doi: 10.1001/jama.2014.10517
54. Enninful-Eghan H, Moore RH, Ichord R, Smith-Whitley K, Kwiatkowski JL. Transcranial doppler ultrasonography and prophylactic transfusion program is effective in preventing overt stroke in children with Sickle cell disease. J Pediatr. (2010) 157:479–84. doi: 10.1016/j.jpeds.2010.03.007
55. Bush AM, Borzage MT, Choi S, Vaclavu L, Tamrazi B, Nederveen AJ, et al. Determinants of resting cerebral blood flow in sickle cell disease. Am J Hematol. (2016) 91:912–7. doi: 10.1002/ajh.24441
56. Fields ME, Guilliams KP, Ragan DK, Binkley MM, Eldeniz C, Chen Y, et al. Regional oxygen extraction predicts border zone vulnerability to stroke in sickle cell disease. Neurology. (2018) 90:e1134–44. doi: 10.1212/WNL.0000000000005194
57. Vu C, Bush A, Choi S, Borzage M, Miao X, Nederveen AJ, et al. Reduced global cerebral oxygen metabolic rate in sickle cell disease and chronic anemias. Am J Hematol. (2021) 96:901–13. doi: 10.1002/ajh.26203
58. Chai Y, Bush AM, Coloigner J, Nederveen AJ, Tamrazi B, Vu C, et al. White matter has impaired resting oxygen delivery in sickle cell patients. Am J Hematol. (2019) 94:467–74. doi: 10.1002/ajh.25423
59. Bush AM, Coates TD, Wood JC. Diminished cerebral oxygen extraction and metabolic rate in sickle cell disease using T2 relaxation under spin tagging MRI. Magn Reson Med. (2018) 80:294–303. doi: 10.1002/mrm.27015
60. Croal PL, Leung J, Phillips CL, Serafin MG, Kassner A. Quantification of pathophysiological alterations in venous oxygen saturation: a comparison of global MR susceptometry techniques. Magn Reson Imaging. (2019) 58:18–23. doi: 10.1016/j.mri.2019.01.008
61. Juttukonda MR, Donahue MJ, Davis LT, Gindville MC, Lee CA, Patel NJ, et al. Preliminary evidence for cerebral capillary shunting in adults with sickle cell anemia. J Cereb Blood Flow Metab. (2019) 39:1099–110. doi: 10.1177/0271678X17746808
62. Li W, Xu X, Liu P, Strouse JJ, Casella JF, Lu H, et al. Quantification of whole-brain oxygenation extraction fraction and cerebral metabolic rate of oxygen consumption in adults with sickle cell anemia using individual T2-based oxygenation calibrations. Magn Reson Med. (2020) 83:1066–80. doi: 10.1002/mrm.27972
63. Mackin RS, Insel P, Truran D, Vichinsky EP, Neumayr LD, Armstrong FD, et al. Neuroimaging abnormalities in adults with sickle cell anemia: associations with cognition. Neurology. (2014) 82:835–41. doi: 10.1212/WNL.0000000000000188
64. Kim JA, Leung J, Lerch JP, Kassner A. Reduced cerebrovascular reserve is regionally associated with cortical thickness reductions in children with sickle cell disease. Brain Res. (2016) 1642:263–9. doi: 10.1016/j.brainres.2016.03.041
65. Choi S, Bush AM, Borzage MT, Joshi AA, Mack WJ, Coates TD, et al. Hemoglobin and mean platelet volume predicts diffuse T1-MRI white matter volume decrease in sickle cell disease patients. Neuroimage Clin. (2017) 15:239–46. doi: 10.1016/j.nicl.2017.04.023
66. Ford AL, Ragan DK, Fellah S, Binkley MM, Fields ME, Guilliams KP, et al. Silent infarcts in sickle cell disease occur in the border zone region and are associated with low cerebral blood flow. Blood. (2018) 132:1714–23. doi: 10.1182/blood-2018-04-841247
67. Musallam KM, Taher AT, Karimi M, Rachmilewitz EA. Cerebral infarction in β-thalassemia intermedia: breaking the silence. Thromb Res. (2012) 130:695–702. doi: 10.1016/j.thromres.2012.07.013
68. Baldeweg T, Hogan AM, Saunders DE, Telfer P, Gadian DG, Vargha-Khadem F, et al. Detecting white matter injury in sickle cell disease using voxel-based morphometry. Ann Neurol. (2006) 59:662–72. doi: 10.1002/ana.20790
69. Ropper AH, Klein J, Samuels MA, Prasad S. Adams and Victor's Principles of Neurology. New York, N.Y: McGraw-Hill Education LLC (2019).
71. Sam K, Conklin J, Holmes KR, Sobczyk O, Poublanc J, Crawley AP, et al. Impaired dynamic cerebrovascular response to hypercapnia predicts development of white matter hyperintensities. Neuroimage Clin. (2016) 11:796–801. doi: 10.1016/j.nicl.2016.05.008
72. Quinn CT. Minireview: clinical severity in sickle cell disease: the challenges of definition and prognostication. Exp Biol Med. (2016) 241:679–88. doi: 10.1177/1535370216640385
73. Novelli EMMD, Gladwin MTMD. Crises in sickle cell disease. Chest. (2016) 149:1082–93. doi: 10.1016/j.chest.2015.12.016
74. Darbari DS, Sheehan VA, Ballas SK. The vaso-occlusive pain crisis in sickle cell disease: definition, pathophysiology, and management. Eur J Haematol. (2020) 105:237–46. doi: 10.1111/ejh.13430
75. Field JJ. Five lessons learned about long-term pain management in adults with sickle cell disease. Hematol Am Soc Hematol Edu Prog. (2017) 2017:406–11. doi: 10.1182/asheducation-2017.1.406
76. Dang YH, Zhao Y, Xing B, Zhao XJ, Huo FQ, Tang JS, et al. The role of dopamine receptors in ventrolateral orbital cortex-evoked anti-nociception in a rat model of neuropathic pain. Neuroscience. (2010) 169:1872–80. doi: 10.1016/j.neuroscience.2010.06.050
77. Vachon-Presseau E, Tétreault P, Petre B, Huang L, Berger SE, Torbey S, et al. Corticolimbic anatomical characteristics predetermine risk for chronic pain. Brain. (2016) 139:1958–70. doi: 10.1093/brain/aww100
78. Ong W-Y, Stohler CS, Herr DR. Role of the prefrontal cortex in pain processing. Mol Neurobiol. (2019) 56:1137–66. doi: 10.1007/s12035-018-1130-9
79. Sheng H-Y, Lv S-S, Cai Y-Q, Shi W, Lin W, Liu T-T, et al. Activation of ventrolateral orbital cortex improves mouse neuropathic pain–induced anxiodepression. JCI Insight. (2020) 5:e133625. doi: 10.1172/jci.insight.133625
80. Smallwood RF, Laird AR, Ramage AE, Parkinson AL, Lewis J, Clauw DJ, et al. Structural brain anomalies and chronic pain: a quantitative meta-analysis of gray matter volume. J Pain. (2013) 14:663–75. doi: 10.1016/j.jpain.2013.03.001
81. Zhang B, Jung M, Tu Y, Gollub R, Lang C, Ortiz A, et al. Identifying brain regions associated with the neuropathology of chronic low back pain: a resting-state amplitude of low-frequency fluctuation study. Br J Anaesth. (2019) 123:e303–11. doi: 10.1016/j.bja.2019.02.021
82. Schweinhardt P, Bushnell MC. Pain imaging in health and disease - how far have we come? J Clin Invest. (2010) 120:3788–97. doi: 10.1172/JCI43498
83. Berkelhammer LD, Williamson AL, Sanford SD, Dirksen CL, Sharp WG, Margulies AS, et al. Neurocognitive sequelae of pediatric sickle cell disease: a review of the literature. Child Neuropsychol. (2007) 13:120–31. doi: 10.1080/09297040600800956
84. Elalfy MS, Aly RH, Azzam H, Aboelftouh K, Shatla RH, Tarif M, et al. Neurocognitive dysfunction in children with beta thalassemia major: psychometric, neurophysiologic and radiologic evaluation. Hematology. (2017) 22:617–22. doi: 10.1080/10245332.2017.1338212
Keywords: chronic anemia, diffusion MRI, tensor analysis, sickle cell disease (SCD), thalassemia, white matter (WM)
Citation: González-Zacarías C, Choi S, Vu C, Xu B, Shen J, Joshi AA, Leahy RM and Wood JC (2022) Chronic anemia: The effects on the connectivity of white matter. Front. Neurol. 13:894742. doi: 10.3389/fneur.2022.894742
Received: 12 March 2022; Accepted: 29 June 2022;
Published: 26 July 2022.
Edited by:
Fenella Jane Kirkham, University College London, United KingdomReviewed by:
Tales Santini, Western University, CanadaCopyright © 2022 González-Zacarías, Choi, Vu, Xu, Shen, Joshi, Leahy and Wood. This is an open-access article distributed under the terms of the Creative Commons Attribution License (CC BY). The use, distribution or reproduction in other forums is permitted, provided the original author(s) and the copyright owner(s) are credited and that the original publication in this journal is cited, in accordance with accepted academic practice. No use, distribution or reproduction is permitted which does not comply with these terms.
*Correspondence: John C. Wood, andvb2RAY2hsYS51c2MuZWR1
Disclaimer: All claims expressed in this article are solely those of the authors and do not necessarily represent those of their affiliated organizations, or those of the publisher, the editors and the reviewers. Any product that may be evaluated in this article or claim that may be made by its manufacturer is not guaranteed or endorsed by the publisher.
Research integrity at Frontiers
Learn more about the work of our research integrity team to safeguard the quality of each article we publish.