- 1Department of Neurosurgery, General Hospital of Ningxia Medical University, Yinchuan, China
- 2Ningxia Key Laboratory of Craniocerebral Diseases, Ningxia Medical University, Yinchuan, China
The purpose of this study was to identify the biomarkers implicated in the development of intracranial hemorrhage (ICH) and potential regulatory pathways. In the transcriptomic data for patients with ICH, we identified DEmiRNAs and DEmRNAs related to hypoxia, inflammation, and their transcription factors (TFs). An ICH-based miRNA-TF-mRNA regulatory network was thus constructed, and four biomarkers (TIMP1, PLAUR, DDIT3, and CD40) were screened for their association with inflammation or hypoxia by machine learning. Following this, SP3 was found to be a transcription factor involved in hypoxia and inflammation, which regulates TIMP1 and PLAUR. From the constructed miRNA-TF-mRNA regulatory network, we identified three axes, hsa-miR-940/RUNX1/TIMP1, hsa-miR-571/SP3/TIMP1, and hsa-miR-571/SP3/PLAUR, which may be involved in the development of ICH. Upregulated TIMP1 and PLAUR were validated in an independent clinical cohort 3 days after ICH onset. According to Gene Set Enrichment Analysis (GSEA), SP3 was discovered to be important in interleukin signaling and platelet activation for hemostasis. Transcription factor SP3 associated with hypoxia or inflammation plays an important role in development of ICH. This study provides potential targets for monitoring the severity of inflammation and hypoxia in patients with ICH.
Introduction
Intracranial hemorrhage (ICH) is a condition characterized by bleeding from the brain parenchyma caused by the rupture of blood vessels in the brain, which leads to compression of the surrounding nerve tissue, disruption of the brain function, and triggering of disorders (1). ICH can be triggered by various factors, such as trauma, hypertension, and infection (2, 3). ICH accounts for 10–15% of strokes and is its most lethal subtype (4–8). The formation of a hematoma from ICH can severely disrupt tracts, leading to various dysfunctions and threatening patients' lives, which makes ICH highly disabling and mortal (4). More than 1 million people are affected by ICH each year (9). The mortality rate for patients with ICH range from 30 to 50% at 1 month and 54% at 1 year (10, 11). The unprecedented virus (COVID-19) have also been identified as potential risk factors for ICH (12). Patients with ICH need to be diagnosed and treated early and accurately in order to achieve the best possible outcome.
Neuronal apoptosis, inflammation, oxidative stress, edema formation, and the breakdown of the blood–brain barrier all contribute to ICH development (13, 14). ICH is not only pathologically characterized by inflammation, but it also causes secondary damage to the brain (15, 16). Inflammatory injury can damage the vascular endothelium and, thus, is involved in ICH development (17, 18). The infiltrating leukocytes can release pro-inflammatory factors, which further damage the blood–brain barrier, thereby worsening the secondary brain injury after ICH development (19–21). Meanwhile, hypoxia can be activated through oxidative stress mechanisms, which in turn are involved in the developmental mechanisms of ICH (14, 22). Therefore, we hypothesize that inflammation and hypoxia play important roles in ICH pathogenesis; however, the molecular mechanisms involved are not yet clear.
Because inflammation is involved in secondary damage after ICH, the degree of inflammation can be used to predict the prognosis of patients with ICH (21, 23, 24). Some indicators of inflammation, such as the neutrophil-to-lymphocyte ratio, have been shown to be useful in predicting the prognosis of patients with ICH, and they are predictive of a good outcome (25). Brain tissue can become hypoxic from ICH, causing irreversible damage (26). Genes associated with hypoxia or inflammation, as well as their pathways of action, play an important role in the development and progression of ICH. ICH development is involved in the Nrf2/HO-1 signaling pathway, according to the previous studies (27). KLF6 acts as a transcription factor that mediates SIRT5 inhibition of the Nrf2/HO-1 signaling pathway, which in turn exacerbates neuronal apoptosis and oxidative stress after ICH development (28). KLF6 plays a crucial role in the inflammatory and hypoxic response (27). Inflammation and hypoxia dramatically impact the survival and quality of life of patients with ICH. Thus, identifying the degree of inflammation and hypoxia in ICH is essential for monitoring the prognosis of patients with ICH.
Recent developments in bioinformatics, including the availability of considerable RNA sequencing data resources, have provided a direction for disease diagnosis and treatment (29–32). Gene expression profiles associated with inflammation or hypoxia can be obtained from RNA sequencing data. This study was designed to examine the genes and pathways that are potentially involved in inflammation during ICH.
Methods
Data Downloaded From the GEO Database
The GEO database was searched for intracerebral hemorrhage-related RNA transcriptomic datasets based on the following keywords: “intracerebral haemorrhage” and “brain haemorrhage”. Exclusion criteria were set as follows: (1) transcriptomic data from animal models or knockout animals; (2) brain hemorrhage caused by vascular malformations, aneurysms, etc.; and (3) drug experiments designed. A dataset containing mixed plasma samples from 15 patients with ICH and eight healthy controls (GSE43618 dataset) was filtered based on the filtering criteria (33). Peripheral blood mRNA transcriptomic data were obtained from GSE125512 for 11 patients with ICH at the onset and 3 days after onset (34). These two datasets were used to identify differentially expressed miRNAs (DEmiRNAs) and differentially expressed mRNAs (DEmRNAs). These DEmRNAs were derived from an analysis of differences between peripheral blood transcriptome expression profiles 3 days after and at ICH onset. The transcriptome data were log-2 transformed with different unit formats, and de-batching between samples was performed. Finally, we collected peripheral blood samples from 20 patients with an ICH and 17 healthy control volunteers in order to validate the genes related to inflammation and hypoxia identified by the bioinformatics analysis. Included patients must meet the following criteria: (1) experience acute cerebral hemorrhage within 3 days; (2) have clear diagnostic imaging and laboratory results; (3) have no vascular malformations, coagulation disorders, or other causes of bleeding; and (4) be free of malignant tumors and other serious diseases. Ethics approval for this study was obtained from the General Hospital of Ningxia Medical University. All the participants provided written informed consent.
Inflammation or Hypoxia-Associated Gene Sets
In order to select a broad list of candidate gene sets for inflammation- and hypoxia-related genes, we searched the Kyoto Encyclopedia of Genes and Genomes (KEGGs) database (www.kegg.jp). Furthermore, the PubMed and Web of Science databases were searched for inflammation- or hypoxia-related gene complements. Ultimately, a total of 50 hypoxia-related genes and 200 inflammation-related genes were identified. These genes are mainly involved in inflammatory or hypoxic response processes during disease development.
Analysis of Variances
First, the limma R package was used to identify DEGs, including DEmiRNAs and DEmRNAs, in the occurrence of ICH (35). We identified mRNAs that are up- and down-regulated in patients with ICH 3 days after cerebral hemorrhage compared with those at the onset of cerebral hemorrhage using differential expression analysis of the peripheral blood transcriptome. DEGs were filtered using p < 0.05 as the threshold. Data were then filtered by genes using Perl (https://www.perl.org/) to obtain dysregulated genes associated with inflammation or with hypoxia and the corresponding gene expression matrix.
Biological Functional Pathway Analysis
Functional pathway analysis of up- or down-regulated DEmRNAs using the Kyoto Encyclopedia of Genes and Genomes (KEGGs) and Gene Ontology (GO). GO terminology is described in three parts: biological processes (BPs), cellular components (CCs), and molecular functions (MFs). The clusterProfiler R package was used to complete GO and KEGG analysis as previous research (36, 37). Further, GSEA is used to analyze functional pathways of dysregulated biology involving key genes (38). Selected functional pathways for differential analysis are referenced from “c2.cp.v7.2.symbols.gmt [Curated]” in MSigDB collections (https://www.gsea-msigdb.org/gsea/msigdb/) gene set. The threshold used to identify dysfunctional pathways was set at a false discovery rate of <0.25 and adjusted p of <0.05.
Construction of miRNA–TF–mRNA Network
According to the previous study, the miRNA–TF–mRNA network was constructed (39–42). First, TF–mRNA relationship pairs were predicted in DEmRNAs using the TRRUST (v2) database (43), where mRNAs were associated with hypoxia or inflammation. To explore regulatory relationships between DEmiRNAs and DEmRNAs (including TFs), miRWalk was used (http://mirwalk.umm.uni-heidelberg.de/). R software was used to match the interrelationships between DEmiRNAs and DEmRNAs. Among the predicted miRNA–mRNA molecular pairs, only those with opposite regulatory directions were subjected to further analysis. Furthermore, Cytoscape was used to visualize the entire miRNA–TF–mRNA interaction network and to identify the hub genes in the network based on the number of connections in each node (44).
Machine Learning
Intelligent machines are converging with advancing biotechnologies to shape the future of medicine (45). Machine learning is used to screen genes associated with the progression of ICH. Support Vector Machine–Recursive Feature Elimination (SVM–RFE) was used to investigate genes associated with hypoxia and inflammation (46). SVM is excellent at handling small datasets and shows good classification performance. Redundant genes are filtered using the iterative algorithm of SVM–RFE, resulting in genes highly correlated with the outcome. Furthermore, the least absolute shrinkage and selection operator (LASSO) is used for gene screening. As previous research, LASSO analysis was performed using the “glmnet” R package (42, 47). ROC curves were used to assess the predictive ability of core genes to distinguish between patients with ICH at different progression stages, thereby testing their reliability for the outcome prediction. Principle component analysis and t-distributed stochastic neighbor embedding (t-SNE) (48) were used to demonstrate the ability of screened core genes to classify patients with ICH at different developmental stages. The R package “Rtsne” was used to implement the t-SNE algorithm based on non-linear dimensionality reduction.
Quantitative qRT-PCR
Total RNA was extracted using TRIzol (TaKaRa Bio, Shiga, Japan) and reverse-transcribed into cDNA using PrimeScript RT Master Mix (TaKaRa Bio, Shiga, Japan). According to the manufacturer's instructions, Real-time PCR was performed using SYBR Green PCR Master Mix (Takara). Using GAPDH as a reference, the 2−ΔΔCt method was applied for the relative quantification of core gene expression levels and normalized.
Statistical Analysis
All the drawings are performed using the R software (version 4.0.5). The “VennDiagram” R package is used to create a Venn diagram to present the results of the gene intersection analysis. Spearman's correlation test was used to assess the correlation between key genes, and correlation coefficients of >0.3 were considered to be co-expression relationships. Differential analysis of gene expression between the two groups was performed using the Wilcoxon rank-sum test. Unless otherwise indicated, p < 0.05 was considered a statistically significant difference.
Results
Neutrophils Play an Important Role in ICH Development
To obtain biomarkers and pathways associated with the occurrence or progression of ICH, we performed a differential expression analysis. First, 46 DEmiRNAs were identified by differential analysis between ICH and healthy control (HC) (Figure 1A). Based on the transcriptomic data obtained from the peripheral blood of ICH at different development stages, 914 DEmRNAs were identified. Compared to the onset of ICH, 444 genes were upregulated and 470 genes were downregulated 3 days after the onset of ICH (Figure 1B). These up- and downregulated genes were then subjected to separate functional pathway analyses. The main pathways shown to be upregulated in ICH by GO and KEGG included neutrophil-mediated immunity, secretory granule lumen, cell adhesion molecule binding, and regulation of actin cytoskeleton (Figure 1C). In addition, the pathways enriched by the downregulated genes contained RNA splicing, nuclear speck, Herpes simplex virus 1 infection, and condensed chromosome (Figure 1D). These results suggest that neutrophils play an important role in the development of ICH.
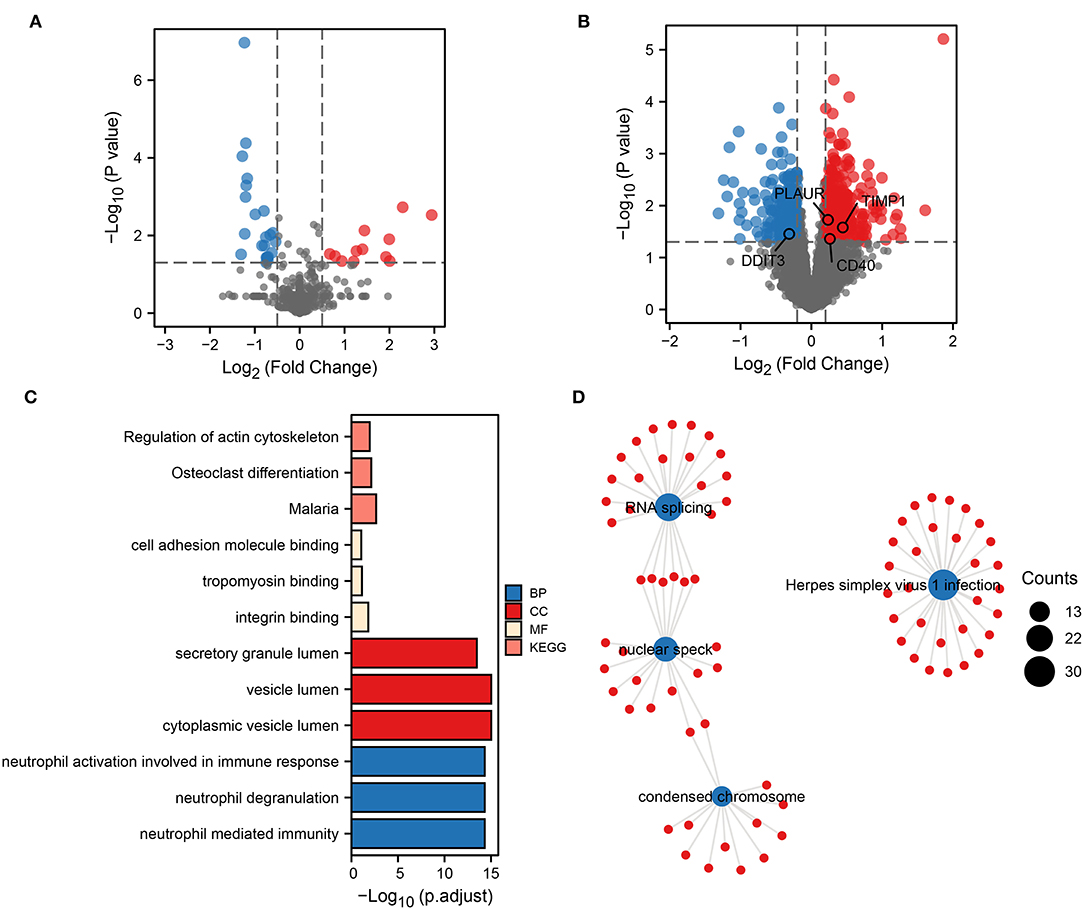
Figure 1. Biological functional pathways of DEGs. Volcano plot showing DEmiRNAs (A) and DEmRNAs (B). Red represents upregulated genes, and blue represents downregulated genes. Upregulated (C) and downregulated (D) biological functional pathways enriched by DEGs.
Identification of Differentially Expressed Genes Associated With Inflammation or Hypoxia
To explore the possible role of inflammation- or hypoxia-related genes in ICH development, we further screened the inflammation- and hypoxia-related genes separately in the DEmRNAs. We identified 15 genes associated with hypoxia; their relative expression between ICHs is shown in Figure 2A. The number of genes related to inflammation was 16 (Figure 2B). The corresponding heat map showed a clear boundary between hypoxia or inflammation-related DEGs in patients with different stages of ICH. This suggests that the genes associated with hypoxia or inflammation play an important role in ICH development.
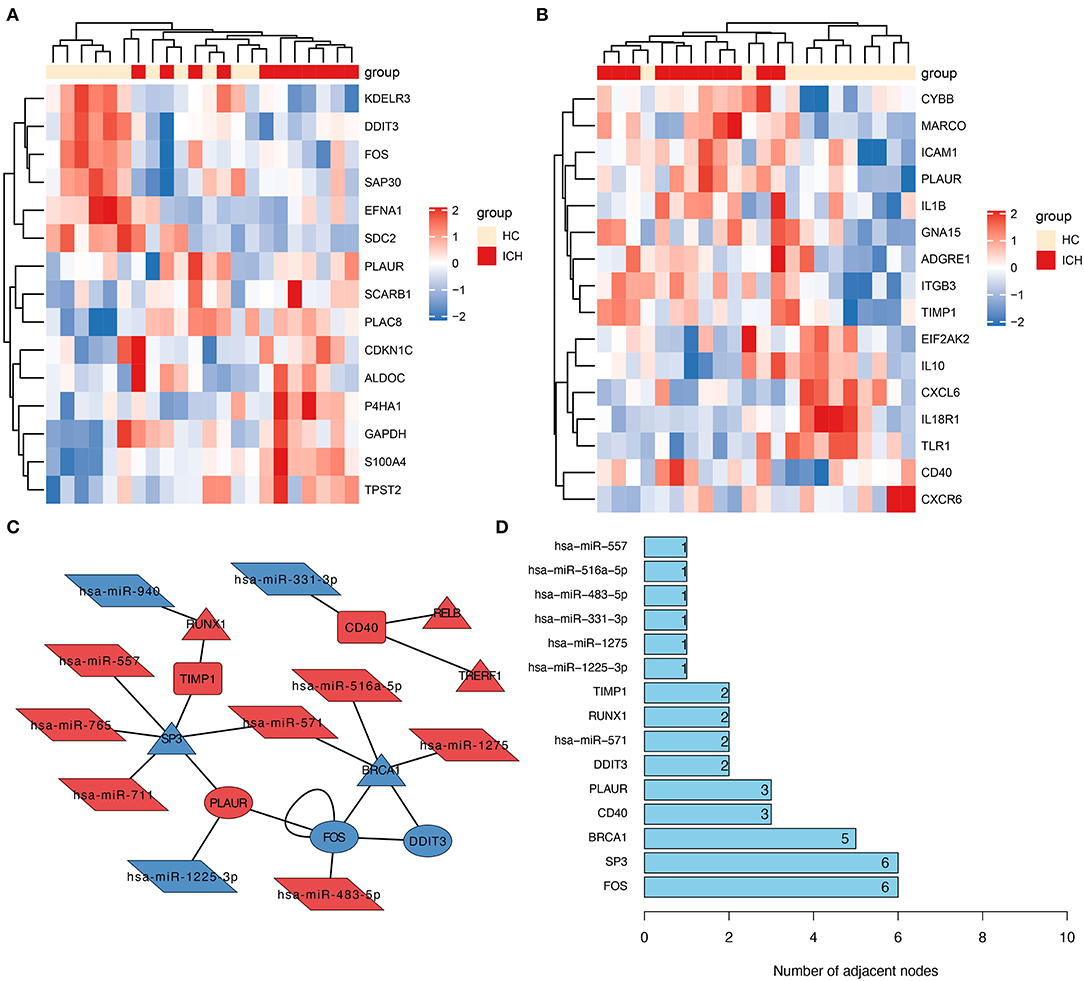
Figure 2. Construction of molecular interactions networks. Heat map showing hypoxia- (A) and inflammation-related (B) DEGs in patients with ICH. The depth of the color was used to indicate the intensity of gene expression. (C) The constructed miRNA–TF–mRNA network. Each node represents a gene and each edge represents a gene interaction. Triangle represents transcription factors, parallelogram represents miRNAs, ellipse represents hypoxia-related genes, and the round rectangle represents inflammation-related genes. (D) Number of junctions between individual genes in this miRNA–TF–mRNA network.
PPI Network Shows SP3 as a Hub Gene and May Be Associated With Hypoxia and Inflammation
From all the DEmRNAs, eight differentially expressed transcription factors (DETFs) were identified, which can regulate some of these genes in DEGs associated with hypoxia or inflammation. Furthermore, 10 DEmiRNAs were predicted to potentially act on these DEmRNAs (or DETFs) associated with hypoxia or inflammation. And the relationship pairs between these DEmiRNAs and DEmRNAs (including DETFs) were constructed as an miRNA–TF–mRNA interaction network (Figure 2C). From this molecular interaction network, SP3 was identified as the hub gene in the network, as it has the highest number of linkages (Figure 2D). From the constructed molecular interaction network, we further identified a possible correlation between SP3 and TIMP1 and PLAUR. TIMP1 is an inflammation-related gene, while PLAUR is a gene associated with hypoxia and inflammation. Therefore, the hub gene SP3 might be a transcription factor associated with hypoxia and inflammation.
Thirteen Important Genes Obtained From Machine Learning Screening
To evaluate the genes associated with hypoxia or inflammation that are closely related to ICH development, SVM–RFE and LASSO analyses were applied to screen DEGs. SVM–RFE showed minimal error in outcome prediction when all 31 hypoxia- or inflammation-related DEmRNAs were selected (Figure 3A). In a subsequent analysis, the Lasso analysis identified 13 hypoxia- or inflammation-related genes that were strongly associated with ICH development (Figure 3B). Figure 3C shows the co-expression network of these 13 genes, and TIMP1 and PLAUR were found to be co-expressed with several genes. Moreover, PCA and tSNE visualizations demonstrate that these 13 genes can be used to distinguish patients with ICH at different stages (Figures 3D,E).
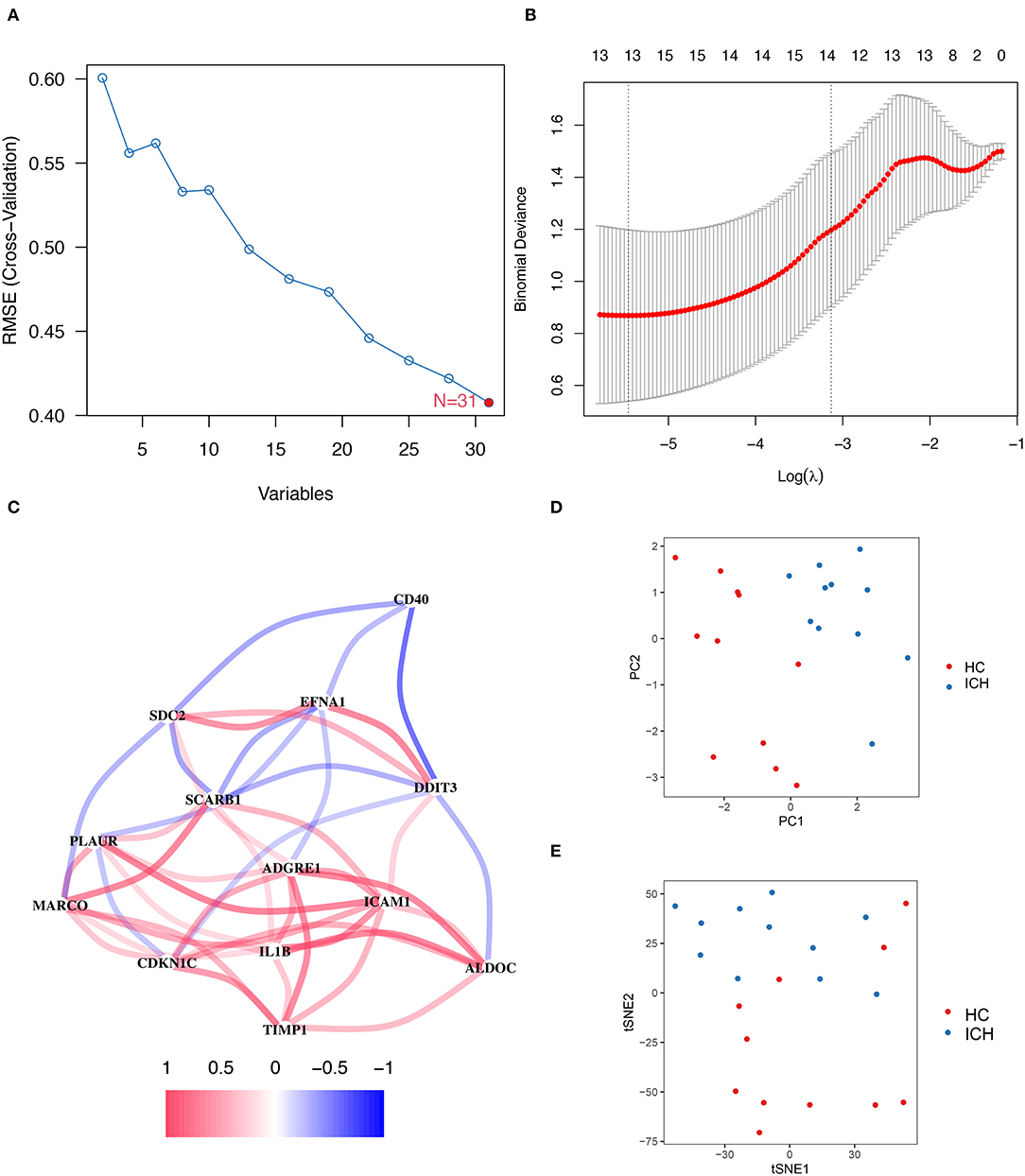
Figure 3. SVM–RFE and LASSO regression analysis results. (A) On a line graph the prediction accuracy of each variable included in the model is displayed. And the SVM–RFE screening process showed the smallest errors were obtained when all 31 genes were included in the model. (B) The LASSO analysis resulted in a final screening of 13 genes. (C) Correlation linkage maps of hypoxia- and inflammation-related genes using machine learning. (D) PCA shows that the visualization of data based on PC1 and PC2 can be clearly distinguish between patients with ICH at different stages. (E) Visualizes the ability to distinguish patients with ICH at different stages of development by tSNE method.
Venn Analysis Identified Four DEGs Associated With Inflammation or Hypoxia
An intersection analysis of hypoxia- or inflammation-related DEGs obtained from machine learning and PPI networks was performed, and four core inflammation- or hypoxia-related DEGs (TIMP1, PLAUR, DDIT3, and CD40) were identified (Figure 4A). The relative expression profiles of these four core genes in patients with ICH are shown in Figure 4B. The ROC curves for PLAUR (AUC = 0.777), DDIT3 (AUC = 0.669), CD40 (AUC = 0.727), and TIMP1 (AUC = 0.719) for the different stages of ICH development are shown in Figures 4C–F, respectively, indicating their moderate predictive power. PCA and tSNE visualization showed that patients with different stages of ICH can be distinguished based on these four genes (TIMP1, PLAUR, DDIT3, and CD40) (Figures 4G,H). Therefore, four biomarkers (TIMP1, PLAUR, DDIT3, and CD40) were screened for their association with inflammation or hypoxia by machine learning.
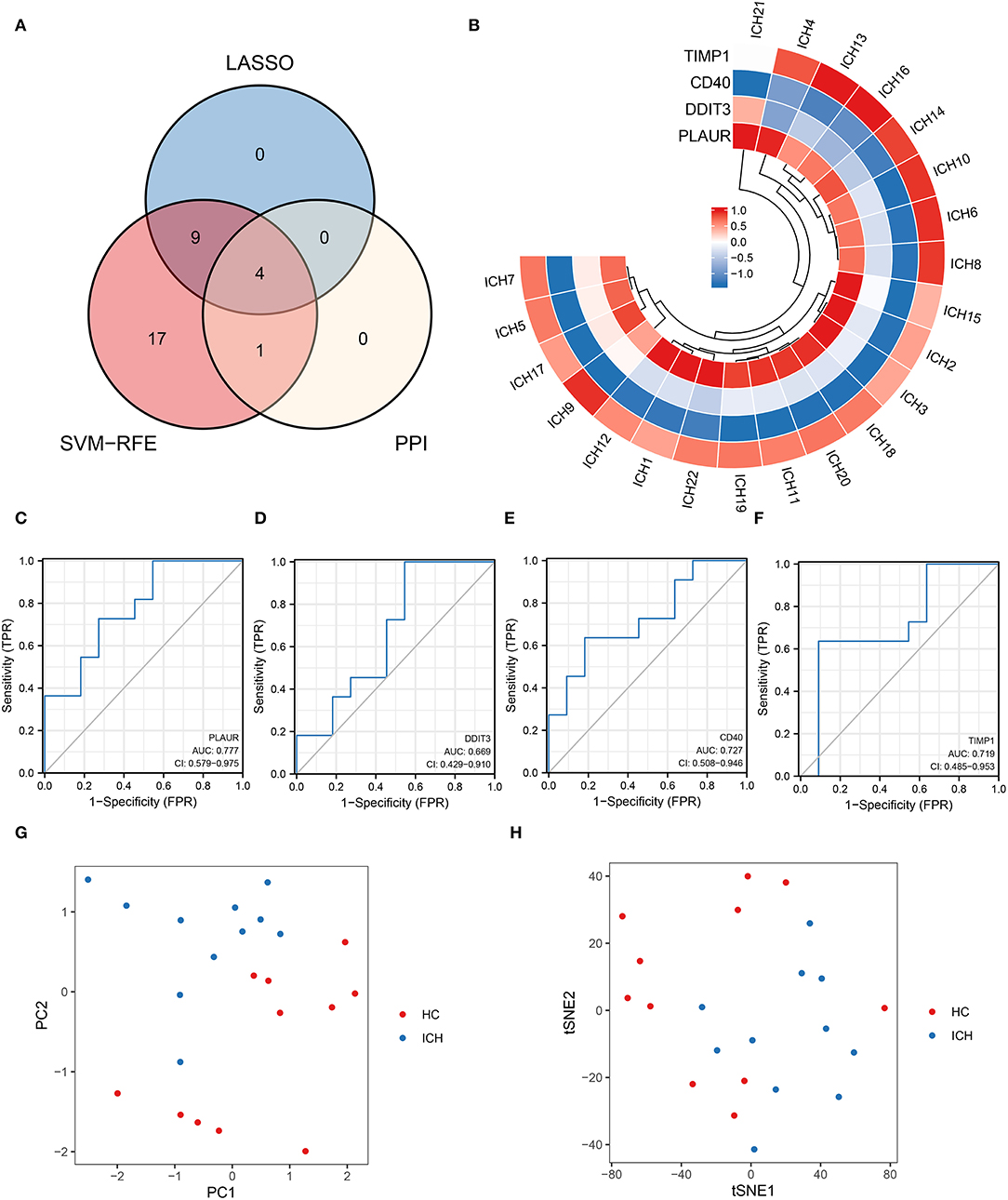
Figure 4. Four key genes obtained from the cross-tabulation analysis. (A) The Venn diagram shows that four core genes are shared in the PPI network, SVM–RFE, and LASSO. (B) Relative expression profiles of these four core genes in all the patients. (C) PALUR, (D) DDIT3, (E) CD40, and (F) the ability of TIMP1 to differentiate between patients with ICH at different development stages. (G) PCA and (H) tSNE visualization showing the ability to discriminate between patients with ICH at different development stages.
Transcription Factors and Corresponding miRNAs That Regulate TIMP1
In the regulatory network, we discover that RUNX1 and SP3 may act as transcriptional regulators of TIMP1 (Figure 2C). RUNX1 is regulated by hsa-miR-940, and SP3 is regulated by hsa-miR-571 (which has the most junctions). The magnitudes of the fold change values for hsa-miR-940 and hsa-miR-571 are shown in Figure 5A. The relative ranking of the fold change values for TIMP1, PLAUR, DDIT3, and CD40 are shown in Figure 5B. Further correlation analysis showed a positive correlation between RUNX1 and TIMP1 (r = 0.24, Figure 5C) and a negative correlation between SP3 and TIMP1 (r = −0.381, Figure 5D). Although there was no statistically significant difference, more cellular research are needed. Therefore, we further predicted two miRNA–TF–mRNA axes around the inflammation-related gene TIMP1, namely, hsa-miR-940/RUNX1/TIMP1 and hsa-miR-571/SP3/TIMP1 (Figure 2C). Validation in an independent clinical cohort showed statistically significant differences in TIMP1 expression between ICH and HC (p < 0.01, Figure 5E).
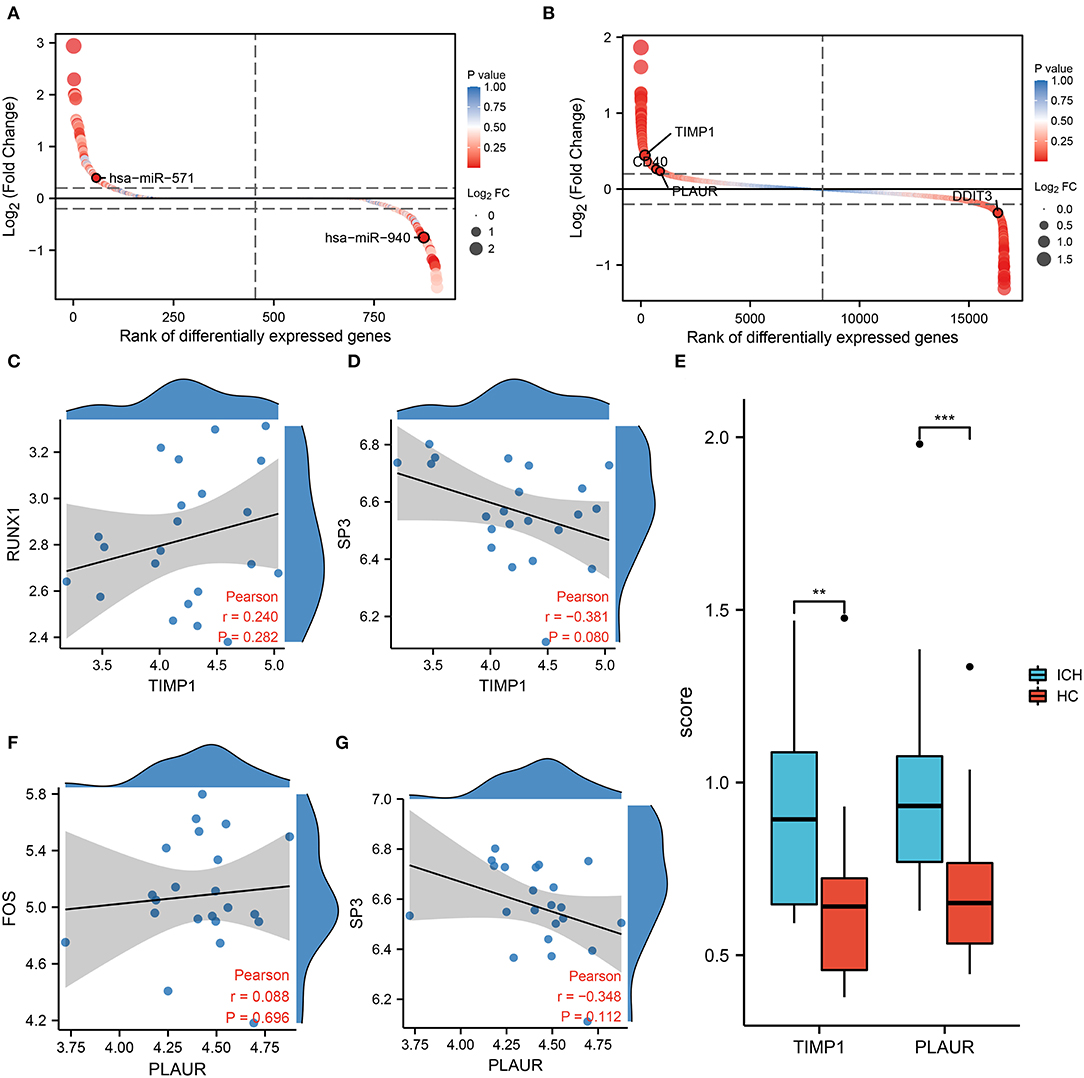
Figure 5. Results of co-expression analysis of four core genes. DEmiRNAs (A) DEmRNAs (B) are arranged by log2 (Fold Change). (C) Correlation analysis between RUNX1 and the inflammation-associated gene TIMP1. (D) Correlation analysis between SP3 and the inflammation-associated gene TIMP1. (E) PCR showed differential expression of TIMP1 and PLAUR between the HC and ICH groups. (F) Correlation analysis between FOS and PLAUR. (G) Correlation analysis between SP3 and PLAUR.
Transcription Factors and Corresponding miRNAs That Regulate PLAUR
In the molecular interaction network (Figure 2C), the transcription factors of the hypoxia-related gene PLAUR were SP3 and FOS. SP3 also exhibited a regulatory effect on the inflammation-related gene TIMP3. Based on a condition of >0.3 correlation coefficient, we found no co-expression between FOS and PLAUR (r = 0.08, p = 0.282, Figure 5F), while there was some negative correlation between SP3 and PLAUR (r = −0.348, Figure 5G). Therefore, we identified SP3 as a key hypoxia- and inflammation-related transcription factor. In addition, hsa-miR-571/SP3/PLAUR was constructed around SP3 and PLAUR as an axis miRNA-TF-mRNA (Figure 2C). There was a significant difference in PLAUR expression between ICH and HC based on the data from an independent clinical cohort (p < 0.01, Figure 5E).
SP3 Is Involved in Leukocyte- and Platelet-Related Physiological Processes
We performed GSEA analysis of the transcription factors SP3 and RUNX1 to explore the pathways in which they might be involved. GSEA showed that the signaling by interleukins, hemostasis, platelet activation signaling and aggregation, and mitotic prometaphase pathways were upregulated in patients with ICH with SP3 downregulation (Figure 6A). In patients with ICH with elevated RUNX1 expression, elastic fiber formation was downregulated, while the transcriptional regulation of the granulopoiesis pathway was upregulated (Figure 6B). The aforementioned results indicate that SP3 may be involved in interleukin signaling and platelet activation for hemostasis, while RUNX1 may be involved in granulopoiesis after ICH onset.
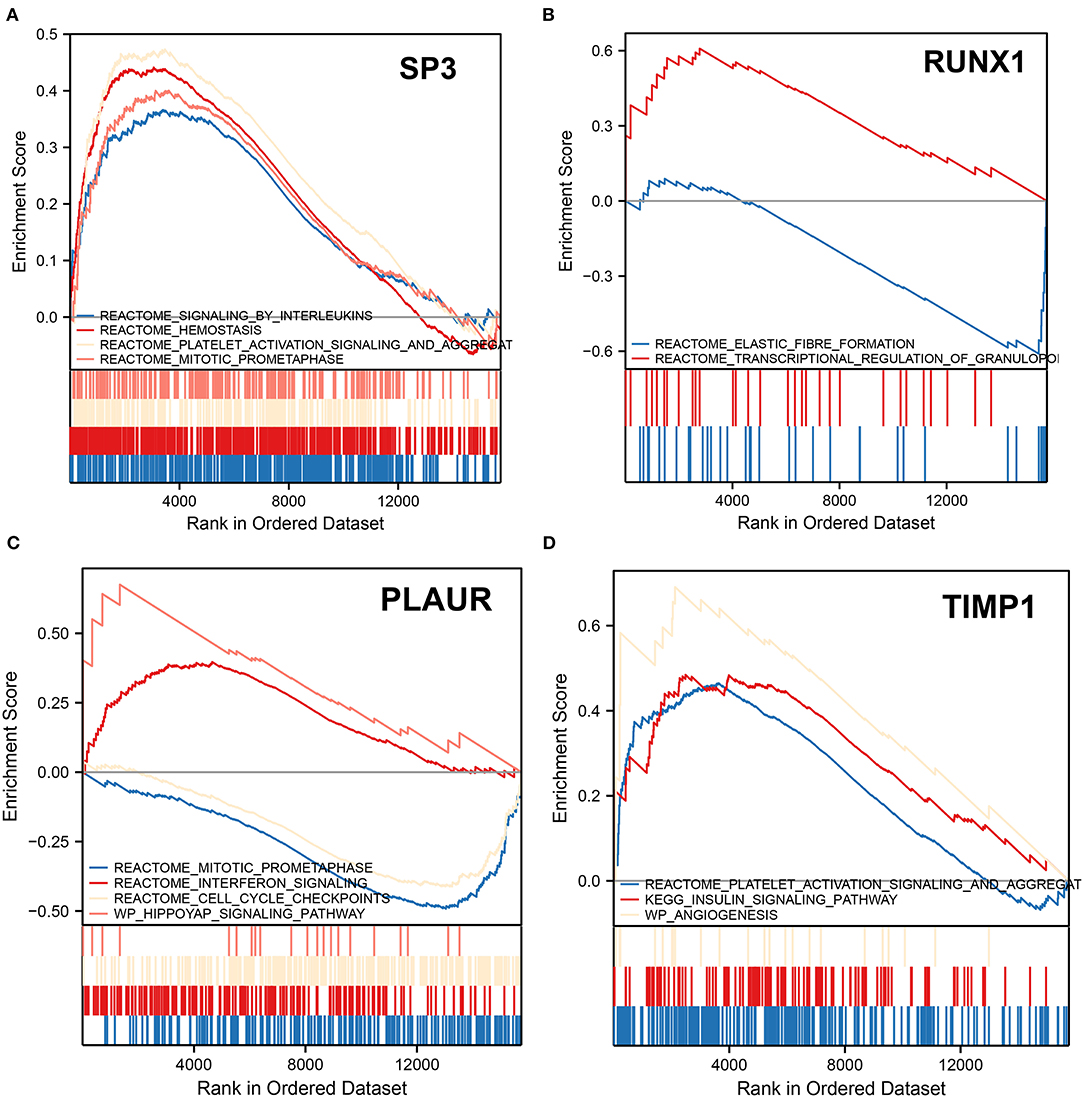
Figure 6. Gene set enrichment analysis. (A) GSEA shows altered pathways in samples with downregulated SP3. (B) GSEA shows altered pathways in samples with upregulated RUNX1. (C) GSEA shows altered pathways in samples upregulated by PLAUR. (D) GSEA shows altered pathways in samples with TIMP1 upregulation. The upward peaks of the curve represent the upward adjustment pathway, while the downward peaks represent the downward adjustment pathway.
TIMP1 Is Associated With Platelets and Angiogenesis
In patients with ICH with PLAUR upregulation, mitotic prometaphase and cell cycle checkpoints were downregulated, while interferon signaling and hippoyap signaling pathway were upregulated (Figure 6C). In patients with ICH with elevated expression of TIMP1, the platelet activation signaling and aggregation, insulin signaling pathway, and angiogenesis pathways were upregulated (Figure 6D). PLAUR and TIMP1 were both upregulated 3 days after ICH onset. Therefore, it is hypothesized that PLAUR, a hypoxia and inflammation-related gene, may be involved in upregulating the interferon signaling pathway and hippo pathway, while TIMP1 may be involved in platelet activation and aggregation, activating insulin signaling pathways, and promoting angiogenesis.
Discussion
Four hypoxia- or inflammation-related biomarkers (TIMP1, PLAUR, DDIT3, and CD40) were identified in this study. Among them, SP3 might act as a transcriptional regulator for TIMP1 and PLAUR. A bioinformatics approach was used to predict the possible roles of the three hypoxia- and inflammation-related miRNA–TF–mRNA axes (hsa-miR-940/RUNX1/TIMP1, hsa-miR-571/SP3/TIMP1, and hsa-miR-571/SP3/PLAUR) in ICH development. Independent clinical cohort studies have validated upregulation of TIMP1 and PLAUR expression after the onset of ICH. In addition, GSEA was used to analyze the functions of SP3, RUNX1, TIMP1, and PLAUR.
TIMP1 and PLAUR have been identified in the previous studies as involved in the progression of ICH. Matrix metalloproteinases (MMPs) are the most important degrading enzymes in the pathogenesis of ICH (49), during extracellular matrix reconstruction and blood–brain barrier disruption (50). TIMP1 is a major endogenous inhibitor of MMP-9 and was found to be significantly more expressed in the serum of patients with ICH than in normal controls in a study of the Chinese Han patients with ICH (51). TIMP-1 expression is also associated with early mortality in ICH as its potential biomarker for predicting mortality (52). The urokinase-type fibrinogen activator encoded by the PLAUR gene plays an important role in the development of cortical neural circuits and in brain tissue remodeling after brain injury (53, 54). Thus, PLAUR can be related to the prognosis of patients with ICH. In this study, TIMP1 and PLAUR were upregulated 3 days after the onset of ICH compared with that before the onset. Previous studies have found that TIMP1 is associated with primary sarcopenia, colon cancer progression and metastasis, and some infectious diseases (55–57). We found that TIMP1 is involved in platelet activation and aggregation, insulin signaling pathway activation, and angiogenesis. The upregulation of TIMP1 can therefore affect recovery, regression, and progression of the patients with ICH by affecting both platelet function and angiogenesis. And PLAUR is associated as an inflammation-related gene with diseases or processes, such as asthma, myocardial infarction, and reduced lung function (58–60). TIMP1 and PLAUR are involved in inflammation and hypoxia-related progression in ICH. MMP-9 is expressed in inflamed tissues and is involved in the inflammatory process (61). TIMP-1 is a natural inhibitor of MMP-9 (62). Thus, TIMP-1 might play a role in the inflammatory response by inhibiting MMP-9. After inflammation occurs, PLAUR binds to PLAU and activates plasminogen to plasmin, promoting inflammatory cell migration and activation and matrix metallopeptidase (MMP) activation, thereby participating in the inflammatory response (60, 63–65). In addition, PLAUR can be regulated by the hypoxia-inducible factor HIF-1 to play a role in the hypoxia-related mechanisms of the disease (66). In this study, TIMP1 and PLAUR upregulation in ICH was validated in an independent cohort, which confirms the involvement of TIMP1 and PLAUR in ICH development.
In this study, we found that both TIMP1 and PLAUR are regulated by SP3, a transcription factor associated with both hypoxia and inflammation. Hypoxia has long been found to downregulate SP3 (67). The SP transcription factor family can be involved in the regulation of hypoxic gene expression in the hippocampus through a mechanism mediated by oxidative stress during hypoxia (68). In addition, SP3 is involved in the molecular regulatory mechanism of hypoxia-inducible factor 1α (69). Following inflammatory stimuli, SP3 and NF–κB interact to regulate inflammatory gene expression (70). SP3 is also involved in LPS-induced cellular inflammation (71). In patients with concomitant SP3 downregulation, signaling by interleukins, hemostasis, platelet activation signaling and aggregation, and mitotic prometaphase pathways were upregulated. These pathways suggest that downregulated SP3 is involved in interleukin signaling, platelet activation, and hemostasis. Therefore, SP3 downregulation might influence the progression and regression of ICH by affecting the degree of platelet activation as well as the level of inflammation, ultimately affecting the prognosis and quality of life of patients.
The miRNA–TF–mRNA network identified hsa-miR-571 as the pivotal miRNA regulating SP3. Previous studies have found that miR-571 functions in DNA replication and genomic stability (72). miR-571 is involved in the inflammatory process in cirrhosis (73), and can regulate the activation of human stem stellate cells (74) by mediating the Notch3 signaling pathway (75). However, there is a lack of studies on the relationship between miR-571 and SP3. In this study, we found the first evidence suggesting that hsa-miR-571 regulated the level of inflammation and platelet activation in ICH by regulating SP3 translation. And hsa-miR-571/SP3/TIMP1 and hsa-miR-571/SP3/PLAUR are two miRNA-TF-mRNAs involved in ICH development.
TIMP1 and PLAUR were differentially expressed in ICH and were upregulated 3 days after the onset of ICH. The upregulation of TIMP1 might have influenced the outcome of patients with ICH by affecting the platelet function and angiogenesis. PLAUR, in turn, was involved in the upregulation of the interferon signaling pathway and the hippo pathway. The hypoxia- and inflammation-related transcription factor SP3 was involved in the regulation of TIMP1 and PLAUR. SP3 might have influenced the progression of ICH by affecting the degree of platelet activation and the inflammation level. These findings provide potential targets for the diagnosis, treatment, and regression of ICH in order to monitor the severity of inflammation and hypoxia in patients with ICH. Although clinical samples were used for validating the study results, the number of clinical samples was small and the strength of validation needs improvement. Bioinformatic findings will need to be validated in the relevant cell lines as well as ICH animal models in the future. In addition to investigate the relationship between SP3 and its counterparts hsa-miR-571, TIMP1, and PLAUR, more research is needed to identify the role of SP3 in ICH.
Conclusion
The hsa-miR-940/RUNX1/TIMP1, hsa-miR-571/SP3/TIMP1, and hsa-miR-571/SP3/PLAUR play important roles in ICH development. The hypoxia- and inflammation-related transcription factor SP3 might be involved in platelet activation in ICH through the regulation of TIMP1/PLAUR, as well as in inflammatory regulation.
Data Availability Statement
The original contributions presented in the study are included in the article/Supplementary Material, further inquiries can be directed to the corresponding author/s.
Ethics Statement
The studies involving human participants were reviewed and approved by General Hospital of Ningxia Medical University's Ethics Committee. The patients/participants provided their written informed consent to participate in this study.
Author Contributions
DW, JF, PW, ZY, and TS performed the data curation and analysis. DW, JF, and TS analyzed and interpreted the results. TS drafted and reviewed the manuscript. All authors read and approved the final manuscript.
Conflict of Interest
The authors declare that the research was conducted in the absence of any commercial or financial relationships that could be construed as a potential conflict of interest.
Publisher's Note
All claims expressed in this article are solely those of the authors and do not necessarily represent those of their affiliated organizations, or those of the publisher, the editors and the reviewers. Any product that may be evaluated in this article, or claim that may be made by its manufacturer, is not guaranteed or endorsed by the publisher.
Acknowledgments
The authors thank all the patients and staff who participated in this study.
Supplementary Material
The Supplementary Material for this article can be found online at: https://www.frontiersin.org/articles/10.3389/fneur.2022.886329/full#supplementary-material
References
1. Choy KW, Tsai AP-Y, Lin PB-C, Wu M-Y, Lee C, Alias A, et al. The role of urocortins in intracerebral hemorrhage. Biomolecules. (2020) 10:96. doi: 10.3390/biom10010096
2. Aguilar MI, Brott TG. Update in intracerebral hemorrhage. Neurohospitalist. (2011) 1:148–59. doi: 10.1177/1941875211409050
3. Cho S-M, Lee T, Starling RC, Thompson NR, Uchino K. The impact of infection and elevated INR in LVAD-associated intracranial hemorrhage: a case-crossover study. ASAIO J. (2019) 65:545–9. doi: 10.1097/MAT.0000000000000887
4. Sun L, Zhang K, Zhai W, Li H, Shen H, Yu Z, et al. TAR DNA binding protein-43 loss of function induced by phosphorylation at S409/410 blocks autophagic flux and participates in secondary brain injury after intracerebral hemorrhage. Front Cell Neurosci. (2018) 12:79. doi: 10.3389/fncel.2018.00079
5. Chen S, Yang Q, Chen G, Zhang JH. An update on inflammation in the acute phase of intracerebral hemorrhage. Transl Stroke Res. (2015) 6:4–8. doi: 10.1007/s12975-014-0384-4
6. Leclerc JL, Santiago-Moreno J, Dang A, Lampert AS, Cruz PE, Rosario AM, et al. Increased brain hemopexin levels improve outcomes after intracerebral hemorrhage. J Cereb Blood Flow Metab. (2018) 38:1032–46. doi: 10.1177/0271678X16679170
7. Zhai W, Chen D, Shen H, Chen Z, Li H, Yu Z, et al. A1 adenosine receptor attenuates intracerebral hemorrhage-induced secondary brain injury in rats by activating the P38-MAPKAP2-Hsp27 pathway. Mol Brain. (2016) 9:66. doi: 10.1186/s13041-016-0247-x
8. Mao L, Li P, Zhu W, Cai W, Liu Z, Wang Y, et al. Regulatory T cells ameliorate tissue plasminogen activator-induced brain haemorrhage after stroke. Brain. (2017) 140:1914–1931. doi: 10.1093/brain/awx111
9. An SJ, Kim TJ, Yoon B-W. Epidemiology, risk factors, and clinical features of intracerebral hemorrhage: an update. J Stroke. (2017) 19:3–10. doi: 10.5853/jos.2016.00864
10. Jiang C, Wang Y, Hu Q, Shou J, Zhu L, Tian N, et al. Immune changes in peripheral blood and hematoma of patients with intracerebral hemorrhage. FASEB j. (2020) 34:2774–91. doi: 10.1096/fj.201902478R
11. Al-Mufti F, Thabet AM, Singh T, El-Ghanem M, Amuluru K, Gandhi CD. Clinical and radiographic predictors of intracerebral hemorrhage outcome. Intervent Neurol. (2018) 7:118–36. doi: 10.1159/000484571
12. Adewunmi T, Modinat A, Lois H. A systematic review on the neurological manifestations of COVID-19. Life Res. (2020) 3:158. doi: 10.53388/life2020-1014-303
13. Iniaghe LO, Krafft PR, Klebe DW, Omogbai EKI, Zhang JH, Tang J. Dimethyl fumarate confers neuroprotection by casein kinase 2 phosphorylation of Nrf2 in murine intracerebral hemorrhage. Neurobiol Dis. (2015) 82:349–58. doi: 10.1016/j.nbd.2015.07.001
14. Wei C-C, Kong Y-Y, Li G-Q, Guan Y-F, Wang P, Miao C-Y. Nicotinamide mononucleotide attenuates brain injury after intracerebral hemorrhage by activating Nrf2/HO-1 signaling pathway. Sci Rep. (2017) 7:717. doi: 10.1038/s41598-017-00851-z
15. Bi Y, Huan Y, Cai W, Wang X, Liang Z, Liu Z, et al. Mild hypothermia in combination with minimally invasive evacuation of hematoma reduces inflammatory damage in patients via the nuclear factor-κB pathway. Exp Ther Med. (2014) 8:1717–22. doi: 10.3892/etm.2014.2012
16. Wilkinson DA, Pandey AS, Thompson BG, Keep RF, Hua Y, Xi G. Injury mechanisms in acute intracerebral hemorrhage. Neuropharmacology. (2018) 134:240–8. doi: 10.1016/j.neuropharm.2017.09.033
17. Yenari MA, Han HS. Neuroprotective mechanisms of hypothermia in brain ischaemia. Nat Rev Neurosci. (2012) 13:267–78. doi: 10.1038/nrn3174
18. Cai PY, Bodhit A, Derequito R, Ansari S, Abukhalil F, Thenkabail S, et al. Vagus nerve stimulation in ischemic stroke: old wine in a new bottle. Front Neurol. (2014) 5:107. doi: 10.3389/fneur.2014.00107
19. Keep RF, Xiang J, Ennis SR, Andjelkovic A, Hua Y, Xi G, Hoff JT. Blood-brain barrier function in intracerebral hemorrhage. In: Zhou L-F, Chen X-C, Huang F-P, Xi G, Keep RF, Hua Y, et al. editors. Cerebral Hemorrhage. Acta Neurochirurgica Supplementum. Vienna: Springer Vienna (2008). p. 73–7.
20. Joice SL, Mydeen F, Couraud P-O, Weksler BB, Romero IA, Fraser PA, et al. Modulation of blood–brain barrier permeability by neutrophils: in vitro and in vivo studies. Brain Res. (2009) 1298:13–23. doi: 10.1016/j.brainres.2009.08.076
21. Yu Z, Zheng J, Guo R, Ma L, You C, Li H. Prognostic impact of leukocytosis in intracerebral hemorrhage: a PRISMA-compliant systematic review and meta-analysis. Medicine. (2019) 98:e16281. doi: 10.1097/MD.0000000000016281
22. Mohammadi H, Shamshirian A, Eslami S, Shamshirian D, Ebrahimzadeh MA. Magnesium sulfate attenuates lethality and oxidative damage induced by different models of hypoxia in mice. BioMed Res Int. (2020) 2020:1–8. doi: 10.1155/2020/2624734
23. Zhou Y, Wang Y, Wang J, Anne Stetler R, Yang Q-W. Inflammation in intracerebral hemorrhage: from mechanisms to clinical translation. Progress Neurobiol. (2014) 115:25–44. doi: 10.1016/j.pneurobio.2013.11.003
24. Brunswick AS, Hwang BY, Appelboom G, Hwang RY, Piazza MA, Connolly ES. Serum biomarkers of spontaneous intracerebral hemorrhage induced secondary brain injury. J Neurol Sci. (2012) 321:1–10. doi: 10.1016/j.jns.2012.06.008
25. Lattanzi S, Cagnetti C, Provinciali L, Silvestrini M. Neutrophil-to-lymphocyte ratio predicts the outcome of acute intracerebral hemorrhage. Stroke. (2016) 47:1654–57. doi: 10.1161/STROKEAHA.116.013627
26. Liu Y, Zhang J, Zan J, Zhang F, Liu G, Wu A. Lidocaine improves cerebral ischemia-reperfusion injury in rats through cAMP/PKA signaling pathway. Exp Ther Med. (2020) 20:495–9. doi: 10.3892/etm.2020.8688
27. Kim G, Ng HP, Chan ER, Mahabeleshwar GH. Kruppel-like factor 6 promotes macrophage inflammatory and hypoxia response. FASEB J. (2020) 34:3209–23. doi: 10.1096/fj.201902221R
28. Sun J, Cai J, Chen J, Li S, Liao X, He Y, et al. Krüppel-like factor 6 silencing prevents oxidative stress and neurological dysfunction following intracerebral hemorrhage via sirtuin 5/Nrf2/HO-1 axis. Front Aging Neurosci. (2021) 13:646729. doi: 10.3389/fnagi.2021.646729
29. Zhao X, Yang J, Yang C. The neuronal transcription factor Creb3l1 potential upregulates Ntrk2 in the hypertensive microenvironment to promote vascular smooth muscle cell-neuron interaction and prevent neurons from ferroptosis: a bioinformatic research of scRNA-seq data. Dis Markers. (2022) 2022:8339759. doi: 10.1155/2022/8339759
30. Lin W, Wang Y, Chen Y, Wang Q, Gu Z, Zhu Y. Role of calcium signaling pathway-related gene regulatory networks in ischemic stroke based on multiple WGCNA and single-cell analysis. Oxid Med Cell Longevity. (2021) 2021:1–35. doi: 10.1155/2021/8060477
31. Sun C, Ma S, Chen Y, Kim NH, Kailas S, Wang Y, et al. Diagnostic value, prognostic value, and immune infiltration of LOX family members in liver cancer: bioinformatic analysis. Front Oncol. (2022) 12:843880. doi: 10.3389/fonc.2022.843880
32. Mei S, Li Y, Kang X. Prognostic and functional analysis of NPY6R in uveal melanoma using bioinformatics. Disease Markers. (2022) 2022:1–14. doi: 10.1155/2022/4143447
33. Guo D, Liu J, Wang W, Hao F, Sun X, Wu X, et al. Alteration in abundance and compartmentalization of inflammation-related miRNAs in plasma after intracerebral hemorrhage. Stroke. (2013) 44:1739–42. doi: 10.1161/STROKEAHA.111.000835
34. Walsh KB, Zhang X, Zhu X, Wohleb E, Woo D, Lu L, et al. Intracerebral hemorrhage induces inflammatory gene expression in peripheral blood: global transcriptional profiling in intracerebral hemorrhage patients. DNA Cell Biol. (2019) 38:660–9. doi: 10.1089/dna.2018.4550
35. Ritchie ME, Phipson B, Wu D, Hu Y, Law CW, Shi W, et al. Limma powers differential expression analyses for RNA-sequencing and microarray studies. Nucleic Acids Res. (2015) 43:e47. doi: 10.1093/nar/gkv007
36. Yu G, Wang L-G, Han Y, He Q-Y. clusterProfiler: an r package for comparing biological themes among gene clusters. OMICS J Integrative Biol. (2012) 16:284–7. doi: 10.1089/omi.2011.0118
37. Lu L, Kang X, Yi B, Jiang C, Yan X, Chen B, et al. Exploring the mechanism of Yiqi Qingre Ziyin method in regulating neuropeptide expression for the treatment of atrophic rhinitis. Dis Mark. (2022) 2022:1–12. doi: 10.1155/2022/2056837
38. Subramanian A, Tamayo P, Mootha VK, Mukherjee S, Ebert BL, Gillette MA, et al. Gene set enrichment analysis: a knowledge-based approach for interpreting genome-wide expression profiles. Proc Natl Acad Sci. (2005) 102:15545–50. doi: 10.1073/pnas.0506580102
39. Feng W, Yang J, Song W, Xue Y. Crosstalk between heart failure and cognitive impairment via hsa-miR-933/RELB/CCL21 pathway. BioMed Res Int. (2021) 2021:1–16. doi: 10.1155/2021/2291899
40. Sun Y, Wang H, Li Y, Liu S, Chen J, Ying H. miR-24 and miR-122 negatively regulate the transforming growth factor-β/Smad signaling pathway in skeletal muscle fibrosis. Mol Ther Nucleic Acids. (2018) 11:528–37. doi: 10.1016/j.omtn.2018.04.005
41. Chen Y, Sun Y, Xu Y, Lin W-W, Luo Z, Han Z, et al. Single-cell integration analysis of heterotopic ossification and fibrocartilage developmental lineage: endoplasmic reticulum stress effector Xbp1 transcriptionally regulates the notch signaling pathway to mediate fibrocartilage differentiation. Oxid Med Cell Longevity. (2021) 2021:1–29. doi: 10.1155/2021/8302831
42. Hu C, Li Q, Shou J, Zhang F, Li X, Wu M, et al. Constructing a predictive model of depression in chemotherapy patients with non-Hodgkin's lymphoma to improve medical staffs' psychiatric care. BioMed Res Int. (2021) 2021:9201235. doi: 10.1155/2021/9201235
43. Han H, Cho J-W, Lee S, Yun A, Kim H, Bae D, et al. TRRUST v2: an expanded reference database of human and mouse transcriptional regulatory interactions. Nucleic Acids Res. (2018) 46:D380–6. doi: 10.1093/nar/gkx1013
44. Cline MS, Smoot M, Cerami E, Kuchinsky A, Landys N, Workman C, et al. Integration of biological networks and gene expression data using Cytoscape. Nat Protoc. (2007) 2:2366–82. doi: 10.1038/nprot.2007.324
45. Moingeon P. Artificial intelligence and the future of medicine: a multidimensional analysis. Life Res. (2021) 4:3. doi: 10.53388/life2021-0103-301
46. Huang M-L, Hung Y-H, Lee WM, Li RK, Jiang B-R. SVM-RFE based feature selection and Taguchi parameters optimization for multiclass SVM classifier. Sci World J. (2014) 2014:795624. doi: 10.1155/2014/795624
47. Liu Z, Li C. A predictive model for the risk of cognitive impairment in patients with gallstones. BioMed Res Int. (2021) 2021:3792407. doi: 10.1155/2021/3792407
48. Cieslak MC, Castelfranco AM, Roncalli V, Lenz PH, Hartline DK. t-Distributed stochastic neighbor embedding (t-SNE): a tool for eco-physiological transcriptomic analysis. Mar Genomics. (2020) 51:100723. doi: 10.1016/j.margen.2019.100723
49. Wu S, Guo T, Qi W, Li Y, Gu J, Liu C, et al. Curcumin ameliorates ischemic stroke injury in rats by protecting the integrity of the blood-brain barrier. Exp Ther Med. (2021) 22:783. doi: 10.3892/etm.2021.10215
50. Simão F, Ustunkaya T, Clermont AC, Feener EP. Plasma kallikrein mediates brain hemorrhage and edema caused by tissue plasminogen activator therapy in mice after stroke. Blood. (2017) 129:2280–90. doi: 10.1182/blood-2016-09-740670
51. Wang H-X, Yang Q-D, Liu B-Q, Zhang L, Ma M-M, Hu Z-Y, et al. TIMP-1 polymorphisms in a Chinese Han population with intracerebral hemorrhage. Int J Neurosci. (2014) 124:61–7. doi: 10.3109/00207454.2013.823604
52. Lorente L, Martín MM, Ramos L, Argueso M, Cáceres JJ, Solé-Violán J, et al. High serum tissue inhibitor of matrix metalloproteinase-1 levels and mortality in patients with spontaneous intracerebral hemorrhage. World Neurosurg. (2020) 134:e476–80. doi: 10.1016/j.wneu.2019.10.106
53. Levi M, Moons L, Bouché A, Shapiro SD, Collen D, Carmeliet P. Deficiency of Urokinase-type plasminogen activator–mediated plasmin generation impairs vascular remodeling during hypoxia-induced pulmonary hypertension in mice. Circulation. (2001) 103:2014–20. doi: 10.1161/01.CIR.103.15.2014
54. Liu Z, Zhang R, Chen X, Yao P, Yan T, Liu W, et al. Identification of hub genes and small-molecule compounds related to intracerebral hemorrhage with bioinformatics analysis. PeerJ. (2019) 7:e7782. doi: 10.7717/peerj.7782
55. Suzan V, Yavuzer H, Bag Soytas R, Bektan Kanat B, Arman P, Emiroglu Gedik T, et al. The relationship between primary sarcopenia and SARC-F, serum MMP9, TIMP1 levels, and MMP9/TIMP1 ratio in the geriatric patients. Eur Geriatr Med. (2021) 12:1229–35. doi: 10.1007/s41999-021-00519-y
56. Song G, Xu S, Zhang H, Wang Y, Xiao C, Jiang T, et al. TIMP1 is a prognostic marker for the progression and metastasis of colon cancer through FAK-PI3K/AKT and MAPK pathway. J Exp Clin Cancer Res. (2016) 35:148. doi: 10.1186/s13046-016-0427-7
57. Fan C, Zhang X, Zhang P, Zhao J, Shen H, Zhang Y, et al. LPS stimulation during HCV infection induces MMP/TIMP1 imbalance in macrophages. J Med Microbiol. (2020) 69:759–766. doi: 10.1099/jmm.0.001185
58. Barton SJ, Koppelman GH, Vonk JM, Browning CA, Nolte IM, Stewart CE, et al. PLAUR polymorphisms are associated with asthma, PLAUR levels, and lung function decline. J Allergy Clin Immunol. (2009) 123:1391–400.e17. doi: 10.1016/j.jaci.2009.03.014
59. Xu J, Li W, Bao X, Ding H, Chen J, Zhang W, et al. Association of putative functional variants in the PLAU gene and the PLAUR gene with myocardial infarction. Clin Sci. (2010) 119:353–9. doi: 10.1042/CS20100151
60. Egervall K, Rosso A, Elmståhl S. Association between cardiovascular disease- and inflammation-related serum biomarkers and poor lung function in elderly. Clin Proteom. (2021) 18:23. doi: 10.1186/s12014-021-09329-7
61. Nuti E, Rossello A, Cuffaro D, Camodeca C, Van Bael J, van der Maat D, et al. Bivalent inhibitor with selectivity for trimeric MMP-9 amplifies neutrophil chemotaxis and enables functional studies on MMP-9 proteoforms. Cells. (2020) 9:1634. doi: 10.3390/cells9071634
62. Li Y-Q, Liu R, Xue J-H, Zhang Y, Gao D-F, Wu X-S, et al. Effects of monocyte-endothelium interactions on the expression of type IV collagenases in monocytes. Exp Ther Med. (2015) 9:527–32. doi: 10.3892/etm.2014.2109
63. Mondino A, Blasi F. uPA and uPAR in fibrinolysis, immunity and pathology. Trends Immunol. (2004) 25:450–5. doi: 10.1016/j.it.2004.06.004
64. Thunø M, Macho B, Eugen-Olsen J. suPAR: the molecular crystal ball. Dis Markers. (2009) 27:157–72. doi: 10.1155/2009/504294
65. Stewart CE, Sayers I. Characterisation of urokinase plasminogen activator receptor variants in human airway and peripheral cells. BMC Mol Biol. (2009) 10:75. doi: 10.1186/1471-2199-10-75
66. Knyazev E, Maltseva D, Raygorodskaya M, Shkurnikov M. HIF-Dependent NFATC1 activation upregulates ITGA5 and PLAUR in intestinal epithelium in inflammatory bowel disease. Front Genet. (2021) 12:791640. doi: 10.3389/fgene.2021.791640
67. Discher DJ, Bishopric NH, Wu X, Peterson CA, Webster KA. Hypoxia regulates β-enolase and pyruvate kinase-M promoters by modulating Sp1/Sp3 binding to a conserved GC element. J Biol Chem. (1998) 273:26087–93. doi: 10.1074/jbc.273.40.26087
68. Hota SK, Hota KB, Prasad D, Ilavazhagan G, Singh SB. Oxidative-stress-induced alterations in Sp factors mediate transcriptional regulation of the NR1 subunit in hippocampus during hypoxia. Free Radical Biol Med. (2010) 49:178–91. doi: 10.1016/j.freeradbiomed.2010.03.027
69. Duval E, Bouyoucef M, Leclercq S, Baugé C, Boumédiene K. Hypoxia inducible factor 1 alpha down-regulates type i collagen through Sp3 transcription factor in human chondrocytes: HIF-1 alpha down regulates COL1A1 through Sp3. IUBMB Life. (2016) 68:756–63. doi: 10.1002/iub.1539
70. Carver BJ, Plosa EJ, Stinnett AM, Blackwell TS, Prince LS. Interactions between NF-κB and SP3 connect inflammatory signaling with reduced FGF-10 expression. J Biol Chem. (2013) 288:15318–25. doi: 10.1074/jbc.M112.447318
71. Zheng C, Li D, Zhan W, He K, Yang H. Downregulation of SENP1 suppresses LPS-induced macrophage inflammation by elevating Sp3 SUMOylation and disturbing Sp3-NF-κB interaction. Am J Transl Res. (2020) 12:7439–48.
72. Zhang Y, Li Z, Hao Q, Tan W, Sun J, Li J, et al. The Cdk2–c-Myc–miR-571 axis regulates DNA replication and genomic stability by targeting geminin. Cancer Res. (2019) 79:4896–910. doi: 10.1158/0008-5472.CAN-19-0020
73. Roderburg C, Mollnow T, Bongaerts B, Elfimova N, Vargas Cardenas D, Berger K, et al. Micro-RNA profiling in human serum reveals compartment-specific roles of miR-571 and miR-652 in liver cirrhosis. PLoS ONE. (2012) 7:e32999. doi: 10.1371/journal.pone.0032999
74. Preusse M, Theis FJ, Mueller NS. miTALOS v2: analyzing tissue specific microRNA function. PLoS ONE. (2016) 11:e0151771. doi: 10.1371/journal.pone.0151771
Keywords: ICH, hypoxia, inflammation, SP3, biomarkers, cerebrovascular disease
Citation: Wan D, Feng J, Wang P, Yang Z and Sun T (2022) Hypoxia- and Inflammation-Related Transcription Factor SP3 May Be Involved in Platelet Activation and Inflammation in Intracranial Hemorrhage. Front. Neurol. 13:886329. doi: 10.3389/fneur.2022.886329
Received: 28 February 2022; Accepted: 27 April 2022;
Published: 02 June 2022.
Edited by:
Yuzhen Xu, Tongji University, ChinaReviewed by:
Xinbing Liu, Changxing People's Hospital, ChinaPengpai Zhang, Shanghai Jiao Tong University, China
Copyright © 2022 Wan, Feng, Wang, Yang and Sun. This is an open-access article distributed under the terms of the Creative Commons Attribution License (CC BY). The use, distribution or reproduction in other forums is permitted, provided the original author(s) and the copyright owner(s) are credited and that the original publication in this journal is cited, in accordance with accepted academic practice. No use, distribution or reproduction is permitted which does not comply with these terms.
*Correspondence: Tao Sun, umc9402321@163.com
†These authors have contributed equally to this work