- 1Research Center for Clinical Oncology, Jiangsu Cancer Hospital, Jiangsu Institute of Cancer Research, The Affiliated Cancer Hospital of Nanjing Medical University, Nanjing, China
- 2Department of Radiology, Jiangsu Cancer Hospital, Jiangsu Institute of Cancer Research, The Affiliated Cancer Hospital of Nanjing Medical University, Nanjing, China
- 3Department of Oncology, Jiangsu Cancer Hospital, Jiangsu Institute of Cancer Research, The Affiliated Cancer Hospital of Nanjing Medical University, Nanjing, China
Introduction: Some previous studies in patients with lung cancer have mainly focused on exploring the cognitive dysfunction and deficits of brain function associated with chemotherapy. However, little is known about functional brain alterations that might occur prior to chemotherapy. Therefore, this study aimed to evaluate brain functional changes in patients with nonchemotherapy before chemotherapy with non-small cell lung cancer (NSCLC).
Methods: Resting-state functional MRI data of 35 patients with NSCLC and 46 matched healthy controls (HCs) were acquired to construct functional brain networks. Graph theoretical analysis was then applied to investigate the differences of the network and nodal measures between groups. Finally, the receiver operating characteristic (ROC) curve analysis was performed to distinguish between NSCLC and HC.
Results: Decreased nodal strength was found in the left inferior frontal gyrus (opercular part), inferior frontal gyrus (triangular part), inferior occipital gyrus, and right inferior frontal gyrus (triangular part) of patients with NSCLC while increased nodal strength was found in the right pallidum and thalamus. NSCLC also showed decreased nodal betweenness in the right superior occipital gyrus. Different hub regions distribution was found between groups, however, no hub regions showed group differences in the nodal measures. Furthermore, the ROC curve analysis showed good performance in distinguishing NSCLC from HC.
Conclusion: These results suggested that topological abnormalities of pallido-thalamo-cortical circuit in functional brain network might be related to NSCLC prior to chemotherapy, which provided new insights concerning the pathophysiological mechanisms of NSCLC and could serve as promising biological markers for the identification of patients with NSCLC.
Introduction
Lung cancer is one of the most malignant cancers with high morbidity and mortality rate for both men and women in the world. Non-small cell lung cancer (NSCLC) is one subtype of lung cancer, which accounts for 85% of all the lung cancers (1). Although lung cancer has not been associated historically with abnormalities of the central neural system, an increasing body of evidence suggests that patients with lung cancer may be associated with neurocognitive impairments (2–5). Survivors of lung cancer have a high incidence of cognitive deficits, such as executive function and working memory (6–8). The above problems may be caused by chemotherapy or by altered brain functional activity associated with cancer (9–11). However, the underlying mechanisms are not completely clear.
Advanced neuroimaging studies in patients with cancer provide a better understanding of chemotherapy-induced neurocognitive dysfunction or that related to cancer itself (12–14). Resting-state functional MRI (rs-fMRI) is a noninvasive neuroimaging technology with relatively high spatial and temporal resolution (15, 16). The method of rs-fMRI has been widely used in the studies of brain function by evaluating the temporal correlations of neuronal activity based on blood oxygenation level-dependent (BOLD) signals between different areas of the brain during rest (17–20). In addition, it has been widely implemented to explore the characteristics of functional brain networks and investigate the neuronal pathophysiology in many neuropsychiatric diseases (21, 22). Previous neuroimaging studies have shown that cross-sectional alterations of brain function were associated with cancer status while longitudinal abnormalities of brain function were related to the neurotoxic brain injury of chemotherapy (23, 24). Changes of brain function have been found to be associated with chemotherapy in patients with lung cancer in previous fMRI studies (11, 25).
Recently, graph theoretical analysis has been applied to explore the topological properties of brain networks in individuals with cognitive impairments, such as Alzheimer's disease and schizophrenia (26, 27). Using this method, the whole brain is considered as a network of nodes and edges (connections between nodes) resembling functional connections between all regions in the brain (28–31). Based on the method of graph theoretical analysis, both the changes of network measures and alterations only affecting distinct nodes (brain regions; nodal measures) can be explored (28, 32–34). A previous study using graph theoretical analysis showed that the functional brain network became inclined toward regular network in breast cancer survivors who were treated with chemotherapy and the functional brain network became inclined toward random network in breast cancer survivors who did not receive chemotherapy treatment (24). Patients with breast cancer were also found to have altered small-world properties and lower tolerance to attacks, which was associated with cognitive impairment (35). Patients with prostate cancer exhibited altered network organization and disrupted nodal characteristics in frontal and temporal regions in a previous study using diffusion-weighted imaging and graph theory (36). Patients with gynecological cancer had significant decrease in both the local efficiency and global efficiency of functional brain networks (37). However, it is unclear whether abnormal brain function already occurs in patients with lung cancer before the start of chemotherapy treatment.
The study about structural brain network demonstrated that patients with NSCLC had reduced clustering coefficient in the left hippocampus and increased shortest path length in the left middle frontal gyrus (orbital part), superior temporal gyrus and right Rolandic operculum, rectus gyrus, putamen, as well as decreased global efficacy in the left inferior frontal gyrus (triangular part), left inferior frontal gyrus (orbital part), right rolandic operculum, right gyrus rectus, right lenticular nucleus (putamen), left superior temporal gyrus and right inferior temporal gyrus and decreased local efficacy in the left middle frontal gyrus (orbital part), left superior temporal gyrus (2, 3). The changes of brain function may have preceded structural abnormalities. However, no study has examined the topological organization of the brain functional network in patients with NSCLC prior to chemotherapy.
In our clinical work, we found that many patients with lung cancer already had serious emotional and cognitive impairments before chemotherapy. However, many previous studies have ignored this problem or failed to explore the mechanisms of cancer-induced damages in the central nervous system from the perspective of neural circuits or brain networks. To better understand the impacts of chemotherapy on the brain, it is appropriate to first observe whether cancer itself has an impact on the function of the brain. Therefore, in this study, we investigated the network and nodal measures of the whole-brain functional network in patients with NSCLC who did not undergo chemotherapy. This allowed us to explore how the functional brain network was affected by the disease of NSCLC. We hypothesized that the topological properties of the functional brain network were disrupted in patients with NSCLC before chemotherapy. In addition, these altered measures might serve as potential biomarkers for distinguishing NSCLC from healthy control (HC).
Materials and Methods
Participants
We recruited 35 patients with NSCLC who had been diagnosed recently at the Department of Oncology, Jiangsu Cancer Hospital and Jiangsu Institute of Cancer Research and The Affiliated Cancer Hospital of Nanjing Medical University. A total of 46 age-, gender-, and education-matched HC were recruited through local advertisement. All the subjects were right-handed (self-reported) (Table 1).
Participants were eligible if they: (1) were aged from 40 to 70 years; (2) had no history of diagnosed neurological disorder, major psychiatric disorder, severe or chronic concomitant systemic illness potentially influencing brain function; (3) had no history of traumatic brain injury, disturbance of consciousness; (4) had no history of alcohol or drug abuse; and (5) had no contraindications to undergo MRI. All the patients had a histologically proven diagnosis of NSCLC and had received no radiation and/or chemotherapy treatment. Patients were excluded, if they had evidence of brain metastases on structural MRI.
This study was approved by the Medical Ethics Committee of Jiangsu Cancer Hospital and Jiangsu Institute of Cancer Research and The Affiliated Cancer Hospital of Nanjing Medical University. All the participants provided a written informed consent before this study.
Magnetic Resonance Imaging Data Acquisition
All the MRI data were obtained with a 3.0 T Philips Aachieva Scanner. T1-weighted images were acquired with the following parameters: repetition time (TR)/echo time (TE) = 9/2.48 ms; flip angle (FA) = 9°; field of view (FOV) = 200 × 200 mm2; matrix = 200 × 200; voxel size = 1 × 1 × 1 mm; slice gap = 0; slice number = 176; acquisition time = 4 min 24 s. The rs-fMRI data were acquired with the following parameters: TR/TE = 3,000/40 ms; FA = 90°; FOV = 240 × 240 mm2; matrix = 80 × 80; voxel size = 3 × 3 × 4 mm; slice gap = 0; slice number = 34; acquisition time = 6 min 48 s.
Magnetic Resonance Imaging Data Preprocessing
Magnetic resonance imaging data were preprocessed using the Data Processing Assistant for rs-fMRI advanced edition (DPARSF) based on MATLAB and SPM (38) (Figure 1). The standard preprocessing steps were as follows: (1) the first 6 volumes were discarded for signal stabilization; (2) time differences in slice acquisition and head motion were corrected; (3) realignment to the middle image; (4) spatial normalization to the Montreal Neurological Institute (MNI) template; (5) resampled to 3 × 3 × 3 mm3; (6) a 4-mm full-width half-maximum (FWHM) Gaussian kernel for spatial smoothing is applied; (7) signal linear detrending was performed; (8) filtered with a temporal band-path of 0.01–0.1 Hz to reduce the low-frequency drift and high-frequency respiratory and cardiac noise; (9) nuisance covariates including the white matter signal, cerebrospinal fluid signal, and Friston 24 motion parameters were regressed out. Participants were excluded if the head motion exceeded 2 mm of translation or 2° of rotation in any direction.
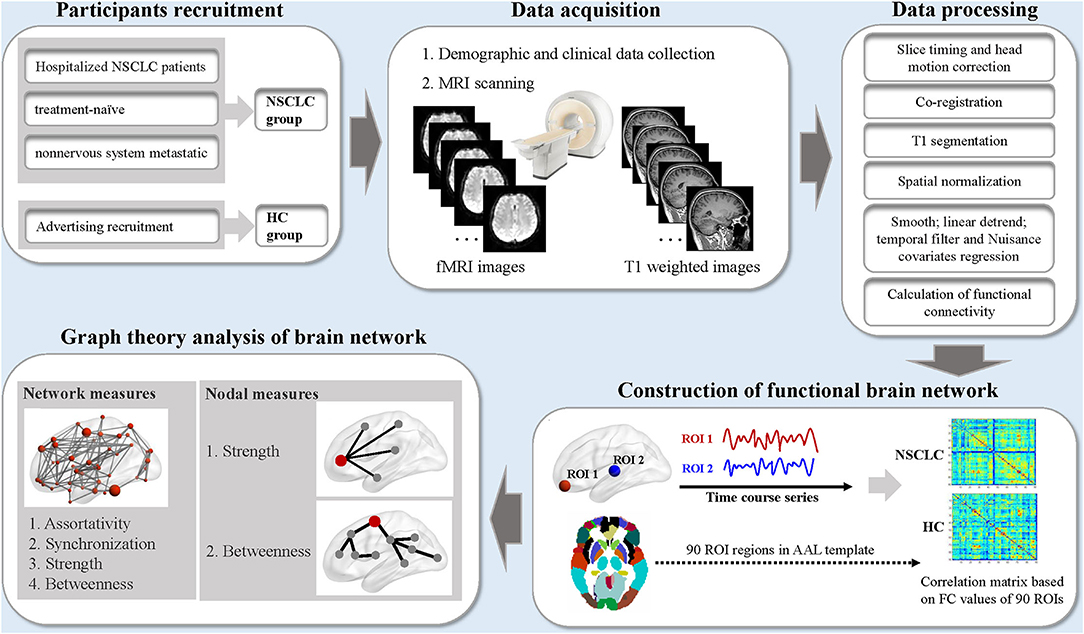
Figure 1. The schematic diagram for the acquisition, preprocessing, and graph theoretical analysis of resting-state functional MRI data in this study. NSCLC, non-small cell lung cancer; HC, healthy control; fMRI, functional MRI; ROI, region of interest; AAL, anatomic automatic labeling. MRI data were processed by the Data Processing Assistant for Resting State fMRI software (DPARSF). The nodal and network measures of the functional brain network were calculated by using the GRaph thEoreTical Network Analysis (GRETNA) toolbox.
Construction of Functional Brain Network
A network can be constructed by defining nodes and estimating edges (28). The whole brain was firstly parcellated into 90 regions of interest (ROIs) by the Automated Anatomical Labeling (AAL) atlas and each of the ROI was considered as a node in the functional brain network (39). Pearson's correlations between the time series of all ROIs were extracted and were transformed into z-scores via Fisher's transformation, which were defined as the connection strength of edges in the functional brain network. Finally, a functional connectivity matrix was obtained, and then undirected weighted network was constructed.
Calculation of Network and Nodal Measures
The network and nodal measures of the functional brain network were calculated using the GRETNA toolbox (40). For the functional brain network at each sparsity threshold, we calculated the following parameters: (1) network and nodal measures including assortativity, synchronization, strength, and betweenness; (2) nodal measures including strength and betweenness (28).
Nodal strength is computed as the sum of weights of all edges that are connected to it in the whole network (41). Nodal betweenness is calculated as the number of all shortest paths that pass through it in the while network (42). Network assortativity is considered as the nodal strength correlation between connected nodes in the network (43, 44). A network with high assortativity indicates that nodes with similar nodal strength tend to be connected to each other (45, 46). In this study, assortativity reflects the tendency of brain regions to be connected to other regions with the same or similar nodal strength. Network synchronization measures how likely it is that all nodes fluctuate in the same wave pattern (47). More detailed information about these measures could be found in the previous study (28). To investigate the group differences of the functional brain network, area under the curve (AUC) was calculated over sparsity ranges for each measure. The AUC metric is a summarized scalar, which is free from single threshold selection and is sensitive at detecting topological characteristics of the brain network. In addition, nodes were identified as hub regions with nodal strength and betweenness at least one SD greater than the mean of all the nodes.
Statistical Analysis
Comparisons of both the network and nodal measures between patients with NSCLC and HC were performed by using the independent two-sample two-tailed t-tests. In addition, the false discovery rate (FDR) was used for multiple comparison corrections (48). The FDR-corrected significance level was set at P < 0.05. Furthermore, the receiver operating characteristic (ROC) curves were generated to investigate the discriminating values of both network and nodal measures for discriminating patients with NSCLC from HC. P < 0.05 was considered as statistically significant.
Results
Differences of Network Measures Between the Groups
In this study, the functional brain networks of all the participants were thresholded with varied sparsity from 0.05 to 0.95 with step 0.05. No significant differences were found in the assortativity and synchronization of the functional brain network between NSCLC and HC (sparsity ranges: 0.05–0.95). In addition, there were no significant group differences in the strength and betweenness of the functional brain network across the sparsity range from 0.05 to 0.95. Moreover, patients with NSCLC exhibited no significant differences in the AUC of these network measures when compared with HC (sparsity ranges from 0.05 to 0.95) (Figure 2).
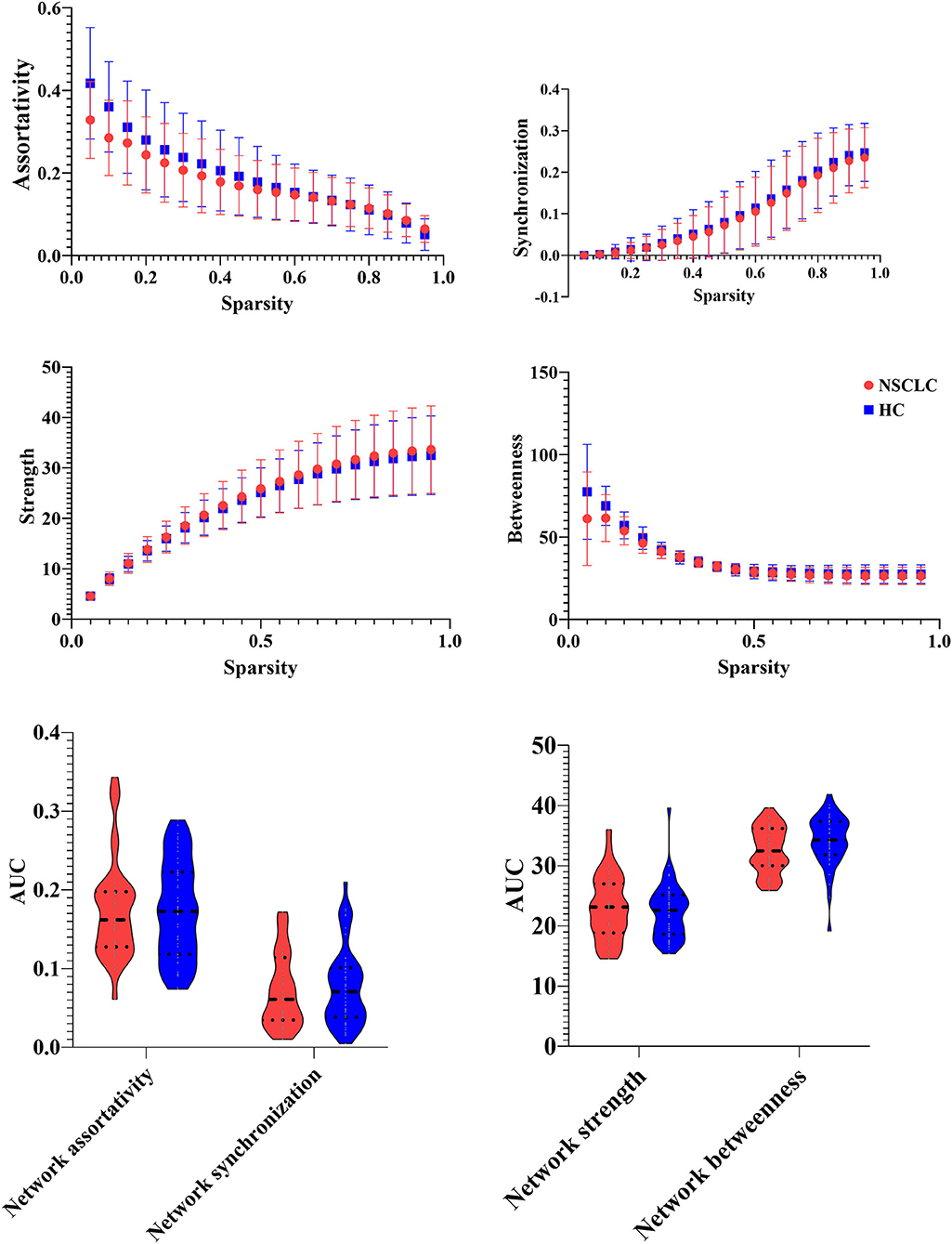
Figure 2. Group differences in the network measures of the functional brain networks among different sparsity thresholds (0.05–0.95) between the groups.
Differences of Nodal Measures Between the Groups
In the range of 0.05–0.95, the AUC of the nodal strength and betweenness in the functional brain networks for the NSCLC and HC groups were calculated and then compared. The decreased AUC of the nodal strength was found in the left inferior frontal gyrus (opercular part), inferior frontal gyrus (triangular part), inferior occipital gyrus, and right inferior frontal gyrus (triangular part) of patients with NSCLC when compared with HC while increased AUC of the nodal strength was found in the right pallidum and thalamus. Furthermore, patients with NSCLC showed decreased AUC of the nodal betweenness in the right superior occipital gyrus when compared with HC. All the above differences survived FDR correction (Table 2; Figure 3).
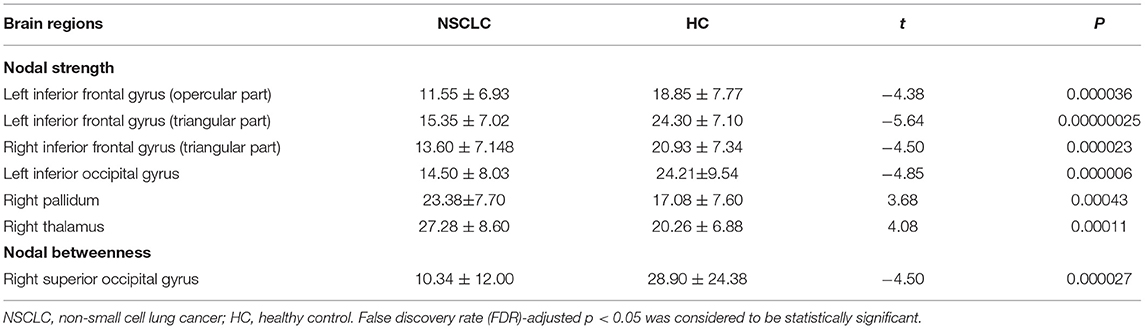
Table 2. Brain regions showed altered nodal measures in the functional brain networks of patients with NSCLC.
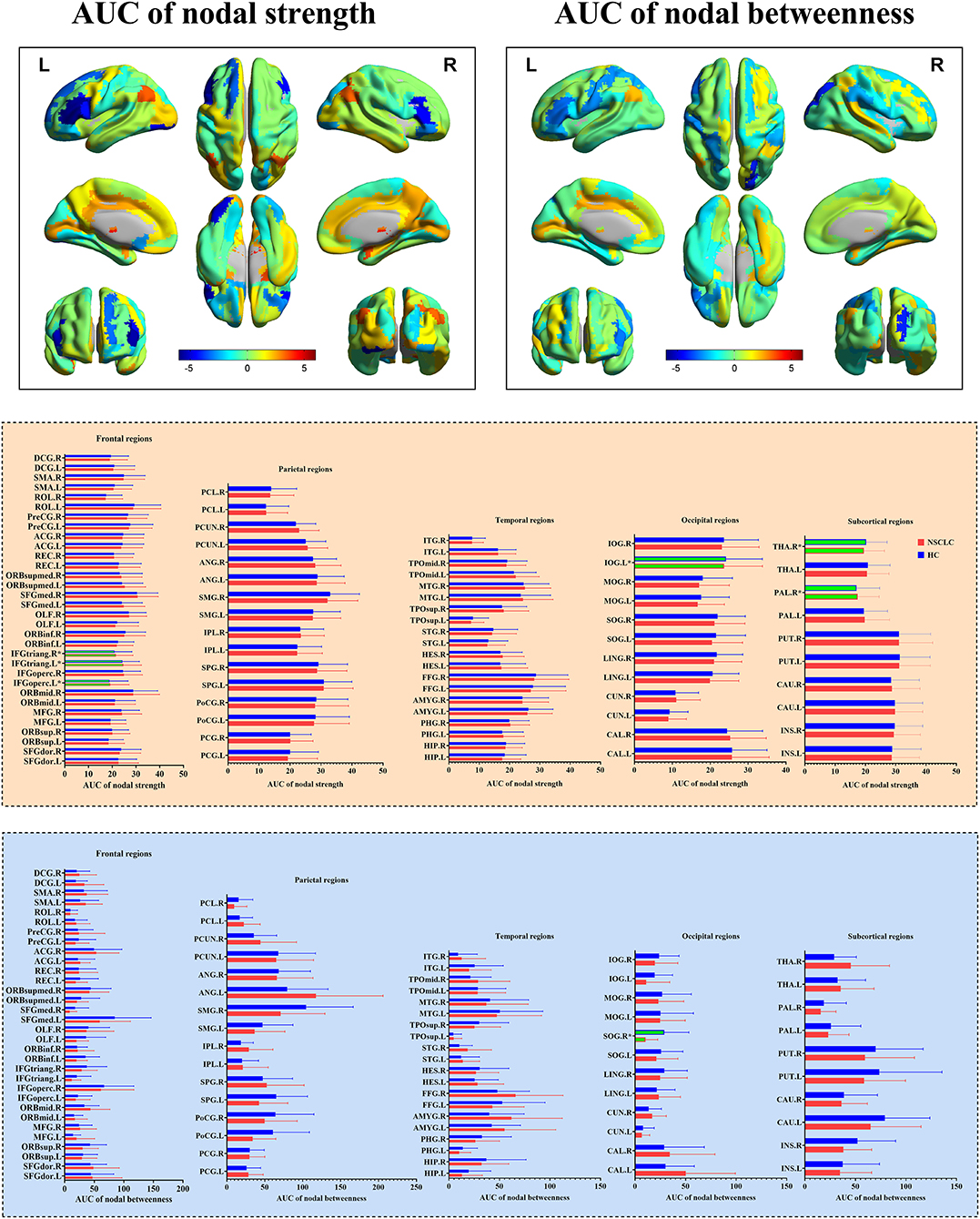
Figure 3. Group differences in the nodal measures of the functional brain networks between NSCLC and HC. NSCLC, non-small cell lung cancer; HC, healthy control. The superscript * and green column indicated brain regions with significant differences surviving false discovery rate (FDR) correction. The color bar indicated t-values of the two-sample t-tests. The abbreviations of brain regions were presented in the Supplementary Material online.
Differences of Hub Regions Distribution Between the Groups
First, nodes with high nodal strength were classified as hub regions, which had intense interconnectivity with other regions in the brain and played an important in the information transfer and integration. Seventeen such hubs were identified in the NSCLC group, including 3 frontal regions, 3 temporal regions, 5 parietal regions, 2 occipital regions, and 4 subcortical regions. In the HC group, 17 regions were also identified as hubs, including 3 frontal regions, 2 temporal regions, 6 parietal regions, and 6 subcortical regions. Eleven of these regions (left and right superior parietal gyrus, putamen, and insula; left anterior cingulate gyrus; right middle frontal gyrus (orbital part), superior frontal gyrus (medial), fusiform gyrus, and supramarginal gyrus) were shared by both the NSCLC and HC groups. The NSCLC group lost hub properties in the left and right postcentral gyrus, caudate nucleus, left rolandic operculum, and fusiform gyrus, while the left and right amygdala, calcarine fissure, left precentral gyrus, and right anterior cingulate gyrus acted as new hub regions in the patient group (Table 3; Figure 4).
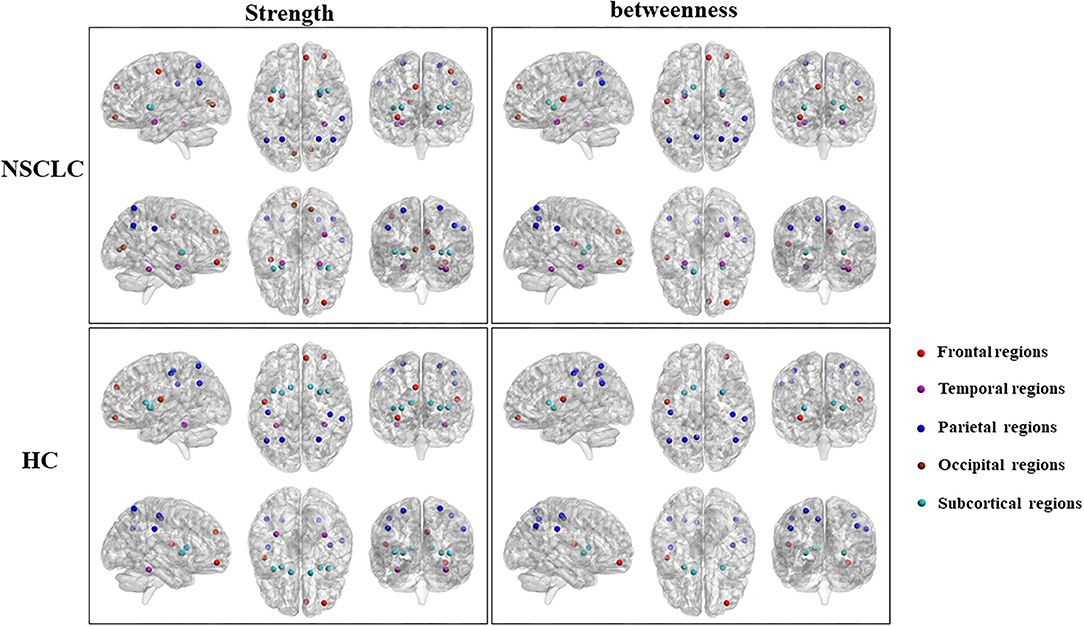
Figure 4. Differences in the distribution of hub regions between NSCLC and HC. NSCLC, non-small cell lung cancer; HC, healthy control. The abbreviations of brain regions were presented in the Supplementary Material online.
Second, nodes with high nodal betweenness were classified as hub regions, which lied on the shortest path between two other regions and played an important role in controlling information flow. Based on this definition of hub regions, patients with NSCLC presented 14 brain regions (3 frontal regions, 3 temporal regions, 5 parietal regions; 23 subcortical regions) acting as hubs while HC presented 12 regions (2 frontal regions, 7 parietal regions; 3 subcortical regions). Nine of these regions (left and right anterior cingulate gyrus and putamen; left rolandic operculum, precuneus, and caudate nucleus; right middle frontal gyrus (orbital part) and supramarginal gyrus) were shared by two groups. The left and right amygdala, right superior frontal gyrus (medial), fusiform gyrus and superior parietal gyrus were defined as hub regions in the NSCLC group, but not in the HC group. In the left and right postcentral gyrus, left superior parietal gyrus was defined as hub regions only in HC (Table 3; Figure 4).
Receiver Operating Characteristic for Discrimination Between NSCLC and HC
Significant differences of the nodal measures suggested that they could be useful biomarkers for discriminating NSCLC from HC. ROC curves were generated and AUCs were calculated (Figure 5). The ROC analysis demonstrated that the strength of left inferior frontal gyrus (opercular part) (AUC = 0.78; p < 0.001, 95% CI: 0.67–0.88; sensitivity: 82.86% and specificity: 65.22%), inferior frontal gyrus (triangular part) (AUC = 0.82; p < 0.001, 95% CI: 0.72–0.92; sensitivity: 71.4% and specificity: 86.96%), inferior occipital gyrus (AUC = 0.79; p < 0.001, 95% CI: 0.69–0.89; sensitivity: 71.4% and specificity: 73.91%) and right inferior frontal gyrus (triangular part) (AUC = 0.77; p < 0.001, 95% CI: 0.66–0.87; sensitivity: 68.6% and specificity: 78.26%), pallidum (AUC = 0.72; p < 0.001, 95% CI: 0.61–0.83; sensitivity: 48.6% and specificity: 89.13%) and thalamus (AUC = 0.73; p < 0.001, 95% CI: 0.62–0.84; sensitivity: 82.9% and specificity: 56.52%) exhibited good performance in distinguishing patients with NSCLC from HC, as well as the betweenness of right superior occipital gyrus (AUC = 0.76; p < 0.001, 95% CI: 0.66–0.87; sensitivity: 60% and specificity: 82.61%).
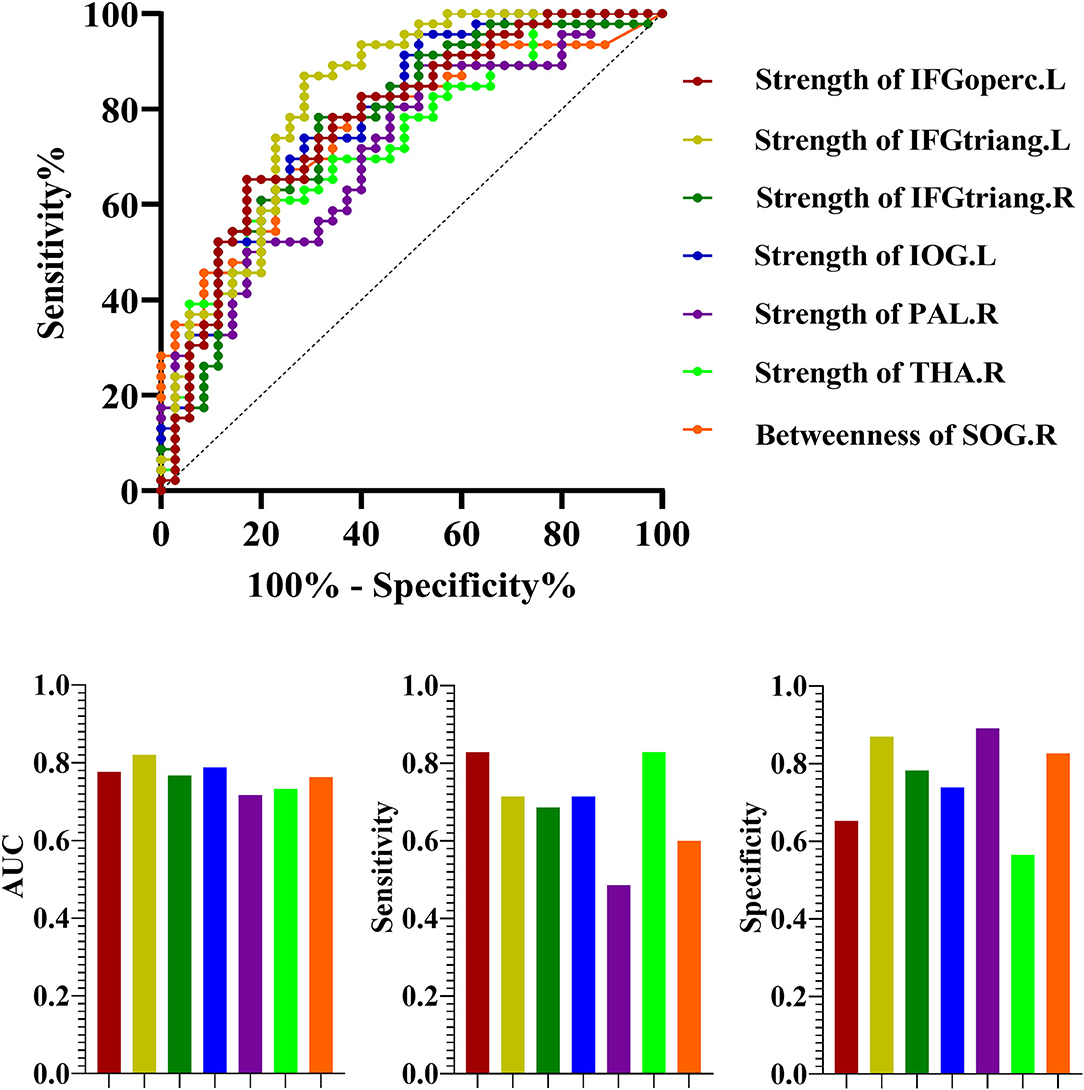
Figure 5. The receiver operating characteristic curve (ROC) for discrimination between NSCLC and HC. NSCLC, non-small cell lung cancer; HC, healthy control; AUC, area under the curve. The abbreviations of brain regions were presented in the Supplementary Material online.
Discussion
In this study, there were no significant differences in the network assortative and synchronization between patients with NSCLC prior to chemotherapy and HC. However, significant group differences were found in the nodal strength and betweenness, mainly located in the frontal and subcortical regions. The frontal regions showed decreased nodal strength while increased nodal strength was identified in the pallidum and thalamus of patients with NSCLC. These findings demonstrated that abnormal topological features of the pallido-thalamo-cortical circuit might be associated with NSCLC, which occurred in the functional brain network of patients with NSCLC before the start of chemotherapy treatment. In addition, the ROC curve revealed that the abnormal nodal measures of pallido-thalamo-cortical circuit exhibited robust accuracy and excellent specificity in distinguishing NSCLC from HC. Therefore, it appeared that nodal strength and betweenness of these brain regions could be used to characterize the severity of NSCLC, at least to some extent.
Chemotherapy-related cognitive impairment (chemobrain) had been found in patients with cancer outside the central nervous system in previous neuropsychological studies (49, 50). Chemotherapy-related cognitive impairment was explored from 1978, however, the first study about cancer-related cognitive impairment was conducted in 2008 (51, 52). The cognitive impairment was detected in 20–30% of patients with cancer outside the central nervous system before the start of chemotherapy (53, 54). Therefore, cancer itself might induce biological mechanisms, which are involved in the development of cognitive impairment in patients with cancer. The brain had been found to play an essential role in the cognitive function, such as attention, memory, and executive function (55, 56). With the development of neuroimaging methods, especially fMRI, the relationships between brain activity and cognition could be revealed (57, 58). Previous fMRI studies had found that many cognitive processes were located in the frontal regions of the brain, which could be activated by tasks related to cognition (59, 60). However, the underlying neurobiological mechanisms related to cancer-related cognitive impairment had not been elucidated.
In this study, increased nodal strength was found in the left inferior frontal gyrus (opercular part), inferior frontal gyrus (triangular part), inferior occipital gyrus, and right inferior frontal gyrus (triangular part) of patients with NSCLC. The inferior frontal gyrus was found to be related to the process such as attention and executive function and non-invasive brain stimulation of the inferior frontal gyrus significantly improved the cognition (61). In addition, the connections between the inferior frontal gyrus and subcortical regions were found to be involved in the executive processing (62). Decreased functional connectivity between the left inferior frontal gyrus (triangular part) and dorsolateral prefrontal cortex was found in patients with lung cancer after chemotherapy when compared with HC while decreased functional connectivity between the left inferior frontal gyrus and the right dorsolateral prefrontal cortex was identified in patients with lung cancer after chemotherapy when compared with those before treatment (6). Abnormal functional connectivity between these two brain regions was found to be correlated to the worse neuropsychologic performance of patients with cancer (63). However, hyperactivity was found in the right inferior frontal gyrus and several other regions in the pathway of visceral to brain signal transduction in patients with NSCLC prior to oncotherapy by using resting-state 18F-fluoro-D-glucose (FDG) PET/CT and the abnormal glucose metabolism might be attributed to NSCLC related visceral sympathetic activation (64).
The interactions between the brain and cancer outside the central nervous system suggested that cancer information could be conveyed into the brain by the special structures within the spinal and cranial and nerves and the brain could consequently regulate the growth of cells of cancer in peripheral tissues by the neuroendocrine-immune system (65). Therefore, non-central nervous system cancer itself could induce abnormal function of certain regions in the brain, which might play an essential role in the progression and metastases of cancer. Decreased nodal strength of bilateral inferior frontal gyrus in this study suggested that reduced functional connectivity between these regions and other regions occurred before the treatment of chemotherapy. This finding was consistent with previous studies, which indicated that patients with cancer had cognitive impairment or emotional problems prior to chemotherapy, as well as impaired brain function or structure. The decreased functional connectivity of the inferior frontal gyrus might also lead to the reduced level of executive control and increased speed of cancer growth.
Meantime, the nodal strength of the right pallidum and thalamus was increased in the NSCLC group. These two subcortical regions were the central components of the cancer-to-brain pathway, which might be hyperactivated as a compensatory reaction (65). The pallidum and thalamus are strongly connected with the cortical regions, especially pallido-thalamo-cortical circuit, which is involved in a variety of cognitive processes (66, 67). Abnormal activation of pallidum was identified in patients with cancer, which might lead to impaired reward and motivation processing (68). Increased activation during working memory was found in the right thalamus in patients with cancer before chemotherapy (69). In addition, the cognitive impairment together with structural brain changes in the thalamus was found in patients with lung cancer (70). The pallidum, one component of the basal ganglia, was found to be associated with the cognitive performance of patients (71). The thalamus had complex connections with the prefrontal regions and thalamo-cortical connections had been found to be involved in various cognitive processes (72). Therefore, we suspected that the increased functional connectivity of the pallidum and thalamus in this study might reflect an attempt to reinstate homeostasis in functions related to cancer and the visceral sympathetic activation.
No significant group differences were found in the network assortative and synchronization. An assortative network has highly connected nodes, which improves network resilience and reduces disruption loss (73, 74). Therefore, this type of network facilitates the spread of information over the network (75). The index of network synchronization reflects the possibility of all the nodes fluctuate in the same wave pattern (47, 76). In this study, there were no significant changes in the global measures including network assortative and synchronization, which suggested that NSCLC was not enough to cause topological properties changes of the functional brain network from the global perspective. Based on both nodal strength and betweenness, patients with NSCLC lost hub properties in some regions, while some hub regions were identified in patients with NSCLC, which might represent a compensatory mechanism. Highly connected nodes or nodes with high betweenness are defined as hubs, which play a key role in the integration of information (28, 77). In this study, no selective damages were found in these hub regions, therefore, the global characteristics of the functional brain networks were not impaired in patients with NSCLC.
These findings about cancer-induced impacts on the brain are of great significance to explore whether brain function or microstructure damages caused by non-central nervous system metastatic cancers can predict the probability or mechanisms of brain metastasis in advanced cancers in the future studies. Although altered nodal measures could be useful biomarkers for discriminating NSCLC from HC by the method of the AUC, we would explore the validity of the prediction with different machine learning models with the increase of sample size and longitudinal follow-up data. In addition, although rs-fMRI is a valid method to observe the changes of brain function and microstructure, this method may be indirect when compared with other methods, such as animal experiments. Therefore, we will also establish NSCLC animal rat models, and observe the cognitive and emotional impairments of rats before and following the use of chemotherapeutic drugs longitudinally in our future studies. The level of dopamine (DA) and 5-hydroxytryptamine (5-HT) in the related brain areas will also be compared before and following chemotherapy. Finally, we aim to provide the theoretical basis for optimizing the efficacy of NSCLC chemotherapy and reducing treatment-related cognitive and emotional impairments, and further to develop the individualized and precise treatments for NSCLC.
Several limitations of this study should be further discussed. First, the sample size was relatively small, which might reduce the generalizability of our results to some extent. Second, the present study was a cross-sectional study, which could not elucidate the causal links between NSCLC and abnormal functional connectivity. Third, lacking the results of cognitive performance did not allow us to explore the relationships between altered brain function and cognitive impairment of patients with NSCLC. Finally, smoking and lifestyle might be also associated with the development of NSCLC and these factors should be considered in our further task-based fMRI studies.
Conclusion
In conclusion, this was the first study to explore the topological features of the functional brain networks of patients with NSCLC prior to chemotherapy. Despite the limitations of this study, the findings revealed that abnormal functional connectivity of the pallido-thalamo-cortical circuit occurred before the start of chemotherapy treatment, which also showed good performance in distinguishing NSCLC from HC. Therefore, this circuit might be a potential biomarker for NSCLC from the perspective of cancer.
Data Availability Statement
The original contributions presented in the study are included in the article/Supplementary Material, further inquiries can be directed to the corresponding authors.
Ethics Statement
The studies involving human participants were reviewed and approved by the Medical Ethics Committee of Jiangsu Cancer Hospital and Jiangsu Institute of Cancer Research and The Affiliated Cancer Hospital of Nanjing Medical University. The patients/participants provided their written informed consent to participate in this study.
Author Contributions
SL, JF, BS, and JW designed the experiments. SL, NY, CL, XL, JN, XP, and RM contributed to clinical data collection and assessment. SL, NY, CL, and XL analyzed the results and contributed to the manuscript. SL, JF, and BS approved the final manuscript. All authors contributed to the article and approved the submitted version.
Funding
This study was supported by the grants of the National Natural Science Foundation of China (No. 81871873), the Foundation of Jiangsu Cancer Hospital (No. ZM201923), the Jiangsu Provincial Top Talent Scientific Research Project of Six One Projects for High Level Health Talents (No. LGY2019076), and the Young Talents Program of Jiangsu Cancer Hospital (No. QL201814).
Conflict of Interest
The authors declare that the research was conducted in the absence of any commercial or financial relationships that could be construed as a potential conflict of interest.
Publisher's Note
All claims expressed in this article are solely those of the authors and do not necessarily represent those of their affiliated organizations, or those of the publisher, the editors and the reviewers. Any product that may be evaluated in this article, or claim that may be made by its manufacturer, is not guaranteed or endorsed by the publisher.
Supplementary Material
The Supplementary Material for this article can be found online at: https://www.frontiersin.org/articles/10.3389/fneur.2022.821470/full#supplementary-material
References
1. Global, regional, and and national burden of respiratory tract cancers and associated risk factors from 1990 to 2019: a systematic analysis for the Global Burden of Disease Study 2019. Lancet Respir Med. (2021) 9:1030–49. doi: 10.1016/S2213-2600(21)00164-8
2. Liu S, Yin N, Ma R, Cao H, Jing C, Zhang Y, et al. Abnormal topological characteristics of brain white matter network relate to cognitive and emotional deficits of non-small cell lung cancer (NSCLC) patients prior to chemotherapy. Int J Neurosci. (2020) 3:1–10. doi: 10.1080/00207454.2020.1813130
3. Liu S, Li X, Ma R, Cao H, Jing C, Wang Z, et al. Cancer-associated changes of emotional brain network in non-nervous system metastatic non-small cell lung cancer patients: a structural connectomic diffusion tensor imaging study. Transl Lung Cancer Res. (2020) 9:1101–11. doi: 10.21037/tlcr-20-273
4. Van Der Weijst L, Surmont V, Schrauwen W, Lievens Y. Real life data on patient-reported outcomes and neuro-cognitive functioning of lung cancer patients: the PRO-long study. Front Oncol. (2021) 11:685605. doi: 10.3389/fonc.2021.685605
5. van de Kamp HJ, Molder MT, Schulkes KJG, Stellingwerf M, van Elden LJR, van Lindert ASR, et al. Impact of lung cancer treatment on cognitive functioning. Clin Lung Cancer. (2020) 21:114–126 e113. doi: 10.1016/j.cllc.2019.06.006
6. Hu L, Chen H, Su W, Zhang Y, You J, Gu W, et al. Aberrant static and dynamic functional connectivity of the executive control network in lung cancer patients after chemotherapy: a longitudinal fMRI study. Brain Imaging Behav. (2020) 14:927–40. doi: 10.1007/s11682-020-00287-6
7. Komaki R, Meyers CA, Shin DM, Garden AS, Byrne K, Nickens JA, et al. Evaluation of cognitive function in patients with limited small cell lung cancer prior to and shortly following prophylactic cranial irradiation. Int J Radiat Oncol Biol Phys. (1995) 33:179–82. doi: 10.1016/0360-3016(95)00026-U
8. Grosshans DR, Meyers CA, Allen PK, Davenport SD, Komaki R. Neurocognitive function in patients with small cell lung cancer : effect of prophylactic cranial irradiation. Cancer. (2008) 112:589–95. doi: 10.1002/cncr.23222
9. Lange M, Joly F, Vardy J, Ahles T, Dubois M, Tron L, et al. Cancer-related cognitive impairment: an update on state of the art, detection, and management strategies in cancer survivors. Ann Oncol. (2019) 30:1925–40. doi: 10.1093/annonc/mdz410
10. Cascella M, Di Napoli R, Carbone D, Cuomo GF, Bimonte S, Muzio MR. Chemotherapy-related cognitive impairment: mechanisms, clinical features and research perspectives. Recenti Prog Med. (2018) 109:523–30. doi: 10.1701/3031.30289
11. Zhang Y, Chen YC, Hu L, You J, Gu W, Li Q, et al. Chemotherapy-induced functional changes of the default mode network in patients with lung cancer. Brain Imaging Behav. (2020) 14:847–56. doi: 10.1007/s11682-018-0030-y
12. Niu R, Du M, Ren J, Qing H, Wang X, Xu G, et al. Chemotherapy-induced grey matter abnormalities in cancer survivors: a voxel-wise neuroimaging meta-analysis. Brain Imaging Behav. (2020). doi: 10.1007/s11682-020-00402-7
13. Dietrich J, Prust M, Kaiser J. Chemotherapy, cognitive impairment and hippocampal toxicity. Neuroscience. (2015) 309:224–32. doi: 10.1016/j.neuroscience.2015.06.016
14. Deprez S, Billiet T, Sunaert S, Leemans A. Diffusion tensor MRI of chemotherapy-induced cognitive impairment in non-CNS cancer patients: a review. Brain Imaging Behav. (2013) 7:409–35. doi: 10.1007/s11682-012-9220-1
15. Lee MH, Smyser CD, Shimony JS. Resting-state fMRI: a review of methods and clinical applications. AJNR Am J Neuroradiol. (2013) 34:1866–72. doi: 10.3174/ajnr.A3263
16. Smitha KA, Akhil Raja K, Arun KM, Rajesh PG, Thomas B, Kapilamoorthy TR, et al. Resting state fMRI: A review on methods in resting state connectivity analysis and resting state networks. Neuroradiol J. (2017) 30:305–17. doi: 10.1177/1971400917697342
17. Fox MD, Raichle ME. Spontaneous fluctuations in brain activity observed with functional magnetic resonance imaging. Nat Rev Neurosci. (2007) 8:700–11. doi: 10.1038/nrn2201
18. Fair DA, Schlaggar BL, Cohen AL, Miezin FM, Dosenbach NU, Wenger KK, et al. method for using blocked and event-related fMRI data to study “resting state” functional connectivity. Neuroimage. (2007) 35:396–405. doi: 10.1016/j.neuroimage.2006.11.051
19. Raichle ME. Two views of brain function. Trends Cogn Sci. (2010) 14:180–90. doi: 10.1016/j.tics.2010.01.008
20. Zhang D, Raichle ME. Disease and the brain's dark energy. Nat Rev Neurol. (2010) 6:15–28. doi: 10.1038/nrneurol.2009.198
21. Dong D, Wang Y, Chang X, Luo C, Yao D. Dysfunction of large-scale brain networks in schizophrenia: a meta-analysis of resting-state functional connectivity. Schizophr Bull. (2018) 44:168–81. doi: 10.1093/schbul/sbx034
22. Pan P, Zhan H, Xia M, Zhang Y, Guan D, Xu Y. Aberrant regional homogeneity in Parkinson's disease: a voxel-wise meta-analysis of resting-state functional magnetic resonance imaging studies. Neurosci Biobehav Rev. (2017) 72:223–31. doi: 10.1016/j.neubiorev.2016.11.018
23. Feng Y, Zhang XD, Zheng G, Zhang LJ. Chemotherapy-induced brain changes in breast cancer survivors: evaluation with multimodality magnetic resonance imaging. Brain Imaging Behav. (2019) 13:1799–814. doi: 10.1007/s11682-019-00074-y
24. Chen VC, Lin KY, Tsai YH, Weng JC. Connectome analysis of brain functional network alterations in breast cancer survivors with and without chemotherapy. PLoS ONE. (2020) 15:e0232548. doi: 10.1371/journal.pone.0232548
25. Mentzelopoulos A, Gkiatis K, Karanasiou I, Karavasilis E, Papathanasiou M, Efstathopoulos E, et al. Chemotherapy-induced brain effects in small-cell lung cancer patients: a multimodal MRI study. Brain Topogr. (2021) 34:167–81. doi: 10.1007/s10548-020-00811-3
26. Lin S, Lin C, Hsieh T, Lin C, Chen S, Chao Y, et al. Multiparametric graph theoretical analysis reveals altered structural and functional network topology in Alzheimer's disease. NeuroImage Clin. (2019) 22:101680. doi: 10.1016/j.nicl.2019.101680
27. Jiang Y, Yao D, Zhou J, Tan Y, Huang H, Wang M, et al. Characteristics of disrupted topological organization in white matter functional connectome in schizophrenia. Psychol Med. (2020) 3:1–11. doi: 10.1017/S0033291720003141
28. Rubinov M, Sporns O. Complex network measures of brain connectivity: uses and interpretations. Neuroimage. (2010) 52:1059–69. doi: 10.1016/j.neuroimage.2009.10.003
29. Reijneveld JC, Ponten SC, Berendse HW, Stam CJ. The application of graph theoretical analysis to complex networks in the brain. Clin Neurophysiol. (2007) 118:2317–31. doi: 10.1016/j.clinph.2007.08.010
30. Bullmore E, Sporns O. Complex brain networks: graph theoretical analysis of structural and functional systems. Nat Rev Neurosci. (2009) 10:186–98. doi: 10.1038/nrn2575
31. Lo CY, He Y, Lin CP. Graph theoretical analysis of human brain structural networks. Rev Neurosci. (2011) 22:551–63. doi: 10.1515/RNS.2011.039
32. Xia M, He Y. Magnetic resonance imaging and graph theoretical analysis of complex brain networks in neuropsychiatric disorders. Brain Connect. (2011) 1:349–65. doi: 10.1089/brain.2011.0062
33. Bassett DS, Bullmore E. Small-world brain networks. Neuroscientist. (2006) 12:512–23. doi: 10.1177/1073858406293182
34. Sporns O. Structure and function of complex brain networks. Dialogues Clin Neurosci. (2013) 15:247–62. doi: 10.31887/DCNS.2013.15.3/osporns
35. Kesler SR, Watson CL, Blayney DW. Brain network alterations and vulnerability to simulated neurodegeneration in breast cancer. Neurobiol Aging. (2015) 36:2429–42. doi: 10.1016/j.neurobiolaging.2015.04.015
36. C RB, Zachariae R, Buus S, C HG, Haldbo-Classen L, Hosseini SMH, et al. Cognitive impairment and associations with structural brain networks, endocrine status, and risk genotypes in patients with newly diagnosed prostate cancer referred to androgen-deprivation therapy. Cancer. (2021) 127:1495–506. doi: 10.1002/cncr.33387
37. Zeng Y, Cheng ASK, Song T, Sheng X, Cheng H, Qiu Y, et al. Changes in functional brain networks and neurocognitive function in Chinese gynecological cancer patients after chemotherapy: a prospective longitudinal study. BMC Cancer. (2019) 19:386. doi: 10.1186/s12885-019-5576-6
38. Chao-Gan Y, Yu-Feng Z. DPARSF A MATLAB Toolbox for “Pipeline” Data Analysis of Resting-State fMRI. Front Syst Neurosci. (2010) 4:13. doi: 10.3389/fnsys.2010.00013
39. Tzourio-Mazoyer N, Landeau B, Papathanassiou D, Crivello F, Etard O, Delcroix N, et al. Automated anatomical labeling of activations in SPM using a macroscopic anatomical parcellation of the MNI MRI single-subject brain. Neuroimage. (2002) 15:273–89. doi: 10.1006/nimg.2001.0978
40. Wang J, Wang X, Xia M, Liao X, Evans A, He Y, et al. GRETNA: a graph theoretical network analysis toolbox for imaging connectomics. Front Hum Neurosci. (2015) 9:386. doi: 10.3389/fnhum.2015.00386
41. Wang JH, Zuo XN, Gohel S, Milham MP, Biswal BB, He Y. Graph theoretical analysis of functional brain networks: test-retest evaluation on short- and long-term resting-state functional MRI data. PLoS ONE. (2011) 6:e21976. doi: 10.1371/journal.pone.0021976
42. Zuo XN, Ehmke R, Mennes M, Imperati D, Castellanos FX, Sporns O, et al. Network centrality in the human functional connectome. Cerebral Cortex. (2012) 22:1862–75. doi: 10.1093/cercor/bhr269
43. Newman ME. Modularity and community structure in networks. Proc Natl Acad Sci U S A. (2006) 103:8577–82. doi: 10.1073/pnas.0601602103
44. Bassett DS, Bullmore E, Verchinski BA, Mattay VS, Weinberger DR, Meyer-Lindenberg A. Hierarchical organization of human cortical networks in health and schizophrenia. J Neurosci. (2008) 28:9239–48. doi: 10.1523/JNEUROSCI.1929-08.2008
45. Newman ME. Assortative mixing in networks. Phys Rev Lett. (2002) 89:208701. doi: 10.1103/PhysRevLett.89.208701
46. Hagmann P, Cammoun L, Gigandet X, Meuli R, Honey CJ, Wedeen VJ, et al. Mapping the structural core of human cerebral cortex. PLoS Biol. (2008) 6:e159. doi: 10.1371/journal.pbio.0060159
47. Xin Z, Chen X, Zhang Q, Wang J, Xi Y, Liu J, et al. Alteration in topological properties of brain functional network after 2-year high altitude exposure: a panel study. Brain Behav. (2020) 10:e01656. doi: 10.1002/brb3.1656
48. Genovese CR, Lazar NA, Nichols T. Thresholding of statistical maps in functional neuroimaging using the false discovery rate. Neuroimage. (2002) 15:870–8. doi: 10.1006/nimg.2001.1037
49. Lv L, Mao S, Dong H, Hu P, Dong R. Pathogenesis, assessments, and management of chemotherapy-related cognitive impairment (CRCI): an updated literature review. J Oncol. (2020) 2020:3942439. doi: 10.1155/2020/3942439
50. Loh KP, Janelsins MC, Mohile SG, Holmes HM, Hsu T, Inouye SK, et al. Chemotherapy-related cognitive impairment in older patients with cancer. J Geriatr Oncol. (2016) 7:270–80. doi: 10.1016/j.jgo.2016.04.008
51. Levine PM, Silberfarb PM, Lipowski ZJ. Mental disorders in cancer patients: a study of 100 psychiatric referrals. Cancer. (1978) 42:1385–91. doi: 10.1002/1097-0142(197809)42:3<1385::aid-cncr2820420349>3.0.co;2-0
52. Ahles TA, Saykin AJ, McDonald BC, Furstenberg CT, Cole BF, Hanscom BS, et al. Cognitive function in breast cancer patients prior to adjuvant treatment. Breast Cancer Res Treat. (2008) 110:143–52. doi: 10.1007/s10549-007-9686-5
53. Schilder CM, Seynaeve C, Linn SC, Boogerd W, Beex LV, Gundy CM, et al. Cognitive functioning of postmenopausal breast cancer patients before adjuvant systemic therapy, and its association with medical and psychological factors. Crit Rev Oncol Hematol. (2010) 76:133–41. doi: 10.1016/j.critrevonc.2009.11.001
54. Hermelink K, Untch M, Lux MP, Kreienberg R, Beck T, Bauerfeind I, et al. Cognitive function during neoadjuvant chemotherapy for breast cancer: results of a prospective, multicenter, longitudinal study. Cancer. (2007) 109:1905–13. doi: 10.1002/cncr.22610
55. Hopfinger JB, Slotnick SD. Attentional control and executive function. Cogn Neurosci. (2020) 11:1–4. doi: 10.1080/17588928.2019.1682985
56. Sheffield JM, Barch DM. Cognition and resting-state functional connectivity in schizophrenia. Neurosci Biobehav Rev. (2016) 61:108–20. doi: 10.1016/j.neubiorev.2015.12.007
57. Rucco R, Lardone A, Liparoti M, Troisi Lopez E, De Micco R, Tessitore A, et al. Brain networks and cognitive impairment in Parkinson's disease. Brain Connect. (2021). doi: 10.1089/brain.2020.0985. [Epub ahead of print].
58. Du J, Zhu H, Zhou J, Lu P, Qiu Y, Yu L, et al. Structural brain network disruption at preclinical stage of cognitive impairment due to cerebral small vessel disease. Neuroscience. (2020) 449:99–115. doi: 10.1016/j.neuroscience.2020.08.037
59. Raju VB, Shukla A, Jacob A, Bharath RD, Kumar VK, Varambally S, et al. The frontal pole and cognitive insight in schizophrenia. Psychiatry Res Neuroimaging. (2021) 308:111236. doi: 10.1016/j.pscychresns.2020.111236
60. Castelnovo V, Canu E, Calderaro D, Riva N, Poletti B, Basaia S, et al. Progression of brain functional connectivity and frontal cognitive dysfunction in ALS. NeuroImage Clin. (2020) 28:102509. doi: 10.1016/j.nicl.2020.102509
61. Eliasova I, Anderkova L, Marecek R, Rektorova I. Non-invasive brain stimulation of the right inferior frontal gyrus may improve attention in early Alzheimer's disease: a pilot study. J Neurol Sci. (2014) 346:318–22. doi: 10.1016/j.jns.2014.08.036
62. Baláz M, Srovnalová H, Rektorová I, Rektor I. The effect of cortical repetitive transcranial magnetic stimulation on cognitive event-related potentials recorded in the subthalamic nucleus. Exp Brain Res. (2010) 203:317–27. doi: 10.1007/s00221-010-2232-4
63. Wang L, Yan Y, Wang X, Tao L, Chen Q, Bian Y, et al. Executive function alternations of breast cancer patients after chemotherapy: evidence from resting-state functional MRI. Acad Radiol. (2016) 23:1264–70. doi: 10.1016/j.acra.2016.05.014
64. Zhang W, Ning N, Li X, Niu G, Bai L, Guo Y, et al. Changes of brain glucose metabolism in the pretreatment patients with non-small cell lung cancer: a retrospective PET/CT Study. PLoS ONE. (2016) 11:e0161325. doi: 10.1371/journal.pone.0161325
65. Ondicova K, Mravec B. Role of nervous system in cancer aetiopathogenesis. Lancet Oncol. (2010) 11:596–601. doi: 10.1016/S1470-2045(09)70337-7
66. Li MG, He JF, Liu XY, Wang ZF, Lou X, Ma L. Structural and functional thalamic changes in Parkinson's disease with mild cognitive impairment. J Magn Reson Imaging. (2020) 52:1207–15. doi: 10.1002/jmri.27195
67. Kawabata K, Watanabe H, Bagarinao E, Ohdake R, Hara K, Ogura A, et al. Cerebello-basal ganglia connectivity fingerprints related to motor/cognitive performance in Parkinson's disease. Parkinsonism Relat Disord. (2020) 80:21–7. doi: 10.1016/j.parkreldis.2020.09.005
68. Nock NL, Dimitropolous A, Tkach J, Frasure H, von Gruenigen V. Reduction in neural activation to high-calorie food cues in obese endometrial cancer survivors after a behavioral lifestyle intervention: a pilot study. BMC Neuroscience. (2012) 13:74. doi: 10.1186/1471-2202-13-74
69. Scherling C, Collins B, Mackenzie J, Bielajew C, Smith A. Pre-chemotherapy differences in visuospatial working memory in breast cancer patients compared to controls: an FMRI study. Front Hum Neurosci. (2011) 5:122. doi: 10.3389/fnhum.2011.00122
70. Simó M, Vaquero L, Ripollés P, Jové J, Fuentes R, Cardenal F, et al. Brain damage following prophylactic cranial irradiation in lung cancer survivors. Brain Imaging Behav. (2016) 10:283–95. doi: 10.1007/s11682-015-9393-5
71. Petkus AJ, Jarrahi B, Holschneider DP, Gomez ME, Filoteo JV, Schiehser DM, et al. Thalamic volume mediates associations between cardiorespiratory fitness (VO(2)max) and cognition in Parkinson's disease. Parkinsonism Relat Disord. (2021) 86:19–26. doi: 10.1016/j.parkreldis.2021.03.019
72. Steiner L, Federspiel A, Slavova N, Wiest R, Grunt S, Steinlin M, et al. Functional topography of the thalamo-cortical system during development and its relation to cognition. NeuroImage. (2020) 223:117361. doi: 10.1016/j.neuroimage.2020.117361
73. Murakami M, Ishikura S, Kominami D, Shimokawa T, Murata M. Robustness and efficiency in interconnected networks with changes in network assortativity. Appl Netw Sci. (2017) 2:6. doi: 10.1007/s41109-017-0025-4
74. Pritchard WS, Laurienti PJ, Burdette JH, Hayasaka S. Functional brain networks formed using cross-sample entropy are scale free. Brain Connect. (2014) 4:454–64. doi: 10.1089/brain.2013.0217
75. Lee DA, Lee HJ, Kim HC, Park KM. Temporal lobe epilepsy with or without hippocampal sclerosis: Structural and functional connectivity using advanced MRI techniques. J Neuroimaging. (2021) 31:973–980. doi: 10.1111/jon.12898
76. Kawasaki M, Kitajo K, Fukao K, Murai T, Yamaguchi Y, Funabiki Y. Frontal theta activation during motor synchronization in autism. Sci Rep. (2017) 7:15034. doi: 10.1038/s41598-017-14508-4
Keywords: non-small cell lung cancer, resting-state, functional MRI, graph theoretical analysis, functional brain network
Citation: Liu S, Yin N, Li C, Li X, Ni J, Pan X, Ma R, Wu J, Feng J and Shen B (2022) Topological Abnormalities of Pallido-Thalamo-Cortical Circuit in Functional Brain Network of Patients With Nonchemotherapy With Non-small Cell Lung Cancer. Front. Neurol. 13:821470. doi: 10.3389/fneur.2022.821470
Received: 24 November 2021; Accepted: 07 January 2022;
Published: 08 February 2022.
Edited by:
Alireza Mansouri, The Pennsylvania State University (PSU), United StatesReviewed by:
Jurgen Germann, University Health Network (UHN), CanadaAlexandre Boutet, University of Toronto, Canada
Copyright © 2022 Liu, Yin, Li, Li, Ni, Pan, Ma, Wu, Feng and Shen. This is an open-access article distributed under the terms of the Creative Commons Attribution License (CC BY). The use, distribution or reproduction in other forums is permitted, provided the original author(s) and the copyright owner(s) are credited and that the original publication in this journal is cited, in accordance with accepted academic practice. No use, distribution or reproduction is permitted which does not comply with these terms.
*Correspondence: Jifeng Feng, ZmppZkB2aXAuc2luYS5jb20=; Bo Shen, c2hlbmJvOTg3QDEyNi5jb20=
†These authors have contributed equally to this work