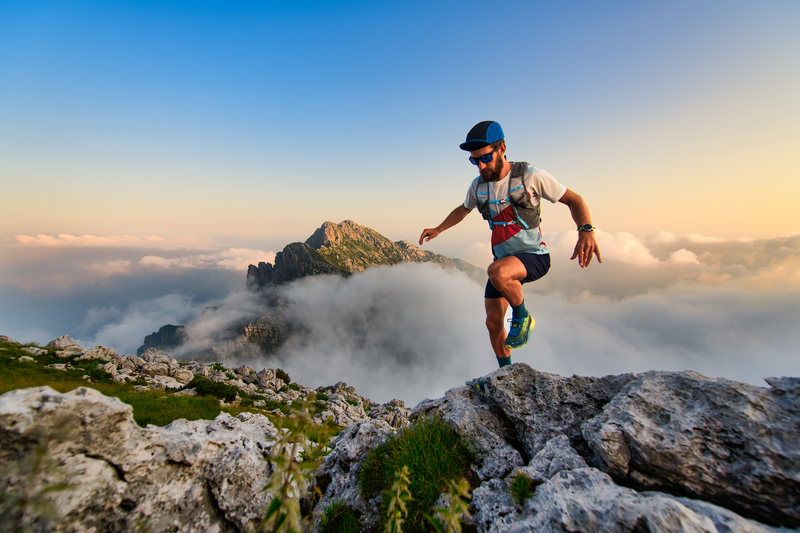
94% of researchers rate our articles as excellent or good
Learn more about the work of our research integrity team to safeguard the quality of each article we publish.
Find out more
REVIEW article
Front. Neurol. , 26 May 2022
Sec. Neuro-Oncology and Neurosurgical Oncology
Volume 13 - 2022 | https://doi.org/10.3389/fneur.2022.865171
This article is part of the Research Topic Advances in Brain Tumors Diagnosis and Treatment View all 27 articles
Gliomas are a heterogenous group of central nervous system tumors with different outcomes and different therapeutic needs. Glioblastoma, the most common subtype in adults, has a very poor prognosis and disabling consequences. The World Health Organization (WHO) classification specifies that the typing and grading of gliomas should include molecular markers. The molecular characterization of gliomas has implications for prognosis, treatment planning, and prediction of treatment response. At present, gliomas are diagnosed via tumor resection or biopsy, which are always invasive and frequently risky methods. In recent years, however, substantial advances have been made in developing different methods for the molecular characterization of tumors through the analysis of products shed in body fluids. Known as liquid biopsies, these analyses can potentially provide diagnostic and prognostic information, guidance on choice of treatment, and real-time information on tumor status. In addition, magnetic resonance imaging (MRI) is another good source of tumor data; radiomics and radiogenomics can link the imaging phenotypes to gene expression patterns and provide insights to tumor biology and underlying molecular signatures. Machine and deep learning and computational techniques can also use quantitative imaging features to non-invasively detect genetic mutations. The key molecular information obtained with liquid biopsies and radiogenomics can be useful not only in the diagnosis of gliomas but can also help predict response to specific treatments and provide guidelines for personalized medicine. In this article, we review the available data on the molecular characterization of gliomas using the non-invasive methods of liquid biopsy and MRI and suggest that these tools could be used in the future for the preoperative diagnosis of gliomas.
Gliomas originate from glial precursor cells and comprise 27% of all primary brain tumors (1). The World Health Organization (WHO) guidelines divides gliomas into diffuse and circumscribed, and diffuse gliomas are now classified as either adult- or pediatric-type diffuse gliomas. In the 2021 WHO classification, adult-type diffuse gliomas are subclassified as astrocytoma, IDH-mutant (grade 2, 3, or 4); oligodendroglioma, IDH-mutant and 1p/19q-codeleted (grade 2 or 3); molecular glioblastoma (lower grade astrocytoma with chromosome 7 gains/chromosome 10 losses, epidermal growth factor receptor (EGFR) amplification, and/or telomerase reverse transcriptase (TERT) and glioblastoma, IDH-wildtype (grade 4). Pediatric-type diffuse gliomas are further subdivided into low- and high-grade tumors. Most pediatric-type gliomas are new, although previously known entities, such as diffuse midline glioma, H3 K27-altered are also included (2). Glioblastoma, the most common malignant central nervous system (CNS) tumor in adults, accounts for 48.6% of all CNS tumors and 57.7% of all gliomas, while the remaining 42.3% are other histologies and grades, with a different prognosis and different treatment options. The standard treatment for gliomas is only follow-up in some resected diffuse gliomas without a high recurrence risk or a combination of surgery, which is essential both to obtain tissue for diagnosis and to debulk the tumor, followed by irradiation and/or chemotherapy depending on the tumor grade and molecular characterization and on patient clinical features (3).
The correct diagnosis and treatment of gliomas is based on what is known as an “integrated diagnosis,” which combines the WHO CNS grade, histologic, and molecular information (2). Glioma grading was traditionally a strict morphological parameter that took into account cell pleomorphism, mitotic activity, vascular proliferation and necrosis. Histological subtyping was also traditionally based on the morphological aspect of tumor cells—whether they were more similar to astrocytes or oligodendrocytes. This traditional method of determining the histopathological diagnosis has several drawbacks, such as intra-tumoral spatial heterogeneity and sampling errors, which are often due to the difficulty of obtaining sufficient tissue in deep tumors or those located in eloquent areas, where surgical resection is limited. These factors have led to high intra-and inter-observer variability in diagnosis (4). The molecular characterization of gliomas has important implications for patient prognosis, treatment planning, and prediction of treatment response and also reduces the variability in diagnosis. Several molecular biomarkers were already incorporated in the 2016 WHO guidelines for gliomas and newly identified biomarkers have been introduced into the WHO 2021 classification. These biomarkers can help to better define both the grade and the histological subtype of diffuse gliomas (5).
Standard clinical protocols for the evaluation of molecular alterations in gliomas are usually based on tissue biopsies (2) but other techniques, such as liquid biopsies and radiomics/radiogenomics, are showing promise. A liquid biopsy enables the analysis of tumor products shed in body fluids and its growing use in other tumors to provide diagnostic and prognostic information and real-time information on tumor status makes it a promising method in gliomas, as well (6–9). Magnetic resonance imaging (MRI), the method of choice for the preoperative assessment of brain tumors, provides valuable information on whole tumor structure and composition, physiology, hemodynamics and microenvironment at voxel level. In addition, diffusion-weighted imaging (DWI), perfusion-weighted imaging (PWI), diffusion tensor imaging (DTI), and spectroscopy (MRS) have improved the imaging characterization of the tumor (10). The digitization of images has led to the development of radiomics, which studies the link between imaging and different phenotypes, and radiogenomics, which can predict the status of molecular markers, genetic mutations and chromosomal aberrations by using imaging features as a surrogate for the presence of these genetic alterations (11, 12). Growing evidence suggests that the underlying gene alteration patterns that steer the characteristics and morphological features of gliomas can be captured by quantitative imaging (13). Artificial intelligence (AI), machine learning and, more recently, deep learning are techniques based on the study of the image. They apply progressively more complicated readings of imaging features and computational processes, which could potentially lead to a highly accurate prediction of the molecular alterations that are currently mandatory for the correct diagnosis of gliomas (5, 14–16).
In summary, liquid biopsy and radiomics/radiogenomics—both individually and in combination—can potentially achieve a non-invasive diagnosis of disease and provide guidance in treatment planning. This is of special interest in brain tumors given the invasiveness of the common procedure for diagnosis and obtaining tumor samples and especially in tumors located in difficult to access locations where biopsy is not exempt from the risk of causing severe neurological lesions such as in midline tumors. Here we review current data on the use of liquid biopsy and radiogenomics in the characterization of gliomas.
The 2016 WHO Classification of Tumors of the Central System (17) defined diagnostic entities combining molecular and histological data. The accelerated understanding of the additional molecular characteristics of brain tumors made it necessary to update this classification and led to the creation of the Consortium to Inform Molecular and Practical Approaches to CNS Tumor Taxonomy (cIMPACT-NOW), which reported seven updates that have now been incorporated in the current WHO 2021 classification (2, 18). In fact, molecular studies are currently mandatory for the correct diagnosis of gliomas in adults and children since they clarify diagnosis and better define prognosis, leading to the optimal therapeutic decision for each patient and tumor subtype (3, 19). Once a brain tumor has been confirmed as a glioma, several molecular alterations are now essential for assigning the grade and histological subtype and for reaching an integrated diagnosis (Table 1).
Mutations in the NADP+ dependent isocitrate dehydrogenase genes IDH1 and IDH2 are involved in the pathogenesis of a subgroup of diffuse and anaplastic gliomas. After they were first characterized (20, 21) and their mechanistic role described (22), the assessment of IDH mutations became an important tool in the diagnosis of gliomas. IDH mutations are associated with longer survival than wild-type IDH regardless of tumor grade. They are present in all oligodendrogliomas by definition (oligodendroglioma, IDH-mutant and 1p/19q-codeleted), in most low-grade diffuse astrocytomas in adults and in a subset of glioblastomas that are now renamed astrocytoma, IDH-mutant, grade 4 (2). There are three isoforms of the IDH gene, of which the most important in gliomas are cytosolic IDH1 and mitochondrial IDH2 mutations; most IDH-mutated gliomas harbor IDH1 mutations in the form of IDH (R132H). (23) A routine use of immunohistochemistry (IHC) to determine the presence of IDH (R132H) is recommended. IDH1-IDH2 sequencing is mandatory in the case of lack of immunopositivity, in order to rule out the presence of non-canonical mutations in all glioblastomas in patients younger than 55 years, in those with loss of ATRX expression, in those with a previous history of a lower-grade glioma, and in all grade 2 or 3 diffuse gliomas. Hotspot mutations are described for both IDH1 (R132) and IDH2 (R172) and are mutually exclusive. IDH2 tumors may have different outcomes than IDH1 tumors. (24, 25) IDH assessment can distinguish diffuse gliomas with IDH mutations from glioblastoma, IDH-wildtype and other types of IDH-wildtype gliomas, including diffuse midline glioma, H3 K27-altered, and diffuse hemispheric glioma, H3 G34-mutant.
Mutations in the alpha-thalassemia/mental-retardation-syndrome-X-linked (ATRX) gene are frequent in astrocytoma, IDH-mutant (more than 90% of cases). They usually coexist with TP53 mutations and are mutually exclusive with 1p/19q codeletions. ATRX mutations are also frequently seen (95%) in diffuse hemispheric glioma, H3 G34-mutant, with wild-type IDH. In contrast to IDH mutations, hotspot ATRX mutations do not occur so all of the gene should be sequenced to rule out mutations. ATRX mutations are usually detected by a loss of ATRX expression by IHC, which can serve as a surrogate of ATRX mutation analysis (2, 26, 27). In an IDH-mutant glioma, the loss of nuclear ATRX immunopositivity is indicative of an astrocytic lineage and thus precludes the need for 1p/19q analysis.
TP53 is a tumor suppressor gene encoding a tumor suppressor protein (p53) that responds to cellular stress by inducing cell cycle arrest, apoptosis, senescence, DNA repair and metabolism changes (28). Although not specific, TP53 mutations are more frequently seen in astrocytoma, IDH-mutant (more than 90% of cases) and, like ATRX mutations, also in diffuse hemispheric glioma, H3 G34-mutant (90%). In daily practice, the TP53 mutation is detected by IHC, where a pattern of more than 10% of tumor cells with strong nuclear positivity or a complete negative stain indicates the presence of a TP53 mutation. As in the case of ATRX mutations, TP53 mutations can occur throughout the gene, with no known hot spot (2).
After the allelic deletions of 1p and 19q were first identified and associated with chemosensitivity, the determination of the concomitant 1p/19q codeletion was deemed essential for the diagnosis of gliomas, as it is one of the defining criteria of oligodendroglioma (29, 30). The presence of the 1p/19q codeletion is used to distinguish oligodendroglioma, IDH-mutant and 1p/19q-codeleted from astrocytoma, IDH-mutant and from other non-glial brain tumors. Although different techniques can be employed, one of the most frequently used is fluorescent in situ hybridization (FISH).
Amplification of the EGFR is one of the most frequent genetic alterations associated with glioblastoma. EGFR amplification results in overexpression of the EGFR transmembrane kinase receptor (31). More than 50% of glioblastomas with EGFR amplification also contain the EGFRvIII gene mutation, which is characterized by the deletion of exons 2 to 7, resulting in a sense mutation with a truncated extracellular domain and ligand-independent constitutive activity (32). EGFR amplification occurs in 40–50% of morphologically defined glioblastoma, IDH wildtype and in a subset of what had previously been classified as IDH-wildtype lower-grade (grade 2 or 3) diffuse astrocytomas (16) which are currently classified as molecular glioblastomas, if they have certain molecular alterations, such as EGFR amplification, TERT promoter mutation and/or +7/−10 signature that confer them a prognosis similar to that of classical glioblastoma (5). EGFR amplification is usually detected by FISH, while reverse transcription polymerase chain reaction (RT-PCR) could constitute a good option for the detection of the EGFRvIII mutation.
Mutations in the promoter of TERT commonly occur in diffuse gliomas (28, 33) but are also present in other types of brain tumors, such as pleomorphic xantoastrocytomas and ependymal tumors. TERT promoter mutations occur in about 70% of glioblastoma, IDH-wildtype and in >95% of oligodendrogliomas, IDH-mutant and 1p/19q-codeleted. As is the case with EGFR amplification, diffuse gliomas formerly classified as IDH-wildtype grade 2–3 diffuse astrocytomas with TERT promoter mutations are now classified as glioblastomas, IDH-wildtype (5). However, some recent studies suggest that in the specific case of grade 2 astrocytomas, the presence of TERT promoter mutations as the only high-grade factor does not seem to justify classification as grade 4 (34). In the case of TERT promoter mutation two different hotspot mutations have been described for TERT: C228T and C250T.
Chromosome 7 harbors genes encoding the Platelet Derived Growth Factor Subunit A (PDGFA) and EGFR, while chromosome 10 harbors the Phosphatase and Tensin Homolog (PTEN) and MGMT. The combination of whole chromosome 7 gain and whole chromosome 10 loss is known as the +7/−10 signature. It is present in 79% of glioblastoma, IDH-wildtype (35) and constitutes the third molecular criteria to define glioblastoma, IDH-wildtype in an otherwise IDH-wildtype morphologically grade 2–3 diffuse astrocytoma (5).
The BRAFV600E mutation is rare in adult-type diffuse gliomas and can occasionally be used to distinguish pilocytic astrocytoma or pleomorphic xanthoastrocytoma from a diffuse astrocytoma (36), even though BRAF fusions are more frequent than this mutation in these subtypes. It can help to identify epithelioid glioblastoma (37) and a subset of pediatric diffuse low-grade gliomas and glioneuronal tumors (2). It is an oncogenic driver mutation and can have consequences in treatment with promising success (38).
H3F3A histone mutations affect two critical amino acids, K27 (K28M) and G34 (G35R/V), and define two pediatric-type diffuse high-grade gliomas: diffuse midline glioma, H3 K27-altered, and diffuse hemispheric glioma, H3 G34-mutant. H3F3A histone mutations are mutually exclusive with IDH mutations (39) and, like IDH mutations, can be detected by IHC or by sequencing. They are more common in children and young adults, although they can be seen at any age. Gliomas with these mutations are now classified as grade 4 (5). The term diffuse midline glioma has now been expanded to incorporate cases with H3.1 or 3.2 mutations and H3-wildtype with EZHIP overexpression and EGFR mutations (2). However, other types of gliomas, like pilocytic astrocytomas, and glioneuronal tumors can harbor H3K27M mutations so the term diffuse midline glioma should be restricted to cases located in midline and radiologically infiltrating.
Multiple studies have identified the homozygous deletion of CDKN2A/B as a marker of poor prognosis in patients with IDH-mutant astrocytomas, and a correlation with shorter survival has been confirmed in several studies (40, 41). The 2021 WHO classification considers the homozygous deletion of CDKN2A/B as a molecular feature of grade 4 in IDH-mutant astrocytomas and also as a molecular feature of grade 3 oligodendroglioma (5). Most laboratories use FISH for CDKN2A assessment in daily practice.
Methylation of the promoter of O(6)-methylguanine-DNA methyltransferase (MGMT) is directly related to the silencing of the repair protein MGMT, which leads to a special sensitivity to alkylating therapy, the current mainstay of glioma treatment (42). MGMT promoter methylation is a recognized prognostic and predictive marker of response to chemotherapy. Several methods can be employed for the determination of MGMT methylation status, with the most frequent being methylation-specific PCR (MS-PCR), pyrosequencing, or multiplex-ligation dependent probe amplification.
Glial fibrillary acidic protein (GFAP) is encoded by the GFAP gene and is expressed in different proportions by both non-tumoral and tumoral glial cells. While its expression is not diagnostic of a specific tumor, it is it is a very useful marker to distinguish a primary from a secondary metastatic tumor.
The presence of circulating cell-free DNA (cfDNA) in healthy individuals and patients was first described by Mandel and Metais (43) and has since been analyzed in different scenarios. For example, aneuploidies were identified in fetal cfDNA using diagnostic kits during pregnancy, and increased levels of cfDNA were observed in autoimmune rheumatic diseases, trauma, burn injuries, sepsis, and myocardial infarction (44). The identification of circulating tumor DNA (ctDNA) occurred later when certain gene alterations found in the tumor, such as KRAS mutations, were detected in the blood of patients (45). The term “liquid biopsy” for the study of ctDNA was first introduced in 2010, with reference to circulating tumor cells found in the peripheral blood of cancer patients (46). Today the concept of liquid biopsy encompasses multiple biological fluids, including blood, urine, cerebrospinal fluid (CSF), and pleural fluid. The analysis of a liquid biopsy can identify multiple molecular alterations in different components released by the tumor and can provide information on DNA, RNA, proteins, carbohydrates, lipids, and metabolites and even tumor cells released by the tumor (Figure 1).
Figure 1. Elements of the liquid biopsy. B lymph, B lymphocyte; CSF, cerebrospinal fluid; cfDNA, cell-free DNA; CTC, circulating tumor cells; ctDNA, circulating tumor DNA; lnc RNA, long non-coding RNA; NK, natural killers; TEP, tumor-educated platelets; T lymph, T lymphocyte; miRNA, microRNA. Extracellular vesicles include exosomes, microvesicles and apoptotic vesicles.
The information obtained from these components has evolved from the identification of single DNA aberrations to more extensive analyses evaluating multiple genes simultaneously by next generation sequencing (NGS) of DNA and RNA. Multiple different NGS methods can provide information on the genome, transcriptome and epigenetic mechanisms that modify genomic information. As such a rich source of biomarkers, the liquid biopsy can be used for screening, early diagnosis, analysis of the molecular heterogeneity of the tumor during its evolution, evaluation of early response, patient follow-up, monitoring of residual minimal disease, real-time analysis of the evolution of treatment resistance, and identification of actionable genetic alterations (47).
Nonetheless, while liquid biopsies have long been used in the diagnosis and monitoring of several cancers, it is only recently that this technique has become the subject of intensive research in CNS tumors (6–9), where both blood and CSF have been shown to provide crucial genomic information (48–51). Tables 2, 3 summarize the most salient studies of molecular alterations identified in liquid biopsies of glioma patients.
Tumors leave traces of their presence by releasing various tumor components, including cells or their fragments, DNA, and RNA. These components have different molecular characteristics than those in healthy tissue and can thus be easily identified in various fluids and be used to identify molecular alterations in the tumor itself. Among the components that carry information from the tumor are ctDNA, circulating tumor RNA (ctRNA), microRNAs (miRNAs), circulating tumor cells (CTCs), extracellular vesicles, tumor-educated platelets (TEPs), and proteins.
DNA and RNA are freely present in blood and CSF as the result of the normal process of digestion, necrosis and apoptosis of both normal and cancer cells (86). The concentration of cfDNA is higher in cancer patients than in healthy individuals and is directly proportional to tumor burden (45) although ctDNA represents <1% of total cfDNA (87). Both cfDNA and ctRNA can be captured, amplified and analyzed to identify molecular alterations specific to certain types of gliomas, such as loss of heterozygosity (LOH), copy number variations (CNVs) in microsatellites, gene mutations, and epigenetic alterations like methylation of tumor suppressor genes. In addition, liquid biopsies can be used for the study of multiple genes by NGS, WES and genome wide methylation profiling (75, 76, 79, 80, 82, 85, 88) (Tables 2, 3).
miRNAs are small non-coding RNAs of about 21–25 nucleotides that modulate gene transcription and expression. They negatively regulate genes at the mRNA and protein levels by degrading their mRNA target or by silencing translation (89). They are involved in multiple cellular processes, including development, apoptosis, proliferation and differentiation, and can act as tumor suppressor genes or oncogenes in several cancers, including gliomas. While mRNA is rapidly degraded by blood RNAses, miRNAs are resistant to these enzymes and are easily detectable in biological fluids (90). Different miRNA signatures associated with specific tumor types have been related to cancer diagnosis and prognosis. The isolation of miRNAs is of special interest due to their frequent deregulation in cancer, their stability in paraffin-embedded tumor tissue and in blood, and their specific profile for each tumor type (91). miRNAs were differentially detected in the blood of glioblastoma patients and in that of healthy controls (92), and miRNAs detected in CSF were able to differentiate between a metastatic brain injury and glioblastoma (93). In addition, specific miRNAs have been suggested as potential biomarkers for the diagnosis and prognosis of gliomas (94, 95).
lncRNAs are non-coding RNAs of ≥200 nucleotides that modulate key molecules at every step of cancer metastasis, including dissemination of carcinoma cells, intravascular transit, and metastatic colonization. Their important role in cancer has recently been recognized (90) and is now being intensely investigated, including in glioblastoma, where they have been detected in the serum of patients (96).
CTCs can be released into the circulation as single cells or clusters of cells from either the primary tumor or metastases. They have been found in several tumor types and are associated with poor outcome and metastasis. Patients with metastatic disease can have up to 10 CTCs per mL of blood, while they are rarely found in healthy individuals (97). CTCs can be isolated through different techniques and commercial platforms, most of which are based on autoantibodies able to detect cell surface proteins to capture CTCs, such as anti-epithelial cell adhesion molecule (EpCAM), and the absence of expression of CD45, a marker of lymphocyte antibodies (98). However, since CTCs are not present in early-stage disease, their usefulness in diagnosis or detection of early relapse is limited (99). In gliomas, systemic metastases are anecdotal, yet glioblastoma sheds CTCs with invasive mesenchymal characteristics into the circulation (100, 101). These cells seem to undergo epithelial-mesenchymal transition, which gives them a mesenchymal phenotype and increased migratory potential (101–103). Few studies have reported different methods for CTC enrichment and identification in gliomas (98, 100, 104–106), mainly due to the fact that the methods used to isolate these cells generally rely on EpCAM, an epithelial marker that is not expressed in glioblastoma cells.
Extracellular vesicles include exosomes (30–100 nm in diameter), microvesicles (100–500 nm), and apoptotic vesicles (>500 nm). A recent consensus recommended classifying them as “small” or “medium/large” according to their physical and biochemical characteristics in order to standardize research methods and results (107). These vesicles are released by normal and tumor cells into the cellular microenvironment and biological fluids and carry in their interior a variety of molecules representative of their cells of origin (108), including fragments of RNA and DNA. Their stability and membranous envelope protect their cargo from nucleases, proteases and other degradation enzymes that are found in the extracellular medium (109), making them a good source of tumor molecular signatures. In glioma patients, circulating extracellular vesicles have been found in CSF and in peripheral blood, indicating that they seem to cross the intact blood-brain barrier (BBB) (56, 59, 60, 62, 81, 110).
Platelets are part of the tumor microenvironment and participate in tumorigenesis, progression, and treatment response (111). The RNA, DNA and proteins released by tumors can be sequestered by platelets, which integrate them in their own genetic material. This provides the platelets with a highly dynamic repertoire of spliced RNA with different functions, giving rise to the concept that tumors can “educate” platelets and tumor-derived alterations can then be studied in blood (63, 112). mRNA sequencing of TEPs has identified differential mRNA profiles in several cancers when compared to healthy individuals (111, 113). TEPs have been reported to play a role in angiogenesis and tumor aggressiveness in gliomas (114).
Proteins can be detected in blood as a normal event or as an indication of abnormal processes in the brain. GAFP, an intermediate filament highly expressed in glial cells, is the protein most studied in gliomas, but other proteins related to brain tumors have also been studied in both blood and CSF (73, 74, 115, 116). The cut-off point of the protein concentration in blood for the diagnosis of brain tumors varies across different studies (73, 74). Nevertheless, the level of some proteins seems to correlate with tumor grade and volume, indicating that they could be useful as a predictor of tumor grade (73). In addition to GAFP, several proteins that are potentially important in gliomas are myelin basic protein, vascular endothelial growth factor, YKL-40, matrix metallopeptidase-9, interleukin 6, 2-hydroxyglutarate (2-HG) (as a surrogate of IDH mutations), histidine, and tryptophan (117, 118).
ctDNA has been detected in blood in <10% of patients with gliomas (119) but at a higher rate in CSF. The amount of ctDNA released in blood and CSF depends on tumor size and grade and, importantly, on the distance from the tumor to the ventricular system (53, 67), and the ctDNA seems to be released from the tumor directly into the CSF, rather than reaching it indirectly through plasma (49, 50, 53, 76). Additionally, the amount of ctDNA obtained may be different if the CSF is obtained intraoperatively, from lumbar puncture, or from ventricular-peritoneal shunts (57, 82). Lumbar puncture is more aggressive than blood extraction and would be contraindicated in patients with cerebral edema, large tumors, hydrocephalus, or midline deviation (82). Moreover, lumbar puncture is difficult to implement serially in cases where the liquid biopsy is used for monitoring response. In contrast, peripheral blood can be obtained non-invasively and used in longitudinal studies for patient monitoring, making it a more convenient alternative for obtaining genomic information on the tumor. Nevertheless, there are also several problems involved in studying ctDNA in blood, including the variable sensitivity of the different techniques, interobserver differences in the interpretation of findings, divergent thresholds used in different studies, and the short half-life (<1.5 h) of ctDNA, which is rapidly cleared from the blood through the liver and kidney (120). The lack of permeability of the BBB may also be an impediment to the release of ctDNA into blood, although several studies in animals and humans have shown that exosomes, microvesicles, and apoptotic vesicles can all cross the intact BBB (56, 121). However, ctDNA fragments are shorter than circulating non-tumor DNA and specific alterations could well not be present in the circulating ctDNA fragment (122) although the sensitivity of the procedure could be improved with selective sequencing and enhanced identification of ctDNA (122, 123). In spite of all these drawbacks, some studies have detected somatic alterations with NGS, and genome wide methylation profiling with new techniques has recently been shown to deliver reliable results with only small amounts of DNA (124) from blood ctDNA or exosomes (83–85) (Table 3). Taken together, all these findings suggest that at present, peripheral blood is the source where further investigation and development should be focused Figure 2.
Figure 2. Liquid biopsy obtained from blood or CSF. BBB, brain-blood barrier; CSF, cerebrospinal fluid; ctDNA, circulating tumor DNA; GWM, genome wide methylation; NGS, next generation sequencing; VPS, ventricular-peritoneal shunt.
Computed tomography (CT) and especially MRI are the standard imaging tests for diagnosis, follow-up and treatment planning in brain tumors (125, 126). Other techniques, including amino acid positron emission tomography (PET), PET/CT and PET/MRI are very slowly being incorporated into the evaluation of brain tumors and are also used in radiogenomics. Table 4 summarizes the most salient studies of tumor subtype and molecular alterations in gliomas detected by conventional radiology and radiogenomics on MRI images.
Table 4. Tumor grade and molecular alterations identified by imaging with conventional radiology and radiogenomics.
Although CT is not the test of choice for the diagnosis and follow-up of gliomas, it has an essential role in the emergency department due to its greater availability and faster image acquisition, which make it possible to diagnose space-occupying lesions in patients who develop neurological focality or focal epileptic seizures. It can also detect certain components of the lesion that may help to identify the type of tumor, such as hyperdensity in lymphomas or gross calcifications in certain types of gliomas (those previously classified as oligodendrogliomas). CT is also a useful tool for the rapid detection of complications during clinical follow-up, including spontaneous, post-surgical and post-treatment complications.
MRI provides extensive qualitative and quantitative data about tumor characteristics in terms of volumetry, microstructure, hemodynamics and metabolism; it is used for confirmation, final radiological diagnosis, surgical and radiation therapy planning, and patient follow-up. Conventional MRI sequences commonly used for the evaluation of intracranial tumors include T1-weighted (T1WI), T2-weighted (T2WI), fluid attenuated inversion recovery (FLAIR), T2* gradient echo and post-contrast T1WI images. These sequences provide exquisite anatomic detail, and the use of a gadolinium-based contrast agent in this protocol allows for the detection of areas where the BBB is compromised. Advanced MRI techniques offer the ability to assess pathophysiological properties of the lesion that may yield important information on tumor infiltration and aggressiveness and treatment response, thus providing a better understanding of underlying tumor biology.
Advanced MRI techniques include DWI, PWI, DTI, and MRS, which are already established as tools for the evaluation of brain tumors. Cystic and necrotic areas allow for more free diffusion of water molecular in comparison with intact tissue, resulting in high apparent diffusion coefficient (ADC) values. In solid tumor tissue, the main factor affecting ADC is the size and complexity of the extracellular space. Increased cell density will limit the extracellular space, suggesting that ADC can be used as an indirect measurement of cellularity (162). PWI can be used to assess the microvascular environment and provide information on tumor grade, treatment response and tumor aggressiveness. Several forms of PWI have been developed. Dynamic susceptibility contrast (DSC) and dynamic contrast-enhanced (DCE) imaging are dependent on the intravenous injection of gadolinium-based contrast agents, whereas arterial spin labeling (ASL) can be acquired without injectable contrast as it uses magnetic labeling of endogenous protons in blood to assess blood volume flow and flow rate. DTI can be used to detect and predict the invasive growth patterns of high-grade gliomas (163). MRS assesses the presence of certain metabolites, which resonate at different frequencies. The main metabolites detected by MRS are choline, N-acetylaspartate (NAA), creatinine, lipids/lactate, and myo-inositol. Choline is a component of the cell membrane and a marker of cell turnover; NAA is a marker of neuronal viability; creatine is important in energy transfer and a stable constant from which ratios are calculated; lipids/lactate are markers of severe cell damage and necrosis; and myo-inositol is a glial lineage marker.
Digitalization has made it possible to store images for post-processing, share data, and create communication networks. As a wealth of information can be extracted from each image, it was a logical next step to employ AI to analyze imaging data. This led to the creation of radiomics, which can extract a large number of features from medical images. Radiomics began at the beginning of the century and has experienced exponential growth in recent years as computing technologies have improved (164). Radiogenomics, a subdiscipline of radiomics, predicts the status of molecular markers, genetic mutations, and chromosomal aberrations in the tissues examined by MRI or PET.
The process of radiomics includes image acquisition, image segmentation, feature extraction, feature selection and informatics. AI can be used to automate the slow process of image segmentation, where the image is decomposed into natural units to distinguish normal tissues like gray matter, white matter and CSF from possible pathological tissues like tumors and edema. The findings would require only validation by the clinician, which would increase the comparability of the results since they would be independent of each radiologist's experience (165).
Feature extraction can be done on one or several previously segmented region-of-interest or volume-of-interest, which would eliminate the problem of tumor heterogeneity to a great extent. With AI, additional information can be extracted on specific quantitatively or semi-quantitatively measurable traits and features that are impossible to detect with the human eye, thus providing superior assessment of imaging findings than would be possible by a radiologist (166). Feature extraction by shape, histogram or texture on particular sequences was first performed manually by predefinition (hand-crafted radiomics). Later, machine learning gave computer systems the ability to recognize patterns among thousands of imaging features and make predictions without being explicitly programmed. Subsequently, deep learning radiomics extracted high-dimensional features from the input images at different levels of scaling and abstraction, such as convolutional neural networks (CNNs) or auto-encoders to define the most relevant features of the input data. CNNs are an adaption of the traditional artificial neural network architecture whereby banks of 2D convolutional filter parameters and non-linear activation functions act as a mapping function to transform a multidimensional input image into a desired output (167). A cascaded system of single-layer neural networks is trained to learn and identify structures in the image with data that are relevant for classification without a prior definition or selection of the feature. Once the network is trained, it can go through the process of model generation without previous selection of features and can be applied to different cases or MRIs.
After feature extraction, the next step is selection of the most important features by eliminating redundancy. Finally, univariate and multivariate methods are applied, including linear and logistic regression, decision trees (e.g., random forests), support vector machines, neural network, and Cox proportional hazards models for survival data, in order to build a model that predicts a particular genetic mutation or other molecular alteration raised. Features should first be obtained from a training set and the most accurate predictive model is obtained from the training set data. This model should then be applied to a validation set to check the reproducibility of the data and to estimate the model performance. Finally—and ideally—the model should be applied to a test dataset that represents real-world data, where different scanners, acquisition protocols and/or segmentation differences coexist, before it is applied in routine clinical practice (165).
In summary, over the years, conventional radiology has made it possible to identify some characteristics of specific histologies. With the advanced methods of AI, like machine learning and deep learning, interest has moved to identify not only histologies but also molecular alterations that could contribute to the diagnosis of gliomas. Importantly, the predictive models obtained through image extraction and selection could be integrated in future MRI machines or PET scanners as useful complements to diagnosis, paving the way for the non-invasive diagnosis of brain tumors. There is also a need for future studies of radiogenomics focusing on the concept of personalized medicine. This approach could help to identify imaging phenotype variations over time that would allow non-invasive longitudinal monitoring of mutational status and hence evaluation of treatment response. In order to optimize machine-learning technology, there is a need to share diverse datasets across institutions. Cooperation between centers and institutions is essential to fulfill this objective. Radiogenomics can expand synergistic analyses of imaging, histopathologic, genetic, and clinical data, which will speed up scientific discovery, lead to quantitative integrated evaluation of patient data and contribute to improving personalized and precision medicine. These integrated diagnostic approaches can enhance the specificity of imaging phenotypes associated with molecular signatures in patients with glioma.
Here we review selected studies of liquid biopsy and radiogenomics focused on the non-invasive diagnosis of diffuse gliomas and the identification of their inherent characteristics, such as tumor grade and molecular alterations. We selected studies using liquid biopsy or radiogenomics oriented toward the determination of tumor grade or molecular alterations identified as mandatory by the WHO 2021 classification (5). We included studies of MRI-based radiogenomics that included a training set and a validation set. We did not include PET-based radiogenomics studies. We have focused our review on studies reporting data for diagnosis—not for follow-up or for pseudoprogression, recurrence or radionecrosis (Tables 2–4).
Grade has traditionally been a strictly morphological diagnosis related to atypia, mitosis, vascular proliferation, and necrosis. In the 2021 WHO classification, however, several molecular alterations have been included: EGFR amplification, TERT promoter mutation and/or +7/-10 signature for glioblastoma, IDH-wild-type; and homozygous deletion of CDKN2A/B for astrocytoma, IDH-mutant, grade 4 and for oligodendroglioma, IDH-mutant and 1p/19-codeleted, grade 3 (5). The determination of tumor grade by liquid biopsy is not based on the morphological parameters defined for tissue but on the detection of these molecular alterations in blood or CSF.
Several features related to high-grade tumors, especially glioblastoma, can be identified by MRI: contrast enhancement, ring appearance, satellite lesions, necrosis, ill defined infiltration, abundant edema, and heterogeneous areas of hypo- or non-enhancing tumor infiltration involving the cortex and deep nuclei (125).
Ki67 is a marker of cell proliferation; higher values are associated with a higher proliferation index and consequently a higher tumor grade. A high proliferation index was related to vascularization and to a higher relative cerebral blood volume (rCBV) in glioblastoma (13, 168), and an inverse correlation was seen between the Ki-67 proliferation index and the apparent diffusion coefficient (ADC) across glioma grades (13). Positive correlations between the Ki-67 proliferation index and metabolite alterations of choline over creatine ratio, lactate over creatine ratio, and myo-inositol were observed with MRS (169). Elevated choline with cell proliferation and malignancy was linked to oncogenic transformation triggered by hypoxia, while a decrease in choline levels was related to necrosis (170).
Tumor grade has been studied by several radiogenomic studies with different methodologies and in gliomas of all grades. Two of the studies included a validation set. The models in these studies reached an AUC of 0.79–0.89 with an accuracy of 0.81–0.9 and a reported sensitivity of 88% and specificity of 90% (127, 128).
IDH mutations have been studied extensively by liquid biopsy and MRI because of their importance in diagnosis, treatment selection and prognosis. IDH mutations in ctDNA have been assessed in both blood and CSF with a sensitivity of 62–80% and specificity of 76–100% (52, 53, 57). Results were slightly superior in CSF but the study of extracellular vesicles in blood showed a sensitivity of 80%, making it an interesting method to further explore (56). Other liquid biopsy studies detected the oncometabolite 2-HG, which is highly specific for IDH1/2-mutant tumors (54, 55). NGS studies detected IDH mutations in ctDNA and extracellular vesicle RNA, using microarrays and genome wide methylation profiling (67, 75, 79, 80, 82, 83). However, most of these studies were exploratory and sensitivity and specificity data were not reported.
Tumors with IDH mutations are usually located in the frontal lobe (126, 171, 172). IDH mutations can be seen in astrocytomas or oligodendrogliomas. In low-grade astrocytomas, defined as IDH-mutated, non-1p/19q codeleted tumors, the T2-FLAIR mismatch sign, referring to a T2-hyperintense lesion that is hypointense on FLAIR with the exception of a hyperintense peripheral rim (131, 171, 173, 174), is quite characteristic and has an estimated sensitivity of 32% and specificity of 100% (175). In addition, IDH-mutated astrocytomas are associated with high ADC values, rostral extension to the lateral ventricle, and sharp borders (176), while IDH-mutant grade 4 astrocytomas have areas of incomplete enhancement (126) (Figure 3). MRS can detect the abnormal accumulation of the oncometabolite 2-HG within the tumor but false-negatives may frequently occur (177, 178). IDH mutations have possibly been the most studied by radiogenomics, including in multicenter studies. Two recent meta-analyses reported high sensitivity (85–88%) and specificity (87%) in the detection of IDH mutations with machine learning (15, 179).
Figure 3. Grade 4 IDH-mutant astrocytoma. (A) axial FLAIR, (B) axial T2-weighted image, (C) axial T1-weighted image with contrast, and (D) ADC map in the superior aspect of the lesion, showing a large infiltrative and expansive insular lesion. Note the partial T2-FLAIR mismatch sign at its lateral margin (circle) and extensive NCE component with no or only subtle enhancement with high ADC values. These findings indicate IDH-mutant astrocytoma. In contrast, the inferior aspect of the lesion (E–H) has a more heterogeneous T2 signal with hypointense areas corresponding to low ADC values and elevated rCBV in perfusion map (I) and intense poorly delimited enhancement with small necrotic areas. These findings indicate a high-grade tumor.
ATRX mutations have been detected in ctDNA from CSF with 75% sensitivity and 100% specificity (53). NGS can also identify ATRX mutations (67, 75, 76, 79, 80, 82, 83).
Conventional radiology does not identify specific characteristics of ATRX-mutated tumors except for the association of ATRX mutations with IDH mutations in astrocytoma. However, machine learning identified ATRX mutations in low-grade gliomas with an AUC of 0.89 (144) and deep learning identified them with 94% sensitivity and 92% specificity (141).
TP53 mutations were detected by PCR in ctDNA from CSF with 57% sensitivity and 100% specificity (53). They are also detectable by NGS (67, 75, 76, 79, 80, 82, 83).
Conventional radiology does not identify specific features of TP53 mutations other than their potential association with astrocytoma. Machine learning detected the mutations with an AUC of 0.89–0.95 and accuracy of 0.92 in low-grade gliomas (144, 146).
The WHO 2021 classification defines oligodendrogliomas as oligodendroglioma, IDH-mutant and 1p/19q codeleted (5), since both alterations are needed for diagnosis. One study of ctDNA in blood detected the deletion with 55% sensitivity and 100% specificity with microsatellite analysis (58). NGS of ctDNA in blood or CSF also detected the codeletion (67, 75, 76, 79, 80, 82, 83).
By conventional imaging, classically diagnosed oligodendrogliomas show calcifications, cortex predilection, heterogeneous, irregular contours with indistinct margins in T1 and T2 sequences, lower ADC values, and mildly elevated rCBV (126, 180). Textural analysis of the T2 signal predicted the 1p/19q codeletion with 93% sensitivity and 96% specificity (181–183). Most of these characteristics were already described when the diagnosis of oligodendroglioma was determined morphologically without the addition of molecular alterations as is now mandatory. More recently, machine learning identified the codeletion, especially in low-grade tumors, with an accuracy of around 0.81 and AUC of 0.88–0.89 (147–149).
Other mutated genes, such as CIC, have been found with high probability in oligodendrogliomas. (2, 161).
EGFR alterations have been linked to glioblastoma, and tumors previously defined as diffuse grade 2–3 astrocytoma, IDH wildtype are currently considered glioblastomas if they harbor EGFR amplification (5). EGFR amplification is commonly studied by fluorescence in situ hybridization and has not been studied in ctDNA but is detectable by NGS (67, 76, 79, 80, 83). Most cases with EGFR amplification harbor the EGFRvIII mutation, which has been detected in ctDNA, exosomes, microvesicles and TEPs in blood (59, 61–63) and in extracellular vesicles in CSF (60) with 61–81% sensitivity in blood and CSF and 89% specificity in CSF. The EGFRvIII mutation is also detectable by NGS.
EGFR alterations have been linked to characteristics of glioblastoma that can be identified by conventional imaging: contrast enhancement, ring appearance, satellite lesions, intratumoral hemorrhage, necrosis, ill-defined infiltration, abundant edema and areas of hypo- or non-enhancing tumor infiltration involving the cortex or deep nuclei (125). However, these characteristics are not commonly found in glioblastoma-like tumors.
Machine learning identified EGFR alterations, including low- and high-grade gliomas not diagnosed with the current guidelines, with an AUC of 0.77–0.90 and accuracy of 0.66–0.82 (142, 150, 151).
TERT promoter mutations are commonly found in glioblastoma and in oligodendroglioma. They were detected in ctDNA in both blood (64–66) and CSF (53, 57, 65), with 62% sensitivity in blood, slightly higher sensitivity in CSF, and 100% specificity in CSF. They have also been detected by NGS in ctDNA in both blood (80) and CSF (76).
Conventional radiology does not identify specific features related to TERT mutations other than those related to oligodendrogliomas or glioblastomas. A study with MRS found that TERT-mutated tumors could have more necrosis, probably related to the high-grade profile that TERT mutations confer to low-grade gliomas (154).
The +7/−10 signature has been proposed as a factor in the change from a low-grade IDH-wild-type tumor to a glioblastoma. LOH in chromosome 10q was identified by PCR-based microsatellite analysis of ctDNA in blood with a sensitivity of 35–58% and specificity of 80–94%. No relationship with tumor aggressivity was reported (58).
Conventional radiology does not identify specific features related to the +7/−10 signature.
The BRAFV600E mutation has been detected in the CSF of patients with brain metastases from melanoma (67). Relatively few gliomas harbor this mutation, making it difficult to show sensitivity and specificity for its detection in primary brain tumors. However, it has been detected by NGS in ctDNA from blood (75, 76) and CSF (79).
Conventional radiology does not identify specific features related to the BRAFV600E mutation.
Tumors with histone mutations in midline locations (thalamus, pons and spinal cord), which are preferably found in children and young adults, may harbor the H3K27M mutation (39). These locations are difficult to biopsy due to the possibility of surgical complications and definite deficits, indicating an urgent need for non-aggressive methods of diagnosis. Several studies of these tumors have analyzed ctDNA in CSF and detected the H3K27 mutation with about 80% sensitivity and 100% specificity (53, 57, 68).
Tumors with this mutation have greater enhancement, with a thick margin, and heterogeneity with cyst in T2 sequences, while midline tumors without this mutation have poor definition of the NCET margin, large edema and cortical invasion (184). Conversely tumors with histone mutation H3.3 G34R/V-mutant are usually hemispheric and are indistinguishable by imaging from other gliomas.
The CDKN2A/B deletion has been detected by NGS in both serum and blood (Table 2). The presence of this deletion worsens the prognosis of an IDH- mutant tumor so these tumors have to be considered as grade 4 according to the new WHO classification (2). The CDKN2A/B deletion can also be detected by deep learning radiogenomics (Table 3).
Due to the limited benefit that patients with tumors without MGMT promoter methylation derive from temozolomide, especially as first-line treatment for glioblastoma, the possibility of detecting MGMT promoter methylation in liquid biopsy has been extensively studied with the aim of avoiding aggressive treatments in patients who will likely not benefit, especially in biopsy-only, low performance status or elderly patients. MGMT status can be assessed in the clinical setting by methylation-specific PCR (MS-PCR), pyrosequencing, or multiplex-ligation dependent probe amplification (185). These methods can have different sensitivities and specificities. MGMT promoter methylation has been analyzed in ctDNA with MS-PCR with 36–79% sensitivity and 38–100% specificity, with somewhat better results in CSF than in blood (58, 69–72). MGMT promoter methylation can also be detected by genome wide methylation profiling in ctDNA or in DNA extracted from extracellular vesicles (83–85).
Several characteristics identified by conventional radiology have been linked to MGMT promoter methylation status but findings are not very specific (186). Ahn et al., found that the volume transfer constant (Ktrans) was significantly higher in the MGMT methylated group of patients, which could be attributed to better penetration of the drugs and better response to treatment (187). Higher ADC values have also been associated with methylation (188).
Recent radiomic studies predicted MGMT methylation status with up to 85% of accuracy (155–158).
GFAP is quite characteristic of gliomas and has been detected in blood and used to differentiate primary tumors from metastases (73) or and to differentiate glioma subtypes (74).
In addition to molecular alterations, tumors exhibit epigenetic modifications, such as DNA methylation in CpG islands, that regulate cell states. Methylation patterns in tumor tissue are different for different tumors and can therefore be used to identify the cell of origin in other cancers and to suggest a specific glioma subtype (88). It is also possible to extract information on DNA methylation from ctDNA. In fact, the amount of DNA needed to detect a methylome in plasma is less than that needed to detect individual mutations (85, 124). A cell-free methylated DNA immunoprecipitation assay clearly distinguished gliomas from other cancers with a mean AUC of 0.99 and also distinguished different glioma subtypes with an AUC near or over 0.80 (85). Glioma-epigenetic-liquid biopsy scoring was able to classify gliomas with 100% sensitivity and 97.78% specificity in ctDNA from plasma (50 ng) (84). In a third study, methylation profiling was performed on DNA extracted from extracellular vesicles in glioblastoma; CNVs and tumor-specific mutations were also examined in the same study (83).
At present, a CNS tumor can only be reliably diagnosed with a sample of tumor tissue, obtained either by resection or by at least a biopsy of the tumor. A suspicious radiological image needs to be classified as a cancerous or non-cancerous pathology and as a primary or secondary tumor. The diversity of histological subtypes among the primary tumors and the resulting differences in prognosis and treatment mean that correct treatment cannot be initiated without the tumor tissue. While it is clear that surgery also exerts a debulking effect and longer survival is directly proportional to surgical radicality, the extent of surgery is often limited by the clinical status or age of the patient and by the possibility of producing irreversible severe neurological lesions with an impact on quality of life in the case of central or deep tumors or those located in eloquent areas (3). As a result, it is often possible to perform only a biopsy of the tumor, without removal of the tumor mass. Surgical risk also limits the possibility of performing follow-up biopsies if there is radiological doubt as to progression, pseudoprogression, recurrence, or radionecrosis and also decreases the ability to obtain new tumor tissue at recurrence for information about molecular changes, such as resistant mutations, as is frequently done in other tumors (189). In contrast, a liquid biopsy of blood or CSF can facilitate the study of molecular alterations present in the tumor and could potentially be a non-aggressive method both for diagnosis prior to any therapeutic approach and for subsequent monitoring and follow-up of patients (6, 7, 9, 190).
Over the last few years, the number of studies on the detection of molecular alterations in blood and CSF from glioma patients has accelerated, with findings showing very high sensitivities and specificities compared to results obtained in tumor tissue. For example, IDH, ATRX, TP53, EGFR, TERT, BRAF, and H3F3A mutations, as well as MGMT promoter methylation, homozygous deletion of CDKN2A/B, and even GFAP have been detected by liquid biopsy. Studies have been performed both in blood and CSF, with ctRNA and ctDNA, and by isolating RNA and DNA from extracellular vesicles like exosomes. In contrast, TEPs have been less widely studied in gliomas (114). A variety of methods were used in these liquid biopsy studies, including PCR, MS-PCR, pyrosequencing, immunofluorescence, IHC, enzyme-linked immuno-sorbent assay, and ELISA. Since ctDNA is highly fractionated and the sequence under study can be lost (122, 123), the greatest handicap associated with liquid biopsies is the difficulty of detecting a sufficient amount of DNA or RNA to be able to proceed to the identification or isolation of the circulating nucleic acid and then proceed with the usual method for the detection of the alterations. CSF seems to provide better results than blood, since the amounts of DNA and RNA are higher due to the proximity of the tumor and the shedding of DNA in the CSF by contiguity (53, 76). However, a simple blood test is less invasive and could be used regularly in follow-up.
Exosomes can cross the BBB, thus facilitating the transmission of molecular information from the tumor to the blood (121) and informative results have been obtained with NGS of ctDNA and ctRNA isolated from circulating exosomes. In addition, plasma methylomes obtained with genome wide methylation profiling are able to distinguish primary from secondary tumors and then distinguish among the different subtypes of primary tumors based on their singular and specific methylation patterns (83). Only small amounts of ctDNA are necessary for genome wide methylation profiling (85, 124), and molecular information has been obtained with other NGS platforms in spite of the difficulty of isolating circulating nucleic acids (75, 80). These data indicate that technological drawbacks can be overcome in a relatively short time and findings validated in prospective studies.
Radiological diagnostics has also advanced technologically in recent years. The radiological characteristics that grossly identified the histological subtypes of gliomas were described before the molecular features were deemed essential for the diagnosis of the subtype; therefore, the radiological image/subtype relationship may not fully fit. For example, prior to the WHO 2016 classification (16), there was a subtype called oligoastrocytoma. The diagnosis of oligodendroglioma was based on the percentage of oligodendroglial cells present in the tumor. Would the presence of gross calcifications be typical of a 1p/19q oligodendroglioma, mutated IDH, as currently defined (5), or could it be that they also occur in tumors that don't presently fit a pure oligodendroglioma (180)? With the current possibilities afforded by image digitization and storage, the application of radiomics, radiogenomics, and machine learning, supported by computational methods and deep learning tools, a wealth of data about the image that had gone unperceived by the human eye is now available. Machine learning and deep learning are currently oriented toward imaging patterns and features that are characteristic of specific molecular alterations (129). Combining MRI with machine learning is rapidly gaining attention as a promising method for the molecular diagnosis of gliomas, with methods based on algorithms that can be incorporated in the MRI machines so that the diagnosis can be automated. In fact, there has recently been an increasing automation of MRI image post-processing programs that could standardize certain procedures and eliminate subjectivity: instant volumetric estimation of the lesion can be acquired without the need for manual segmentation; multiple volumes in perfusion maps can be calculated automatically; and the elimination of artifacts can be optimized. The qualitative and quantitative results provided by these programs could give us greater confidence, increase productivity, and create a workflow similar to that of the neuroimaging tool already used to improve decision making in the care of acute stroke victims. Automated and transparent circuits could be created for instant delivery of reports by email and automatic data flow to hospital picture archiving and communication systems (PACS), integrating all the information obtained from radiogenomics.
Liquid biopsy and radiogenomics share the same final objective: to obtain an accurate diagnosis of a CNS tumor with a non-invasive method showing high sensitivity and specificity that can be validated and then applied in the clinical setting. Such a method could be applied before initiating any aggressive therapy, including surgery, and could be used to better guide subsequent treatment. Furthermore, the same non-invasive methods could be used in a variety of situations for disease monitoring: a differential diagnosis between pseudoprogression, recurrence, and radionecrosis; the evolution of molecular alterations over the course of disease; and the appearance of new alterations, whether or not they are related to treatment (130, 190). Importantly, these methods could be used identify candidates for targeted therapies and lead to an increase in phase 0, or window-of-opportunity, studies since treatment could be initiated before surgery and there would be no need for two surgeries—one for diagnosis and another to check trial endpoints, such as drug-specific target effects, pharmacokinetics, and therapeutic concentrations in the tumor (132).
We can predict that diagnostic liquid biopsies and radiogenomics will likely be used initially for additional diagnostic information but will probably reach a point in the future where they will be incorporated in routine clinical practice. Nevertheless, the full clinical implementation of these methods is still in the distant future, pending the resolution of several issues. Both methods need studies shielded from tissue genomic results, with first assays in an initial training set, subsequent cross-validation, and then independent external testing in multicenter studies. Subsequently, both methods will need a clear objective with clinical relevance and applicability before being fully consolidated in clinical practice. They will need a generalization of algorithms, data collection protocols that include patient consent and correct labeling, and legal regulations—though errors in computational methods may be difficult to identify, especially in radiogenomics.
In addition, each of the methods faces its own specific difficulties. Some of the issues hampering the generalization and validation of liquid biopsy for clinical use are the selection of the best source (blood or CSF), the optimization of the amount of DNA or RNA required for analysis, the standardization of protocols for sample collection, the study of individual molecular alterations essential for diagnosis or for selecting patients for targeted therapy, and the certainty of methylation profiling as a tool for better diagnosis and subtyping. Several questions in radiogenomics also need to be clarified. Should MRI modalities and features be tailored to individual molecular markers or to glioma subtypes? How can subjectivity be eliminated from procedures like manual segmentation or qualitative evaluations in deep learning? How can stability and reproducibility of features be guaranteed under different conditions? In addition, different imaging acquisition methods and processing methods need to be standardized, the variability and heterogeneity of machine and deep learning need to be reduced, algorithms need to be constructed to automatically read results, and the biological basis for the relationship between the molecular and the radiological alterations needs to be explained.
In the coming years the scientific community will have a lot of work to do to validate the sources of information from which the data are obtained, the methodology, the sensitivity, specificity, accuracy and reproducibility. Currently, due to the profusion of published data, we run the risk of adopting a technique that does not meet the confidence to be adopted in clinical practice where it has to prove its validity to achieve a non-invasive diagnosis of CNS lesions.
In summary, however, according to the data currently available, liquid biopsy and radiogenomics have the potential to achieve their ultimate goal—the non-invasive diagnosis of CNS tumors and identification of their molecular alterations. One of the objectives of our article was to put on the table the need to address this issue in the scientific discussion forums that mark the future guidelines for action. This review has focused only on gliomas but in fact, the data from the studies included here could be extended to other CNS tumors or imaging tools. If we are to reach these objectives, we need to define parameters that will expedite relevant studies of these methods so that the findings can be validated in multicenter studies and applied to clinical practice.
JP and SC reviewed and drafted the sections on imaging. CC reviewed and drafted the sections on pathology and glioma diagnosis. TM reviewed and drafted the sections on liquid biopsy. AL-P, MD, AH, and CB reviewed and drafted the sections on clinical applications. CB designed the review and drafted the initial manuscript. All authors reviewed and approved the final version of the manuscript.
CB received funding from ISCIII/AES (INT19/00032) and a grant from the Spanish Society of Medical Oncology (Beca SEOM de Intensificación 2021). CC and CB received funding from ISCIII/AES (PI21/00816). AH received a grant from the Spanish Society of Medical Oncology (Beca SEOM). MD received a grant from the ISCIII/AES (CM19/00245).
The authors declare that the research was conducted in the absence of any commercial or financial relationships that could be construed as a potential conflict of interest.
All claims expressed in this article are solely those of the authors and do not necessarily represent those of their affiliated organizations, or those of the publisher, the editors and the reviewers. Any product that may be evaluated in this article, or claim that may be made by its manufacturer, is not guaranteed or endorsed by the publisher.
1. Ostrom QT, Patil N, Cioffi G, Waite K, Kruchko C, Barnholtz-Sloan JS. CBTRUS statistical report: primary brain and other central nervous system tumors diagnosed in the United States in 2013–2017. Neuro Oncol. (2020) 22:iv1–96. doi: 10.1093/neuonc/noaa200
2. WHO Classification of Tumours Editorial Board. Central Nervous System Tumours. WHO Classification of Tumours Series, 5th ed. Vol. 6. Lyon: International Agency for Research on Cancer (2021). Available online at: https://publications.iarc.fr/601
3. Weller M, van den Bent M, Preusser M, Le Rhun E, Tonn JC, Minniti G, et al. EANO guidelines on the diagnosis and treatment of diffuse gliomas of adulthood. Nat Rev Clin Oncol. (2021) 18:170–86. doi: 10.1038/s41571-020-00447-z
4. van den Bent MJ. Interobserver variation of the histopathological diagnosis in clinical trials on glioma: a clinician's perspective. Acta Neuropathol. (2010) 120:297–304. doi: 10.1007/s00401-010-0725-7
5. Louis DN, Perry A, Wesseling P, Brat DJ, Cree IA, Figarella-Branger D, et al. The 2021 WHO classification of tumors of the central nervous system: a summary. Neuro Oncol. (2021) 23:1231–51. doi: 10.1093/neuonc/noab106
6. Jones J, Nguyen H, Drummond K, Morokoff A. Circulating biomarkers for glioma: a review. Neurosurgery. (2021) 88:E221–30. doi: 10.1093/neuros/nyaa540
7. Müller Bark J, Kulasinghe A, Chua B, Day BW, Punyadeera C. Circulating biomarkers in patients with glioblastoma. Br J Cancer. (2020) 122:295–305. doi: 10.1038/s41416-019-0603-6
8. Le Rhun E, Seoane J, Salzet M, Soffietti R, Weller M. Liquid biopsies for diagnosing and monitoring primary tumors of the central nervous system. Cancer Lett. (2020) 480:24–8. doi: 10.1016/j.canlet.2020.03.021
9. Sareen H, Garrett C, Lynch D, Powter B, Brungs D, Cooper A, et al. The role of liquid biopsies in detecting molecular tumor biomarkers in brain cancer patients. Cancers. (2020) 12:1831. doi: 10.3390/cancers12071831
10. Guzman-De-Villoria JA, Mateos-Perez JM, Fernandez-Garcia P, Castro E, Desco M. Added value of advanced over conventional magnetic resonance imaging in grading gliomas and other primary brain tumors. Cancer Imaging. (2014) 14:35. doi: 10.1186/s40644-014-0035-8
11. Jain R, Chi AS. Radiogenomics identifying important biological pathways in gliomas. Neuro Oncol. (2021) 23:177–8. doi: 10.1093/neuonc/noaa290
12. Sanvito F, Castellano A, Falini A. Advancements in neuroimaging to unravel biological and molecular features of brain tumors. Cancers. (2021) 13:424. doi: 10.3390/cancers13030424
13. Seow P, Wong JHD, Ahmad-Annuar A, Mahajan A, Abdullah NA, Ramli N. Quantitative magnetic resonance imaging and radiogenomic biomarkers for glioma characterisation: a systematic review. Br J Radiol. (2018) 91:20170930. doi: 10.1259/bjr.20170930
14. Gore S, Chougule T, Jagtap J, Saini J, Ingalhalikar M. A review of radiomics and deep predictive modeling in glioma characterization. Acad Radiol. (2020) 28:1599–621. doi: 10.1016/j.acra.2020.06.016
15. Jian A, Jang K, Manuguerra M, Liu S, Magnussen J, Di Ieva A. Machine learning for the prediction of molecular markers in glioma on magnetic resonance imaging: a systematic review and meta-analysis. Neurosurgery. (2021) 89:31–44. doi: 10.1093/neuros/nyab103
16. Louis DN, Perry A, Reifenberger G, von Deimling A, Figarella-Branger D, Cavenee WK, et al. The 2016 World Health Organization classification of tumors of the central nervous system: a summary. Acta Neuropathol. (2016) 131:803–20. doi: 10.1007/s00401-016-1545-1
17. Louis DN, Ohgaki H, Wiestler OD, Cavenee WK. WHO Classification of Tumours of the Central Nervous System. International Agency for Research on Cancer, Lyon (2016).
18. Gonzalez Castro LN, Wesseling P. The cIMPACT-NOW updates and their significance to current neuro-oncology practice. Neuro Oncol Pract. (2021) 8:4–10. doi: 10.1093/nop/npaa055
19. Wen PY, Packer RJ. The 2021 WHO Classification of Tumors of the Central Nervous System: clinical implications. Neuro Oncol. (2021) 23:1215–17. doi: 10.1093/neuonc/noab120
20. Balss J, Meyer J, Mueller W, Korshunov A, Hartmann C, von Deimling A. Analysis of the IDH1 codon 132 mutation in brain tumors. Acta Neuropathol. (2008) 116:597–602. doi: 10.1007/s00401-008-0455-2
21. Yan H, Parsons DW, Jin G, McLendon R, Rasheed BA, Yuan W, et al. IDH1 and IDH2 mutations in gliomas. N Engl J Med. (2009) 360:765–73. doi: 10.1056/NEJMoa0808710
22. Zhao S, Lin Y, Xu W, Jiang W, Zha Z, Wang P, et al. Glioma-derived mutations in IDH1 dominantly inhibit IDH1 catalytic activity and induce HIF-1alpha. Science. (2009) 324:261–5. doi: 10.1126/science.1170944
23. Ducray F, Marie Y, Sanson M. IDH1 and IDH2 mutations in gliomas. N Engl J Med. (2009) 360:2248–9; author reply 2249. doi: 10.1056/NEJMc090593
24. V. Di Nunno, Franceschi E, Tosoni A, Gatto L, Maggio I, Lodi R, et al. Clinical and molecular features of patients with gliomas harboring IDH1 non-canonical mutations: a systematic review and meta-analysis. Adv Ther. (2022) 39:165–77. doi: 10.1007/s12325-021-01977-3
25. Franceschi E, De Biase D, Di Nunno V, Pession A, Tosoni A, Gatto L Tallini G, et al. IDH1 Non-canonical mutations and survival in patients with glioma. Diagnostics. (2021) 11:342. doi: 10.3390/diagnostics11020342
26. Liu XY, Gerges N, Korshunov A, Sabha N, Khuong-Quang DA, Fontebasso AM, et al. Frequent ATRX mutations and loss of expression in adult diffuse astrocytic tumors carrying IDH1/IDH2 and TP53 mutations. Acta Neuropathol. (2012) 124:615–25. doi: 10.1007/s00401-012-1031-3
27. Ikemura M, Shibahara J, Mukasa A, Takayanagi S, Aihara K, Saito N, et al. Utility of ATRX immunohistochemistry in diagnosis of adult diffuse gliomas. Histopathology. (2016) 69:260–7. doi: 10.1111/his.12927
28. Brennan CW, Verhaak RG, McKenna A, Campos B, Noushmehr H, Salama SR, et al. The somatic genomic landscape of glioblastoma. Cell. (2013) 155:462–77. doi: 10.1016/j.cell.2013.09.034
29. Ino Y, Betensky RA, Zlatescu MC, Sasaki H, Macdonald DR, Stemmer-Rachamimov AO, et al. Molecular subtypes of anaplastic oligodendroglioma: implications for patient management at diagnosis. Clin Cancer Res. (2001) 7:839–45.
30. Reifenberger J, Reifenberger G, Liu L, James CD, Wechsler W, Collins VP. Molecular genetic analysis of oligodendroglial tumors shows preferential allelic deletions on 19q and 1p. Am J Pathol. (1994) 145:1175–90.
31. Ekstrand AJ, James CD, Cavenee WK, Seliger B, Pettersson RF, Collins VP. Genes for epidermal growth factor receptor, transforming growth factor alpha, and epidermal growth factor and their expression in human gliomas in vivo. Cancer Res. (1991) 51:2164–72.
32. Ekstrand AJ, Longo N, Hamid ML, Olson JJ, Liu L, Collins VP, et al. Functional characterization of an EGF receptor with a truncated extracellular domain expressed in glioblastomas with EGFR gene amplification. Oncogene. (1994) 9:2313–20.
33. Arita H, Narita Y, Fukushima S, Tateishi K, Matsushita Y, Yoshida A, et al. Upregulating mutations in the TERT promoter commonly occur in adult malignant gliomas and are strongly associated with total 1p19q loss. Acta Neuropathol. (2013) 126:267–76. doi: 10.1007/s00401-013-1141-6
34. Berzero G, Di Stefano AL, Ronchi S, Bielle F, Villa C, Guillerm E, et al. IDH-wildtype lower-grade diffuse gliomas: the importance of histological grade and molecular assessment for prognostic stratification. Neuro Oncol. (2021) 23:955–66. doi: 10.1093/neuonc/noaa258
35. Bigner SH, Mark J, Burger PC, Mahaley MS Jr., Bullard DE, et al.Specific chromosomal abnormalities in malignant human gliomas. Cancer Res. (1988) 48:405–11.
36. Korshunov A, Meyer J, Capper D, Christians A, Remke M, Witt H, et al. Combined molecular analysis of BRAF and IDH1 distinguishes pilocytic astrocytoma from diffuse astrocytoma. Acta Neuropathol. (2009) 118:401–5. doi: 10.1007/s00401-009-0550-z
37. Korshunov A, Chavez L, Sharma T, Ryzhova M, Schrimpf D, Stichel D, et al. Epithelioid glioblastomas stratify into established diagnostic subsets upon integrated molecular analysis. Brain Pathol. (2018) 28:656–62. doi: 10.1111/bpa.12566
38. Wen PY, Stein A, van den Bent M, De Greve J, Wick A, de Vos F, et al. Dabrafenib plus trametinib in patients with BRAF(V600E)-mutant low-grade and high-grade glioma (ROAR): a multicentre, open-label, single-arm, phase 2, basket trial. Lancet Oncol. (2022) 23:53–64. doi: 10.1016/S1470-2045(21)00578-7
39. Sturm D, Witt H, Hovestadt V, Khuong-Quang DA, Jones DT, Konermann C, et al. Hotspot mutations in H3F3A and IDH1 define distinct epigenetic and biological subgroups of glioblastoma. Cancer Cell. (2012) 22:425–37. doi: 10.1016/j.ccr.2012.08.024
40. Shirahata M, Ono T, Stichel D, Schrimpf D, Reuss DE, Sahm F, et al. Novel, improved grading system(s) for IDH-mutant astrocytic gliomas. Acta Neuropathol. (2018) 136:153–66. doi: 10.1007/s00401-018-1849-4
41. Brat DJ, Aldape K, Colman H, Figrarella-Branger D, Fuller GN, Giannini C, et al. cIMPACT-NOW update 5: recommended grading criteria and terminologies for IDH-mutant astrocytomas. Acta Neuropathol. (2020) 139:603–8. doi: 10.1007/s00401-020-02127-9
42. Esteller M, Garcia-Foncillas J, Andion E, Goodman SN, Hidalgo OF, Vanaclocha V, et al. Inactivation of the DNA-repair gene MGMT and the clinical response of gliomas to alkylating agents. N Engl J Med. (2000) 343:1350–4. doi: 10.1056/NEJM200011093431901
43. Mandel P, Metais P. Nuclear acids in human blood plasma. C R Seances Soc Biol Fil. (1948) 142:241–3.
44. Aucamp J, Bronkhorst AJ, Badenhorst CPS, Pretorius PJ. The diverse origins of circulating cell-free DNA in the human body: a critical re-evaluation of the literature. Biol Rev Camb Philos Soc. (2018) 93:1649–83. doi: 10.1111/brv.12413
45. Leon SA, Shapiro B, Sklaroff DM, Yaros MJ. Free DNA in the serum of cancer patients and the effect of therapy. Cancer Res. (1977) 37:646–50.
46. Pantel K, Alix-Panabieres C. Circulating tumour cells in cancer patients: challenges and perspectives. Trends Mol Med. (2010) 16:398–406. doi: 10.1016/j.molmed.2010.07.001
47. Ignatiadis M, Sledge GW, Jeffrey SS. Liquid biopsy enters the clinic — implementation issues and future challenges. Nat Rev Clin Oncol. (2021) 18:297–312. doi: 10.1038/s41571-020-00457-x
48. Balana C, Ramirez JL, Taron M, Roussos Y, Ariza A, Ballester R, et al. O6-methyl-guanine-DNA methyltransferase methylation in serum and tumor DNA predicts response to 1,3-bis(2-chloroethyl)-1-nitrosourea but not to temozolamide plus cisplatin in glioblastoma multiforme. Clin Cancer Res. (2003) 9:1461–8.
49. De Mattos-Arruda L, Mayor R, Ng CK, Weigelt B, Martinez-Ricarte F, Torrejon D, et al. Cerebrospinal fluid-derived circulating tumour DNA better represents the genomic alterations of brain tumours than plasma. Nat Commun. (2015) 6:8839. doi: 10.1038/ncomms9839
50. Wang Y, Springer S, Zhang M, McMahon KW, Kinde I, Dobbyn L, et al. Papadopoulos N, Kinzler KW, Vogelstein B, Bettegowda C,. Detection of tumor-derived DNA in cerebrospinal fluid of patients with primary tumors of the brain and spinal cord. Proc Natl Acad Sci USA. (2015) 112:9704–9. doi: 10.1073/pnas.1511694112
51. Gatto L, Franceschi E, Di Nunno V, Tosoni A, Lodi R, Brandes AA. Liquid biopsy in glioblastoma management: from current research to future perspectives. Oncologist. (2021) 26:865–78. doi: 10.1002/onco.13858
52. Boisselier B, Gallego Perez-Larraya J, Rossetto M, Labussiere M, Ciccarino P, Marie Y, et al. Detection of IDH1 mutation in the plasma of patients with glioma. Neurology. (2012) 79:1693–8. doi: 10.1212/WNL.0b013e31826e9b0a
53. Martinez-Ricarte F, Mayor R, Martinez-Saez E, Rubio-Perez C, Pineda E, Cordero E, et al. Molecular diagnosis of diffuse gliomas through sequencing of cell-free circulating tumor DNA from cerebrospinal fluid. Clin Cancer Res. (2018) 24:2812–19. doi: 10.1158/1078-0432.CCR-17-3800
54. Lombardi G, Corona G, Bellu L, Della Puppa A, Pambuku A, Fiduccia P, et al. Diagnostic value of plasma and urinary 2-hydroxyglutarate to identify patients with isocitrate dehydrogenase-mutated glioma. Oncologist. (2015) 20:562–7. doi: 10.1634/theoncologist.2014-0266
55. Kalinina J, Ahn J, Devi NS, Wang L, Li Y, Olson JJ, et al. Selective detection of the D-enantiomer of 2-hydroxyglutarate in the CSF of glioma patients with mutated isocitrate dehydrogenase. Clin Cancer Res. (2016) 22:6256–65. doi: 10.1158/1078-0432.CCR-15-2965
56. Garcia-Romero N, Carrion-Navarro J, Esteban-Rubio S, Lazaro-Ibanez E, Peris-Celda M, Alonso MM, et al. DNA sequences within glioma-derived extracellular vesicles can cross the intact blood-brain barrier and be detected in peripheral blood of patients. Oncotarget. (2017) 8:1416–28. doi: 10.18632/oncotarget.13635
57. Fujioka Y, Hata N, Akagi Y, Kuga D, Hatae R, Sangatsuda Y, et al. Molecular diagnosis of diffuse glioma using a chip-based digital PCR system to analyze IDH, TERT, and H3 mutations in the cerebrospinal fluid. J Neurooncol. (2021) 152:47–54. doi: 10.1007/s11060-020-03682-7
58. Lavon I, Refael M, Zelikovitch B, Shalom E, Siegal T. Serum DNA can define tumor-specific genetic and epigenetic markers in gliomas of various grades. Neuro Oncol. (2010) 12:173–80. doi: 10.1093/neuonc/nop041
59. Manda SV, Kataria Y, Tatireddy BR, Ramakrishnan B, Ratnam BG, Lath R, et al. Exosomes as a biomarker platform for detecting epidermal growth factor receptor-positive high-grade gliomas. J Neurosurg. (2018) 128:1091–101. doi: 10.3171/2016.11.JNS161187
60. Figueroa JM, Skog J, Akers J, Li H, Komotar R, Jensen R, et al. Detection of wild-type EGFR amplification and EGFRvIII mutation in CSF-derived extracellular vesicles of glioblastoma patients. Neuro Oncol. (2017) 19:1494–502. doi: 10.1093/neuonc/nox085
61. Salkeni MA, Zarzour A, Ansay TY, McPherson CM, Warnick RE, Rixe O, et al. Detection of EGFRvIII mutant DNA in the peripheral blood of brain tumor patients. J Neurooncol. (2013) 115:27–35. doi: 10.1007/s11060-013-1209-0
62. Skog J, Wurdinger T, van Rijn S, Meijer DH, Gainche L, Sena-Esteves M, et al. Glioblastoma microvesicles transport RNA and proteins that promote tumour growth and provide diagnostic biomarkers. Nat Cell Biol. (2008) 10:1470–6. doi: 10.1038/ncb1800
63. Nilsson RJ, Balaj L, Hulleman E, van Rijn S, Pegtel DM, Walraven M, et al. Blood platelets contain tumor-derived RNA biomarkers. Blood. (2011) 118:3680–3. doi: 10.1182/blood-2011-03-344408
64. Muralidharan K, Yekula A, Small JL, Rosh ZS, Kang KM, Wang L, et al. TERT promoter mutation analysis for blood-based diagnosis and monitoring of gliomas. Clin Cancer Res. (2021) 27:169–78. doi: 10.1158/1078-0432.CCR-20-3083
65. Juratli TA, Stasik S, Zolal A, Schuster C, Richter S, Daubner D, et al. TERT promoter mutation detection in cell-free tumor-derived DNA in patients with IDH wild-type glioblastomas: a pilot prospective study. Clin Cancer Res. (2018) 24:5282–91. doi: 10.1158/1078-0432.CCR-17-3717
66. Gandhi P, Khare R, Garg N. Evaluating the potential of circulating hTERT levels in glioma: can plasma levels serve as an independent prognostic marker? J Neurooncol. (2017) 135:255–61. doi: 10.1007/s11060-017-2578-6
67. Pentsova EI, Shah RH, Tang J, Boire A, You D, Briggs S, et al. Evaluating cancer of the central nervous system through next-generation sequencing of cerebrospinal fluid. J Clin Oncol. (2016) 34:2404–15. doi: 10.1200/JCO.2016.66.6487
68. Huang TY, Piunti A, Lulla RR, Qi J, Horbinski CM, Tomita T, et al. Detection of Histone H3 mutations in cerebrospinal fluid-derived tumor DNA from children with diffuse midline glioma. Acta Neuropathol Commun. (2017) 5:28. doi: 10.1186/s40478-017-0436-6
69. Estival A, Sanz C, Ramirez J-L, Maria Velarde J, Domenech M, Carrato C, et al. Pyrosequencing versus methylation-specific PCR for assessment of MGMT methylation in tumor and blood samples of glioblastoma patients. Sci Rep. (2019) 9:11125. doi: 10.1038/s41598-019-47642-2
70. Wang Z, Jiang W, Wang Y, Guo Y, Cong Z, Du F, et al. MGMT promoter methylation in serum and cerebrospinal fluid as a tumor-specific biomarker of glioma. Biomed Rep. (2015) 3:543–8. doi: 10.3892/br.2015.462
71. Fiano V, Trevisan M, Trevisan E, Senetta R, Castiglione A, Sacerdote C, et al. MGMT promoter methylation in plasma of glioma patients receiving temozolomide. J Neurooncol. (2014) 117:347–57. doi: 10.1007/s11060-014-1395-4
72. Gong M, Shi W, Qi J, Shao G, Shi Z, Wang J, et al. Alu hypomethylation and MGMT hypermethylation in serum as biomarkers of glioma. Oncotarget. (2017) 8:76797–806. doi: 10.18632/oncotarget.20012
73. Jung CS, Foerch C, Schanzer A, Heck A, Plate KH, Seifert V, et al. Serum GFAP is a diagnostic marker for glioblastoma multiforme. Brain. (2007) 130:3336–41. doi: 10.1093/brain/awm263
74. Kiviniemi A, Gardberg M, Frantzen J, Parkkola R, Vuorinen V, Pesola M, et al. Serum levels of GFAP and EGFR in primary and recurrent high-grade gliomas: correlation to tumor volume, molecular markers, progression-free survival. J Neurooncol. (2015) 124:237–45. doi: 10.1007/s11060-015-1829-7
75. Liang J, Zhao W, Lu C, Liu D, Li P, Ye X, et al. Next-generation sequencing analysis of ctDNA for the detection of glioma and metastatic brain tumors in adults. Front Neurol. (2020) 11:544. doi: 10.3389/fneur.2020.00544
76. Miller AM, Shah RH, Pentsova EI, Pourmaleki M, Briggs S, Distefano N, et al. Tracking tumour evolution in glioma through liquid biopsies of cerebrospinal fluid. Nature. (2019) 565:654–8. doi: 10.1038/s41586-019-0882-3
77. Zhao Y, He JY, Cui JZ, Meng ZQ, Zou YL, Guo XS, et al. Detection of genes mutations in cerebrospinal fluid circulating tumor DNA from neoplastic meningitis patients using next generation sequencing. BMC Cancer. (2020) 20:690. doi: 10.1186/s12885-020-07172-x
78. Wang L, Yekula A, Muralidharan K, Small JL, Rosh ZS, Kang KM, et al. Novel gene fusions in glioblastoma tumor tissue and matched patient plasma. Cancers. (2020) 12:1219. doi: 10.3390/cancers12051219
79. Zhao Z, Zhang C, Li M, Shen Y, Feng S, Liu J, et al. Applications of cerebrospinal fluid circulating tumor DNA in the diagnosis of gliomas. Jpn J Clin Oncol. (2020) 50:325–32. doi: 10.1093/jjco/hyz156
80. Piccioni DE, Achrol AS, Kiedrowski LA, Banks KC, Boucher N, Barkhoudarian G, et al. Analysis of cell-free circulating tumor DNA in 419 patients with glioblastoma and other primary brain tumors. CNS Oncol. (2019) 8:CNS34. doi: 10.2217/cns-2018-0015
81. Noerholm M, Balaj L, Limperg T, Salehi A, Zhu LD, Hochberg FH, et al. RNA expression patterns in serum microvesicles from patients with glioblastoma multiforme and controls. BMC Cancer. (2012) 12:22. doi: 10.1186/1471-2407-12-22
82. Pan C, Diplas BH, Chen X, Wu Y, Xiao X, Jiang L, et al. Molecular profiling of tumors of the brainstem by sequencing of CSF-derived circulating tumor DNA. Acta Neuropathol. (2019) 137:297–306. doi: 10.1007/s00401-018-1936-6
83. Maire CL, Fuh MM, Kaulich K, Fita KD, Stevic I, Heiland DH, et al. Genome-wide methylation profiling of glioblastoma cell-derived extracellular vesicle DNA allows tumor classification. Neuro Oncol. (2021) 23:1087–99. doi: 10.1093/neuonc/noab012
84. Sabedot TS, Malta TM, Snyder J, Nelson K, Wells M, deCarvalho AC, et al. A serum-based DNA methylation assay provides accurate detection of glioma. Neuro Oncol. (2021) 23:1494–508. doi: 10.1093/neuonc/noab023
85. Nassiri F, Chakravarthy A, Feng S, Shen SY, Nejad R, Zuccato JA, et al. Detection and discrimination of intracranial tumors using plasma cell-free DNA methylomes. Nat Med. (2020) 26:1044–7. doi: 10.1038/s41591-020-0932-2
86. Stroun M, Maurice P, Vasioukhin V, Lyautey J, Lederrey C, Lefort F, et al. The origin and mechanism of circulating DNA. Ann N Y Acad Sci. (2000) 906:161–8. doi: 10.1111/j.1749-6632.2000.tb06608.x
87. Diehl F, Schmidt K, Choti MA, Romans K, Goodman S, Li M Jr., et al. Circulating mutant DNA to assess tumor dynamics. Nat Med. (2008) 14:985–90. doi: 10.1038/nm.1789
88. Shen SY, Singhania R, Fehringer G, Chakravarthy A, Roehrl MHA, Chadwick D, et al.D. De Carvalho, Sensitive tumour detection and classification using plasma cell-free DNA methylomes. Nature. (2018) 563:579–83. doi: 10.1038/s41586-018-0703-0
89. Yates LA, Norbury CJ, Gilbert RJ. The long and short of microRNA. Cell. (2013) 153:516–9. doi: 10.1016/j.cell.2013.04.003
90. Sole C, Arnaiz E, Manterola L, Otaegui D, Lawrie CH. The circulating transcriptome as a source of cancer liquid biopsy biomarkers. Semin Cancer Biol. (2019) 58:100–108. doi: 10.1016/j.semcancer.2019.01.003
91. Mitchell PS, Parkin RK, Kroh EM, Fritz BR, Wyman SK, Pogosova-Agadjanyan EL, et al. Circulating microRNAs as stable blood-based markers for cancer detection. Proc Natl Acad Sci U S A. (2008) 105:10513–8. doi: 10.1073/pnas.0804549105
92. Dong L, Li Y, Han C, Wang X, She L, Zhang H. miRNA microarray reveals specific expression in the peripheral blood of glioblastoma patients. Int J Oncol. (2014) 45:746–56. doi: 10.3892/ijo.2014.2459
93. Akers JC, Hua W, Li H, Ramakrishnan V, Yang Z, Quan K, et al. A cerebrospinal fluid microRNA signature as biomarker for glioblastoma. Oncotarget. (2017) 8:68769–779. doi: 10.18632/oncotarget.18332
94. Lai NS, Wu DG, Fang XG, Lin YC, Chen SS, Li ZB, et al. Serum microRNA-210 as a potential noninvasive biomarker for the diagnosis and prognosis of glioma. Br J Cancer. (2015) 112:1241–6. doi: 10.1038/bjc.2015.91
95. Maggio I, Franceschi E, Gatto L, Tosoni A, Di Nunno V, Tonon C, et al. Radiomics, mirnomics, and radiomirRNomics in glioblastoma: defining tumor biology from shadow to light. Expert Rev Anticancer Ther. (2021) 21:1265–72. doi: 10.1080/14737140.2021.1971518
96. Shen J, Hodges TR, Song R, Gong Y, Calin GA, Heimberger AB, et al. Serum HOTAIR and GAS5 levels as predictors of survival in patients with glioblastoma. Mol Carcinog. (2018) 57:137–41. doi: 10.1002/mc.22739
97. Lianidou ES, Strati A, Markou A. Circulating tumor cells as promising novel biomarkers in solid cancers. Crit Rev Clin Lab Sci. (2014) 51:160–71. doi: 10.3109/10408363.2014.896316
98. Gao F, Cui Y, Jiang H, Sui D, Wang Y, Jiang Z, et al. Circulating tumor cell is a common property of brain glioma and promotes the monitoring system. Oncotarget. (2016) 7:71330–340. doi: 10.18632/oncotarget.11114
99. Rossi E, Fabbri F. CTCs 2020: great expectations or unreasonable dreams. Cells. (2019) 8:989. doi: 10.3390/cells8090989
100. Liu T, Xu H, Huang M, Ma W, Saxena D, Lustig RA, et al. Circulating glioma cells exhibit stem cell-like properties. Cancer Res. (2018) 78:6632–42. doi: 10.1158/0008-5472.CAN-18-0650
101. Sullivan JP, Nahed BV, Madden MW, Oliveira SM, Springer S, Bhere D, et al. Brain tumor cells in circulation are enriched for mesenchymal gene expression. Cancer Discov. (2014) 4:1299–309. doi: 10.1158/2159-8290.CD-14-0471
102. Aiello NM, Maddipati R, Norgard RJ, Balli D, Li J, Yuan S, et al. EMT subtype influences epithelial plasticity and mode of cell migration. Dev Cell. (2018) 45:681–95 e4. doi: 10.1016/j.devcel.2018.05.027
103. Masuda T, Hayashi N, Iguchi T, Ito S, Eguchi H, Mimori K. Clinical and biological significance of circulating tumor cells in cancer. Mol Oncol. (2016) 10:408–17. doi: 10.1016/j.molonc.2016.01.010
104. Bang-Christensen SR, Pedersen RS, Pereira MA, Clausen TM, Loppke C, Sand NT, et al. Capture and detection of circulating glioma cells using the recombinant VAR2CSA malaria protein. Cells. (2019) 8:998. doi: 10.3390/cells8090998
105. Krol I, Castro-Giner F, Maurer M, Gkountela S, Szczerba BM, Scherrer R, et al. Detection of circulating tumour cell clusters in human glioblastoma. Br J Cancer. (2018) 119:487–91. doi: 10.1038/s41416-018-0186-7
106. Macarthur KM, Kao GD, Chandrasekaran S, Alonso-Basanta M, Chapman C, Lustig RA, et al. Detection of brain tumor cells in the peripheral blood by a telomerase promoter-based assay. Cancer Res. (2014) 74:2152–9. doi: 10.1158/0008-5472.CAN-13-0813
107. Thery C, Witwer KW, Aikawa E, Alcaraz MJ, Anderson JD, Andriantsitohaina R, et al. Minimal information for studies of extracellular vesicles 2018 (MISEV2018): a position statement of the International Society for Extracellular Vesicles and update of the MISEV2014 guidelines. J Extracell Vesicles. (2018) 7:1535750. doi: 10.1080/20013078.2018.1535750
108. Redzic JS, Ung TH, Graner MW. Glioblastoma extracellular vesicles: reservoirs of potential biomarkers. Pharmgenomics Pers Med. (2014) 7:65–77. doi: 10.2147/PGPM.S39768
109. Martins VR, Dias MS, Hainaut P. Tumor-cell-derived microvesicles as carriers of molecular information in cancer. Curr Opin Oncol. (2013) 25:66–75. doi: 10.1097/CCO.0b013e32835b7c81
110. Manterola L, Guruceaga E, Gallego Perez-Larraya J, Gonzalez-Huarriz M, Jauregui P, Tejada S, et al. A small noncoding RNA signature found in exosomes of GBM patient serum as a diagnostic tool. Neuro Oncol. (2014) 16:520–7. doi: 10.1093/neuonc/not218
111. Best MG, Wesseling P, Wurdinger T. Tumor-educated platelets as a noninvasive biomarker source for cancer detection and progression monitoring. Cancer Res. (2018) 78:3407–412. doi: 10.1158/0008-5472.CAN-18-0887
112. Wurdinger T, In 't Veld S, Best MG. Platelet RNA as pan-tumor biomarker for cancer detection. Cancer Res. (2020) 80:1371–3. doi: 10.1158/0008-5472.CAN-19-3684
113. Joosse SA, Pantel K. Tumor-educated platelets as liquid biopsy in cancer patients. Cancer Cell. (2015) 28:552–4. doi: 10.1016/j.ccell.2015.10.007
114. Campanella R, Guarnaccia L, Cordiglieri C, Trombetta E, Caroli M, Carrabba G, et al. Tumor-educated platelets and angiogenesis in glioblastoma: another brick in the wall for novel prognostic and targetable biomarkers, changing the vision from a localized tumor to a systemic pathology. Cells. (2020) 9:294. doi: 10.3390/cells9020294
115. Lange RP, Everett A, Dulloor P, Korley FK, Bettegowda C, Blair C, et al. Evaluation of eight plasma proteins as candidate blood-based biomarkers for malignant gliomas. Cancer Invest. (2014) 32:423–9. doi: 10.3109/07357907.2014.933237
116. Spreafico F, Bongarzone I, Pizzamiglio S, Magni R, Taverna E, De Bortoli M, et al. Proteomic analysis of cerebrospinal fluid from children with central nervous system tumors identifies candidate proteins relating to tumor metastatic spread. Oncotarget. (2017) 8:46177–90. doi: 10.18632/oncotarget.17579
117. Hormigo A, Gu B, Karimi S, Riedel E, Panageas KS, Edgar MA, et al. YKL-40 and matrix metalloproteinase-9 as potential serum biomarkers for patients with high-grade gliomas. Clin Cancer Res. (2006) 12:5698–704. doi: 10.1158/1078-0432.CCR-06-0181
118. Locasale JW, Melman T, Song S, Yang X, Swanson KD, Cantley LC, et al. Metabolomics of human cerebrospinal fluid identifies signatures of malignant glioma. Mol Cell Proteomics. (2012) 11:M111 014688. doi: 10.1074/mcp.M111.014688
119. Bettegowda C, Sausen M, Leary RJ, Kinde I, Wang Y, Agrawal N Jr., et al. Detection of circulating tumor DNA in early- and late-stage human malignancies. Sci Transl Med. (2014) 6:224ra24. doi: 10.1158/1538-7445.AM2014-5606
120. Wang J, Bettegowda C. Applications of DNA-based liquid biopsy for central nervous system neoplasms. J Mol Diagn. (2017) 19:24–34. doi: 10.1016/j.jmoldx.2016.08.007
121. Morad G, Carman CV, Hagedorn EJ, Perlin JR, Zon LI, Mustafaoglu N, et al. Tumor-derived extracellular vesicles breach the intact blood-brain barrier via transcytosis. ACS Nano. (2019) 13:13853–65. doi: 10.1021/acsnano.9b04397
122. Underhill HR, Kitzman JO, Hellwig S, Welker NC, Daza R, Baker DN, et al. Fragment length of circulating tumor DNA. PLoS Genet. (2016) 12:e1006162. doi: 10.1371/journal.pgen.1006162
123. Mouliere F, Chandrananda D, Piskorz AM, Moore EK, Morris J, Ahlborn LB, et al. Enhanced detection of circulating tumor DNA by fragment size analysis. Sci Transl Med. (2018) 10:eaat4921. doi: 10.1126/scitranslmed.aat4921
124. Shen SY, Burgener JM, Bratman SV, De Carvalho DD. Preparation of cfMeDIP-seq libraries for methylome profiling of plasma cell-free DNA. Nat Protoc. (2019) 14:2749–80. doi: 10.1038/s41596-019-0202-2
125. Upadhyay N, Waldman AD. Conventional MRI evaluation of gliomas. Br J Radiol. (2011) 2:S107–11. doi: 10.1259/bjr/65711810
126. Lasocki A, Anjari M, Kokurcan SO, Thust SC. Conventional MRI features of adult diffuse glioma molecular subtypes: a systematic review. Neuroradiology. (2021) 63:353–62. doi: 10.1007/s00234-020-02532-7
127. Hwan-Ho C, Hyunjin P. Classification of low-grade and high-grade glioma using multi-modal image radiomics features. Annu Int Conf IEEE Eng Med Biol Soc. (2017) 2017:3081–84. doi: 10.1109/EMBC.2017.8037508
128. Gao M, Huang S, Pan X, Liao X, Yang R, Liu J. Machine learning-based radiomics predicting tumor grades and expression of multiple pathologic biomarkers in gliomas. Front Oncol. (2020) 10:1676. doi: 10.3389/fonc.2020.01676
129. Arita H, Kinoshita M, Kawaguchi A, Takahashi M, Narita Y, Terakawa Y, et al. Lesion location implemented magnetic resonance imaging radiomics for predicting IDH and TERT promoter mutations in grade II/III gliomas. Sci Rep. (2018) 8:11773. doi: 10.1038/s41598-018-30273-4
130. Kocakavuk E, Anderson KJ, Varn FS, Johnson KC, Amin SB, Sulman EP, et al. Radiotherapy is associated with a deletion signature that contributes to poor outcomes in patients with cancer. Nat Genet. (2021) 53:1088–96. doi: 10.1038/s41588-021-00874-3
131. Jain R, Johnson DR, Patel SH, Castillo M, Smits M, van den Bent MJ, et al. “Real world” use of a highly reliable imaging sign: “T2-FLAIR mismatch” for identification of IDH mutant astrocytomas. Neuro Oncol. (2020) 22:936–43. doi: 10.1093/neuonc/noaa041
132. Vogelbaum MA, Krivosheya D, Borghei-Razavi H, Sanai N, Weller M, Wick W, et al. Phase 0 and window of opportunity clinical trial design in neuro-oncology: a RANO review. Neuro Oncol. (2020) 22:1568–79. doi: 10.1093/neuonc/noaa149
133. Han Y, Wang W, Yang Y, Sun YZ, Xiao G, Tian Q, et al. Amide proton transfer imaging in predicting isocitrate dehydrogenase 1 mutation status of grade II/III gliomas based on support vector machine. Front Neurosci. (2020) 14:144. doi: 10.3389/fnins.2020.00144
134. Li Z, Wang Y, Yu J, Guo Y, Cao W. Deep Learning based Radiomics (DLR) and its usage in noninvasive IDH1 prediction for low grade glioma. Sci Rep. (2017) 7:5467. doi: 10.1038/s41598-017-05848-2
135. Niu L, Feng WH, Duan CF, Liu YC, Liu JH, Liu XJ. The value of enhanced MR radiomics in estimating the IDH1 genotype in high-grade gliomas. BioMed Res Int. (2020) 2020:4630218. doi: 10.1155/2020/4630218
136. Park CJ, Choi YS, Park YW, Ahn SS, Kang SG, Chang JH, et al. Diffusion tensor imaging radiomics in lower-grade glioma: improving subtyping of isocitrate dehydrogenase mutation status. Neuroradiology. (2020) 62:319–36. doi: 10.1007/s00234-019-02312-y
137. Peng H, Huo J, Li B, Cui Y, Zhang H, Zhang L, et al. Predicting Isocitrate Dehydrogenase (IDH) mutation status in gliomas using multiparameter MRI radiomics features. J Magn Reson Imaging. (2020) 53:1399–407. doi: 10.1002/jmri.27434
138. Sakai Y, Yang C, Kihira S, Tsankova N, Khan F, Hormigo A, et al. MRI radiomic features to predict IDH1 mutation status in gliomas: a machine learning approach using gradient tree boosting. Int J Mol Sci. (2020) 21:8004. doi: 10.3390/ijms21218004
139. Wu S, Meng J, Yu Q, Li P, Fu S. Radiomics-based machine learning methods for isocitrate dehydrogenase genotype prediction of diffuse gliomas. J Cancer Res Clin Oncol. (2019) 145:543–50. doi: 10.1007/s00432-018-2787-1
140. Yu J, Shi Z, Lian Y, Li Z, Liu T, Gao Y, et al. Noninvasive IDH1 mutation estimation based on a quantitative radiomics approach for grade II glioma. Eur Radiol. (2017) 27:3509–22. doi: 10.1007/s00330-016-4653-3
141. Calabrese E, Villanueva-Meyer JE, Cha S. A fully automated artificial intelligence method for non-invasive, imaging-based identification of genetic alterations in glioblastomas. Sci Rep. (2020) 10:11852. doi: 10.1038/s41598-020-68857-8
142. Kihira S, Tsankova NM, Bauer A, Sakai Y, Mahmoudi K, Zubizarreta N, et al. Multiparametric MRI texture analysis in prediction of glioma biomarker status: added value of MR diffusion. Neurooncol Adv. (2021) 3:vdab051. doi: 10.1093/noajnl/vdab051
143. Ren Y, Zhang X, Rui W, Pang H, Qiu T, Wang J, et al. Noninvasive prediction of IDH1 mutation and ATRX expression loss in low-grade gliomas using multiparametric MR radiomic features. J Magn Reson Imaging. (2019) 49:808–17. doi: 10.1002/jmri.26240
144. Li Y, Liu X, Qian Z, Sun Z, Xu K, Wang K, et al. Genotype prediction of ATRX mutation in lower-grade gliomas using an MRI radiomics signature. Eur Radiol. (2018) 28:2960–8. doi: 10.1007/s00330-017-5267-0
145. Li Y, Qian Z, Xu K, Wang K, Fan X, Li S, et al. MRI features predict p53 status in lower-grade gliomas via a machine-learning approach. Neuroimage Clin. (2018) 17:306–11. doi: 10.1016/j.nicl.2017.10.030
146. Zhang X, Tian Q, Wang L, Liu Y, Li B, Liang Z, et al. Radiomics strategy for molecular subtype stratification of lower-grade glioma: detecting IDH and TP53 mutations based on multimodal MRI. J Magn Reson Imaging. (2018) 48:916–26. doi: 10.1002/jmri.25960
147. Casale R, Lavrova E, Sanduleanu S, Woodruff HC, Lambin P. Development and external validation of a non-invasive molecular status predictor of chromosome 1p/19q co-deletion based on MRI radiomics analysis of Low Grade Glioma patients. Eur J Radiol. (2021) 139:109678. doi: 10.1016/j.ejrad.2021.109678
148. Kong Z, Jiang C, Zhang Y, Liu S, Liu D, Liu Z, et al. Thin-slice magnetic resonance imaging-based radiomics signature predicts chromosomal 1p/19q co-deletion status in grade II and III gliomas. Front Neurol. (2020) 11:551771. doi: 10.3389/fneur.2020.551771
149. Han Y, Xie Z, Zang Y, Zhang S, Gu D, Zhou M, et al. Non-invasive genotype prediction of chromosome 1p/19q co-deletion by development and validation of an MRI-based radiomics signature in lower-grade gliomas. J Neurooncol. (2018) 140:297–306. doi: 10.1007/s11060-018-2953-y
150. Li Y, Liu X, Xu K, Qian Z, Wang K, Fan X, et al. MRI features can predict EGFR expression in lower grade gliomas: a voxel-based radiomic analysis. Eur Radiol. (2018) 28:356–62. doi: 10.1007/s00330-017-4964-z
151. Hu LS, Ning S, Eschbacher JM, Baxter LC, Gaw N, Ranjbar S, et al. Radiogenomics to characterize regional genetic heterogeneity in glioblastoma. Neuro Oncol. (2017) 19:128–37. doi: 10.1093/neuonc/now135
152. Fang S, Fan Z, Sun Z, Li Y, Liu X, Liang Y, et al. Radiomics features predict telomerase reverse transcriptase promoter mutations in world health organization grade II gliomas via a machine-learning approach. Front Oncol. (2020) 10:606741. doi: 10.3389/fonc.2020.606741
153. Jiang C, Kong Z, Zhang Y, Liu S, Liu Z, Chen W, et al. Conventional magnetic resonance imaging-based radiomic signature predicts telomerase reverse transcriptase promoter mutation status in grade II and III gliomas. Neuroradiology. (2020) 62:803–13. doi: 10.1007/s00234-020-02392-1
154. Tian H, Wu H, Wu G, Xu G. Noninvasive prediction of TERT promoter mutations in high-grade glioma by radiomics analysis based on multiparameter MRI. BioMed Res Int. (2020) 2020:3872314. doi: 10.1155/2020/3872314
155. Wei J, Yang G, Hao X, Gu D, Tan Y, Wang X, et al. A multi-sequence and habitat-based MRI radiomics signature for preoperative prediction of MGMT promoter methylation in astrocytomas with prognostic implication. Eur Radiol. (2019) 29:877–88. doi: 10.1007/s00330-018-5575-z
156. Jiang C, Kong Z, Liu S, Feng S, Zhang Y, Zhu R, et al. Fusion radiomics features from conventional MRI predict MGMT promoter methylation status in lower grade gliomas. Eur J Radiol. (2019) 121:108714. doi: 10.1016/j.ejrad.2019.108714
157. Li ZC, Bai H, Sun Q, Li Q, Liu L, Zou Y, et al. Multiregional radiomics features from multiparametric MRI for prediction of MGMT methylation status in glioblastoma multiforme: a multicentre study. Eur Radiol. (2018) 28:3640–50. doi: 10.1007/s00330-017-5302-1
158. Xi YB, Guo F, Xu ZL, Li C, Wei W, Tian P, et al. Radiomics signature: a potential biomarker for the prediction of MGMT promoter methylation in glioblastoma. J Magn Reson Imaging. (2018) 47:1380–7. doi: 10.1002/jmri.25860
159. Korfiatis P, Kline TL, Lachance DH, Parney IF, Buckner JC, Erickson BJ. Residual deep convolutional neural network predicts MGMT methylation status. J Digit Imaging. (2017) 30:622–8. doi: 10.1007/s10278-017-0009-z
160. Li Y, Qian Z, Xu K, Wang K, Fan X, Li S, et al. Radiomic features predict Ki-67 expression level and survival in lower grade gliomas. J Neurooncol. (2017) 135:317–24. doi: 10.1007/s11060-017-2576-8
161. Zhang L, Giuste F, Vizcarra JC, Li X, Gutman D. Radiomics features predict CIC mutation status in lower grade glioma. Front Oncol. (2020) 10:937. doi: 10.3389/fonc.2020.00937
162. Ellingson BM, Malkin MG, Rand SD, Connelly JM, Quinsey C, LaViolette PS, et al. Validation of functional diffusion maps (fDMs) as a biomarker for human glioma cellularity. J Magn Reson Imaging. (2010) 31:538–48. doi: 10.1002/jmri.22068
163. Mohsen LA, Shi V, Jena R, Gillard JH, Price SJ. Diffusion tensor invasive phenotypes can predict progression-free survival in glioblastomas. Br J Neurosurg. (2013) 27:436–41. doi: 10.3109/02688697.2013.771136
164. Lambin P, Rios-Velazquez E Leijenaar R, Carvalho S, van Stiphout RG, Granton P, Zegers CM, Gillies R, et al. Radiomics: extracting more information from medical images using advanced feature analysis. Eur J Cancer. (2012) 48:441–6. doi: 10.1016/j.ejca.2011.11.036
165. Lohmann P, Galldiks N, Kocher M, Heinzel A, Filss CP, Stegmayr C, et al. Radiomics in neuro-oncology: basics, workflow, and applications. Methods. (2021) 188:112–21. doi: 10.1016/j.ymeth.2020.06.003
166. Zhao SS, Feng XL, Hu YC, Han Y, Tian Q, Sun YZ, et al. Better efficacy in differentiating WHO grade II from III oligodendrogliomas with machine-learning than radiologist's reading from conventional T1 contrast-enhanced and fluid attenuated inversion recovery images. BMC Neurol. (2020) 20:48. doi: 10.1186/s12883-020-1613-y
167. Chang P, Grinband J, Weinberg BD, Bardis M, Khy M, Cadena G, et al. Deep-learning convolutional neural networks accurately classify genetic mutations in gliomas. AJNR Am J Neuroradiol. (2018) 39:1201–7. doi: 10.3174/ajnr.A5667
168. Rotkopf LT, Wiestler B, Preibisch C, Liesche-Starnecker F, Pyka T, Norenberg D, et al. The wavelet power spectrum of perfusion weighted MRI correlates with tumor vascularity in biopsy-proven glioblastoma samples. PloS ONE. (2020) 15:e0228030. doi: 10.1371/journal.pone.0228030
169. Fudaba H, Shimomura T, Abe T, Matsuta H, Momii Y, Sugita K, et al. Comparison of multiple parameters obtained on 3T pulsed arterial spin-labeling, diffusion tensor imaging, and MRS and the Ki-67 labeling index in evaluating glioma grading. AJNR Am J Neuroradiol. (2014) 35:2091–8. doi: 10.3174/ajnr.A4018
170. Li X, Lu Y, Pirzkall A, McKnight T, Nelson SJ. Analysis of the spatial characteristics of metabolic abnormalities in newly diagnosed glioma patients. J Magn Reson Imaging. (2002) 16:229–37. doi: 10.1002/jmri.10147
171. Wang Y, Zhang T, Li S, Fan X, Ma J, Wang L, et al. Anatomical localization of isocitrate dehydrogenase 1 mutation: a voxel-based radiographic study of 146 low-grade gliomas. Eur J Neurol. (2015) 22:348–54. doi: 10.1111/ene.12578
172. Smits M, van den Bent MJ. Imaging correlates of adult glioma genotypes. Radiology. (2017) 284:316–31. doi: 10.1148/radiol.2017151930
173. Patel SH, Poisson LM, Brat DJ, Zhou Y, Cooper L, Snuderl M, et al. T2-FLAIR mismatch, an imaging biomarker for IDH and 1p/19q status in lower-grade gliomas: a TCGA/TCIA project. Clin Cancer Res. (2017) 23:6078–85. doi: 10.1158/1078-0432.CCR-17-0560
174. Broen MPG, Smits M, Wijnenga MMJ, Dubbink HJ, Anten M, Schijns O, et al. The T2-FLAIR mismatch sign as an imaging marker for non-enhancing IDH-mutant, 1p/19q-intact lower-grade glioma: a validation study. Neuro Oncol. (2018) 20:1393–9. doi: 10.1093/neuonc/noy048
175. Goyal A, Yolcu YU, Goyal A, Kerezoudis P, Brown DA, Graffeo CS, et al. The T2-FLAIR-mismatch sign as an imaging biomarker for IDH and 1p/19q status in diffuse low-grade gliomas: a systematic review with a Bayesian approach to evaluation of diagnostic test performance. Neurosurg Focus. (2019) 47:E13. doi: 10.3171/2019.9.FOCUS19660
176. Lai A, Kharbanda S, Pope WB, Tran A, Solis OE, Peale F, et al. Evidence for sequenced molecular evolution of IDH1 mutant glioblastoma from a distinct cell of origin. J Clin Oncol. (2011) 29:4482–90. doi: 10.1200/JCO.2010.33.8715
177. Andronesi OC, Kim GS, Gerstner E, Batchelor T, Tzika AA, Fantin VR, et al. Detection of 2-hydroxyglutarate in IDH-mutated glioma patients by in vivo spectral-editing and 2D correlation magnetic resonance spectroscopy. Sci Transl Med. (2012) 4:116ra4. doi: 10.1126/scitranslmed.3002693
178. Diamandis E, Gabriel CPS, Wurtemberger U, Guggenberger K, Urbach H, Staszewski O, et al. MR-spectroscopic imaging of glial tumors in the spotlight of the 2016 WHO classification. J Neurooncol. (2018) 139:431–40. doi: 10.1007/s11060-018-2881-x
179. Zhao J, Huang Y, Song Y, Xie D, Hu M, Qiu H, et al. Diagnostic accuracy and potential covariates for machine learning to identify IDH mutations in glioma patients: evidence from a meta-analysis. Eur Radiol. (2020) 30:4664–74. doi: 10.1007/s00330-020-06717-9
180. Jenkinson MD, du Plessis DG, Smith TS, Joyce KA, Warnke PC, Walker C. Histological growth patterns and genotype in oligodendroglial tumours: correlation with MRI features. Brain. (2006) 129:1884–91. doi: 10.1093/brain/awl108
181. Brown R, Zlatescu M, Sijben A, Roldan G, Easaw J, Forsyth P, et al. The use of magnetic resonance imaging to noninvasively detect genetic signatures in oligodendroglioma. Clin Cancer Res. (2008) 14:2357–62. doi: 10.1158/1078-0432.CCR-07-1964
182. Chawla S, Krejza J, Vossough A, Zhang Y, Kapoor GS, Wang S, et al. Differentiation between oligodendroglioma genotypes using dynamic susceptibility contrast perfusion-weighted imaging and proton MR spectroscopy. AJNR Am J Neuroradiol. (2013) 34:1542–9. doi: 10.3174/ajnr.A3384
183. Kapoor GS, Gocke TA, Chawla S, Whitmore RG, Nabavizadeh A, Krejza J, et al. Magnetic resonance perfusion-weighted imaging defines angiogenic subtypes of oligodendroglioma according to 1p19q and EGFR status. J Neurooncol. (2009) 92:373–86. doi: 10.1007/s11060-009-9880-x
184. Li Q, Dong F, Jiang B, Zhang M. Exploring MRI characteristics of brain diffuse midline gliomas with the H3 K27M mutation using radiomics. Front Oncol. (2021) 11:646267. doi: 10.3389/fonc.2021.646267
185. Christians A, Hartmann C, Benner A, Meyer J, von Deimling A, Weller M, et al. Prognostic value of three different methods of MGMT promoter methylation analysis in a prospective trial on newly diagnosed glioblastoma. PloS ONE. (2012) 7:e33449. doi: 10.1371/journal.pone.0033449
186. Moon WJ, Choi JW, Roh HG, Lim SD, Koh YC. Imaging parameters of high grade gliomas in relation to the MGMT promoter methylation status: the CT, diffusion tensor imaging, and perfusion MR imaging. Neuroradiology. (2012) 54:555–63. doi: 10.1007/s00234-011-0947-y
187. Ahn SS, Shin NY, Chang JH, Kim SH, Kim EH, Kim DW, et al. Prediction of methylguanine methyltransferase promoter methylation in glioblastoma using dynamic contrast-enhanced magnetic resonance and diffusion tensor imaging. J Neurosurg. (2014) 121:367–73. doi: 10.3171/2014.5.JNS132279
188. Korfiatis P, Kline TL, Coufalova L, Lachance DH, Parney IF, Carter RE, et al. MRI texture features as biomarkers to predict MGMT methylation status in glioblastomas. Med Phys. (2016) 43:2835. doi: 10.1118/1.4948668
189. Yoshida R, Sasaki T, Umekage Y, Tanno S, Ono Y, Ogata M, et al. Highly sensitive detection of ALK resistance mutations in plasma using droplet digital PCR. BMC Cancer. (2018) 18:1136. doi: 10.1186/s12885-018-5031-0
Keywords: glioma, glioblastoma, radiomics, radiogenomics, liquid biopsy, diagnosis, preoperative, noninvasive
Citation: Balana C, Castañer S, Carrato C, Moran T, Lopez-Paradís A, Domenech M, Hernandez A and Puig J (2022) Preoperative Diagnosis and Molecular Characterization of Gliomas With Liquid Biopsy and Radiogenomics. Front. Neurol. 13:865171. doi: 10.3389/fneur.2022.865171
Received: 29 January 2022; Accepted: 05 May 2022;
Published: 26 May 2022.
Edited by:
Dario de Biase, University of Bologna, ItalyReviewed by:
Vincenzo Di Nunno, AUSL Bologna, ItalyCopyright © 2022 Balana, Castañer, Carrato, Moran, Lopez-Paradís, Domenech, Hernandez and Puig. This is an open-access article distributed under the terms of the Creative Commons Attribution License (CC BY). The use, distribution or reproduction in other forums is permitted, provided the original author(s) and the copyright owner(s) are credited and that the original publication in this journal is cited, in accordance with accepted academic practice. No use, distribution or reproduction is permitted which does not comply with these terms.
*Correspondence: Carmen Balana, Y2JhbGFuYUBpY29uY29sb2dpYS5uZXQ=
Disclaimer: All claims expressed in this article are solely those of the authors and do not necessarily represent those of their affiliated organizations, or those of the publisher, the editors and the reviewers. Any product that may be evaluated in this article or claim that may be made by its manufacturer is not guaranteed or endorsed by the publisher.
Research integrity at Frontiers
Learn more about the work of our research integrity team to safeguard the quality of each article we publish.