- 1Division of Oncologic Imaging, University of Alberta, Edmonton, AB, Canada
- 2Neuroscience and Mental Health Institute, University of Alberta, Edmonton, AB, Canada
- 3Faculty of Medicine, University Bern, Bern, Switzerland
- 4Department of Neurology, University of Alberta, Edmonton, AB, Canada
- 5Division of Neuroscience, San Raffaele Scientific Institute, University Vita Salute San Raffaele, Milan, Italy
- 6Medical Isotope and Cyclotron Facility, University of Alberta, Edmonton, AB, Canada
- 7Lady Davis Institute for Medical Research, Department of Neurology and Neurosurgery, McGill University, Montreal, QC, Canada
- 8Department of Nuclear Medicine, University of Ulm Medical Center, Ulm, Germany
- 9Department of Diagnostic and Interventional Radiology, University of Ulm Medical Center, Ulm, Germany
- 10Department of Neurology, Ulm University Medical Center, Ulm, Germany
Neuroimaging assessment of motor neuron disease has turned into a cornerstone of its clinical workup. Amyotrophic lateral sclerosis (ALS), as a paradigmatic motor neuron disease, has been extensively studied by advanced neuroimaging methods, including molecular imaging by MRI and PET, furthering finer and more specific details of the cascade of ALS neurodegeneration and symptoms, facilitated by multicentric studies implementing novel methodologies. With an increase in multimodal neuroimaging data on ALS and an exponential improvement in neuroimaging technology, the need for harmonization of protocols and integration of their respective findings into a consistent model becomes mandatory. Integration of multimodal data into a model of a continuing cascade of functional loss also calls for the best attempt to correlate the different molecular imaging measurements as performed at the shortest inter-modality time intervals possible. As outlined in this perspective article, simultaneous PET/MRI, nowadays available at many neuroimaging research sites, offers the perspective of a one-stop shop for reproducible imaging biomarkers on neuronal damage and has the potential to become the new gold standard for characterizing motor neuron disease from the clinico-radiological and neuroscientific perspectives.
Introduction
Based on current guidelines, clinical workup of amyotrophic lateral sclerosis (ALS) will include neuroimaging to rule out structural lesions and neurologic conditions that sometimes account for early clinical features seen in patients suspected of having primary motor neuron disease (1). The clinical management of this highly invalidating condition, however, clearly necessitates, as early as possible, accurate diagnostic and prognostic information on the associated motor neuron degeneration to direct appropriate clinical handling of the individual patient. Neuroimaging has proven to provide reliable in vivo biomarkers to better define the various clinical entities within ALS and to provide additional complementary information to the standard clinical workup (2).
Current situation of neuroimaging for motor neuron disease
Instrumentation and image processing—MRI
Recent MRI applications for motor neuron disease including ALS have focused both on quantitative and qualitative analysis of structural changes in T1-weighted images as assessed by automatic analysis approaches and, in a body of more recent studies, on the analysis of microstructural alterations within the brain and spinal cord by the application of varieties of diffusion-weighted MRI sequences.
Additionally, substantial efforts have been made utilizing PET to generate newer and more biologically based classifications of ALS and its subtypes (3–5). PET, as a non-invasive in vivo imaging technique, provides quantitative data at the molecular level, with novel radiotracers targeting neurons, microglia and astrocytes metabolism, receptor and protein density, as well as oxidative stress.
Advances in computational analyses of multimodal imaging datasets, including deep learning-based applications of artificial intelligence (AI), are just opening the door for a more comprehensive understanding of the pathophysiological cascade of neurodegeneration in motor neuron disease. Here, hypothesis-guided approaches including neuropathological concepts and network-based analyses will be center stage and will eventually find their way into clinical practice.
Crucial information will also be derived from neuroimaging fingerprinting of genetically defined ALS phenotypes like the association with C9orf72 hexanucleotide repeat expansions, especially in longitudinal investigations of presymptomatic mutation carriers. Neuroimaging offers the possibility to stratify ALS patients according to their intrinsic progression rate, based on cross-sectional and longitudinal studies, thus helping to optimize disease management, enhancing the design of drug trials, and guiding the use of novel individualized treatments when these become available (6, 7).
Further advances in the clinical application of neuroimaging in motor neuron disease will have to rely extensively on a new stage of neuroscientific cooperation, building on existing collaborations between researchers and infrastructures specialized in ALS and facilitating multicenter joint projects that enable grand-scale projects, such as those, for example, led by the Neuroimaging Society in Amyotrophic Lateral Sclerosis (8) or the Canadian ALS Neuroimaging Consortium (CALSNIC) (9). While multicentric studies are most welcome to increase the number of observations in subgroups and different disease stages, the accordingly increasing amount of data collected is paralleled by the need for harmonization of protocols, being the foundation for deducting evidence out of data, and integration of their respective findings into a consistent model of a possible continuing cascade of functional loss.
Integration of multimodal data into a consistent model aiming to correlate the different anatomical, functional, and molecular imaging measurements also calls for the best attempt to perform all measurements to correlate at the shortest intermodality time intervals possible, which is most important in a rapidly progressing disease. But even in the case of a primarily slowly progressing disease, pathological processes can be expected to be accentuated at times, as typically is the case for inflammatory cascades. Any attempt to draw conclusions from observations even a few days apart may thus be jeopardized by the underlying pathological process itself.
The advent of simultaneous PET/MRI, which industries had quickly turned from prototype research instruments into reliable, integrated commercial scanners, has proven to provide a stable, reproducible, and calibrated hybrid modality that not only by design acquires data simultaneously, but also adds value by its potential of dynamically mutually informing their reconstruction algorithms with either modality data. With PET/MRI available at many neuroimaging research sites, the perspective of a one-stop shop for reproducible imaging biomarkers on neuronal damage gains importance, and the call for harmonization of protocols becomes feasible, as the stringent design of hybrid PET/MRI eases the implementation of research protocols and research MR sequences at the existent sites.
The two available PET/MRI scanner models built by the industry providers GE Medical and Siemens Healthineers make use of their respective provider's 3T MRI platform and state-of-the-art PET technology, enabling rapid adaption of advances in image acquisition and reconstruction to their clinical platforms. Improved MR sequences, e.g., providing diffusion imaging at a resolution of 1 × 1 × 1 mm3, necessary to characterize microstructural abnormalities (10), can thus be easily adopted and distributed throughout the neuroimaging research sites. Harmonization of MR protocols across different vendors also has proven feasible for the specific needs to characterize microstructural changes in corticospinal, corticorubral, corticostriatal, and hippocampal tracts at different stages of disease progression in ALS (8). The same holds true for protocols for resting state fMRI (11) and MR spectroscopy (MRS) (12).
While PET measurements, in principle, allow for absolute quantitation measures, such as regional cerebral blood volume, regional cerebral glucose metabolism, or regional receptor occupancy, the evaluation of multicentric data makes procedures for harmonization of data acquisition and processing mandatory (13–16), which is simplified for PET/MRI, where, by design, variability of the underlying technology is limited. Given that technical prerequisites have been met, quantitative measures of different systems relevant to motor neuron disease can be non-invasively acquired and PET/MRI proves useful for individual assessment of the stage of disease (Figure 1).
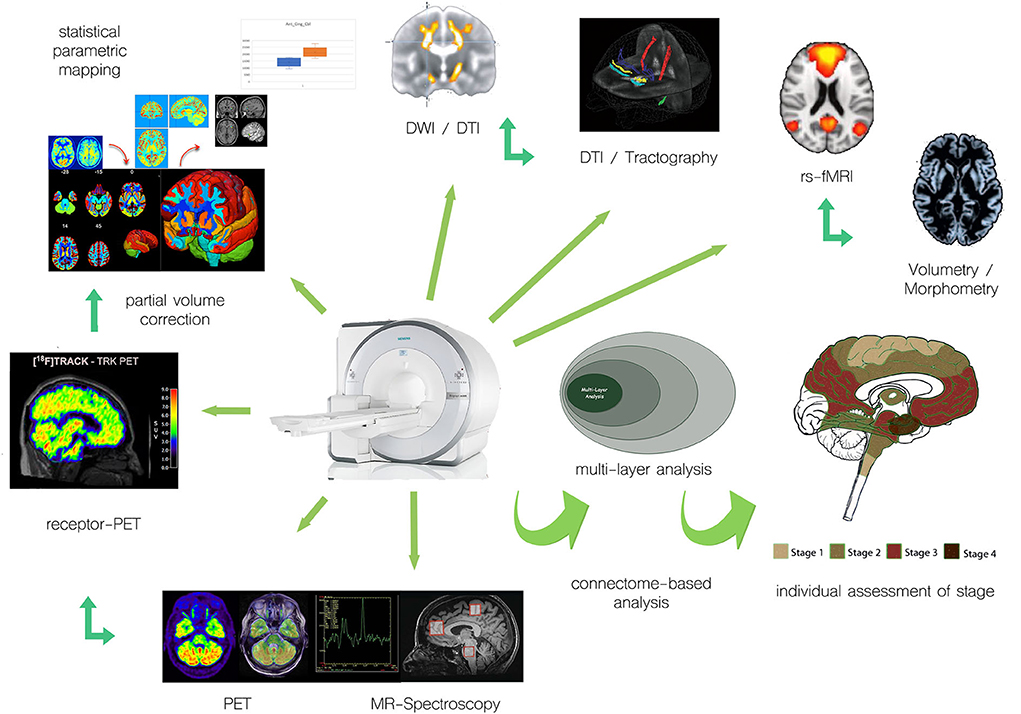
Figure 1. Examples of integration of multimodal PET and MR approaches for individual assessment of motor neuron disease/ALS.
Molecular imaging of disease-inherent pathological alterations—PET
Glucose metabolism and regional inflammatory changes
While many of the more recent radiotracers are still experimental, measures of regional glucose metabolism using 18F-FDG-PET have reached clinical utility in ALS, as evaluated by a panel of experts in the field of nuclear medicine and neurology. By an analysis of the most relevant 18F-FDG-PET investigations by the Population, Intervention, Comparison, Outcome (PICO) model, the provided incremental value as compared with the information resulting from the clinical tests routinely performed had been assessed, concluding that 18F-FDG-PET offers good evidence to support ALS diagnosis (17). This analysis, however, was based on stand-alone 18F-FDG-PET investigations, which did not take into account same-time structural or functional MRI measures. The metabolic patterns identified in ALS consisted of significant hypometabolism in prefrontal, frontal, precentral, and postcentral regions, bilaterally, associated with significant hypermetabolism in posterior occipital and middle temporal cortices, cerebellum, midbrain, and corticospinal tracts, findings which are atypical in functional studies investigating neurodegenerative diseases. Clinically relevant is that the extent of metabolic brain changes in frontal lobes is correlated with cognitive dysfunction (18), thus distinguishing patients with cognitive impairment and possible overlap with frontotemporal dementia from those with pure motor disease (19). The pattern of glucose metabolism also was found to discriminate ALS patients from patients with Parkinson's plus syndromes (20), and, if using machine learning-based techniques, such as support-vector machine discriminant analysis, FDG PET also has proven to be useful for automatically classifying patients with amyotrophic lateral sclerosis vs. controls (21).
More recent studies have found an inverse correlation between precentral and postcentral metabolic activity and clinical stages (22), as well as an inverse correlation between prefrontal and limbic metabolism and apathy (23). When coregistered MRI for partial volume correction was used, FDG PET was able to identify metabolic changes in presymptomatic carriers of the C9orf72 repeat expansion (24). Also, precentral metabolism distinguished patients with the SOD1 mutations (SOD-1 ALS) from sporadic ALS (sALS). Specifically, right precentral and paracentral metabolism was relatively increased in patients with SOD-1 ALS as compared to sALS (25). It was hypothesized that a relative increase of 18F-FDG-related signal in ALS patients in pyramidal cells in the motor cortex and in their projections to the spinal cord is secondary to a widespread microglial activation and astrocytosis reactive to the reduced neuronal density, with the proliferation of astrocytes being the main determinant of glucose uptake from the intraparenchymal capillaries (26). To better delineate the spatial pattern of metabolic changes in the brain stem and cervical spinal cord, an innovative study capitalized on the potential of integrated PET/MRI to improve result accuracy in small anatomic structures by separately analyzing glucose metabolic patterns in the midbrain/pons, medulla oblongata and cervical spinal cord of ALS and frontotemporal dementia (FTD) patients as compared to normal controls (27). They found a significant and intercorrelating increment in glucose metabolism in the midbrain/pons and medulla oblongata in ALS/FTD patients (spinal-ALS and FTD-motor neuron disease subgroups), interpreted to relate to neuroinflammation, namely activated microglia. While they did not report relevant associations between clinical and metabolic features, medulla oblongata hypermetabolism was associated with shortened survival of ALS patients. In the context of their study, the simultaneously available MRI was instrumental for the identification of corticospinal tract hyperintensities to differentiate ALS from clinically overlapping FTD patients of the motor neuron subgroup, and for the detection of the brain stem and cervical spinal cord hypermetabolism in favor of regional neuro-inflammation linked to activated microglia. Confirmatory studies, directly measuring microglial activation using radioligands targeting TSPO (18 kDa translocator protein), have been performed either using the first-generation TSPO ligand 11C-PK-11195 (28, 29) or one of the second-generation ligands 18F-FEPPA (30), 18F-DPA714 (31), or 11C-PBR28, the latter of which has proven to allow for microglia imaging of fiber tracts (32). Technical issues that have not been addressed by a “PET-only” approach for those investigations include the need for partial volume correction, as the measured signal would depend on the regional volume of the anatomical structure of interest. Partial volume correction, however, is extensively dependent on coincident, morphological imaging, where the quality of coregistration of sequentially acquired datasets is a major determinant of bias. It can therefore be expected that using combined information out of a single coordinate system of acquisition, TSPO-PET/MRI will represent an even more useful biomarker in cross-sectionally and longitudinally evaluating the spread of inflammatory lesions.
Novel markers for neuroinflammation
Purinergic P2X7 ionotropic receptor
More recently, novel markers for neuroinflammation targeting the purinergic P2X7 ionotropic receptor (P2X7R) have been successfully introduced into human PET-imaging [(33) brain kinetic modeling and quantification of brain P2X7 receptors in patients with Parkinson's disease and healthy volunteers] (34). As P2X7R is expressed in astrocytes, microglia, and oligodendrocytes, where they mediate inflammasome signaling (35). A couple of preclinical and clinical studies suggest the implication of P2X7Rs in ALS pathogenesis (36–38). As a whole group of P2X7-Receptor antagonists is currently under development, posing a possible new therapeutic approach for ALS (39). A quantitative assessment of P2X7R as an initial and longitudinal biomarker, along with morphological and functional MR measures will be a basic necessity in according clinical studies, naturally favoring PET/MRI as an imaging modality of choice.
C-X-C motif chemokine receptor antagonists
Another promising candidate target suitable as a biomarker for neuroinflammation is the cyclooxygenase-enzyme-2 (COX-2), the possible involvement of which in ALS is indicated by preclinical data (36) and clearly needs systematic in vivo studies that will be enabled by the very recent development of appropriate radiopharmaceutical targets (40–42). If a significant involvement of COX-2 could be documented at any stage of disease, this could open the rationale for stage-dependent antioxidative treatment (43, 44), and would again need to be reflected in MRI-based functional measures.
More experimental measures for neuroinflammation are based on findings indicating the implication of C-X-C motif chemokine receptor (CXCR)-4 and CXCR-3, which critically contribute to the disease process in systemic inflammation (45–47), opening another door to possible future therapeutic principles targeting the respective C-X-C motif chemokine receptor (48, 49). As a couple of specific radiopharmaceutical targets have been developed for preclinical and clinical use (40–42, 50), the selection of an appropriate tracer as a biomarker in motor neuron disease would depend on binding characteristics, kinetic analysis, and correlation with functional measures derived from MRI. Again, interpretation of novel preclinical and clinical neuropharmacological imaging data will crucially depend on its supportive correlation with coincident imaging findings using a different modality.
Neurotrophins
Tyrosine kinase receptor antagonists
There is growing evidence for neurotrophins being involved in neurodegenerative diseases including ALS (40–42, 50, 51). While neurotrophins have not yet proven a significant therapeutic potential in clinical trials, partly because of the difficulties of protein delivery and pharmacokinetics in the nervous system, the binding target of neurotrophins includes a family of tyrosine kinase (TrK) receptors. Within this class of receptors, synthetic antibodies have recently been linked to PET-radioligands targeting the TrK-B receptor, which have passed the preclinical and clinical assessments (52–54). Quantitative characterization of TrK-B alterations in ALS is currently underway by the same research group, accounting for the volumetric changes inherent in the disease by applying PET/MRI in the first place, complemented by magnetic resonance spectroscopy data. The recent development of TrkB agonistic antibodies and BDNF-targeted gene therapies (55, 56) could prove useful, and changes in TrK-B alterations as measured by PET/MRI during targeted therapy could potentially qualify as an imaging endpoint in clinical trials in motor neuron disease (52–54, 57).
Markers of neuronal integrity
GABA-A—Benzodiazepine receptor complex
The selective PET ligands 11C-Flumazenil and 2′-18F-Fluoroflumazenil, binding to the GABA-A—receptor, widely expressed on pyramidal neurons, have been suggested to be used as a surrogate in vivo marker of neuronal density (58–62). First, applications in neurodegenerative disease have shown a regional neuronal loss in the motor and premotor cortex as well as in extramotor areas in ALS (63, 64), which were also associated with specific cognitive deficits (65, 66). The specificity of GABA-A receptor antagonists to characterize neuronal loss without a possible confound by GABAergic dysfunction has however been questioned, and quantitation methods applied have been scrutinized (67). It has been demonstrated that PET imaging and quantitation using Flumazenil-based radioligands highly depend on the availability of concurrent high-resolution MRI (68), which would ideally be conceptualized by PET/MRI.
Antagonists to synaptic vesicle protein 2A
The synaptic vesicle protein 2A (SV2A), a 12-transmembrane domain glycoprotein, is ubiquitously expressed in normal synaptic vesicles throughout the brain, with a particularly high regional expression in the thalamus and basal ganglia (69), only sparing the trigeminal and facial nerve nuclei (70, 71). SV2A has thus been claimed as a potential biomarker for synaptic vesicle density. While it is critical to Ca2+-dependent exocytosis (72), its exact physiological role still is subject to further research, and deficiencies in the expression of SV2A have been described in a growing number of neurodegenerative disorders, including frontotemporal dementia (FTD), Parkinson's Disease (PD), Alzheimer's disease (AD), corticobasal degeneration (73–77), as well as further neurological conditions, such as epilepsy (78–80), where it has been identified as the binding site for the antiepileptic drug levetiracetam (81).
In analyses of rat brain homogenates, the number of expressed SV2a proteins per vesicle was found to be highly reproducible at 2–5 copies per vesicle (82, 83). It has been suggested that SV2A plays a central role in exocytosis mediated by Ca2+ (71). The deficiency of SV2A in SV2A knockout mice resulted in presynaptic Ca2+ accumulation, destabilizing synaptic circuits, and inducing seizures (84). It has also been suggested that SV2A is modulating endocytosis to the SV of the SV protein synaptotagmin-1 (SYT1), and by this mechanism is involved in the homeostasis of the readily releasable pool of SVs (85).
SV2A has thus attracted attention as a target binding site for PET-tracers, and subsequently, with the SV2A antagonist levetiracetam serving as a blueprint, a number of suitable ligands have been developed and translated into human studies (86, 87). The binding of the PET ligand 11C-UCB-J to SV2A has been demonstrated to quantitatively correlate and co-localize with synaptophysin (SYN), a key protein located in the pre- or postsynaptic neurons, using a combined in vivo–in vitro validation, furthering evidence for SV2A targeting PET tracers to serve as synaptic density marker, which allows for the quantification of synaptic density in vivo (88).
Recently, a second-generation SV2A antagonist 18F-SynVesT-1 (SDM-8) (89) has been introduced with superior SV2A binding affinity, improved imaging properties, enhanced metabolic stability, and an easier path for radiochemical synthesis (90, 91). For SV2A-markers to prove useful for longitudinal studies of neuronal density in progressive neurodegenerative diseases, such as ALS, confounding effects due to atrophy have to be accounted for, and simultaneous PET/MRI delivers the most accurate, concurrent quantitative anatomic information to perform partial volume correction reflecting the state of morphologic changes at the exact same time point of PET data acquisition.
Further understanding of ALS pathophysiology will be achieved by the study of multimodal MRI and PET data through network-based analyses with hypothesis-guided approaches, including neuropathological concepts, although advanced neuroimaging still awaits translation into clinical settings.
Proteinopathy—PET surrogate markers
ALS and the ALS-FTLD spectrum disease are characterized as TDP-43 proteinopathy, where TAR DNA-Binding Protein 43 kDa (TDP-43) links both familial and sporadic forms of ALS. Cytoplasmatic aggregates of TDP-43 are a hallmark of the disease on a cellular level, and protein mislocalization is often regarded as a key mechanism underlying ALS. Up to now, there is no direct PET imaging ligand successfully targeting TDP-43, and out of the notion that multiple pathological proteins may be present in neurodegenerative disease, several groups have investigated the utility of established tau-directed PET tracers to characterize ALS (76, 77, 92–94). As diffusion tensor MR imaging (DTI) has been previously established to identify TDP-43 associated alterations (95), combined PET/MRI will be the modality of choice to further elucidate the role of tau PET imaging in ALS.
Imaging of disease-inherent pathological alterations—MRI
Neuroimaging with MRI has an essential role in the clinical diagnostic processes for ALS in the exclusion of other etiologies of the clinical presentation (96). In addition, MRI approaches with advanced postprocessing have been established as biological markers of the disease with reliable measures for monitoring disease progression and have greatly improved our understanding of its in vivo pathoanatomy (6). Here, diffusion-weighted imaging techniques including diffusion tensor imaging (DTI) had been a focus of research in many studies to investigate microstructural white matter tract alterations in MND (97, 98).
Diffusion tensor imaging
By using the DTI, diffusivity in human brain white matter can be non-invasively mapped to first quantify its regional directional dependence, and second, to obtain a reconstruction of fiber tracts by fiber tracking techniques (99). DTI has been established as a robust non-invasive technical tool to investigate the WM neuronal tracts in vivo to define anatomical signatures of the different phenotypes of MND and to track in vivo the progressive spread of pathological protein aggregates (100). As the neuropathological basis of the ALS-associated propagation patterns in the brain, four neuropathological stages have been defined for ALS, based upon the distribution patterns of phosphorylated 43 kDa TAR DNA-binding protein (101, 102): the sequential protein pathology is spreading initially from the motor neocortex toward the spinal cord and brainstem, followed by spreading to frontal, parietal, and, ultimately, anteromedial temporal lobes. This corticoefferent spreading model has been transferred to DTI-based concepts by a tract of interest (TOI)-based mapping, and DTI seems to be a valid surrogate marker to assess the spreading of TDP-43 pathology in vivo within the corresponding neuronal WM tracts (103–105). TOI-based mapping as a hypothesis-driven approach images the neuropathologically proposed sequential progression of ALS in the respective cerebral tract systems, i.e., the CST (as a correlate of ALS-stage 1), the corticorubral and corticopontine tracts (corresponding to ALS stage 2), the corticostriatal pathway (as a correlate of ALS stage 3), and the proximal portion of the perforant path (corresponding to ALS stage 4) (106). This tract-based in vivo-staging concept was applied to further ALS variants like primary lateral sclerosis (PLS) (104), lower motor neuron disease/progressive muscular atrophy (107), progressive bulbar palsy (108), and flail limb syndrome (109). In all of these restricted phenotypical ALS variants, an ALS-like in vivo alteration pattern of corticoefferent fibers according to the ALS disease propagation model could be shown. DTI-based methods, thus, seem to be a valuable tool for guiding the pathoanatomy definition of MND subtypes, in accordance with current proposals for clinical diagnosis, i.e., the Gold Coast Criteria (110). These results encourage future neuroimaging studies across the phenotypical ALS spectrum to contribute to our understanding of potential modifiers of the clinical presentations in ALS (109).
To this end, it is important to acquire longitudinal imaging data given that longitudinal MRI studies have the potential to provide crucial insights into the natural trajectory of ALS-associated neurodegenerative processes, although it has to be considered that standardized design is required to enable meaningful data interpretation (111). Longitudinal MRI studies in ALS have already been applied to subject groups of heterogeneous sample size (112–116), and reported fractional anisotropy (FA) reduction in the CST as the common core finding. Other DTI measures beyond FA add information on the ALS-associated pathoanatomy, such as a segmental radial diffusivity profile developed by Schuster and colleagues (111). Neurite orientation dispersion and density imaging (NODDI), a multicompartment model of diffusion MRI, demonstrated axonal loss within the CST together with dendritic alterations within the precentral gyrus, suggesting microstructural cortical dendritic changes occur together with CST axonal damage (117).
Protocol standardization and multisite MRI data
Advanced imaging protocols with more sophisticated techniques to analyze ever-increasing datasets to guide in the understanding of the anatomical and temporal factors of the biological processes of ALS benefit from collaborations across the entire ALS research community (118). Multicenter approaches like the Neuroimaging Society in Amyotrophic Lateral Sclerosis (NiSALS) with up-to-date, ultimately harmonized neuroimaging protocols aim to obtain high subject numbers and therefore to increase the reliability of results (8, 119, 120). Given that multicenter imaging studies have the limitation of scanner and protocol variability, there were successful approaches to merge data recorded at different sites and/or with different DTI protocols (8, 121, 122).
The Canadian ALS Neuroimaging Consortium (CALSNIC) was established in part to address the challenges associated with protocol variability when pooling multisite data. CALSNIC is a multicenter imaging biomarker validation platform that established from its inception harmonized clinical and imaging protocols across multiple MR platforms. Operating at research sites in Canada and the USA, the platform has conducted two prospective longitudinal studies (CALSNIC1 and CALSNIC2), each including multimodal MRI, neurocognitive assessments, and speech recordings (9). To date, there have been 250 patients with ALS/MND and 200 healthy controls enrolled, with CALSNIC2 ongoing. A recent study of longitudinal DTI-based microstructural alterations in ALS from CALSNIC determined a time interval of about 110 days is the minimum follow-up time to detect longitudinal microstructural alterations (123). Other longitudinal observations within this time frame reported by this consortium include gray and white matter atrophy with deformation-based morphometry (124), altered motor and prefrontal cortex neurochemistry using magnetic resonance spectroscopy (125), and regional texture changes in T1-weighted images (126). Collaborative and multicenter projects like this will be useful in ascertaining the reliability of imaging biomarkers under development (127).
MR findings with respect to genetic phenotype
The field of genetic ALS continues to develop rapidly with multiple disease gene discoveries per year (128), with the autosomal dominant inheritance of a hexanucleotide expansion in the first intron of the C9orf72 gene being the most common cause of familial ALS in people of Northern European ancestry, also as a major contributor to frontotemporal pathology in ALS. DTI studies in patients with C9orf72 expansion in cross-sectional and longitudinal design demonstrated alterations in motor tracts (129–131); in addition, further white matter areas were found to be affected, e.g., in the frontal white matter (132) and segmentally in the corpus callosum (133). In addition, the in vivo histopathological staging approach was also applied to C9orf72-associated ALS and demonstrated a corticoefferent involvement pattern according to the staging scheme—a pattern that was not observed in Super Oxide Dismutase 1-associated ALS (134). In the last decade, the pre-symptomatic phase of the disease has gained increasing interest, addressing people with family history and genetic risk for ALS without manifestations of the disease (135). Neuroimaging studies in presymptomatic ALS offer opportunities to characterize early genotype-associated signatures and propagation patterns and factors (7). Current initiatives have, thus, integrated natural history and biomarker data on presymptomatic ALS for the design and implementation of pre-symptomatic ALS trials (136). Specifically, in C9orf72 mutation carriers, DTI studies reported regional reductions of white matter integrity (131, 137), as an indicator of general developmental tardiness. At the spinal level, C9orf72-positive subjects older than 40 years were shown to exhibit considerable WM atrophy at C2–C7 vertebral levels in conjunction with progressive pyramidal tract FA reductions (138).
Resting-state functional MRI
Brain regions that are co-activated under resting conditions delineate the so-called “resting-state” (RS) functional networks. The assessment of connectivity alterations between RS networks has provided important insights into brain functional reorganization in several neurodegenerative diseases, including ALS, in which motor and—when present—cognitive impairment may undermine the use of task-based fMRI (139–141).
Several studies showed decreased functional connectivity of the sensorimotor network in ALS patients (142), whereas others found increased connectivity (143), or complex regional patterns of decreased and increased functional connectivity (144, 145). Altered functional connectivity has also been shown in brain networks related to cognition and behavior (especially the default mode and frontoparietal networks) (146, 147), consistent with the multisystem involvement of ALS pathology. It has been suggested that an increase in brain functional connectivity might prevail in earlier stages of the disease as a compensatory mechanism, with a subsequent decrease as pathological burden accumulates. Consistent with this hypothesis, increased functional connectivity was found to be higher in patients with less severe microstructural damage to the CST (148), and associated with a lower rate of disease progression, shorter disease duration (145), and preserved motor function (148). Decreased RS functional connectivity in the sensorimotor and thalamic networks, paralleling progression of structural alterations and clinical decline, was observed over a 2-year period in ALS patients (149). The co-occurrent progressive increase of functional connectivity in extra-motor networks, such as the left fronto-parietal and the temporal RS networks (147, 149), is also consistent with a “disconnection” hypothesis due to the loss of compensation. However, some studies also showed increased functional connectivity within the regions of structural disruption in ALS correlating with faster disease progression (142), and greater clinical and executive cognitive impairment (143, 146). Therefore, a more direct pathogenic involvement of increased functional connectivity related to the loss of local inhibitory circuitry within the primary motor and frontal cortex is also possible.
Graph analysis and connectomics
The human brain is a highly integrated neural network consisting of several cortical and subcortical regions that are structurally and functionally interconnected, forming co-operating sub-networks. Graph theoretical models have conceptualized such complex organization as the brain “connectome”, consisting of anatomic regions defined as “nodes”, which are linked by “edges” (i.e., structural or functional connections). In ALS, graph analysis and connectomics might represent a powerful approach to detect upper motor neuron degeneration, extramotor brain changes, and network reorganization associated with the disease.
Two independent studies applied network-based statistics to DT MRI of patients with ALS, both demonstrating the presence of an impaired sub-network including bilateral primary motor regions, supplementary motor areas, basal ganglia, and associative parietal areas (150, 151). Patients with a C9orf72 mutation showed a more widespread white matter involvement (152). In a longitudinal study, the sub-network of impaired connectivity expanded over time, involving frontal, temporal, and parietal regions (150), consistent with the proposed model of TDP-43 pathological spreading. In line with such hypothesis, a study evaluated brain structural connectivity in a consistent set of healthy controls, showing that regions involved in subsequent stages of ALS pathology are highly interconnected by WM tracts, which may serve as anatomical “infrastructures” facilitating TDP-43 spread (153). More recently, a computational model was applied to the MRI scan of ALS patients to simulate this progressive network degeneration (154). Computer-simulated aggregation levels mimic true disease patterns in ALS patients. Simulated patterns of involvement across cortical areas show significant overlap with the patterns of empirically impaired brain regions on later scans, in accordance with established pathological staging systems (152).
Few studies applied network-based analyses to the assessment of functional alterations in ALS patients using resting-state functional MRI (rs-fMRI), demonstrating complex connectivity alterations encompassing frontal, temporal, and occipital regions (155, 156). A recent study assessed the functional and structural connectivity patterns across the ALS-FTD spectrum, investigating whether and where MRI connectivity alterations of ALS patients with any degree of cognitive impairment (i.e., ALS-ci/bi and ALS-FTD) resembled more the pattern of connectome damage of ALS or bvFTD (157). As compared with controls, ALS-ci/bi patients demonstrated an “ALS-like” pattern of structural damage, diverging from ALS without cognitive impairment with similar motor impairment for the presence of enhanced functional connectivity within sensorimotor areas and decreased functional connectivity within the “bvFTD-like” pattern. On the other hand, ALS-FTD patients resembled both structurally and functionally the bvFTD-like pattern of connectome damage with, in addition, the structural ALS-like damage in the motor areas. A maladaptive role of functional rearrangements in ALS-ci/bi concomitantly with similar structural alterations compared to ALS without cognitive impairment supports the hypothesis that ALS-ci/bi might be considered as a phenotypic variant of ALS, rather than a consequence of disease worsening.
In a multicenter study (158), compared with healthy controls, patients with ALS and patients with PLS showed altered structural global network properties, as well as local topologic alterations and decreased structural connectivity in sensorimotor, basal ganglia, frontal, and parietal areas. Patients with PMA showed, instead, preserved global structure. Increased local functional connectivity was observed in patients with ALS in the precentral, middle, and superior frontal areas, and in patients with PLS in the sensorimotor, basal ganglia, and temporal networks. In patients with ALS and patients with PLS, structural connectivity alterations correlated with motor impairment, whereas functional connectivity disruption was closely related to executive dysfunction and behavioral disturbances (158).
Magnetic resonance spectroscopy
In addition to evaluating structural, microstructural, and functional changes, magnetic resonance spectroscopy (MRS) permits the probing of neurochemical correlates of neurodegeneration in ALS (12). Numerous studies using varying techniques (single voxel or multivoxel) have consistently revealed reduced N-acetylaspartate (NAA, a chemical marker of neuronal integrity) in motor and extra-motor regions in ALS. Other metabolites of interest include myo-inositol (mIns, a glial marker), and the excitatory and inhibitory neurotransmitter system involving glutamate, glutamine, and GABA (159). Technological advances in MR hardware and spectral acquisition and editing methods have increased the ability to more readily resolve such metabolites. For example, this includes ultra-high field studies at 7 tesla for glutamate, glutamine, and GABA (160, 161) and MEGA-PRESS for GABA (162, 163) detection. Combined PET-MR imaging with MRS provides the opportunity to explore complementary pathological or pathophysiological mechanisms simultaneously from the molecular and neurochemical perspectives. In a PET-MR study in ALS that included MRS motor cortex inflammation, measured using the TSPO tracer [11C]-PBR28, and gliosis, measured using the myo-inositol signal, were found to be directly correlated in the motor cortex (164).
Machine learning classifiers
One of the overarching aims in advanced neuroimaging biomarker development in neurodegenerative disorders like ALS is the observer-independent classification of imaging data for individual patient's stratification for later use in multicenter therapeutic trials; to this end, there is a rapidly growing interest in Machine Learning (ML) models, classifiers, and predictive modeling in ALS (165). The choice of the ML model in ALS neuroimaging needs to be carefully tailored to a proposed application based on the characteristics of the available data and the profile of the candidate model, as proposed by Grollemund et al. (166). A recent systematic review on MRI feature selection for ML-based neuroimaging classifiers in ALS suggested the integration of DTI, volumetric, and texture data (167), but potential future applications might include a multiparametric MRI combination of more approaches such as intrinsic functional connectivity MRI. Connectome-based analyses of multiparametric MRI have already demonstrated their potential as a tool for patient stratification and as a prognostic biomarker in ALS to predict disease progression (154). PET/MRI is able to provide a multiparametric protocol, where a multimodal composite score may combine the aforementioned PET and MR techniques to address specific questions [e.g., (168)]—for a review see (169).
Summary
Neuroimaging fingerprinting of genetically defined ALS phenotypes will be important, especially longitudinal investigations of presymptomatic mutation carriers. Neuroimaging offers the possibility to undertake cross-sectional and longitudinal studies to stratify ALS patients according to their intrinsic progression rate, thus helping to optimize disease management, enhancing the design of drug trials, and guiding the use of individualized treatments when these become available. Recent research has contributed to the change in perception of neuroimaging in motor neuron disease, which traditionally had been primarily an academic tool with limited direct relevance to individualized patient care, but, with the advances in computational imaging, has emerged as a viable clinical tool with true biomarker potential (170).
MRI and PET provide methodologically different and partially complementary information on disease pathology. There are multiple aspects where applied neuroimaging and biomarker imaging strategies in neurodegenerative disease are influenced by and directly benefit from simultaneous PET/MRI:
• Concurrent acquisition and analysis augment the precision of partial volume correction for PET data by minimizing the main confounds introduced by small misregistration and data resampling inaccuracies of post-hoc coregistration of PET and segmented MRI acquired at different time points and in different scanner coordinate systems (171, 172).
• Novel regularized PET image reconstruction techniques based on anatomical priors derived from concurrent MRI significantly improve PET image quality (173–175).
• Novel readout and quantitation techniques including radiomics and machine learning/artificial intelligence informed algorithms benefit directly from inherently coregistered data and the high degree of standardization possible in PET/MRI, likely to result in improved performance of AI applications (176– 180).
• Data consistency of simultaneous PET/MRI improves data pooling of different varieties of radioligands of the same functional target, assisting in moving toward multicenter therapeutic trials.
• Simultaneous PET/MRI and the inherent temporal synchronicity of findings will be instrumental in the development of tailored imaging probes or assessing the effects of drug challenges in treatments (181–184).
• PET/MRI enables to the design of more complex prospective trials using multiple tracers to characterize a disease, capitalizing on an intelligent spread of complex MR protocols over consecutive PET/MRI sessions using different tracers to max out the gain of information by each session and still ensure patient compliance (185).
Future developments may include a possible combination of rapid multi-tracer PET in a single PET/MRI session, making use of the high spatial information provided by MRI to improve signal separation in multi-tracer and multi-isotope studies, where typically staggered injection of ultra-short lived radionuclides combined with longer half-lived ones is practiced, and where spatial registration between different stages is crucial (186). Other future applications might include the combination of simultaneous PET/MRI with hyperpolarized MR imaging (187), to add even more layers of complementary metabolic information.
To summarize, the roles of MRI and PET as straight-forward diagnostic tools in ALS and further neurodegenerative disorders are emerging; the concepts to use them as a biological marker or as a read-out in clinical trials are existing and have to be probed for their clinical relevance. Combined PET/MRI has the potential as a future gold standard for characterizing motor-neuron disease and offers an important contribution to the standardization of imaging across multiple centers.
Author contributions
FJ, JK, and H-PM contributed to conception and design. FJ wrote the first draft of the manuscript and designed the figure and done the final revision of the manuscript. JK, H-PM, SK, and FA contributed complete sections. FW, AT, WT, and RS performed a review for intellectual and scientific content. All authors contributed to the article and approved the submitted version.
Conflict of interest
FJ serves as Associate Editor for Frontiers in Neurology, Section Applied Neuroimaging and as Associate Editor for Frontiers in Oncology, Section Cancer Imaging and Image-directed Interventions. He has received the ALS Canada-Brain Canada Discovery Grant 2021 and receives or has received research operating funds from Biogen and the University of Alberta. SK has received research operating funds from Biogen. FA serves as Section Editor of NeuroImage: Clinical. She has received speaker honoraria from Roche and Biogen Idec; and receives or has received research supports from the Italian Ministry of Health, AriSLA (Fondazione Italiana di Ricerca per la SLA), the European Research Council and Foundation Research on Alzheimer Disease. H-PM serves as Associate Editor for Frontiers in Neurology, Section Applied Neuroimaging. JK serves as Specialty Chief Editor for Frontiers in Neurology, Section Applied Neuroimaging and as Associate Editor Neurology for Therapeutic Advances in Chronic Disease. He has received consulting fees as an advisory board member or honoraria as a speaker from AbbVie, BIAL, Biogen, Boehringer Ingelheim, Desitin, Esteve, Licher MT, Medtronic, Novartis, STADA, UCB Pharma, Zambon.
The remaining authors declare that the research was conducted in the absence of any commercial or financial relationships that could be construed as a potential conflict of interest.
Publisher's note
All claims expressed in this article are solely those of the authors and do not necessarily represent those of their affiliated organizations, or those of the publisher, the editors and the reviewers. Any product that may be evaluated in this article, or claim that may be made by its manufacturer, is not guaranteed or endorsed by the publisher.
References
1. Brooks BR. El escorial world federation of neurology criteria for the diagnosis of amyotrophic lateral sclerosis. J Neurol Sci. (1994) 124:96–107. doi: 10.1016/0022-510X(94)90191-0
2. Kassubek J, Pagani M. Imaging in amyotrophic lateral sclerosis: MRI and pet. Curr Opin Neurol. (2019) 32:740–6. doi: 10.1097/WCO.0000000000000728
3. Marini C, Cistaro A, Campi C, Calvo A, Caponnetto C., Flavio, et al. A Pet/Ct approach to spinal cord metabolism in amyotrophic lateral sclerosis. Eur J Nucl Med Mol Imaging. (2016) 43:2061–71. doi: 10.1007/s00259-016-3440-3
4. Massone AM, Campi C, Beltrametti MC, Marini C (editors). FDG-PET and the assessment of spinal cord metabolism in amyotrophic lateral sclerosis (ALS). In: 2016 IEEE Nuclear Science Symposium, Medical Imaging Conference and Room-Temperature Semiconductor Detector Workshop (NSS/MIC/RTSD), (2016).
5. Sala A, Iaccarino L, Fania P, Vanoli EG, Fallanca F, Pagnini C, et al. Testing the diagnostic accuracy of [18f]Fdg-pet in discriminating spinal- and bulbar-onset amyotrophic lateral sclerosis. Eur J Nucl Med Mol Imaging. (2019) 46:1117–31. doi: 10.1007/s00259-018-4246-2
6. Chiò A, Pagani M, Agosta F, Calvo A, Cistaro A, Filippi M. Neuroimaging in amyotrophic lateral sclerosis: Insights into structural and functional changes. The Lancet Neurol. (2014) 13:1228–40. doi: 10.1016/S1474-4422(14)70167-X
7. Chipika RH, Siah WF, McKenna MC, Li Hi Shing S, Hardiman O, Bede P. The presymptomatic phase of amyotrophic lateral sclerosis: are we merely scratching the surface? J Neurol. (2021) 268:4607–29. doi: 10.1007/s00415-020-10289-5
8. Müller H-P, Turner MR, Grosskreutz J, Abrahams S, Bede P, Govind V, et al. A large-scale multicentre cerebral diffusion tensor imaging study in amyotrophic lateral sclerosis. J Neurol Neurosurg Psychiatry. (2016) 87:570–9. doi: 10.1136/jnnp-2015-311952
9. Kalra S, Khan M, Barlow L, Beaulieu C, Benatar M, Briemberg H. The Canadian Als Neuroimaging Consortium (Calsnic) - a Multicentre Platform for Standardized Imaging Clinical Studies in Als (2020). Available online at: https://www.medrxiv.org/content/10.1101/2020.07.10.20142679v2 (accessed July 15, 2020).
10. Treit S, Steve T, Gross DW, Beaulieu C. High resolution in-vivo diffusion imaging of the human hippocampus. Neuroimage. (2018) 182:479–87. doi: 10.1016/j.neuroimage.2018.01.034
11. Agosta F, Spinelli EG, Filippi M. Neuroimaging in amyotrophic lateral sclerosis: current and emerging uses. Expert Rev Neurother. (2018) 18:395–406. doi: 10.1080/14737175.2018.1463160
12. Kalra S. Magnetic resonance spectroscopy in als. Front Neurol. (2019) 10:482. doi: 10.3389/fneur.2019.00482
13. Armstrong IS, Thomson KE, Rowley LM, McGowan DR. Harmonizing standardized uptake value recovery between two Pet/Ct systems from different manufacturers when using resolution modelling and time-of-flight. Nucl Med Commun. (2017) 38:650–5. doi: 10.1097/MNM.0000000000000682
14. de Jong EEC, van Elmpt W, Hoekstra OS, Groen HJM, Smit EF, Boellaard R, et al. Quality assessment of positron emission tomography scans: recommendations for future multicentre trials. Acta Oncol. (2017) 56:1459–64. doi: 10.1080/0284186X.2017.1346824
15. Namías M, Bradshaw T, Menezes VO, Machado MAD, Jeraj R. A novel approach for quantitative harmonization in pet. Phys Med Biol. (2018) 63:095019. doi: 10.1088/1361-6560/aabb5f
16. Ferretti A, Chondrogiannis S, Rampin L, Bellan E, Marzola MC, Grassetto G, et al. How to harmonize suvs obtained by hybrid Pet/Ct scanners with and without point spread function correction. Phys Med Biol. (2018) 63:235010. doi: 10.1088/1361-6560/aaee27
17. Agosta F, Altomare D, Festari C, Orini S, Gandolfo F, Boccardi M, et al. Clinical utility of Fdg-pet in amyotrophic lateral sclerosis and huntington's disease. Eur J Nucl Med Mol Imaging. (2018) 45:1546–56. doi: 10.1007/s00259-018-4033-0
18. Canosa A, Pagani M, Cistaro A, Montuschi A, Iazzolino B, Fania P, et al. 18f-Fdg-pet correlates of cognitive impairment in Als. Neurology. (2016) 86:44–9. doi: 10.1212/WNL.0000000000002242
19. Canosa A, Moglia C, Manera U, Vasta R, Torrieri MC, Arena V, et al. Metabolic brain changes across different levels of cognitive impairment in als: a (18)F-Fdg-pet study. J Neurol Neurosurg Psychiatry. (2020). doi: 10.1136/jnnp-2020-323876
20. Devrome M, Van Weehaeghe D, De Vocht J, Van Damme P, Van Laere K, Koole M. Glucose metabolic brain patterns to discriminate amyotrophic lateral sclerosis from parkinson plus syndromes. Eur J Necl Med Mol Imaging Res. (2018) 8:110. doi: 10.1186/s13550-018-0458-5
21. D'Hulst L, Van Weehaeghe D, Chiò AA-O, Calvo A, Moglia C, Canosa A, et al. Multicenter validation of [(18)F]-FDG PET and support-vector machine discriminant analysis in automatically classifying patients with amyotrophic lateral sclerosis versus controls. Amyotroph Lateral Scler Frontotemporal Degener. (2018) 19:570–7. doi: 10.1080/21678421.2018.1476548
22. Canosa A, Calvo A, Moglia C, Manera U, Vasta R, Di Pede F, et al. Brain metabolic changes across king's stages in amyotrophic lateral sclerosis: a (18)F-2-Fluoro-2-Deoxy-D-glucose-positron emission tomography study. Eur J Nucl Med Mol Imaging. (2021) 48:1124–33. doi: 10.1007/s00259-020-05053-w
23. Canosa A, Vacchiano V, D'Ovidio F, Calvo A, Moglia C, Manera U, et al. Brain metabolic correlates of apathy in amyotrophic lateral sclerosis: an 18f-Fdg-positron emission tomography study. Eur J Neurol. (2021) 28:745–53. doi: 10.1111/ene.14637
24. De Vocht J, Blommaert J, Devrome M, Radwan A, Van Weehaeghe D, De Schaepdryver M, et al. Use of multimodal imaging and clinical biomarkers in presymptomatic carriers of C9orf72 repeat expansion. JAMA Neurol. (2020) 77:1008–17. doi: 10.1001/jamaneurol.2020.1087
25. Canosa A, Calvo A, Moglia C, Vasta R, Palumbo F, Solero L, et al. Amyotrophic lateral sclerosis with sod1 mutations shows distinct brain metabolic changes. Eur J Nucl Med Mol Imaging. (2022) 49:2242–50. doi: 10.1007/s00259-021-05668-7
26. Pagani M, Chiò A, Valentini MC, Öberg J, Nobili F, Calvo A, et al. Functional pattern of brain Fdg-pet in amyotrophic lateral sclerosis. Neurology. (2014) 83:1067–74. doi: 10.1212/WNL.0000000000000792
27. Zanovello M, Soraru G, Campi C, Anglani M, Spimpolo A, Berti S, et al. Brain stem glucose hypermetabolism in amyotrophic lateral sclerosis/frontotemporal dementia and shortened survival: an (18)F-Fdg Pet/MRI Study. J Nucl Med. (2022) 63:777–84. doi: 10.2967/jnumed.121.262232
28. Sitte HH, Wanschitz J, Budka H, Berger ML. Autoradiography with [3h]Pk11195 of spinal tract degeneration in amyotrophic lateral sclerosis. Acta Neuropathol. (2001) 101:75–8. doi: 10.1007/s004010000312
29. Turner MR, Cagnin A, Turkheimer FE, Miller CCJ, Shaw CE, Brooks DJ, et al. Evidence of widespread cerebral microglial activation in amyotrophic lateral sclerosis: an [11c](R)-Pk11195 positron emission tomography study. Neurobiol Dis. (2004) 15:601–9. doi: 10.1016/j.nbd.2003.12.012
30. Suridjan I, Rusjan PM, Kenk M, Verhoeff NP, Voineskos AN, Rotenberg D, et al. Quantitative imaging of neuroinflammation in human white matter: a positron emission tomography study with translocator protein 18 Kda Radioligand, [18f]-Feppa. Synapse. (2014) 68:536–47. doi: 10.1002/syn.21765
31. Le Pogam A, Vercouilly J, Corcia P, Praline J, Guilloteau D, Baulieu JL, et al. Preliminary results of pet with 18f-Dpa 714 in humans for detection of microglial activation in amyotrophic lateral sclerosis (Als). J Nucl Med. (2008) 49(supplement 1):231P.
32. Radlinska BA, Ghinani SA, Lyon P, Jolly D, Soucy J-P, Minuk J, et al. Multimodal microglia imaging of fiber tracts in acute subcortical stroke. Ann Neurol. (2009) 66:825–32. doi: 10.1002/ana.21796
33. Koole M, Schmidt ME, Hijzen A, Ravenstijn P, Vandermeulen C, Van Weehaeghe D, et al. (18)F-Jnj-64413739, a novel pet ligand for the P2x7 ion channel: radiation dosimetry, kinetic modeling, test-retest variability, and occupancy of the P2x7 antagonist Jnj-54175446. J Nucl Med. (2019) 60:683–90. doi: 10.2967/jnumed.118.216747
34. Hagens MHJ, Golla SSV, Janssen B, Vugts DJ, Beaino W, Windhorst AD, et al. The P2x7 Receptor Tracer [11c]Smw139 as an in vivo marker of neuroinflammation in multiple sclerosis: a first-in man study. Eur J Nucl Med Mol Imaging. (2020) 47:379–89. doi: 10.1007/s00259-019-04550-x
35. Franceschini A, Capece M, Chiozzi P, Falzoni S, Sanz JM, Sarti AC, et al. The P2x7 receptor directly interacts with the Nlrp3 inflammasome scaffold protein. FASEB J. (2015) 29:2450–61. doi: 10.1096/fj.14-268714
36. Yiangou Y, Facer P, Durrenberger P, Chessell IP, Naylor A, Bountra C, et al. Cox-2, Cb2 and P2x7-immunoreactivities are increased in activated microglial cells/macrophages of multiple sclerosis and amyotrophic lateral sclerosis spinal cord. BMC Neurol. (2006) 6:12. doi: 10.1186/1471-2377-6-12
37. Gandelman M, Peluffo H, Beckman JS, Cassina P, Barbeito L. Extracellular Atp and the P2x7 receptor in astrocyte-mediated motor neuron death: implications for amyotrophic lateral sclerosis. J Neuroinflamm. (2010) 7:33. doi: 10.1186/1742-2094-7-33
38. Liu J, Prell T, Stubendorff B, Keiner S, Ringer T, Gunkel A, et al. Down-regulation of purinergic P2x7 receptor expression and intracellular calcium dysregulation in peripheral blood mononuclear cells of patients with amyotrophic lateral sclerosis. Neurosci Lett. (2016) 630:77–83. doi: 10.1016/j.neulet.2016.07.039
39. Ruiz-Ruiz C, Calzaferri F, García AG. P2x7 receptor antagonism as a potential therapy in amyotrophic lateral sclerosis. Front Mol Neurosci. (2020) 13:93. doi: 10.3389/fnmol.2020.00093
40. Bhardwaj A, Kaur J, Wuest M, Wuest F. In situ click chemistry generation of cyclooxygenase-2 inhibitors. Natu Commun. (2017) 8:1. doi: 10.1038/s41467-016-0009-6
41. Tietz O, Marshall A, Bergman C, Wuest M, Wuest F. Impact of structural alterations on the radiopharmacological profile of 18f-Labeled pyrimidines as cyclooxygenase-2 (Cox-2) imaging agents. Nucl Med Biol. (2018) 62–3:9–17. doi: 10.1016/j.nucmedbio.2018.05.001
42. Litchfield M, Wuest M, Glubrecht D, Wuest F. Radiosynthesis and biological evaluation of [18 F]triacoxib: a new radiotracer for pet imaging of Cox-2. Mol Pharm. (2020) 17:251–61. doi: 10.1021/acs.molpharmaceut.9b00986
43. Carrera-Juliá S, Moreno ML, Barrios C, de la Rubia Ortí JE, Drehmer E. Antioxidant alternatives in the treatment of amyotrophic lateral sclerosis: a comprehensive review. Front Physiol. (2020) 11:63. doi: 10.3389/fphys.2020.00063
44. McCombe PA, Lee JD, Woodruff TM, Henderson RD. The peripheral immune system and amyotrophic lateral sclerosis. Front Neurol. (2020) 11:279. doi: 10.3389/fneur.2020.00279
45. Luo Y, Xue H, Pardo AC, Mattson MP, Rao MS, Maragakis NJ. Impaired Sdf1/Cxcr4 signaling in glial progenitors derived from Sod1(G93a) mice. J Neurosci Res. (2007) 85:2422–32. doi: 10.1002/jnr.21398
46. Perner C, Perner F, Stubendorff B, Förster M, Witte OW, Heidel FH, et al. Dysregulation of chemokine receptor expression and function in leukocytes from als patients. J Neuroinflamm. (2018) 15:99. doi: 10.1186/s12974-018-1135-3
47. Calvo A, Moglia C, Canosa A, Cammarosano S, Ilardi A, Bertuzzo D, et al. Common polymorphisms of chemokine (C-X3-C Motif) receptor 1 gene modify amyotrophic lateral sclerosis outcome: a population-based study. Muscle Nerve. (2018) 57:212–6. doi: 10.1002/mus.25653
48. Lapa C, Kircher S, Schirbel A, Rosenwald A, Kropf S, Pelzer T, et al. Targeting Cxcr4 with [68ga]pentixafor: a suitable theranostic approach in pleural mesothelioma? Oncotarget. (2017) 8:96732–7. doi: 10.18632/oncotarget.18235
49. Kircher M, Herhaus P, Schottelius M, Buck AK, Werner RA, Wester H-J, et al. Cxcr4-directed theranostics in oncology and inflammation. Ann Nucl Med. (2018) 32:503–11. doi: 10.1007/s12149-018-1290-8
50. Burke BP, Miranda CS, Lee RE, Renard I, Nigam S, Clemente GS, et al. Copper-64 pet imaging of the cxcr4 chemokine receptor using a cross-bridged cyclam bis-tetraazamacrocyclic antagonist. J Nucl Med. (2020) 61:123–8. doi: 10.2967/jnumed.118.218008
51. Dawbarn D, Allen SJ. Neurotrophins and neurodegeneration. Neuropathol Appl Neurobiol. (2003) 29:211–30. doi: 10.1046/j.1365-2990.2003.00487.x
52. Bailey JJ, Kaiser L, Lindner S, Wust M, Thiel A, Soucy JP, et al. First-in-human brain imaging of [(18)F]Track, a pet tracer for tropomyosin receptor kinases. ACS Chem Neurosci. (2019) 10:2697–702. doi: 10.1021/acschemneuro.9b00144
53. Schirrmacher R, Bailey JJ, Mossine AV, Scott PJH, Kaiser L, Bartenstein P, et al. Radioligands for tropomyosin receptor kinase (Trk) positron emission tomography imaging. Pharmaceuticals. (2019) 12:7. doi: 10.3390/ph12010007
54. Singleton TA, Bdair H, Bailey JJ, Choi S, Aliaga A, Rosa-Neto P, et al. Efficient radiosynthesis and preclinical evaluation of [18 F]fompyd as a positron emission tomography tracer candidate for Trkb/C receptor imaging. J Labell Comp Radiopharm. (2020) 63:144–50. doi: 10.1002/jlcr.3827
55. Tuszynski MH, Yang JH, Barba D., U HS, Bakay RA, Pay MM, et al. Nerve growth factor gene therapy: activation of neuronal responses in Alzheimer disease. JAMA Neurol. (2015) 72:1139–47. doi: 10.1001/jamaneurol.2015.1807
56. Osborne A, Wang AXZ, Tassoni A, Widdowson PS, Martin KR. Design of a novel gene therapy construct to achieve sustained brain-derived neurotrophic factor signaling in neurons. Hum Gene Ther. (2018) 29:828–41. doi: 10.1089/hum.2017.069
57. Guo W, Pang K, Chen Y, Wang S, Li H, Xu Y, et al. Trkb agonistic antibodies superior to bdnf: utility in treating motoneuron degeneration. Neurobiol Dis. (2019) 132:104590. doi: 10.1016/j.nbd.2019.104590
58. Hammers A, Koepp MJ, Richardson MP, Hurlemann R, Brooks DJ, Duncan JS. Grey and white matter flumazenil binding in neocortical epilepsy with normal MRI. A pet study of 44 patients. Brain. (2003) 126:1300–18. doi: 10.1093/brain/awg138
59. Andersson JD, Matuskey D, Finnema SJ. Positron emission tomography imaging of the gamma-aminobutyric acid system. Neurosci Lett. (2019) 691:35–43. doi: 10.1016/j.neulet.2018.08.010
60. Yamauchi H, Kudoh T, Kishibe Y, Iwasaki J, Kagawa S. Selective neuronal damage and borderzone infarction in carotid artery occlusive disease: a 11c-flumazenil pet study. J Nucl Med. (2005) 46:1973–9.
61. Ihara M, Tomimoto H, Ishizu K, Yoshida H, Sawamoto N, Hashikawa K, et al. Association of vascular parkinsonism with impaired neuronal integrity in the striatum. J Neural Transm. (2007) 114:577–84. doi: 10.1007/s00702-006-0610-7
62. Mitterhauser M, Wadsak W, Wabnegger L, Mien LK, Tögel S, Langer O, et al. Biological evaluation of 2′-[18f]fluoroflumazenil ([18f]Ffmz), a potential gaba receptor ligand for pet. Nucl Med Biol. (2004) 31:291–5. doi: 10.1016/j.nucmedbio.2003.09.003
63. Lloyd CM, Richardson MP, Brooks DJ, Al-Chalabi A, Leigh PN. Extramotor involvement in Als: pet studies with the Gaba(a) Ligand [11c]Flumazenil. Brain. (2000) 123:2289–96. doi: 10.1093/brain/123.11.2289
64. Turner MR, Hammers A, Al-Chalabi A, Shaw CE, Andersen PM, Brooks DJ, et al. Cortical involvement in four cases of primary lateral sclerosis using [(11)C]-flumazenil pet. J Neurol. (2007) 254:1033–6. doi: 10.1007/s00415-006-0482-7
65. Wicks P, Turner MR, Abrahams S, Hammers A, Brooks DJ, Leigh PN, et al. Neuronal loss associated with cognitive performance in amyotrophic lateral sclerosis: an (11c)-flumazenil pet study. Amyotr Lateral Scler. (2008). doi: 10.1080/17482960701737716
66. Yabe I, Tsuji-Akimoto S, Shiga T, Hamada S, Hirata K, Otsuki M, et al. Writing errors in als related to loss of neuronal integrity in the anterior cingulate gyrus. J Neurol Sci. (2012) 315:55–9. doi: 10.1016/j.jns.2011.11.039
67. Millet P, Graf C, Buck A, Walder B, Ibáñez V. Evaluation of the reference tissue models for pet and spect benzodiazepine binding parameters. Neuroimage. (2002) 17:928–42. doi: 10.1006/nimg.2002.1233
68. Richardson MP, Fristen KJ, Sisodiya SM, Koepp MJ, Ashburner J, Free SL, et al. Cortical grey matter and benzodiazepine receptors in malformations of cortical development. A voxel-based comparison of structural and functional imaging data. Brain. (1997) 120:1961–73.
69. Bajjalieh SM, Frantz GD, Weimann JM, McConnell SK, Scheller RH. Differential expression of synaptic vesicle protein 2 (Sv2) isoforms. J Neurosci. (1994) 14:5223. doi: 10.1523/JNEUROSCI.14-09-05223.1994
70. Bartholome O, Van den Ackerveken P, Sanchez Gil J, de la Brassinne Bonardeaux O, Leprince P, Franzen R, et al. Puzzling out synaptic vesicle 2 family members functions. Front Mol Neurosci. (2017) 10:148. doi: 10.3389/fnmol.2017.00148
71. Mendoza-Torreblanca JG, Vanoye-Carlo A, Phillips-Farfan BV, Carmona-Aparicio L, Gomez-Lira G. Synaptic vesicle protein 2a: basic facts and role in synaptic function. Eur J Neurosci. (2013) 38:3529–39. doi: 10.1111/ejn.12360
72. Vogl C, Tanifuji S, Danis B, Daniels V, Foerch P, Wolff C, et al. Synaptic vesicle glycoprotein 2a modulates vesicular release and calcium channel function at peripheral sympathetic synapses. Eur J Neurosci. (2015) 41:398–409. doi: 10.1111/ejn.12799
73. Chen MK, Mecca AP, Naganawa M, Finnema SJ, Toyonaga T, Lin SF, et al. Assessing synaptic density in alzheimer disease with synaptic vesicle glycoprotein 2a positron emission tomographic imaging. JAMA Neurol. (2018) 75:1215–24. doi: 10.1001/jamaneurol.2018.1836
74. Malpetti M, Holland N, Jones PS, Ye R, Cope TE, Fryer TD, et al. Synaptic density in carriers of C9orf72 mutations: a [(11) C]Ucb-J Pet Study. Ann Clin Transl Neurol. (2021) 8:1515–23. doi: 10.1002/acn3.51407
75. Delva A, Van Weehaeghe D, Koole M, Van Laere K, Vandenberghe W. Loss of presynaptic terminal integrity in the substantia nigra in early Parkinson's disease. Mov Disord. (2020) 35:1977–86. doi: 10.1002/mds.28216
76. Holland N, Jones PS, Savulich G, Wiggins JK, Hong YT, Fryer TD, et al. Synaptic loss in primary tauopathies revealed by [(11) C]Ucb-J positron emission tomography. Mov Disord. (2020) 35:1834–42. doi: 10.1002/mds.28188
77. Holland N, Malpetti M, Rittman T, Mak EE, Passamonti L, Kaalund SS, et al. Molecular pathology and synaptic loss in primary tauopathies: an 18f-Av-1451 and 11c-Ucb-J Pet Study. Brain. (2022) 145:340–8. doi: 10.1093/brain/awab282
78. Finnema SJ, Toyonaga T, Detyniecki K, Chen MK, Dias M, Wang Q, et al. Reduced synaptic vesicle protein 2a binding in temporal lobe epilepsy: a [(11) C]Ucb-J positron emission tomography study. Epilepsia. (2020) 61:2183–93. doi: 10.1111/epi.16653
79. Crowder KM, Gunther JM, Jones TA, Hale BD, Zhang HZ, Peterson MR, et al. Abnormal neurotransmission in mice lacking synaptic vesicle protein 2a (Sv2a). Proc Natl Acad Sci U A. (1999) 96:15268–73. doi: 10.1073/pnas.96.26.15268
80. Crevecoeur J, Kaminski RM, Rogister B, Foerch P, Vandenplas C, Neveux M, et al. Expression pattern of synaptic vesicle protein 2 (Sv2) Isoforms in patients with temporal lobe epilepsy and hippocampal sclerosis. Neuropathol Appl Neurobiol. (2014) 40:191–204. doi: 10.1111/nan.12054
81. Lynch BA, Lambeng N, Nocka K, Kensel-Hammes P, Bajjalieh SM, Matagne A, et al. The synaptic vesicle protein Sv2a is the binding site for the antiepileptic drug levetiracetam. Proc Natl Acad Sci USA. (2004) 101:9861–6. doi: 10.1073/pnas.0308208101
82. Mutch SA, Kensel-Hammes P, Gadd JC, Fujimoto BS, Allen RW, Schiro PG, et al. Protein quantification at the single vesicle level reveals that a subset of synaptic vesicle proteins are trafficked with high precision. J Neurosci. (2011) 31:1461–70. doi: 10.1523/JNEUROSCI.3805-10.2011
83. Takamori S, Holt M, Stenius K, Lemke EA, Gronborg M, Riedel D, et al. Molecular anatomy of a trafficking organelle. Cell. (2006) 127:831–46. doi: 10.1016/j.cell.2006.10.030
84. Janz R, Goda Y, Geppert M, Missler M, Sudhof TC. Sv2a and Sv2b function as redundant Ca2+ regulators in neurotransmitter release. Neuron. (1999) 24:1003–16. doi: 10.1016/S0896-6273(00)81046-6
85. Yao J, Nowack A, Kensel-Hammes P, Gardner RG, Bajjalieh SM. Cotrafficking of Sv2 and synaptotagmin at the synapse. J Neurosci. (2010) 30:5569–78. doi: 10.1523/JNEUROSCI.4781-09.2010
86. Bretin F, Bahri MA, Bernard C, Warnock G, Aerts J, Mestdagh N, et al. Biodistribution and radiation dosimetry for the novel Sv2a Radiotracer [(18)F]Ucb-H: first-in-human study. Mol Imaging Biol. (2015) 17:557–64. doi: 10.1007/s11307-014-0820-6
87. Nabulsi NB, Mercier J, Holden D, Carr S, Najafzadeh S, Vandergeten MC, et al. Synthesis and preclinical evaluation of 11c-Ucb-J as a pet tracer for imaging the synaptic vesicle glycoprotein 2a in the brain. J Nucl Med. (2016) 57:777–84. doi: 10.2967/jnumed.115.168179
88. Finnema SJ, Nabulsi NB, Eid T, Detyniecki K, Lin S-F, Chen M-K, et al. Imaging synaptic density in the living human brain. Sci Transl Med. (2016) 8:348ra96. doi: 10.1126/scitranslmed.aaf6667
89. Naganawa M, Li S, Nabulsi N, Henry S, Zheng MQ, Pracitto R, et al. First-in-human evaluation of (18)F-Synvest-1, a radioligand for pet imaging of synaptic vesicle glycoprotein 2a. J Nucl Med. (2021) 62:561–7. doi: 10.2967/jnumed.120.249144
90. Cai Z, Li S, Zhang W, Pracitto R, Wu X, Baum E, et al. Synthesis and preclinical evaluation of an (18)F-labeled synaptic vesicle glycoprotein 2a pet imaging probe: [(18)F]Synvest-2. ACS Chem Neurosci. (2020) 11:592–603. doi: 10.1021/acschemneuro.9b00618
91. Sadasivam P, Fang XT, Toyonaga T, Lee S, Xu Y, Zheng MQ, et al. Quantification of Sv2a binding in rodent brain using [(18)F]synvest-1 and pet imaging. Mol Imaging Biol. (2021) 23:372–81. doi: 10.1007/s11307-020-01567-9
92. Kokubo Y. The current state of amyotrophic lateral sclerosis/parkinsonism-dementia complex in the Kii Peninsula, Japan, 2019. Brain Nerve. (2019) 71:1236–44. doi: 10.11477/mf.1416201433
93. Shinotoh H, Shimada H, Kokubo Y, Tagai K, Niwa F, Kitamura S, et al. Tau imaging detects distinctive distribution of tau pathology in Als/Pdc on the Kii Peninsula. Neurology. (2019) 92:e136–e47. doi: 10.1212/WNL.0000000000006736
94. Fujishiro H, Hasegawa M, Arai T. The molecular pathology of frontotemporal lobar degeneration. Seishin Shinkeigaku Zasshi. (2010) 112:313–24.
95. Muller HP, Brenner D, Roselli F, Wiesner D, Abaei A, Gorges M, et al. Longitudinal diffusion tensor magnetic resonance imaging analysis at the cohort level reveals disturbed cortical and callosal microstructure with spared corticospinal tract in the Tdp-43 (G298s) Als Mouse Model. Transl Neurodegener. (2019) 8:27. doi: 10.1186/s40035-019-0163-y
96. Kassubek J, Muller HP. Advanced neuroimaging approaches in amyotrophic lateral sclerosis: refining the clinical diagnosis. Expert Rev Neurother. (2020) 20:237–49. doi: 10.1080/14737175.2020.1715798
97. Ciccarelli O, Behrens TE, Johansen-Berg H, Talbot K, Orrell RW, Howard RS, et al. Investigation of white matter pathology in Als and Pls using tract-based spatial statistics. Hum Brain Mapp. (2009) 30:615–24. doi: 10.1002/hbm.20527
98. Filippini N, Douaud G, Mackay CE, Knight S, Talbot K, Turner MR. Corpus callosum involvement is a consistent feature of amyotrophic lateral sclerosis. Neurology. (2010) 75:1645–52. doi: 10.1212/WNL.0b013e3181fb84d1
99. Mori S, van Zijl PC. Fiber tracking: principles and strategies - a technical review. NMR Biomed. (2002) 15:468–80. doi: 10.1002/nbm.781
100. Basaia S, Filippi M, Spinelli EG, Agosta F. White matter microstructure breakdown in the motor neuron disease spectrum: recent advances using diffusion magnetic resonance imaging. Front Neurol. (2019) 10:193. doi: 10.3389/fneur.2019.00193
101. Braak H, Brettschneider J, Ludolph AC, Lee VM, Trojanowski JQ, Del Tredici K. Amyotrophic lateral sclerosis–a model of corticofugal axonal spread. Nat Rev Neurol. (2013) 9:708–14. doi: 10.1038/nrneurol.2013.221
102. Jucker M, Walker LC. Self-propagation of pathogenic protein aggregates in neurodegenerative diseases. Nature. (2013) 501:45–51. doi: 10.1038/nature12481
103. Kassubek J, Muller HP, Del Tredici K, Lulé D, Gorges M, Braak H, et al. Imaging the pathoanatomy of amyotrophic lateral sclerosis in vivo: targeting a propagation-based biological marker. J Neurol Neurosurg Psychiatry. (2018) 89:374–81. doi: 10.1136/jnnp-2017-316365
104. Muller HP, Agosta F, Gorges M, Kassubek R, Spinelli EG, Riva N, et al. Cortico-efferent tract involvement in primary lateral sclerosis and amyotrophic lateral sclerosis: a two-centre tract of interest-based DTI analysis. Neuroimage Clin. (2018) 20:1062–9. doi: 10.1016/j.nicl.2018.10.005
105. Gorges M, Del Tredici K, Dreyhaupt J, Braak H, Ludolph AC, Muller HP, et al. Corticoefferent pathology distribution in amyotrophic lateral sclerosis: in vivo evidence from a meta-analysis of diffusion tensor imaging data. Sci Rep. (2018) 8:15389. doi: 10.1038/s41598-018-33830-z
106. Kassubek J, Muller HP, Del Tredici K, Brettschneider J, Pinkhardt EH, Lulé D, et al. Diffusion tensor imaging analysis of sequential spreading of disease in amyotrophic lateral sclerosis confirms patterns of Tdp-43 pathology. Brain. (2014) 137(Pt 6):1733–40. doi: 10.1093/brain/awu090
107. Rosenbohm A, Muller HP, Hubers A, Ludolph AC, Kassubek J. Corticoefferent pathways in pure lower motor neuron disease: a diffusion tensor imaging study. J Neurol. (2016) 263:2430–7. doi: 10.1007/s00415-016-8281-2
108. Muller HP, Gorges M, Del Tredici K, Ludolph AC, Kassubek J. The same cortico-efferent tract involvement in progressive bulbar palsy and in 'classical' als: a tract of interest-based MRI study. Neuroimage Clin. (2019) 24:101979. doi: 10.1016/j.nicl.2019.101979
109. Rosenbohm A, Del Tredici K, Braak H, Huppertz HJ, Ludolph AC, Muller HP, et al. Involvement of cortico-efferent tracts in flail arm syndrome: a tract-of-interest-based DTI study. J Neurol. (2022) 269:2619–26. doi: 10.1007/s00415-021-10854-6
110. Hannaford A, Pavey N, van den Bos M, Geevasinga N, Menon P, Shefner JM, et al. Diagnostic utility of gold coast criteria in amyotrophic lateral sclerosis. Ann Neurol. (2021) 89:979–86. doi: 10.1002/ana.26045
111. Schuster C, Elamin M, Hardiman O, Bede P. Presymptomatic and longitudinal neuroimaging in neurodegeneration–from snapshots to motion picture: a systematic review. J Neurol Neurosurg Psychiatry. (2015) 86:1089–96. doi: 10.1136/jnnp-2014-309888
112. Agosta F, Pagani E, Petrolini M, Caputo D, Perini M, Prelle A, et al. Assessment of white matter tract damage in patients with amyotrophic lateral sclerosis: a diffusion tensor MR imaging tractography study. Am J Neuroradiol. (2010) 31:1457–61. doi: 10.3174/ajnr.A2105
113. Zhang Y, Schuff N, Woolley SC, Chiang GC, Boreta L, Laxamana J, et al. Progression of white matter degeneration in amyotrophic lateral sclerosis: a diffusion tensor imaging study. Amyotroph Lateral Scler. (2011) 12:421–9. doi: 10.3109/17482968.2011.593036
114. Kwan JY, Meoded A, Danielian LE, Wu T, Floeter MK. Structural imaging differences and longitudinal changes in primary lateral sclerosis and amyotrophic lateral sclerosis. Neuroimage Clin. (2012) 2:151–60. doi: 10.1016/j.nicl.2012.12.003
115. Abhinav K, Yeh FC, El-Dokla A, Ferrando LM, Chang YF, Lacomis D, et al. Use of diffusion spectrum imaging in preliminary longitudinal evaluation of amyotrophic lateral sclerosis: development of an imaging biomarker. Front Hum Neurosci. (2014) 8:270. doi: 10.3389/fnhum.2014.00270
116. Cardenas-Blanco A, Machts J, Acosta-Cabronero J, Kaufmann J, Abdulla S, Kollewe K, et al. Structural and diffusion imaging versus clinical assessment to monitor amyotrophic lateral sclerosis. Neuroimage Clin. (2016) 11:408–14. doi: 10.1016/j.nicl.2016.03.011
117. Broad RJ, Gabel MC, Dowell NG, Schwartzman DJ, Seth AK, Zhang H, et al. Neurite orientation and dispersion density imaging (noddi) detects cortical and corticospinal tract degeneration in Als. J Neurol Neurosurg Psychiatry. (2019) 90:404–11. doi: 10.1136/jnnp-2018-318830
118. Barritt AW, Gabel MC, Cercignani M, Leigh PN. Emerging magnetic resonance imaging techniques and analysis methods in amyotrophic lateral sclerosis. Front Neurol. (2018) 9:1065. doi: 10.3389/fneur.2018.01065
119. Turner MR, Grosskreutz J, Kassubek J, Abrahams S, Agosta F, Benatar M, et al. Towards a neuroimaging biomarker for amyotrophic lateral sclerosis. Lancet Neurol. (2011) 10:400–3. doi: 10.1016/S1474-4422(11)70049-7
120. Filippi M, Agosta F, Grosskreutz J, Benatar M, Kassubek J, Verstraete E, et al. Progress towards a neuroimaging biomarker for amyotrophic lateral sclerosis. Lancet Neurol. (2015) 14:786–8. doi: 10.1016/S1474-4422(15)00134-9
121. Pagani E, Hirsch JG, Pouwels PJ, Horsfield MA, Perego E, Gass A, et al. Intercenter differences in diffusion tensor MRI acquisition. J Magn Reson Imaging. (2010) 31:1458–68. doi: 10.1002/jmri.22186
122. Rosskopf J, Muller HP, Dreyhaupt J, Gorges M, Ludolph AC, Kassubek J. Ex post facto assessment of diffusion tensor imaging metrics from different MRI protocols: preparing for multicentre studies in Als. Amyotroph Lateral Scler Frontotemporal Degener. (2015) 16:92–101. doi: 10.3109/21678421.2014.977297
123. Kalra S, Muller HP, Ishaque A, Zinman L, Korngut L, Genge A, et al. A Prospective harmonized multicenter DTI study of cerebral white matter degeneration in Als. Neurology. (2020) 95:e943–52. doi: 10.1212/WNL.0000000000010235
124. Dadar M, Manera AL, Zinman L, Korngut L, Genge A, Graham SJ, et al. Cerebral atrophy in amyotrophic lateral sclerosis parallels the pathological distribution of Tdp43. Brain Commun. (2020) 2:fcaa061. doi: 10.1093/braincomms/fcaa061
125. Ta D, Ishaque A, Srivastava O, Hanstock C, Seres P, Eurich DT, et al. Progressive neurochemical abnormalities in cognitive and motor subgroups of amyotrophic lateral sclerosis: a prospective multicenter study. Neurology. (2021) 97:e803–13. doi: 10.1212/WNL.0000000000012367
126. Ishaque A, Ta D, Khan M, Zinman L, Korngut L, Genge A, et al. Distinct patterns of progressive gray and white matter degeneration in amyotrophic lateral sclerosis. Hum Brain Mapp. (2022) 43:1519–34. doi: 10.1002/hbm.25738
127. Ta D, Khan M, Ishaque A, Seres P, Eurich D, Yang YH, et al. Reliability of 3d texture analysis: a multicenter MRI study of the brain. J Magn Reson Imaging. (2020) 51:1200–9. doi: 10.1002/jmri.26904
128. Brenner D, Weishaupt JH. Update on amyotrophic lateral sclerosis genetics. Curr Opin Neurol. (2019) 32:735–9. doi: 10.1097/WCO.0000000000000737
129. Agosta F, Ferraro PM, Riva N, Spinelli EG, Domi T, Carrera P, et al. Structural and functional brain signatures of C9orf72 in motor neuron disease. Neurobiol Aging. (2017) 57:206–19. doi: 10.1016/j.neurobiolaging.2017.05.024
130. Floeter MK, Danielian LE, Braun LE, Wu T. Longitudinal diffusion imaging across the C9orf72 clinical spectrum. J Neurol Neurosurg Psychiatry. (2018) 89:53–60. doi: 10.1136/jnnp-2017-316799
131. Floeter MK, Gendron TF. Biomarkers for amyotrophic lateral sclerosis and frontotemporal dementia associated with hexanucleotide expansion mutations in C9orf72. Front Neurol. (2018) 9:1063. doi: 10.3389/fneur.2018.01063
132. Westeneng HJ, Walhout R, Straathof M, Schmidt R, Hendrikse J, Veldink JH, et al. Widespread structural brain involvement in als is not limited to the C9orf72 repeat expansion. J Neurol Neurosurg Psychiatry. (2016) 87:1354–60. doi: 10.1136/jnnp-2016-313959
133. Müller HP, Lule D, Roselli F, Behler A, Ludolph AC, Kassubek J. Segmental involvement of the corpus callosum in C9orf72-associated als: a tract of interest-based DTI study. Ther Adv Chronic Dis. (2021) 12:20406223211002969. doi: 10.1177/20406223211002969
134. Müller H-P, Del Tredici K, Lulé D, Müller K, Weishaupt JH, Ludolph AC, et al. In vivo histopathological staging in C9orf72-associated als: a tract of interest DTI study. NeuroImage Clin. (2020) 27:102298. doi: 10.1016/j.nicl.2020.102298
135. Benatar M, Turner MR, Wuu J. Defining pre-symptomatic amyotrophic lateral sclerosis. Amyotroph Lateral Scler Frontotemporal Degener. (2019) 20:303–9. doi: 10.1080/21678421.2019.1587634
136. Benatar M, Wuu J, McHutchison C, Postuma RB, Boeve BF, Petersen R, et al. Preventing amyotrophic lateral sclerosis: insights from pre-symptomatic neurodegenerative diseases. Brain. (2021) 145:27–44. doi: 10.1093/brain/awab404
137. Lulé DE, Muller HP, Finsel J, Weydt P, Knehr A, Winroth I, et al. Deficits in verbal fluency in presymptomatic C9orf72 mutation gene carriers-a developmental disorder. J Neurol Neurosurg Psychiatry. (2020) 91:1195–200. doi: 10.1136/jnnp-2020-323671
138. Querin G, Bede P, El Mendili MM Li M, Pelegrini-Issac M, Rinaldi D, et al. Presymptomatic spinal cord pathology in C9orf72 mutation carriers: a longitudinal neuroimaging study. Ann Neurol. (2019) 86:158–67. doi: 10.1002/ana.25520
139. Mohammadi B, Kollewe K, Samii A, Krampfl K, Dengler R, Münte TF. Changes of resting state brain networks in amyotrophic lateral sclerosis. Exp Neurol. (2009) 217:147–53. doi: 10.1016/j.expneurol.2009.01.025
140. Fekete T, Zach N, Mujica-Parodi LR, Turner MR. Multiple kernel learning captures a systems-level functional connectivity biomarker signature in amyotrophic lateral sclerosis. PLoS ONE. (2014) 8:e85190. doi: 10.1371/journal.pone.0085190
141. Trojsi F, Esposito F, de Stefano M, Buonanno D, Conforti FL, Corbo D, et al. Functional overlap and divergence between Als and Bvftd. Neurobiol Aging. (2015) 36:413–23. doi: 10.1016/j.neurobiolaging.2014.06.025
142. Douaud G, Filippini N, Knight S, Talbot K, Turner MR. Integration of structural and functional magnetic resonance imaging in amyotrophic lateral sclerosis. Brain. (2011) 134(Pt 12):3470–9. doi: 10.1093/brain/awr279
143. Agosta F, Canu E, Inuggi A, Chiò A, Riva N, Silani V, et al. Resting state functional connectivity alterations in primary lateral sclerosis. Neurobiol Aging. (2014) 35:916–25. doi: 10.1016/j.neurobiolaging.2013.09.041
144. Agosta F, Canu E, Valsasina P, Riva N, Prelle A, Comi G, et al. Divergent brain network connectivity in amyotrophic lateral sclerosis. Neurobiol Aging. (2013) 34:419–27. doi: 10.1016/j.neurobiolaging.2012.04.015
145. Luo C, Chen Q, Huang R, Chen X, Chen K, Huang X, et al. Patterns of spontaneous brain activity in amyotrophic lateral sclerosis: a resting-state Fmri Study. PLoS ONE. (2012) 7:e45470-e. doi: 10.1371/journal.pone.0045470
146. Schulthess I, Gorges M, Müller HP, Lulé D, Del Tredici K, Ludolph AC, et al. Functional connectivity changes resemble patterns of Ptdp-43 pathology in amyotrophic lateral sclerosis. Sci Rep. (2016) 6:38391. doi: 10.1038/srep38391
147. Castelnovo V, Canu E, Calderaro D, Riva N, Poletti B, Basaia S, et al. Progression of brain functional connectivity and frontal cognitive dysfunction in Als. Neuroimage Clin. (2020) 28:102509. doi: 10.1016/j.nicl.2020.102509
148. Agosta F, Valsasina P, Absinta M, Riva N, Sala S, Prelle A, et al. Sensorimotor functional connectivity changes in amyotrophic lateral sclerosis. Cereb Cortex. (2011) 21:2291–8. doi: 10.1093/cercor/bhr002
149. Menke RAL, Proudfoot M, Talbot K, Turner MR. The two-year progression of structural and functional cerebral MRI in amyotrophic lateral sclerosis. Neuroimage Clin. (2018) 17:953–61. doi: 10.1016/j.nicl.2017.12.025
150. Verstraete E, Veldink JH, van den Berg LH, van den Heuvel MP. Structural brain network imaging shows expanding disconnection of the motor system in amyotrophic lateral sclerosis. Hum Brain Mapp. (2014) 35:1351–61. doi: 10.1002/hbm.22258
151. Buchanan CR, Pettit LD, Storkey AJ, Abrahams S, Bastin ME. Reduced structural connectivity within a prefrontal-motor-subcortical network in amyotrophic lateral sclerosis. J Magn Reson Imaging. (2015) 41:1342–52. doi: 10.1002/jmri.24695
152. van der Burgh HK, Westeneng HJ, Walhout R, van Veenhuijzen K, Tan HHG, Meier JM, et al. Multimodal longitudinal study of structural brain involvement in amyotrophic lateral sclerosis. Neurology. (2020) 94:e2592–604. doi: 10.1212/WNL.0000000000009498
153. Schmidt R, de Reus MA, Scholtens LH, van den Berg LH, van den Heuvel MP. Simulating disease propagation across white matter connectome reveals anatomical substrate for neuropathology staging in amyotrophic lateral sclerosis. Neuroimage. (2016) 124(Pt A):762–9. doi: 10.1016/j.neuroimage.2015.04.005
154. Meier JM, van der Burgh HK, Nitert AD, Bede P, de Lange SC, Hardiman O, et al. Connectome-based propagation model in amyotrophic lateral sclerosis. Ann Neurol. (2020) 87:725–38. doi: 10.1002/ana.25706
155. Zhou C, Hu X, Hu J, Liang M, Yin X, Chen L, et al. Altered brain network in amyotrophic lateral sclerosis: a resting graph theory-based network study at voxel-wise level. Front Neurosci. (2016) 10:204. doi: 10.3389/fnins.2016.00204
156. Geevasinga N, Korgaonkar MS, Menon P, Van den Bos M, Gomes L, Foster S, et al. Brain functional connectome abnormalities in amyotrophic lateral sclerosis are associated with disability and cortical hyperexcitability. Eur J Neurol. (2017) 24:1507–17. doi: 10.1111/ene.13461
157. Cividini C, Basaia S, Spinelli EG, Canu E, Castelnovo V, Riva N, et al. Amyotrophic lateral sclerosis-frontotemporal dementia: shared and divergent neural correlates across the clinical spectrum. Neurology. (2021) 98:e402–15. doi: 10.1212/wnl.0000000000013123
158. Basaia S, Agosta F, Cividini C, Trojsi F, Riva N, Spinelli EG, et al. Structural and functional brain connectome in motor neuron diseases: a multicenter MRI study. Neurology. (2020) 95:e2552–64. doi: 10.1212/WNL.0000000000010731
159. Caldwell S, Rothman DL. (1)H Magnetic resonance spectroscopy to understand the biological basis of als, diagnose patients earlier, and monitor disease progression. Front Neurol. (2021) 12:701170. doi: 10.3389/fneur.2021.701170
160. Cheong I, Marjanska M, Deelchand DK, Eberly LE, Walk D, Oz G. Ultra-high field proton MR spectroscopy in early-stage amyotrophic lateral sclerosis. Neurochem Res. (2017) 42:1833–44. doi: 10.1007/s11064-017-2248-2
161. Atassi N, Xu M, Triantafyllou C, Keil B, Lawson R, Cernasov P, et al. Ultra high-field (7tesla) magnetic resonance spectroscopy in amyotrophic lateral sclerosis. PLoS ONE. (2017) 12:e0177680. doi: 10.1371/journal.pone.0177680
162. Foerster BR, Pomper MG, Callaghan BC, Petrou M, Edden RA, Mohamed MA, et al. An imbalance between excitatory and inhibitory neurotransmitters in amyotrophic lateral sclerosis revealed by use of 3-T proton magnetic resonance spectroscopy. JAMA Neurol. (2013) 70:1009–16. doi: 10.1001/jamaneurol.2013.234
163. Weerasekera A, Peeters R, Sima D, Dresselaers T, Sunaert S, De Vocht J, et al. Motor cortex metabolite alterations in amyotrophic lateral sclerosis assessed in vivo using edited and non-edited magnetic resonance spectroscopy. Brain Res. (2019) 1718:22–31. doi: 10.1016/j.brainres.2019.04.018
164. Ratai EM, Alshikho MJ, Zurcher NR, Loggia ML, Cebulla CL, Cernasov P, et al. Integrated imaging of [(11)C]-Pbr28 pet, MR diffusion and magnetic resonance spectroscopy (1)H-Mrs in amyotrophic lateral sclerosis. Neuroimage Clin. (2018) 20:357–64. doi: 10.1016/j.nicl.2018.08.007
165. Bede P. From qualitative radiological cues to machine learning: MRI-based diagnosis in neurodegeneration. Futur Neurol. (2017) 12:5–8. doi: 10.2217/fnl-2016-0029
166. Grollemund V, Pradat PF, Querin G, Delbot F, Le Chat G, Pradat-Peyre JF, et al. Machine learning in amyotrophic lateral sclerosis: achievements, pitfalls, and future directions. Front Neurosci. (2019) 13:135. doi: 10.3389/fnins.2019.00135
167. Kocar TD, Muller HP, Ludolph AC, Kassubek J. Feature selection from magnetic resonance imaging data in als: a systematic review. Ther Adv Chronic Dis. (2021) 12:20406223211051002. doi: 10.1177/20406223211051002
168. Lulé D, Michels S, Finsel J, Braak H, Del Tredici K, Strobel J, et al. Clinicoanatomical substrates of selfish behaviour in amyotrophic lateral sclerosis - an observational cohort study. Cortex. (2022) 146:261–70. doi: 10.1016/j.cortex.2021.11.009
169. Bede P, Hardiman O. Lessons of als imaging: pitfalls and future directions - a critical review. Neuroimage Clin. (2014) 4:436–43. doi: 10.1016/j.nicl.2014.02.011
170. Bede P, Querin G, Pradat PF. The changing landscape of motor neuron disease imaging: the transition from descriptive studies to precision clinical tools. Curr Opin Neurol. (2018) 31:431–8. doi: 10.1097/WCO.0000000000000569
171. Greve DN, Salat DH, Bowen SL, Izquierdo-Garcia D, Schultz AP, Catana C, et al. Different partial volume correction methods lead to different conclusions: an (18)F-Fdg-pet study of aging. Neuroimage. (2016) 132:334–43. doi: 10.1016/j.neuroimage.2016.02.042
172. Hellem MNN, Vinther-Jensen T, Anderberg L, Budtz-Jorgensen E, Hjermind LE, Larsen VA, et al. Hybrid 2-[18f] Fdg Pet/MRI in premanifest huntington's disease gene-expansion carriers: the significance of partial volume correction. PLoS ONE. (2021) 16:e0252683. doi: 10.1371/journal.pone.0252683
173. Kang SK, Lee JS. Anatomy-guided pet reconstruction usingl1bowsher prior. Phys Med Biol. (2021) 66. doi: 10.1088/1361-6560/abf2f7
174. Xie N, Gong K, Guo N, Qin Z, Wu Z, Liu H, et al. Penalized-likelihood pet image reconstruction using 3d structural convolutional sparse coding. IEEE Trans Biomed Eng. (2022) 69:4–14. doi: 10.1109/TBME.2020.3042907
175. Xie Z, Li T, Zhang X, Qi W, Asma E, Qi J. Anatomically aided pet image reconstruction using deep neural networks. Med Phys. (2021) 48:5244–58. doi: 10.1002/mp.15051
176. Wang J, Guo K, Cui B, Hou Y, Zhao G, Lu J. Individual [(18)F]Fdg Pet and functional MRI based on simultaneous Pet/MRI may predict seizure recurrence after temporal lobe epilepsy surgery. Eur Radiol. (2022) 32:3880–8. doi: 10.1007/s00330-021-08490-9
177. Gong K, Catana C, Qi J, Li Q. Direct reconstruction of linear parametric images from dynamic pet using nonlocal deep image prior. IEEE Trans Med Imaging. (2022) 41:680–9. doi: 10.1109/TMI.2021.3120913
178. Poirier SE, Kwan BYM, Jurkiewicz MT, Samargandy L, Steven DA, Suller-Marti A, et al. (18)F-Fdg pet-guided diffusion tractography reveals white matter abnormalities around the epileptic focus in medically refractory epilepsy: implications for epilepsy surgical evaluation. Eur J Hybrid Imaging. (2020) 4:10. doi: 10.1186/s41824-020-00079-7
179. Ladefoged CN, Hansen AE, Henriksen OM, Bruun FJ, Eikenes L, Oen SK, et al. Ai-driven attenuation correction for brain pet/MRI: clinical evaluation of a dementia cohort and importance of the training group size. Neuroimage. (2020) 222:117221. doi: 10.1016/j.neuroimage.2020.117221
180. Tondo G, Esposito M, Dervenoulas G, Wilson H, Politis M, Pagano G. Hybrid Pet- MRI applications in movement disorders. Int Rev Neurobiol. (2019) 144:211–57. doi: 10.1016/bs.irn.2018.10.003
181. Schoenberger M, Schroeder FA, Placzek MS, Carter RL, Rosen BR, Hooker JM, et al. In vivo [(18)F]Ge-179 brain signal does not show nmda-specific modulation with drug challenges in rodents and nonhuman primates. ACS Chem Neurosci. (2018) 9:298–305. doi: 10.1021/acschemneuro.7b00327
182. Wimalarathne DDN, Ruan W, Sun X, Liu F, Gai Y, Liu Q, et al. Impact of tof on brain pet with short-lived (11)C-labeled tracers among suspected patients with Ad/Pd: using hybrid Pet/MRI. Front Med. (2022) 9:823292. doi: 10.3389/fmed.2022.823292
183. Delva A, Van Weehaeghe D, van Aalst J, Ceccarini J, Koole M, Baete K, et al. Quantification and discriminative power of (18)F-Fe-Pe2i pet in patients with parkinson's disease. Eur J Nucl Med Mol Imaging. (2020) 47:1913–26. doi: 10.1007/s00259-019-04587-y
184. Van Weehaeghe D, Koole M, Schmidt ME, Deman S, Jacobs AH, Souche E, et al. [(11)C]Jnj54173717, a Novel P2x7 receptor radioligand as marker for neuroinflammation: human biodistribution, dosimetry, brain kinetic modelling and quantification of brain P2x7 receptors in patients with Parkinson's disease and healthy volunteers. Eur J Nucl Med Mol Imaging. (2019) 46:2051–64. doi: 10.1007/s00259-019-04369-6
185. Emsell L, Laroy M, Van Cauwenberge M, Vande Casteele T, Vansteelandt K, Van Laere K, et al. The leuven late life depression (L3d) study: pet-MRI biomarkers of pathological brain ageing in late-life depression: study protocol. BMC Psychiatry. (2021) 21:64. doi: 10.1186/s12888-021-03063-y
186. Kadrmas DJ, Hoffman JM. Methodology for quantitative rapid multi-tracer pet tumor characterizations. Theranostics. (2013) 3:757–73. doi: 10.7150/thno.5201
Keywords: ALS, motor neuron disease, PET/MRI, DTI, fMRI, SV2A, TrkB/BDNF
Citation: Juengling FD, Wuest F, Kalra S, Agosta F, Schirrmacher R, Thiel A, Thaiss W, Müller H-P and Kassubek J (2022) Simultaneous PET/MRI: The future gold standard for characterizing motor neuron disease—A clinico-radiological and neuroscientific perspective. Front. Neurol. 13:890425. doi: 10.3389/fneur.2022.890425
Received: 05 March 2022; Accepted: 20 July 2022;
Published: 17 August 2022.
Edited by:
Peter Sörös, University of Oldenburg, GermanyReviewed by:
Philippe Corcia, Université de Tours, FranceDongsheng Fan, Peking University Third Hospital, China
Copyright © 2022 Juengling, Wuest, Kalra, Agosta, Schirrmacher, Thiel, Thaiss, Müller and Kassubek. This is an open-access article distributed under the terms of the Creative Commons Attribution License (CC BY). The use, distribution or reproduction in other forums is permitted, provided the original author(s) and the copyright owner(s) are credited and that the original publication in this journal is cited, in accordance with accepted academic practice. No use, distribution or reproduction is permitted which does not comply with these terms.
*Correspondence: Freimut D. Juengling, fjuengli@ualberta.ca