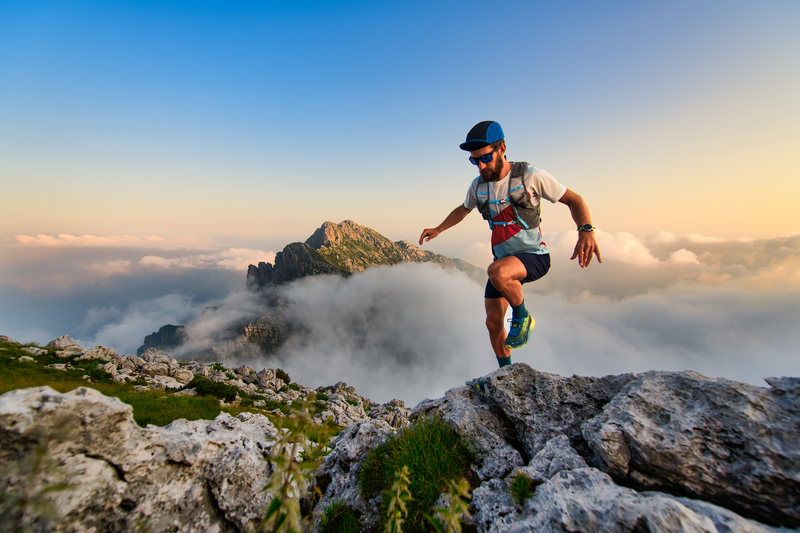
94% of researchers rate our articles as excellent or good
Learn more about the work of our research integrity team to safeguard the quality of each article we publish.
Find out more
ORIGINAL RESEARCH article
Front. Neurol. , 12 January 2023
Sec. Stroke
Volume 13 - 2022 | https://doi.org/10.3389/fneur.2022.1084616
This article is part of the Research Topic Post-Stroke Complications: Mechanisms, Diagnosis, and Therapies View all 20 articles
Objectives: Inflammatory response biomarkers are promising prognostic factors to improve the prognosis of stroke-associated pneumonia (SAP) after ischemic stroke. This study aimed to investigate the prognostic significance of inflammatory response biomarkers on admission in SAP after spontaneous intracerebral hemorrhage (SICH) and establish a corresponding nomogram.
Methods: The data of 378 patients with SICH receiving conservative treatment from January 2019 to December 2021 at Taizhou People's Hospital were selected. All eligible patients were randomized into the training (70%, 265) and validation cohorts (30%, 113). In the training cohort, multivariate logistic regression analysis was used to establish an optimal nomogram, including inflammatory response biomarkers and clinical risk factors. The area under the receiver operating characteristic (ROC) curve (AUC), calibration curve, and decision curve analysis (DCA) were used to evaluate the nomogram's discrimination, calibration, and performance, respectively. Moreover, this model was further validated in a validation cohort.
Results: A logistic regression analysis showed that intraventricular hemorrhage (IVH), hypertension, dysphagia, Glasgow Coma Scale (GCS), National Institute of Health Stroke Scale (NIHSS), systemic inflammation response index (SIRI), and platelet/lymphocyte ratio (PLR) were correlated with SAP after SICH (P < 0.05). The nomogram was composed of all these statistically significant factors. The inflammatory marker-based nomogram showed strong prognostic power compared with the conventional factors, with an AUC of 0.886 (95% CI: 0.841–0.921) and 0.848 (95% CI: 0.799–0.899). The calibration curves demonstrated good homogeneity between the predicted risks and the observed outcomes. In addition, the model has a significant net benefit for SAP, according to DCA. Also, internal validation demonstrated the reliability of the prediction nomogram. The length of hospital stay was shorter in the non-SAP group than in the SAP group. At the 3-month follow-up, clinical outcomes were worse in the SAP group (P < 0.001).
Conclusion: SIRI and PLR at admission can be utilized as prognostic inflammatory biomarkers in patients with SICH in the upper brain treated with SAP. A nomogram covering SIRI and PLR can more accurately predict SAP in patients' supratentorial SICH. SAP can influence the length of hospital stay and the clinical outcome.
Spontaneous intracerebral hemorrhage (SICH) has high morbidity, mortality, and medical complications, in addition to primary brain injury, are significant causes of adverse outcomes (1, 2). After the development of stroke-associated pneumonia (SAP), unfavorable conditions may result in prolonged hospitalization, poor functional recovery, high social and economic burden, and even death (3). A study found that the median length of hospital stay was longer in patients with SAP (13 days) than in those without SAP (5 days) (4). Therefore, as a rapidly progressive disease with high mortality, early identification and effective indicators of SAP prevention are essential. Several risk factors for pneumonia in stroke patients, namely age, immunosuppression, dysphagia, previous medical history (i.e., diabetes, atrial fibrillation, alcohol consumption, and COPD), and stroke severity, were highlighted (5–8). However, these risk factors largely depend on clinical symptoms, and clinical monitoring of SAP remains imprecise. To predict SAP occurrence, an objective predictor is essential.
There is growing evidence that the immunodeficiency syndrome caused by stroke promotes the development of SAP, suggesting the significance of immune-inflammatory processes in SAP (9, 10). Routine blood markers (i.e., neutrophils, lymphocytes, and monocytes) are common systemic inflammation and infection markers. In addition, the systemic inflammation response index (SIRI), neutrophil/lymphocyte ratio (NLR), monocyte/lymphocyte ratio (MLR), and platelet/lymphocyte ratio (PLR) have better predictive power than conventional inflammatory factors (11–14). Most studies on risk factors for SAP are based on ischemic stroke. The pathophysiological mechanisms of SICH and acute ischemic stroke (AIS) are very different. Previous studies have shown that high NLR and SIRI predict SAP in patients with AIS (15, 16). However, the clinical significance of these inflammatory factors for SAP after SICH remains questionable. Furthermore, no predictive models of inflammatory indicators have been developed to predict the occurrence of SAP after SICH. Identifying risk factors is based on targeted primary prevention strategies and may influence clinical management by optimizing patient care.
This study established a predictive nomogram as a simple statistical visualization tool to predict disease onset, progression, prognosis, and survival (17–19). This study aimed to assess whether inflammatory response biomarkers on admission contribute to the early prediction of SAP after SICH.
This observational study was approved by the local Ethics Committee of Taizhou People's Hospital and did not require individual patient consent (KY2022-094-01). The subjects of this retrospective study were patients with SICH who were admitted to Taizhou People's Hospital for conservative treatment from January 2019 to December 2021. SICH was determined by admission computed CT scanning. The decision of treatment modality of SICH (conservative treatment) was determined according to the diagnosis and treatment protocol, guidelines, and specific conditions of each patient. Inclusion criteria were: (1) CT diagnosis of SICH following the fourth national diagnostic criteria for cerebrovascular disease in 1995 in China, (2) CT follow-up within 24 h after admission, (3) age ≥18 years, (4) all patients were treated conservatively, and (5) diagnosing pneumonia was based on the diagnostic criteria for SAP in 2015 (20). We excluded patients with (1) infectious diseases, fever, or prophylactic antibiotics within 2 weeks before patient admission; (2) patients with infratentorial SICH; (3) cerebral hemorrhage due to trauma, subarachnoid hemorrhage due to aneurysm rupture and trauma; (4) patients after surgical treatment; (5) patients with autoimmune diseases, malignancies, hematological diseases, severe liver and kidney diseases, and history of major surgery; and (6) patients with incomplete information. We excluded patients with infratentorial cerebral hemorrhage. Patients with severe symptoms (GCS score ≤3) were excluded. Ultimately, 378 eligible patients were recruited. Patients were randomly classified into the training and validation cohorts in a 7:3 ratio (Figure 1).
Figure 1. A flowchart of the present study. SICH, spontaneous intracerebral hemorrhage; SAP, stroke-associated pneumonia.
All participating patients were reviewed for a range of risk factors associated with SAP, including age, gender, body mass index (BMI), Glasgow Coma Scale (GCS), National Institute of Health Stroke Scale (NIHSS) score, systolic blood pressure (SBP) and diastolic blood pressure (DBP) at admission, time from onset to hospitalization, dysphagia, hematoma volume, hematoma location, and intraventricular hemorrhage (IVH). Medical history was collected, that is, hypertension, diabetes, atrial fibrillation, smoking, drinking, and antiplatelet or anticoagulation. Laboratory parameters (red blood cells (RBC), white blood cells (WBC), platelets, absolute neutrophil count (ANC), absolute monocyte count (AMC), absolute lymphocyte count (ALC), and albumin) were obtained within 24 h of admission for all subjects. We collected the length of hospital stay and the functional recovery of the patients. To obtain their functional recovery, we followed up the patients or their families 3 months after discharge using a telephone.
A trained neurologist assessed the GCS and NIHSS scores at admission to assess the severity of SICH. The following formulae were used to compute the lymphocyte-based inflammatory index in this study: SIRI (21), NLR (15), MLR (14), and PLR (22) from the first peripheral blood count at admission.
The primary outcome of our study was SAP after SICH. Patients with signs or symptoms of respiratory infection underwent routine blood tests, and chest CT scans to diagnose pneumonia. Physicians from neurology and radiology jointly diagnosed pneumonia (20). Secondary outcomes were the length of stay and functional recovery. The modified Rankin Scale (mRS) score was utilized to evaluate functional recovery after 3 months, that is, an mRS score of 3–6 indicates poor clinical outcome.
Normally distributed continuous variables were expressed as mean ± standard deviation (M ± SD), and skewed distributions were expressed as median with interquartile range (IQR, Q1–Q3). Categorical variables are expressed as frequencies and percentages (%). Where appropriate, the t-test, the Mann–Whitney U-test, and the chi-square test were utilized for comparisons.
Multivariate logistic regression models considered variables with P < 0.05 in the univariate analysis results to obtain independent predictors. The Hosmer–Leeshawn test was utilized to assess the model's goodness of fit. In addition, a Nomogram with independent predictors was constructed from the training cohort. The area under the receiver operating characteristic (ROC) curve (AUC) and the calibration curves were utilized to assess the predictive power and compliance of the model. We performed a decision curve analysis (DCA) to quantify the net benefit of different threshold probabilities to determine the clinical utility of the nomogram we developed. After that, the visual prediction model was validated internally. Statistical analyses were performed on SPSS 26.0 (IBM Corporation, Chicago, IL) and R statistical software (R, version 4.1.1). Statistically significant differences were considered to be two-tailed at a P-value of < 0.05.
A total of 378 patients with SICH, including 265 in the training and 113 in the validation cohort, were included. Except for a statistically significant difference in GCS at admission (P < 0.05) regarding baseline characteristics, other variables did not differ between the two cohorts (Table 1). Patients with SAP (36. 2%) tended to be older, had higher rates of hypertension, antiplatelet or anticoagulation, dysphagia, and IVH, and had larger hematoma volumes, lower GCS scores, RBCs, ALC, hemoglobin, and albumin, and higher NIHSS scores, WBCs, ANC, SIRI, NLR, MLR, and PLR in the training cohort (P < 0.05, Table 2). Patients in the SAP group had a longer length of hospital stay (P < 0.05, Table 2). Three months after discharge, the mRS score of the SAP group differed from that of the non-SAP group (Table 2, P < 0.001). Clinical outcomes (mRS3-6) were significantly worse in the SAP group than in the non-SAP group (71.9 vs. 49.1%, P < 0.001, Table 2).
Table 2. General characteristics of patients with SICH according to the presence of SAP in the training cohort.
The variables with P < 0.05 in the univariate analysis were included in the multivariate logistic regression analysis. IVH, hypertension, dysphagia, GCS, NIHSS, SIRI, and PLR were independent predictors of SAP after SICH (P < 0.05, Table 3). The Hosmer–Lemeshow test showed a good fit for the model (P = 0.961).
An SAP predictive nomogram was established using the seven significant predictors mentioned earlier (Figure 2). The predictors were scored, and then a straight line was plotted through the total score to investigate the likelihood of assessing post-SICH SAP based on the total score.
In the training cohort, ROC analysis revealed an AUC of 0.886 (95% CI: 0.841–0.921, P < 0.001) for the nomogram for SAP (Figure 3A), higher than that without inflammatory factors (SIRI and PLR) (AUC = 0.848, 95% CI: 0.799–0.899, P < 0.001). The calibration curve was close to the ideal diagonal (Figure 4A).
Figure 3. ROC curves for the raining cohort (A) and validation cohort (B). ROC, receiver operating characteristic; AUC, area under the ROC.
Figure 4. Calibration curves for predicting the probability of SAP after SICH. (A) Training cohort. (B) Validation cohort.
Furthermore, 113 patients were utilized for the internal validation of the nomogram. The AUC of it was also 0.837 (95% CI: 0.756–0.900, P < 0.001), higher than the AUC without inflammatory factors (AUC = 0.752, 95% CI: 0.662–0.828, P < 0.001) (Figure 3B), confirming the nomogram's reliable accuracy. The calibration curve showed good consistency between the predicted and actual observed results in predicting SAP after SICH (Figure 4B). In addition, the DCA graph showed that the net benefit of the prediction model was better than that of the model without inflammatory factors over the risk range of SAP in both cohorts (Figure 5). These data suggest that our nomogram has important implications for clinical decision-making.
Our single-center retrospective study showed that IVH, hypertension, dysphagia, GCS, NIHSS, SIRI, and PLR were independent predictors of SAP after SICH. We further developed a nomogram to predict the incidence of SAP after SICH by these seven essential predictors. This nomogram yielded better accuracy and presented better clinical utility for the individualized prediction of SAP after SICH compared with the conventional factors without inflammatory factors. Our study is the first to include inflammatory markers in a predictive model for predicting SAP after SICH. Furthermore, this study makes predicting the probability of SAP after SICH easier. In addition, the nomogram underwent rigorous internal validation, implying stable prognostic performance.
SAP is the most common stroke-associated infection that can prolong hospitalization and even severely influence the prognosis and mortality of stroke patients (5). Therefore, early determination of disease trends and aggressive and effective treatment and prevention of patients who may develop SAP can reduce adverse outcomes. Despite the clinical significance of SAP after SICH, no substantial progress has been made in preventing SAP, including the prophylactic use of antibiotics and the process of care (23). It is well known that inflammatory factors, namely NLR, PLR, MLR, and SIRI, are new composite inflammatory markers based on traditional inflammatory cell counts that provide a more comprehensive picture of the inflammatory symptom status of the body. Numerous clinical studies (14, 15, 24–27) have confirmed that the above indicators have good predictive value for the occurrence, development, and prognosis of tumors, stroke, and other diseases. However, do these indicators have a similar clinical value for SAP after SICH?
We analyzed the relationship between peripheral blood and SAP in patients with SICH on admission. This study showed that SICH patients with hypertension, IVH, dysphagia, higher NIHSS scores, and lower GCS scores were more likely to have SAP. These results are similar to previous studies (5–7). In addition, this study added some inflammatory markers according to inflammatory cells in peripheral blood. Patients with SICH with higher SIRI and PLR were more likely to develop SAP. This result may provide a new idea to differentiate SAP and non-SAP individuals after SICH quantitatively and to develop targeted medical interventions for individuals.
It was revealed that elevated SIRI is an independent indicator of poor prognosis in stroke (24–26), aneurysmal subarachnoid hemorrhage (28), and some tumors (29–31). Yan et al. (16) found that higher SIRI was a significant risk factor for pneumonia in patients with acute ischemic stroke. A SIRI threshold of ≥2.74 was correlated with an increased incidence of SAP in patients with AIS (OR: 5.82, 95% CI: 4.54, 7.49, P < 0.001). In addition, the RCS model showed an increasing trend in the risk of SAP with increasing SIRI. Our study included patients with SICH who received conservative treatment. These results were similar to the previous studies mentioned above. SIRI revealed a positive association with SAP after SICH.
It was reported that PLR is a prognostic indicator of inflammatory response in various conditions, such as acute pulmonary embolism (32), myocardial infarction (33), various cancers (34), and stroke (27). Deng et al. (35) revealed that PLR was a predictor of stroke-associated infection in patients with AIS. A recent study reported that changes in peripheral PLR during treatment could reflect disease progression and prognosis in patients with COVID-19. Furthermore, the greater ΔPLR correlated with a more severe cytokine storm, a longer hospital stay, and a worse prognosis (36). The predictive value of PLR in patients with SAP vs. SICH has not been investigated. Furthermore, the combined effect of inflammatory factors on SAP has been well reported. We built a new nomogram to predict SAP risk in patients with SICH during hospitalization. The nomogram we constructed with inflammatory factors showed better and more accurate predictions than the nomogram without inflammatory factors. Internal validation further validated the predictive ability of the nomogram. Therefore, SIRI and PLR should be considered when predicting SAP in patients with SICH receiving conservative treatment.
Consistent with other reports (16, 37), the hospital stay length was prolonged in the SAP group. Studies have shown that stroke-related infections, especially pneumonia, are independently associated with poor functional prognosis after stroke. Our study also observed that subjects with SAP had worse functional outcomes at 3-month follow-up, consistent with previous studies (38–40). Two phase-II studies on prophylactic antibiotic therapy showed benefits on temperature, the incidence of infection, and even functional outcomes (41, 42). The current management of SAP does not prescribe prophylactic antibiotics (43). The challenge now is to study the effect of preventive treatment on functional outcomes. A phase-III trial was conducted but was stopped early.
There may be several possible mechanisms between the inflammatory response and SAP. First, experimental studies have shown that many inflammatory processes occur after cerebral hemorrhage, including infiltration of leukocytes (44), activation of microglia (45), and release of inflammatory cytokines (46). However, brain injury affects the physiological interaction between the central nervous system and the immune system, resulting in a systemic immunosuppressive syndrome (47) manifested by a decrease in lymphocytes (40) that promotes susceptibility to infection. Finally, inflammatory factors may be the connecting point between cerebral hemorrhage severity and SAP.
Despite the good performance of our nomogram, several limitations should be noted in our study. First, incomplete statistical indicators, such as the time of SAP, pathogenic spectrum analysis, mechanical ventilation use, indwelling gastric tubes, and aspiration events, were not well documented. Therefore, this classification of suspected risk factors and pathogens was not included in the statistical analysis, resulting in the exclusion of confounding confounders in the multivariate logistic regression. Second, the retrospective analysis was limited to a single center, and did not further comparison of the dynamics of inflammatory indicators. Therefore, the results of this study were further validated in prospective multicenter cohort studies and other populations. Finally, the training and validation cohorts were from the same hospital. Therefore, multicenter studies need to seek external validation assessments before clinical application.
Admission SIRI and PLR can be utilized as potential prognostic inflammatory biomarkers in patients with SICH who underwent SAP. It helps to select high-risk patients for timely initiation of individualized therapy as these variables can be easily and rapidly obtained from blood cell counts. Combining nomograms for admission SIRI, PLR, and clinical risk factors will more reliably predict SAP in patients with SICH. In the future, large studies are needed to externally validate the nomograms for SAP after SICH in different populations. If proven valid, it will provide clinicians with an accurate and effective tool for early prediction and timely management of SAP after SICH. SAP can influence the length of hospital stay and the clinical outcome.
The raw data supporting the conclusions of this article will be made available by the authors, without undue reservation. Requests to access the datasets should be directed to JJ, amp4ODMwODI5QDE2My5jb20=.
The studies involving human participants were reviewed and approved by the Ethics Committee of Taizhou People's Hospital. Written informed consent from the patients/participants or patients/participants' legal guardian/next of kin was not required to participate in this study in accordance with the national legislation and the institutional requirements.
JJ and YL: study design, result interpretation, writing, reviewing, and editing. HL: data acquisition. TY: writing of the original draft and statistical analysis. All authors contributed to the execution of this work and the preparation of this manuscript. All authors have read and agreed to publish the final version of the manuscript.
This study was supported by Taizhou, the fifth “311 Project” scientific research support project (RCPY 202129) to JJ, Science and Technology Development Fund of Shanghai Pudong New Area (Grant No. PKJ2021-Y09), and Scientific research start-up fund from the Affiliated Taizhou People's Hospital of Nanjing Medical University (2021).
The authors declare that the research was conducted in the absence of any commercial or financial relationships that could be construed as a potential conflict of interest.
All claims expressed in this article are solely those of the authors and do not necessarily represent those of their affiliated organizations, or those of the publisher, the editors and the reviewers. Any product that may be evaluated in this article, or claim that may be made by its manufacturer, is not guaranteed or endorsed by the publisher.
1. van Asch CJ, Luitse MJ, Rinkel GJ, van der Tweel I, Algra A, Klijn CJ. Incidence, case fatality, and functional outcome of intracerebral haemorrhage over time, according to age, sex, and ethnic origin: a systematic review and meta-analysis. Lancet Neurol. (2010) 9:167–76. doi: 10.1016/S1474-4422(09)70340-0
2. Koennecke HC, Belz W, Berfelde D, Endres M, Fitzek S, Hamilton F, et al. Factors influencing in-hospital mortality and morbidity in patients treated on a stroke unit. Neurology. (2011) 77:965–72. doi: 10.1212/WNL.0b013e31822dc795
3. Tinker RJ, Smith CJ, Heal C, Bettencourt-Silva JH, Metcalf AK, Potter JF, et al. Predictors of mortality and disability in stroke-associated pneumonia. Acta Neurol Belg. (2021) 121:379–85. doi: 10.1007/s13760-019-01148-w
4. Lakshminarayan K, Tsai AW, Tong X, Vazquez G, Peacock JM, George MG, et al. Utility of dysphagia screening results in predicting poststroke pneumonia. Stroke. (2010) 41:2849–54. doi: 10.1161/STROKEAHA.110.597039
5. Badve MS, Zhou Z, van de Beek D, Anderson CS, Hackett ML. Frequency of post-stroke pneumonia: systematic review and meta-analysis of observational studies. Int J Stroke. (2019) 14:125–36. doi: 10.1177/1747493018806196
6. Liu DD, Chu SF, Chen C, Yang PF, Chen NH, He X. Research progress in stroke-induced immunodepression syndrome (sids) and stroke-associated pneumonia (Sap). Neurochem Int. (2018) 114:42–54. doi: 10.1016/j.neuint.2018.01.002
7. Lord AS, Langefeld CD, Sekar P, Moomaw CJ, Badjatia N, Vashkevich A, et al. Infection after intracerebral hemorrhage: risk factors and association with outcomes in the ethnic/racial variations of intracerebral hemorrhage study. Stroke. (2014) 45:3535–42. doi: 10.1161/STROKEAHA.114.006435
8. Soares R, Fernandes A, Taveira I, Marreiros A, Nzwalo H. Predictors of pneumonia in patients with acute spontaneous intracerebral hemorrhage in Algarve, Southern Portugal. Clin Neurol Neurosurg. (2022) 221:107387. doi: 10.1016/j.clineuro.2022.107387
9. Prass K, Meisel C, Höflich C, Braun J, Halle E, Wolf T, et al. Stroke-induced immunodeficiency promotes spontaneous bacterial infections and is mediated by sympathetic activation reversal by Poststroke T Helper Cell Type 1-like immunostimulation. J Exp Med. (2003) 198:725–36. doi: 10.1084/jem.20021098
10. Chamorro Á, Meisel A, Planas AM, Urra X, van de Beek D, Veltkamp R. The immunology of acute stroke. Nat Rev Neurol. (2012) 8:401–10. doi: 10.1038/nrneurol.2012.98
11. Curbelo J, Luquero Bueno S, Galván-Román JM, Ortega-Gómez M, Rajas O, Fernández-Jiménez G, et al. Inflammation biomarkers in blood as mortality predictors in community-acquired pneumonia admitted patients: importance of comparison with neutrophil count percentage or neutrophil-lymphocyte ratio. PLoS ONE. (2017) 12:e0173947. doi: 10.1371/journal.pone.0173947
12. Yun S, Yi HJ, Lee DH, Sung JH. Systemic inflammation response index and systemic immune-inflammation index for predicting the prognosis of patients with aneurysmal subarachnoid hemorrhage. J Stroke Cerebrovasc Dis. (2021) 30:105861. doi: 10.1016/j.jstrokecerebrovasdis.2021.105861
13. Urbanowicz T, Michalak M, Olasińska-Wiśniewska A, Rodzki M, Witkowska A, Gasecka A, et al. Neutrophil counts, neutrophil-to-lymphocyte ratio, and systemic inflammatory response index (siri) predict mortality after off-pump coronary artery bypass surgery. Cells (2022) 11. doi: 10.3390/cells11071124
14. Cao F, Wan Y, Lei C, Zhong L, Lei H, Sun H, et al. Monocyte-to-lymphocyte ratio as a predictor of stroke-associated pneumonia: a retrospective study-based investigation. Brain Behav. (2021) 11:e02141. doi: 10.1002/brb3.2141
15. Nam KW, Kim TJ, Lee JS, Kwon HM, Lee YS, Ko SB, et al. High neutrophil-to-lymphocyte ratio predicts stroke-associated pneumonia. Stroke. (2018) 49:1886–92. doi: 10.1161/STROKEAHA.118.021228
16. Yan D, Dai C, Xu R, Huang Q, Ren W. Predictive ability of systemic inflammation response index for the risk of pneumonia in patients with acute ischemic stroke. Gerontology. (2022) 18:1–8. doi: 10.1159/000524759
17. Mu X, Li Y, He L, Guan H, Wang J, Wei Z, et al. Prognostic nomogram for adenoid cystic carcinoma in different anatomic sites. Head Neck. (2021) 43:48–59. doi: 10.1002/hed.26443
18. Hu Q, Shi Y, Hua ZY, Bao L, Li F, Wei H, et al. A prediction nomogram for acute kidney injury in very-low-birth-weight infants: a retrospective study. Front Pediatr. (2020) 8:575097. doi: 10.3389/fped.2020.575097
19. Zhang W, Fang M, Dong D, Wang X, Ke X, Zhang L, et al. Development and validation of a Ct-based radiomic nomogram for preoperative prediction of early recurrence in advanced gastric cancer. Radiother Oncol. (2020) 145:13–20. doi: 10.1016/j.radonc.2019.11.023
20. Smith CJ, Kishore AK, Vail A, Chamorro A, Garau J, Hopkins SJ, et al. Diagnosis of stroke-associated pneumonia: recommendations from the pneumonia in stroke consensus group. Stroke. (2015) 46:2335–40. doi: 10.1161/STROKEAHA.115.009617
21. Qi Q, Zhuang L, Shen Y, Geng Y, Yu S, Chen H, et al. A novel systemic inflammation response index (siri) for predicting the survival of patients with pancreatic cancer after chemotherapy. Cancer. (2016) 122:2158–67. doi: 10.1002/cncr.30057
22. Deng Q, He B, Liu X, Yue J, Ying H, Pan Y, et al. Prognostic value of pre-operative inflammatory response biomarkers in gastric cancer patients and the construction of a predictive model. J Transl Med. (2015) 13:66. doi: 10.1186/s12967-015-0409-0
23. Ingeman A, Andersen G, Hundborg HH, Svendsen ML, Johnsen SP. Processes of care and medical complications in patients with stroke. Stroke. (2011) 42:167–72. doi: 10.1161/STROKEAHA.110.599738
24. Zhang Y, Xing Z, Zhou K, Jiang S. The predictive role of systemic inflammation response index (siri) in the prognosis of stroke patients. Clin Interv Aging. (2021) 16:1997–2007. doi: 10.2147/CIA.S339221
25. Li J, Yuan Y, Liao X, Yu Z, Li H, Zheng J. Prognostic significance of admission systemic inflammation response index in patients with spontaneous intracerebral hemorrhage: a propensity score matching analysis. Front Neurol. (2021) 12:718032. doi: 10.3389/fneur.2021.718032
26. Yi HJ, Sung JH, Lee DH. Systemic inflammation response index and systemic immune-inflammation index are associated with clinical outcomes in patients treated with mechanical thrombectomy for large artery occlusion. World Neurosurg. (2021) 153:e282–e9. doi: 10.1016/j.wneu.2021.06.113
27. Zhang W, Shen Y. Platelet-to-lymphocyte ratio as a new predictive index of neurological outcomes in patients with acute intracranial hemorrhage: a retrospective study. Med Sci Monit. (2018) 24:4413–20. doi: 10.12659/MSM.910845
28. Zhang P, Li Y, Zhang H, Wang X, Dong L, Yan Z, et al. Prognostic value of the systemic inflammation response index in patients with aneurismal subarachnoid hemorrhage and a nomogram model construction. Br J Neurosurg. (2020) 12:1–7. doi: 10.1080/02688697.2020.1831438
29. Topkan E, Selek U, Kucuk A, Haksoyler V, Ozdemir Y, Sezen D, et al. Prechemoradiotherapy systemic inflammation response index stratifies stage iiib/c non-small-cell lung cancer patients into three prognostic groups: a propensity score-matching analysis. J Oncol. (2021) 2021:6688138. doi: 10.1155/2021/6688138
30. Xin Y, Zhang X, Li Y, Yang Y, Chen Y, Wang Y, et al. A systemic inflammation response index (siri)-based nomogram for predicting the recurrence of early stage hepatocellular carcinoma after radiofrequency ablation. Cardiovasc Intervent Radiol. (2022) 45:43–53. doi: 10.1007/s00270-021-02965-4
31. Zhang Y, Sun Y, Zhang Q. Prognostic value of the systemic immune-inflammation index in patients with breast cancer: a meta-analysis. Cancer Cell Int. (2020) 20:224. doi: 10.1186/s12935-020-01308-6
32. Kundi H, Balun A, Cicekcioglu H, Cetin M, Kiziltunc E, Cetin ZG, et al. The relation between platelet-to-lymphocyte ratio and pulmonary embolism severity index in acute pulmonary embolism. Heart Lung. (2015) 44:340–3. doi: 10.1016/j.hrtlng.2015.04.007
33. Ozcan Cetin EH, Cetin MS, Aras D, Topaloglu S, Temizhan A, Kisacik HL, et al. Platelet to lymphocyte ratio as a prognostic marker of in-hospital and long-term major adverse cardiovascular events in st-segment elevation myocardial infarction. Angiology. (2016) 67:336–45. doi: 10.1177/0003319715591751
34. Yang W, Liu Y. Platelet-lymphocyte ratio is a predictor of venous thromboembolism in cancer patients. Thromb Res. (2015) 136:212–5. doi: 10.1016/j.thromres.2014.11.025
35. Deng QW, Gong PY, Chen XL, Liu YK, Jiang T, Zhou F, et al. Admission blood cell counts are predictive of stroke-associated infection in acute ischemic stroke patients treated with endovascular therapy. Neurol Sci. (2021) 42:2397–409. doi: 10.1007/s10072-020-04827-2
36. Qu R, Ling Y, Zhang YH, Wei LY, Chen X, Li XM, et al. Platelet-to-lymphocyte ratio is associated with prognosis in patients with coronavirus disease-19. J Med Virol. (2020) 92:1533–41. doi: 10.1002/jmv.25767
37. Teh WH, Smith CJ, Barlas RS, Wood AD, Bettencourt-Silva JH, Clark AB, et al. Impact of stroke-associated pneumonia on mortality, length of hospitalization, and functional outcome. Acta Neurol Scand. (2018) 138:293–300. doi: 10.1111/ane.12956
38. Lindner A, Kofler M, Rass V, Ianosi B, Gaasch M, Schiefecker AJ, et al. Early predictors for infectious complications in patients with spontaneous intracerebral hemorrhage and their impact on outcome. Front Neurol. (2019) 10:817. doi: 10.3389/fneur.2019.00817
39. Huang L, Zhang R, Ji J, Long F, Wang Y, Lu J, et al. Hypersensitive C-reactive protein-albumin ratio is associated with stroke-associated pneumonia and early clinical outcomes in patients with acute ischemic stroke. Brain Behav. (2022) 12:e2675. doi: 10.1002/brb3.2675
40. Morotti A, Marini S, Jessel MJ, Schwab K, Kourkoulis C, Ayres AM, et al. Lymphopenia, infectious complications, and outcome in spontaneous intracerebral hemorrhage. Neurocrit Care. (2017) 26:160–6. doi: 10.1007/s12028-016-0367-2
41. Harms H, Prass K, Meisel C, Klehmet J, Rogge W, Drenckhahn C, et al. Preventive antibacterial therapy in acute ischemic stroke: a randomized controlled trial. PLoS ONE. (2008) 3:e2158. doi: 10.1371/journal.pone.0002158
42. Schwarz S, Al-Shajlawi F, Sick C, Meairs S, Hennerici MG. Effects of prophylactic antibiotic therapy with mezlocillin plus sulbactam on the incidence and height of fever after severe acute ischemic stroke: the mannheim infection in stroke study (Miss). Stroke. (2008) 39:1220–7. doi: 10.1161/STROKEAHA.107.499533
43. Shi K, Wood K, Shi FD, Wang X, Liu Q. Stroke-induced immunosuppression and poststroke infection. Stroke Vasc Neurol. (2018) 3:34–41. doi: 10.1136/svn-2017-000123
44. Del Bigio MR, Yan HJ, Buist R, Peeling J. Experimental intracerebral hemorrhage in rats. magnetic resonance imaging and histopathological correlates. Stroke. (1996) 27:2312–9. doi: 10.1161/01.STR.27.12.2312
45. Gong C, Hoff JT, Keep RF. Acute inflammatory reaction following experimental intracerebral hemorrhage in rat. Brain Res. (2000) 871:57–65. doi: 10.1016/S0006-8993(00)02427-6
46. Castillo J, Dávalos A, Alvarez-Sabín J, Pumar JM, Leira R, Silva Y, et al. Molecular signatures of brain injury after intracerebral hemorrhage. Neurology. (2002) 58:624–9. doi: 10.1212/WNL.58.4.624
Keywords: spontaneous intracerebral hemorrhage, pneumonia, nomogram, inflammatory response biomarkers, systemic inflammation response index, platelet/lymphocyte ratio
Citation: Yu T, Liu H, Liu Y and Jiang J (2023) Inflammatory response biomarkers nomogram for predicting pneumonia in patients with spontaneous intracerebral hemorrhage. Front. Neurol. 13:1084616. doi: 10.3389/fneur.2022.1084616
Received: 30 October 2022; Accepted: 01 December 2022;
Published: 12 January 2023.
Edited by:
Cheong-Meng Chong, University of Macau, ChinaReviewed by:
Wei Yue, Tianjin Huanhu Hospital, ChinaCopyright © 2023 Yu, Liu, Liu and Jiang. This is an open-access article distributed under the terms of the Creative Commons Attribution License (CC BY). The use, distribution or reproduction in other forums is permitted, provided the original author(s) and the copyright owner(s) are credited and that the original publication in this journal is cited, in accordance with accepted academic practice. No use, distribution or reproduction is permitted which does not comply with these terms.
*Correspondence: Jianxin Jiang, amp4ODMwODI5QDE2My5jb20=; Ying Liu,
MTM5NTI2MTg4MDFAMTYzLmNvbQ==
Disclaimer: All claims expressed in this article are solely those of the authors and do not necessarily represent those of their affiliated organizations, or those of the publisher, the editors and the reviewers. Any product that may be evaluated in this article or claim that may be made by its manufacturer is not guaranteed or endorsed by the publisher.
Research integrity at Frontiers
Learn more about the work of our research integrity team to safeguard the quality of each article we publish.