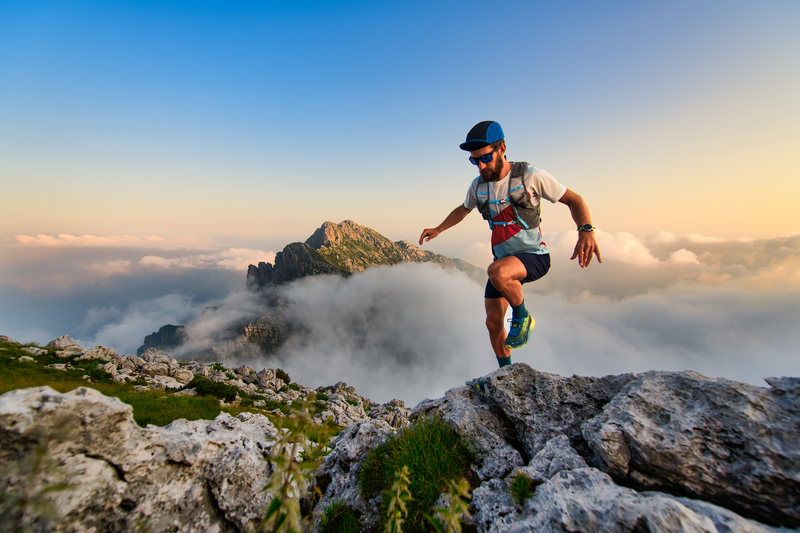
94% of researchers rate our articles as excellent or good
Learn more about the work of our research integrity team to safeguard the quality of each article we publish.
Find out more
ORIGINAL RESEARCH article
Front. Neurol. , 20 June 2022
Sec. Neurological Biomarkers
Volume 13 - 2022 | https://doi.org/10.3389/fneur.2022.889106
This article is part of the Research Topic Potential Biomarkers in Neurovascular Disorders View all 50 articles
Objectives: Ultrasound (US) imaging is a relatively novel strategy to monitor the activity of the blood–brain barrier, which can facilitate the diagnosis and treatment of neurovascular-related metastatic tumors. The purpose of this study was to investigate the clinical significance of applying a combination of US imaging outcomes and the associated genes. This was performed to construct line drawings to facilitate the prediction of brain metastases arising from breast cancer.
Methods: The RNA transcript data from The Cancer Genome Atlas (TCGA) database was obtained for breast cancer, and the differentially expressed genes (DEGs) associated with tumor and brain tumor metastases were identified. Subsequently, key genes associated with survival prognosis were subsequently identified from the DEGs.
Results: Tripartite motif-containing protein 67 (TRIM67) was identified and the differential; in addition, the survival analyses of the TCGA database revealed that it was associated with brain tumor metastases and overall survival prognosis. Applying independent clinical cohort data, US-related features (microcalcification and lymph node metastasis) were associated with breast cancer tumor metastasis. Furthermore, ultrasonographic findings of microcalcifications showed correlations with TRIM67 expression. The study results revealed that six variables [stage, TRIM67, tumor size, regional lymph node staging (N), age, and HER2 status] were suitable predictors of tumor metastasis by applying support vector machine–recursive feature elimination. Among these, US-predicted tumor size correlated with tumor size classification, whereas US-predicted lymph node metastasis correlated with tumor N classification. The TRIM67 upregulation was accompanied by upregulation of the integrated breast cancer pathway; however, it leads to the downregulation of the miRNA targets in ECM and membrane receptors and the miRNAs involved in DNA damage response pathways.
Conclusions: The TRIM67 is a risk factor associated with brain metastases from breast cancer and it is considered a prognostic survival factor. The nomogram constructed from six variables—stage, TRIM67, tumor size, N, age, HER2 status—is an appropriate predictor to estimate the occurrence of breast cancer metastasis.
Brain metastases (BM) have gradually become the most common malignancy of the central nervous system, with 20–40% of patients with cancer developing BM (1, 2). Primarily, BM arises from tumors in the lungs and the breast; however, they are observed at other sites as well (3). Annually, the global incidence of BM has progressively increased and is associated with a poor prognosis. The overall survival varies from 3 to 25 months depending on tumor type and subtype, BM has become a clinically important disease that poses a remarkable threat to human health (2, 4). An estimated 30–50% of patients with metastatic breast cancer (MBC) develop BM (5, 6). The development of BM depends on the action of neurovascular units (NVU), thereby emphasizing the unique and close relationship between the vessels and cells in the brain (7). Brain endothelial cells (BECs) occupy a central position in the NVU and they are regulated by neuronal cells in the formation and maintenance of the blood–brain barrier. Tumor cells must overcome the tightly connected endothelial barrier of BECs to ensure the occurrence of BM (8). Breast cancer (BRCA) is a relatively common type of tumor that leads to the formation of neurovascular metastases and has a very poor prognosis; furthermore, its presence is a notable health risk for patients with cancer (9, 10). Identifying neurovascular metastases from brain tumors is essential for monitoring the prognosis of patients with breast cancer.
Understanding ultrasound (US) signals related to neurovascular tissues in disease conditions will be instrumental in elucidating disease mechanisms and identifying potential diagnostic and treatment targets (11). Ultrasound examinations expose the patient to considerably low levels of radiation, and the test itself is non-invasive and reproducible. Considering this, US examinations are a common and convenient modality in the management of breast cancer (12). Previously, several studies have examined the role of US imaging in the identification of breast cancer metastasis and prognosis. Ultrasound imaging has a suitable degree of sensitivity and specificity in diagnosing breast cancer metastasis to axillary lymph nodes (13). Tumors diagnosed via breast US have better prognostic features in terms of size and lymphovascular invasion (14). Furthermore, the formation of microcalcifications affected the growth and infiltration of breast cancer and is associated with disease prognosis in patients (15). Ultrasonography can provide a multidirectional, multi-angle sweep of microcalcified breast cancer lesions; this feature is more widely used in the screening and diagnosis of microcalcifications in breast cancer (16). In addition, ultrasonographic observation of lesion blood flow grading correlated with positive ER expression of the estrogen receptor, which can indirectly indicate the sensitivity of breast cancer to treatment (17, 18). However, neurovascular metastases in breast cancer were closely related to gene expression characteristics, and the items examined via breast US imaging alone were biased as they do not adequately reflect this.
The recent developments in bioinformatics have facilitated the diagnosis and treatment of the disease with the help of large-scale biological data (19–22). The considerable amount of RNA sequencing data and the increasing number of prognostic factors and therapeutic targets identified have considerably aided in the examination of the mechanisms related to cancer development and clinical treatment (23, 24). One study used RNA sequencing to probe the cellular origin and evolution of breast cancer in BRCA1 mutant carriers (25). Additionally, bioinformatics has reportedly been used to identify differentially expressed genes (DEGs) associated with breast cancer metastasis and provide potential therapeutic targets for metastatic breast cancer (26). Bioinformatics data combined with imaging findings can also play a crucial role in breast cancer diagnosis and prognosis. Breast US imaging combined with plasma miR-21 and miR-27a significantly improves the diagnostic efficiency of breast cancer, thereby facilitating early clinical intervention (27). We believe that a combination of US imaging and transcriptional profiling can predict the risk of cerebral neurovascular metastases in patients with breast cancer.
In this study, we aimed to investigate the genes associated with the development of neurovascular BM in patients with breast cancer and to explore the role of ultrasonography in the examination of tumor metastases. Furthermore, this was performed to identify potential diagnostic and therapeutic targets/features to monitor and improve the risk of BM in patients with breast cancer and to extend their life expectancy.
We retrospectively analyzed patients with surgically confirmed breast cancer who presented themselves to our hospital between August 2015 and March 2021. The following cases were excluded in this study: (1) cases of male breast cancer; (2) cases where the elapsed time between surgery and US was more than 2 weeks; (3) cases with incomplete clinical information; (4) cases with unilateral multiple foci; and (5) cases with primary malignancy at a different site. Using the above exclusion criteria, a total of 97 breast cancer patients with adequate follow-up were finally screened. Postoperative pathological staging was based on the American Joint Committee on Cancer (AJCC) TNM staging criteria for breast cancer. Informed consent was obtained from all patients. Consent was also obtained from the Ethics Committee of Cangzhou Central Hospital. The Cancer Genome Atlas (TCGA; https://portal.gdc.cancer.gov/) project database was searched for 1,041 breast invasive carcinoma (BRCA) patient samples consisting of 60 tumors with non-brain metastasis (nBM–BRCA), 4 tumors with brain metastasis (BM–BRCA), and 977 tumors without distant brain metastasis (nDM–BRCA). Transcriptome data were downloaded in transcripts per million (TPM) unit format and were log-transformed.
Sonography was performed using a Siemens-2000 color Doppler diagnostic US machine with a probe frequency of 7–15 Hz. Different clinical features were analyzed using US including tumor size, microcalcification status, blood flow grading, and lymph node metastases. The longest measured diameter of a lesion in any section scan was considered as the size of the primary tumor. The size (<1-mm in diameter), number, and distribution of calcified spots were used as bases to determine the status of microcalcifications in breast cancer. Blood flow was graded into four levels according to Adler blood flow grading: Grade 0, no blood flow in the tumor lesion; Grade I, little blood flow, i.e., one or two spots of blood flow (<1-mm in diameter) are visible; Grade II, moderate blood flow, i.e., one main vessel or several small vessels visible; Grade III, significant blood flow, i.e., four or more vessels visible (28). Ultrasound was used to assess axillary lymph node metastases by (1) high-frequency US sonograph showing a lymph node thickness of more than 3 mm or a solid axillary hypoechogenicity, or (2) color flow imaging showing abundant blood flow within the lymph nodes.
To explore the relationship between tumor features and metastasis, logistics regression was used to determine the predictive power of the observed tumor features from the sonographs in relation to tumor metastasis (29, 30). The purpose of using logistic regression in this step is not to train diagnostic classifiers, but to test for multivariate differences. First, univariate logistics regression was used to analyze the general clinical and US-related features of the patients, with the relevant characteristics screened at p < 0.05 level of significance. Then, the screened US and clinical characteristics were subjected to multivariate logistic regression analysis. The odds ratio (OR) and 95% confidence interval (95% CI) for each variable were assessed as statistical indicators. The variables with a significance level of p < 0.05 in both univariate and multifactorial logistic regression analyses were considered as independent predictors of tumor metastasis.
Distant metastases- and BM-associated differentially expressed genes (DEGs) were identified through the differential analysis of transcriptomic data from tumor tissue samples using the limma package (31). The DEGs were filtered based on a threshold value of p < 0.05. Subsequently, intersection analysis of DM- and BM-associated DEGs was performed to identify genes that were differentially expressed in both DM and BM. From the obtained gene list, survival analysis was employed to further screen for prognosis-related genes.
Quantitative RT-PCR (qRT-PCR) was used to quantify the RNA expression levels of key genes present in tumor samples collected after surgical resection. The total RNA was extracted using the mirVana™ miRNA isolation kit (Ambion) according to the manufacturer's protocol. The harvested total RNA was reverse transcribed to cDNA using RevertAid first strand cDNA synthesis kit (Thermo Scientific™, USA). The qRT-PCR was performed using SYBR® Green Realtime PCR master mix (Toyobo, Japan) on ABI 7500 real-time PCR system (Applied Biosystems, CA, USA). Using GAPDH as the reference gene, the 2−ΔΔCT method was used for the relative quantification of gene expression levels. In the final conclusion, gene expression levels were reported as the fold-change (FC) relative to the reference.
To screen for features associated with tumor metastases, an exploratory analysis of the patient's general characteristics, US-related features, postoperative pathological features and key genes was applied support vector machine–recursive feature elimination (SVM–RFE) (32). In supervised machine learning, support vector machines (SVMs) are often used for non-linear classification. In addition, SVM showed good performance in processing data sets with small sample sizes (30, 33, 34). Support vector machine–recursive feature elimination filtered the variables of the prediction results by iteratively removing redundant feature variables. Using a cross-validation approach, the root mean squared error (RMSE) parameter was used to assess the predictive performance of the model and to screen for the best combination of variables. The entire dataset was divided into a training set and a test set in a ratio of 7:3. The training set was used for feature selection and model training, and the test set was used for testing the model. The area under curve (AUC) of the receiver operating characteristic curve (ROC) was also applied to assess the predictive performance of the model; pROC curves are plotted using the “pROC” package.
A principal component analysis (PCA) was applied to further extract features from the SVM–RFE that reflect tumor metastasis in order to determine whether they provided adequate information for classification. To develop predictive models for these characteristics, multivariate logistic regression was applied. Decision curve analysis (DCA) assesses the usefulness and safety of clinical prediction models by calculating the net benefit ratio for different threshold probabilities. To facilitate clinical use, a visualization tool was constructed by applying the “rms” to the nomogram of this clinical prediction model. Calibration curves are used to assess the agreement between predicted probabilities and actual outcomes.
For BRCA patients, Kaplan–Meier (KM) curves were used to calculate the probability of overall survival (OS) at 1, 3, and 5 years. Cox regression analysis was used to perform a difference-in-difference test analysis of the survival curves between the two groups. The time-dependent ROC and area under the curve (AUC) values were used to quantify the predictive performance of the target gene for survival in BRCA patients (35). Survival data were analyzed using “survival” package, and visualization was carried out using survminer R package.
Analysis of functional pathways dysregulated in TCGA–BRCA tumor samples with dysregulated genes using Gene Set Enrichment Analysis (GSEA) as the previous researches (36–39). The pathways in the analysis were annotated using the “c2.cp.v7.2.symbols.gmt [Curated]” gene set from MSigDB Collections (https://www.gsea-msigdb.org/gsea/msigdb/). Package clusterProfiler was used to calculate the statistical significance of the enrichment of functional pathways (40). The threshold of statistical significance for functional pathway enrichment was set at adjusted p < 0.05.
All statistical analyses and figures were obtained using the R (version 4.0.2) software. In the statistical analysis of patients' baseline data, the Pearson's Chi-squared test or Fisher's exact test was used to test the count data. Differences in the measurements between the two groups were determined using the Wilcoxon rank–sum test or unpaired t-test. Correlation analysis was performed using the Spearman method. The results from these statistical analyses were plotted using the “ggplot2” package. Computed p-values of <0.05 were considered to be statistically significant.
Among the 97 patients with breast cancer enrolled in the clinical cohort study, distant metastases (DM) developed and did not develop in 34 and 63, respectively. The clinical baseline information, postoperative pathological features, and US features of the patients with breast cancer are summarized in Table 1. Patients with breast cancer with and without DM showed significant differences in terms of TNM stage, metastasized staging (M), tumor size staging (T), regional lymph node staging (N), HER2, tumor size, microcalcification, Adler blood flow grading, and prediction of lymph node metastasis. No statistically significant differences were observed in terms of age, menopausal status, tumor location, histology, ER, and PR. However, microcalcifications confirmed by preoperative US, higher blood flow grading, and lymph node metastases may indicate a higher risk of DM.
Typically, the logistics model is used to screen for risk factors associated with the poor outcomes. In this section, we have included only general clinical features and ultrasonography-related features for analysis to explore the ability of preoperative ultrasonography and diagnosis to assess the likelihood of DM in patients with tumors. Results of logistic regression analysis to screen the predictors associated with DM are presented in Table 2. Univariate logistic analysis showed that tumor size in US imaging (p = 0.015), microcalcification (p < 0.001), Adler blood flow grading (p = 0.003), and lymph node metastasis (p = 0.001) were significantly associated with the occurrence of DM in patients with breast cancer. Further multivariate logistic regression analysis showed that microcalcification (p = 0.02) and lymph node metastasis (p = 0.011) were independent predictors of DM.
We performed differential expression analysis of the RNA transcript data between the two groups at the tumor tissue level. Differential analysis of The Cancer Genome Atlas (TCGA) data and patients with breast cancer with and without DM revealed 3,759 DEGs, which were identified as DM-associated DEGs (Figure 1A). Similarly, 2,029 BM-associated DEGs were identified between the BM and nBM groups (Figure 1B), and Cox analysis revealed 1,727 survival-associated DEGs. Cross-tabulation analysis subsequently showed that four genes—TRIM67, SFXN2, CEL, and IGKV6-21—were associated with DM, BM, and survival outcomes. Additionally, TRIM67 expression was significantly different between the DM and nDM groups (p = 0.021) and between the BM and nBM groups (p = 0.007) (Figures 1C,D). Therefore, TRIM67 was used for further analysis.
Figure 1. Analysis of variance showed that TRIM67 was upregulated in both DM and BM. (A) Volcano plot showing differentially expressed genes between the DM and nDM groups, where TRIM67 was found to be upregulated in DM; (B) Differential expression analysis between BM and nBM groups showed that TRIM67 was upregulated in BM; (C) There was a statistically significant difference in TRIM67 expression between the DM and nDM groups (p = 0.021); (D) There was a statistically significant difference in TRIM67 expression between the BM and nBM groups (p = 0.007).
To investigate whether US can assess TRIM67 expression, an exploratory analysis of the relationship between US findings and TRIM67 was performed. The results showed a significant difference between microcalcifications and TRIM67 expression in the US findings (p = 0.027). The TRIM67 expression was higher in the group with microcalcifications than in the group without microcalcifications (Figure 2A). the TRIM67 expression showed no significant differences from Adlerian blood flow grading, lymph node metastasis prediction, and tumor size (Figures 2B–D). However, the results appeared to imply that a higher blood flow grading, positive lymph node metastasis prediction, and larger tumor size correlated with a higher TRIM67 expression.
Figure 2. Relationship between US-related features and TRIM67 expression. (A) Microcalcifications in tumor tissue correlated with TRIM67 expression (p = 0.027); (B,C) The bar graph shows the relationship between Adler blood flow grading and lymph node metastasis in relation to differential expression of TRIM67; (D) Correlation analysis of tumor size on US and TRIM67 expression.
The general patient characteristics, the ultrasonographic information, the postoperative pathological information, and the predictive power of TRIM67 in tumor metastasis were assessed using SVM–RFE. The results showed that the model had the lowest RMSE (Figure 3A) when six variables (stage, TRIM67, tumor size, N, age, and HER2 status) were included in the SVM model. The receiver operating characteristic (ROC) curves of TRIM67 [area under ROC curve (AUC), 0.643], tumor size (AUC, 0.654), and age (AUC, 0.580) for the prediction of tumor metastasis are shown in Figure 3B, indicating their general predictive ability. The AUCs of the multifactor logistic regression model constructed by combining the above six characteristics were 0.917, 0.865, and 0.912 for the training group, test group, and all groups, respectively (Figure 3C). The clinical prediction model showed an acceptable predictive power for tumor metastasis. Table 3 shows the coefficients for these six variables in the clinical prediction model. A positive correlation was found between the probability of tumor metastasis and stages III–IV, TRIM67, size, N1–N3, and positive HER2. The DCA of the clinical prediction model in the training, test, and overall groups is shown in Figure 3D, demonstrating the large clinical range of the model.
Figure 3. The training process and performance evaluation of SVM-RFE models. (A) The variable screening process showed the smallest RMSE for the model when six variables were selected; (B) The ROCs show the predictive power of TRIM67, tumor size, and age on tumor metastasis in a selection of six variables; (C) The ROC shows the predictive power of a logistic regression model combining six indicators for tumor metastasis; (D) The DCA suggested that the clinical prediction model has a good range of clinical use in the training, test, and overall groups.
Developing a predictive model has the potential to be useful in clinical practice is our aim. First, the correction curves showed a good degree of agreement between the predicted outcomes of this constructed clinical prediction model and the patients' perceptions of the true outcomes (Figure 4A). Subsequently, the PCA analysis showed that patients with breast cancer with and without tumor metastases could be differentiated according to the variables used in the model (Figure 4B). Ultimately, based on a multivariate logistic regression model, the nomogram was prepared to facilitate its use in clinical settings (Figure 4C). Nomograms are composed of selected feature variables and their corresponding scores. The total score was obtained by summing the corresponding scores for each variable before converting it directly to obtain the probability of tumor metastasis. Therefore, the nomogram ensures that a patient's likelihood of developing metastases can easily be assessed by using important clinical features, preoperative US findings, and postoperative pathological information.
Figure 4. Construction of a clinical prediction model for tumor metastasis. (A) The correction curves show that the predicted outcomes of this clinical prediction model are in good agreement with the true outcomes of the patients; (B) The PCA visualization demonstrates the efficacy of using the six variables to differentiate patients with breast cancer who developed tumor metastases; (C) The nomogram, constructed based on a clinical prediction model, allows an assessment of the likelihood of tumor metastasis based on these six variables.
Variables in the clinical prediction model have been discussed further in this section. The tumor size observed on US examinations appeared to correlate with the T stage in the TNM classification (Figure 5A). Furthermore, the distribution of N grades in the TNM grading showed significant differences between the US-predicted lymph node metastasis group and the US-predicted non-lymphoid node metastasis group (p < 0.05, Figure 5B). These results demonstrated that, in the clinical prediction model, the tumor size measured using US reflects the T-staging of the tumor, whereas the N-staging corresponds to the lymph node metastasis predicted by US. This illustrated the important role of ultrasonography in predicting the development of tumor metastases in patients with breast cancer. In addition, normal and tumor tissues showed different TRIM67 expression levels (Figure 5C). Additionally, significant differences in TRIM67 expression were identified between T1, T2, T3, and T4 (Figure 5D). Therefore, T staging can reflect TRIM67 expression to some extent. The abovementioned results demonstrated the complex interrelationship between ultrasonography-related features, postoperative pathological grading, and TRIM67 expression.
Figure 5. Relationship between preoperative ultrasonography and TRIM67 and postoperative pathological features. (A) The bar chart shows a correlation between the T-grading of the tumor and the size of the tumor as measured using preoperative US; (B) There was a correlation between US-predicted lymph node metastasis and the N grade of the tumor; (C) There were differences in TRIM67 expression between normal and tumor tissues; (D) There were differences in TRIM67 expression between different tumor T-grades.
The KM curve for TRIM67 is shown in Figure 6A. Median overall survival time from the initial diagnosis of breast cancer was significantly shorter in the high TRIM67 group than in the low TRIM67 group (9.6 vs. 12.2 years, respectively, p = 0.031). Univariate Cox analysis showed a statistical difference between TRIM67 expression and survival outcome (p = 0.032, HR = 1.42, 95% CI: 1.03–1.95). The ROC curves showed that the predicted AUC of TRIM67 for overall survival at 1, 3, and 5 years were 0.543, 0.570, and 0.556, respectively (Figure 6B). These results suggested that elevated TRIM67 was associated with a poor prognosis in patients with breast cancer.
Figure 6. Survival analysis and GSEA for TRIM67. (A) KM curves showed significant differences (p = 0.032) in survival curves between high and low TRIM67 groups for patients with breast cancer; (B) ROC shows the predictive power of TRIM67 for overall survival at 1, 3, and 5 years; (C) GSEA shows the functional pathways that are enriched when TRIM67 is upregulated.
To further explore the possible functional pathways involved in TRIM67 in BM in patients with breast cancer, we used RNA sequencing data from patients with breast cancer who developed distant tumor metastasis in TCGA for Gene Set Enrichment Analysis (GSEA). The results showed that in TRIM67-upregulated tumors, the integrated breast cancer pathway was upregulated, whereas the miRNA targets in ECM and membrane receptors and miRNAs involved in DNA damage response pathways were downregulated (Figure 6C). The TRIM67 upregulation appeared to accompany the upregulation of the pathways related to breast cancer. In addition, TRIM67 upregulation may be associated with the downregulation of certain miRNA-regulated functional pathways, including the role of membrane receptors targeted by miRNAs and their response in the DNA damage response. Alterations in these pathways may be associated with the development of BM in patients with breast cancer, and ultimately affect patient OS.
Ultrasound imaging is a new strategy for monitoring the activity of the blood–brain barrier, which can facilitate the diagnosis and treatment of metastatic tumors related to neurovascular tissues. In this study, we explored the possible role of US as well as TRIM67 in the development of BM in breast cancer through a retrospective clinical cohort study and bioinformatics analysis. The TRIM67 was identified as a factor associated with neurovascular metastasis, tumor metastasis, and prognostic survival in brain tumors in bioinformatics analysis of TCGA and breast cancer.
The TRIM67 expression was correlated with microcalcifications on ultrasonography. Following this, we incorporated TRIM67 expression data, general clinical information, postoperative pathology, and US-related characteristics and screened six variables (stage, TRIM67, tumor size, N, age, and HER2 status) with the SVM–RFE method, which could predict the likelihood of tumor metastasis. Of these six features, tumor size measured using US correlates with the T classification of the tumor, whereas lymph node metastasis was predicted by US correlated with the N classification.
In this study, TRIM67 was upregulated in patients with BM from breast cancer and DM. The previous studies on TRIM67 in tumors have demonstrated that the TRIM67 protein functions differently in different tumors. The TRIM67 was reportedly downregulated in colorectal cancer and TRIM67 inhibited tumor proliferation and metastasis (41, 42). The TRIM67 promoted the progression of non-small cell lung cancer through the Notch pathway, including promoting cell proliferation, migration, and EMT (43). However, upregulation of TRIM67 expression increased apoptosis in non-small cell lung cancer cells (44). Although the relationship between TRIM67 and tumors remains unclear, all studies mentioned above reported that TRIM67 was associated with tumor size, lymph node metastasis, tumor stage, and disease prognosis (41–43). Similar to the previous studies, we observed that TRIM67 was associated with BM in breast cancer and was a risk factor for a poor prognosis. Microcalcifications on US findings correlated with TRIM67 expression, and a higher blood flow grade, positive prediction of lymph node metastasis, and larger tumor size corresponded with higher TRIM67 expression. In TRIM67 upregulated tumors, the integrated breast cancer pathway was upregulated, whereas miRNA targets in ECM and membrane receptors and the miRNAs involved in DNA damage response pathways were downregulated. Therefore, these pathways may play an important role in the metastasis of breast cancer.
Microcalcification is an important early sign of breast cancer and its early diagnosis depends on the identification of suspected microcalcifications (45–47). The morphology and distribution of microcalcifications can be used to differentiate between benign and malignant lesions (48, 49). The previous studies have observed the presence of an association between microcalcifications and lymph node metastasis and prognosis in breast cancer (50, 51). BMP-2 is closely associated with microcalcification (50). The BMP signaling affects the invasiveness of breast cancer tumor cells (52). Similar to the previous studies, we observed that microcalcification and lymph node metastasis measured using US examinations were independent risk factors for distant tumor metastasis based on preoperative US. Ultrasound has been widely used to detect lymph node metastases in breast cancer. Ultrasound and needle biopsy of suspected lymph nodes could effectively identify the presence of lymph node metastases from breast cancer (53). Therefore, it is our understanding that microcalcification and lymph node metastasis play an important role in predicting breast cancer metastasis. In this study, TRIM67 was associated with tumor microcalcification, and ultrasonography may reflect changes in TRIM67 to some extent by monitoring the status of microcalcifications. The TRIM67 expression is associated with DM in breast cancer; therefore, US combined with examination of TRIM67 expression may have a better predictive function in breast cancer metastases.
The six variables screened by SVM–RFE were used to construct clinical prediction models. Using the nomogram, we can easily assess the likelihood of tumor metastasis in patients with breast cancer using important clinical features, preoperative US findings, and postoperative pathological information. Tumor size measured via US examination reflects the T-grade of the tumor, whereas the N-grade corresponds to the lymph node metastases predicted by US. Similar findings have been observed in the previous studies on the relationship between US and clinicopathological features of breast cancer. Li et al. reported that the pathological grading of breast cancer correlated with the shape of the tumor measured using US (54). Lamb et al. found that the grade and infiltration of breast cancer correlated with the echogenicity and tumor margin measured by US (55). This highlighted the important role of ultrasonography in predicting the development of tumor metastases in patients with breast cancer.
Upregulation of TRIM67 was accompanied by upregulation of the pathways associated with breast cancer. In addition, the upregulation of TRIM67 may be associated with the downregulation of certain miRNA-regulated functional pathways, including the role of membrane receptors targeted by miRNAs and response in the DNA damage response. Alterations in these pathways may be associated with the development of BM in patients with breast cancer and ultimately affect their OS. In the future, further in-depth studies are warranted to address the relationship between the role of US and related molecules in breast cancer.
There are some limitations to this study. Patients with BM from breast cancer were not compared to patients without BM as a separate group in the clinical study population. We aim to expand the sample size for further detailed analysis in the future. Additionally, we will further investigate the mechanism of TRIM67 in breast cancer metastasis at the basic experimental level.
Several risk factors were examined in this study associated with BRCA brain metastases. Ultrasound and its prediction of TRIM67 play an important predictive role in neurovascular-related metastases from breast cancer. The nomogram of six variables—stage, TRIM67, tumor size, N, age, and HER2 status—were biomarkers of the likelihood of breast cancer metastasis.
The original contributions presented in the study are included in the article/supplementary material, further inquiries can be directed to the corresponding authors.
The studies involving human participants were reviewed and approved by the Ethics Committee of Cangzhou Central Hospital. The patients/participants provided their written informed consent to participate in this study.
ZX, TM, YQ, and YG performed the data curation and analysis. ZX and TM analyzed and interpreted the results. ZX drafted and reviewed the manuscript. TM provided funding. All authors read and approved the final manuscript.
The authors declare that the research was conducted in the absence of any commercial or financial relationships that could be construed as a potential conflict of interest.
All claims expressed in this article are solely those of the authors and do not necessarily represent those of their affiliated organizations, or those of the publisher, the editors and the reviewers. Any product that may be evaluated in this article, or claim that may be made by its manufacturer, is not guaranteed or endorsed by the publisher.
The authors wish to thank all patients and staff who participated in this study.
TCGA, The Cancer Genome Atlas; BRCA, Breast invasive carcinoma; BM, Brain metastasis; nDM–BRCA, BRCA with no distance metastasis; nBM–BRCA, BRCA with non-brain metastasis; TPM, Transcripts per million; DE–mRNAs, Differentially expressed mRNAs; DEGs, Differentially expressed genes; GSEA, Gene Set Enrichment Analysis; KM, Kruskal–Wallis; OS, Overall survival; qRT-PCR, Quantitative Reverse Transcription-PCR.
1. Badakhshi H, Engeling F, Budach V, Ghadjar P, Zschaeck S, Kaul D. Are prognostic indices for brain metastases of melanoma still valid in the stereotactic era? Radiat Oncol. (2018) 13:3. doi: 10.1186/s13014-017-0951-4
2. Kotecki N, Lefranc F, Devriendt D, Awada A. Therapy of breast cancer brain metastases: challenges, emerging treatments and perspectives. Ther Adv Med Oncol. (2018) 10:175883591878031. doi: 10.1177/1758835918780312
3. Tao J, Gao Z, Huang R, Li H. Therapeutic effect of combined hyperbaric oxygen and radiation therapy for single brain metastasis and its influence on osteopontin and MMP-9. Exp Ther Med. (2018) 17:465–471. doi: 10.3892/etm.2018.6930
4. Taillibert S, Le Rhun É. Épidémiologie des lésions métastatiques cérébrales. Cancer Radiothér. (2015) 19:3–9. doi: 10.1016/j.canrad.2014.11.001
5. Garcia-Alvarez A, Papakonstantinou A, Oliveira M. Brain metastases in HER2-positive breast cancer: current and novel treatment strategies. Cancers. (2021) 13:2927. doi: 10.3390/cancers13122927
6. Brosnan EM, Anders CK. Understanding patterns of brain metastasis in breast cancer and designing rational therapeutic strategies. Ann Transl Med. (2018) 6:163. doi: 10.21037/atm.2018.04.35
7. Iadecola C. The neurovascular unit coming of age: a journey through neurovascular coupling in health and disease. Neuron. (2017) 96:17–42. doi: 10.1016/j.neuron.2017.07.030
8. Haskó J, Fazakas C, Molnár K, Mészáros Á, Patai R, Szabó G, et al. Response of the neurovascular unit to brain metastatic breast cancer cells. Acta Neuropathol Commun. (2019) 7:133. doi: 10.1186/s40478-019-0788-1
9. Arnold J, Schattschneider J, Blechner C, Krisp C, Schlüter H, Schweizer M, et al. Tubulin Tyrosine Ligase Like 4 (TTLL4) overexpression in breast cancer cells is associated with brain metastasis and alters exosome biogenesis. J Exp Clin Cancer Res. (2020) 39:205. doi: 10.1186/s13046-020-01712-w
10. Patil A, Sherbet GV. Therapeutic approach to the management of HER2-positive breast cancer metastatic to the brain. Cancer Lett. (2015) 358:93–9. doi: 10.1016/j.canlet.2014.12.026
11. Liebner S, Dijkhuizen RM, Reiss Y, Plate KH, Agalliu D, Constantin G. Functional morphology of the blood–brain barrier in health and disease. Acta Neuropathol. (2018) 135:311–36. doi: 10.1007/s00401-018-1815-1
12. Wu G-G, Zhou L-Q, Xu J-W, Wang J-Y, Wei Q, Deng Y-B, et al. Artificial intelligence in breast ultrasound. WJR. (2019) 11:19–26. doi: 10.4329/wjr.v11.i2.19
13. Zhang Y-N, Wang C-J, Xu Y, Zhu Q-L, Zhou Y-D, Zhang J, et al. Sensitivity, specificity and accuracy of ultrasound in diagnosis of breast cancer metastasis to the axillary lymph nodes in Chinese patients. Ultrasound Med Biol. (2015) 41:1835–41. doi: 10.1016/j.ultrasmedbio.2015.03.024
14. Gutierrez Blanco C, Evans EB, Porter AJ. Breast cancer diagnosis in a specialised breast clinic: are cancers detected by ultrasound alone less aggressive? J Med Imaging Radiat Oncol. (2019) 63:40–6. doi: 10.1111/1754-9485.12826
15. Alsheh Ali M, Eriksson M, Czene K, Hall P, Humphreys K. Detection of potential microcalcification clusters using multivendor for-presentation digital mammograms for short-term breast cancer risk estimation. Med Phys. (2019) 46:1938–46. doi: 10.1002/mp.13450
16. Relea A, Alonso JA, González M, Zornoza C, Bahamonde S, Viñuela BE, et al. Utilidad del artefacto Doppler de centelleo de color (twinkle) en la detección ecográfica de microcalcificaciones mamarias. Radiología. (2018) 60:413–23. doi: 10.1016/j.rx.2018.04.004
17. Zhao X, Yang X, Fu L, Yu K. Associations of estrogen receptor, progesterone receptor, human epidemic growth factor Receptor-2 and Ki-67 with ultrasound signs and prognosis of breast cancer patients. CMAR. (2021) 13:4579–86. doi: 10.2147/CMAR.S276422
18. Yan J, Liu X-L, Han L-Z, Xiao G, Li N-L, Deng Y-N, et al. Relation between Ki-67, ER, PR, Her2/neu, p21, EGFR, and TOP II-α expression in invasive ductal breast cancer patients and correlations with prognosis. Asian Pac J Cancer Prev. (2015) 16:823–9. doi: 10.7314/APJCP.2015.16.2.823
19. Canzoneri R, Lacunza E, Abba MC. Genomics and bioinformatics as pillars of precision medicine in oncology. Medicina. (2019) 79:587–92.
20. Kang X, Chen Y, Yi B, Yan X, Jiang C, Chen B, et al. An integrative microenvironment approach for laryngeal carcinoma: the role of immune/methylation/autophagy signatures on disease clinical prognosis and single-cell genotypes. J Cancer. (2021) 12:4148–71. doi: 10.7150/jca.58076
21. Sun C, Ma S, Chen Y, Kim NH, Kailas S, Wang Y, et al. Diagnostic value, prognostic value, and immune infiltration of LOX family members in liver cancer: bioinformatic analysis. Front Oncol. (2022) 12:843880. doi: 10.3389/fonc.2022.843880
22. Lois H. New advances in immunotherapy for prostate cancer. Life Res. (2019) 2:152. doi: 10.53388/life2019-1025-302
23. Du Y, Miao W, Jiang X, Cao J, Wang B, Wang Y, et al. The epithelial to mesenchymal transition related gene calumenin is an adverse prognostic factor of bladder cancer correlated with tumor microenvironment remodeling, gene mutation, and ferroptosis. Front Oncol. (2021) 11:683951. doi: 10.3389/fonc.2021.683951
24. An N, Yang X. Identification of prognostic genes through expression differentiation during metastatic process in lung adenocarcinoma. Sci Rep. (2017) 7:11119. doi: 10.1038/s41598-017-11520-6
25. Hu L, Su L, Cheng H, Mo C, Ouyang T, Li J, et al. Single-cell RNA sequencing reveals the cellular origin and evolution of breast cancer in BRCA1 mutation carriers. Cancer Res. (2021) 81:2600–11. doi: 10.1158/0008-5472.CAN-20-2123
26. Wang L, Mo C, Wang L, Cheng M. Identification of genes and pathways related to breast cancer metastasis in an integrated cohort. Eur J Clin Invest. (2021) 51:e13525. doi: 10.1111/eci.13525
27. Li M, Li Q, Yin Q, Wang Y, Shang JM, Wang LH. Evaluation of color Doppler ultrasound combined with plasma miR-21 and miR-27a in the diagnosis of breast cancer. Clin Transl Oncol. (2021) 23:709–17. doi: 10.1007/s12094-020-02501-9
28. Adler DD, Carson PL, Rubin JM, Quinn-Reid D. Doppler ultrasound color flow imaging in the study of breast cancer: Preliminary findings. Ultrasound Med Biol. (1990) 16:553–9. doi: 10.1016/0301-5629(90)90020-D
29. Min Y, Huang Y, Wei M, Wei X, Chen H, Wang X, et al. Preoperatively predicting the central lymph node metastasis for papillary thyroid cancer patients with Hashimoto's Thyroiditis. Front Endocrinol. (2021) 12:713475. doi: 10.3389/fendo.2021.713475
30. Ying C, Guo C, Wang Z, Chen Y, Sun J, Qi X, et al. A prediction modeling based on the Hospital for Special Surgery (HSS) knee score for poor postoperative functional prognosis of elderly patients with patellar fractures. Biomed Res Int. (2021) 2021:6620504. doi: 10.1155/2021/6620504
31. Ritchie ME, Phipson B, Wu D, Hu Y, Law CW, Shi W, et al. limma powers differential expression analyses for RNA-sequencing and microarray studies. Nucleic Acids Res. (2015) 43:e47. doi: 10.1093/nar/gkv007
32. Huang M-L, Hung Y-H, Lee WM, Li RK, Jiang B-R. SVM-RFE based feature selection and Taguchi parameters optimization for multiclass SVM classifier. ScientificWorldJournal. (2014) 2014:795624. doi: 10.1155/2014/795624
33. Bhadra S, Kumar CJ. An insight into diagnosis of depression using machine learning techniques: a systematic review. Curr Med Res Opin. (2022) 38:749–71. doi: 10.1080/03007995.2022.2038487
34. Hu C, Li Q, Shou J, Zhang F-X, Li X, Wu M, et al. Constructing a predictive model of depression in chemotherapy patients with Non-Hodgkin's Lymphoma to improve medical staffs' psychiatric care. Biomed Res Int. (2021) 2021:9201235. doi: 10.1155/2021/9201235
35. Blanche P, Dartigues J-F, Jacqmin-Gadda H. Estimating and comparing time-dependent areas under receiver operating characteristic curves for censored event times with competing risks. Stat Med. (2013) 32:5381–97. doi: 10.1002/sim.5958
36. Subramanian A, Tamayo P, Mootha VK, Mukherjee S, Ebert BL, Gillette MA, et al. Gene set enrichment analysis: a knowledge-based approach for interpreting genome-wide expression profiles. Proc Natl Acad Sci. (2005) 102:15545–50. doi: 10.1073/pnas.0506580102
37. Mei S, Li Y, Kang X. Prognostic and functional analysis of NPY6R in Uveal Melanoma using bioinformatics. Dis Markers. (2022) 2022:4143447. doi: 10.1155/2022/4143447
38. Lin W, Wang Y, Chen Y, Wang Q, Gu Z, Zhu Y. Role of calcium signaling pathway-related gene regulatory networks in ischemic stroke based on multiple WGCNA and single-cell analysis. Oxid Med Cell Longev. (2021) 2021:8060477. doi: 10.1155/2021/8060477
39. Chen Y, Sun Y, Xu Y, Lin W-W, Luo Z, Han Z, et al. Single-cell integration analysis of heterotopic ossification and fibrocartilage developmental lineage: endoplasmic reticulum stress effector Xbp1 transcriptionally regulates the notch signaling pathway to mediate fibrocartilage differentiation. Oxid Med Cell Longev. (2021) 2021:7663366. doi: 10.1155/2021/7663366
40. Yu G, Wang L-G, Han Y, He Q-Y. clusterProfiler: an R package for comparing biological themes among gene clusters. OMICS. (2012) 16:284–7. doi: 10.1089/omi.2011.0118
41. Liu Y, Wang G, Jiang X, Li W, Zhai C, Shang F, et al. TRIM67 inhibits tumor proliferation and metastasis by mediating MAPK11 in Colorectal Cancer. J Cancer. (2020) 11:6025–37. doi: 10.7150/jca.47538
42. Wang S, Zhang Y, Huang J, Wong CC, Zhai J, Li C, et al. TRIM67 activates p53 to suppress colorectal cancer initiation and progression. Cancer Res. (2019) 79:4086–98. doi: 10.1158/0008-5472.CAN-18-3614
43. Jiang J, Ren H, Xu Y, Wudu M, Wang Q, Liu Z, et al. TRIM67 promotes the proliferation, migration, and invasion of non-small-cell lung cancer by positively regulating the notch pathway. J Cancer. (2020) 11:1240–9. doi: 10.7150/jca.38286
44. Liu R, Chen Y, Shou T, Hu J, Chen J, Qing C. TRIM67 promotes NF-κB pathway and cell apoptosis in GA-13315-treated lung cancer cells. Mol Med Rep. (2019) 20:2936–44. doi: 10.3892/mmr.2019.10509
45. Nalawade YV. Evaluation of breast calcifications. Indian J Radiol Imaging. (2009) 19:282–6. doi: 10.4103/0971-3026.57208
46. Gerasimova-Chechkina E, Toner BC, Batchelder KA, White B, Freynd G, Antipev I, et al. Loss of Mammographic tissue homeostasis in invasive lobular and ductal breast carcinomas vs. benign lesions. Front Physiol. (2021) 12:660883. doi: 10.3389/fphys.2021.660883
47. Lai C-J, Shaw CC, Geiser W, Chen L, Arribas E, Stephens T, et al. Comparison of slot scanning digital mammography system with full-field digital mammography system: comparison of slot scanning digital mammography system with full-field digital mammography system. Med Phys. (2008) 35:2339–46. doi: 10.1118/1.2919768
48. Burnside ES, Ochsner JE, Fowler KJ, Fine JP, Salkowski LR, Rubin DL, et al. Use of microcalcification descriptors in BI-RADS 4th edition to stratify risk of malignancy. Radiology. (2007) 242:388–95. doi: 10.1148/radiol.2422052130
49. Liu J, Huang L. Image-guided vacuum-assisted breast biopsy in the diagnosis of breast microcalcifications. J Int Med Res. (2018) 46:2743–53. doi: 10.1177/0300060518770577
50. Zhang L, Hao C, Wu Y, Zhu Y, Ren Y, Tong Z. Microcalcification and BMP-2 in breast cancer: correlation with clinicopathological features and outcomes. OTT. (2019) 12:2023–33. doi: 10.2147/OTT.S187835
51. Zheng K, Tan J-X, Li F, Wei Y-X, Yin X-D, Su X-L, et al. Relationship between mammographic calcifications and the clinicopathologic characteristics of breast cancer in Western China: a retrospective multi-center study of 7317 female patients. Breast Cancer Res Treat. (2017) 166:569–82. doi: 10.1007/s10549-017-4406-2
52. Ren J, Wang Y, Ware T, Iaria J, ten Dijke P, Zhu H-J. Reactivation of BMP signaling by suboptimal concentrations of MEK inhibitor and FK506 reduces organ-specific breast cancer metastasis. Cancer Lett. (2020) 493:41–54. doi: 10.1016/j.canlet.2020.07.042
53. Afzal S, Masroor I, Munir A, Idress R, Khan P, Khan S. Preoperative ultrasound-guided core biopsy of axillary nodes for staging of clinically negative axilla in breast cancer patients – A pilot study. Cureus. (2020) 12:e6718. doi: 10.7759/cureus.6718
54. Li J, Zhang K, Shi Z, Zhang X, Xie J, Liu J, et al. Triple-negative invasive breast carcinoma: the association between the sonographic appearances with clinicopathological feature. Sci Rep. (2018) 8:9040. doi: 10.1038/s41598-018-27222-6
Keywords: breast cancer, brain metastases, ultrasonography, TRIM67, biomarkers
Citation: Xuan Z, Ma T, Qin Y and Guo Y (2022) Role of Ultrasound Imaging in the Prediction of TRIM67 in Brain Metastases From Breast Cancer. Front. Neurol. 13:889106. doi: 10.3389/fneur.2022.889106
Received: 03 March 2022; Accepted: 16 May 2022;
Published: 20 June 2022.
Edited by:
Yuzhen Xu, Tongji University, ChinaReviewed by:
Junming Xu, Shanghai General Hospital, ChinaCopyright © 2022 Xuan, Ma, Qin and Guo. This is an open-access article distributed under the terms of the Creative Commons Attribution License (CC BY). The use, distribution or reproduction in other forums is permitted, provided the original author(s) and the copyright owner(s) are credited and that the original publication in this journal is cited, in accordance with accepted academic practice. No use, distribution or reproduction is permitted which does not comply with these terms.
*Correspondence: Ting Ma, bWF0aW5nNTA1OEAxNjMuY29t
Disclaimer: All claims expressed in this article are solely those of the authors and do not necessarily represent those of their affiliated organizations, or those of the publisher, the editors and the reviewers. Any product that may be evaluated in this article or claim that may be made by its manufacturer is not guaranteed or endorsed by the publisher.
Research integrity at Frontiers
Learn more about the work of our research integrity team to safeguard the quality of each article we publish.