- Intensive Care Unit, The Second People's Hospital, Hefei, China
Objective: Poststroke cognitive impairment (PSCI) is a serious complication of stroke. The neutrophil-to-lymphocyte ratio (NLR) is a marker of peripheral inflammation. The relationship between the NLR and PSCI is far from well studied, and the thesis of this study was to assess the predictive value of the NLR in patients with PSCI, and establish and verify the corresponding prediction model based on this relationship.
Methods: A total of 367 stroke patients were included in this study. Neutrophils, lymphocytes, and NLRs were measured at baseline, and clinical and neuropsychological assessments were conducted 3 months after stroke. The National Institutes of Health Scale (NIHSS) was used to assess the severity of stroke. A Chinese version of the Mini Mental State Examination (MMSE) was used for the assessment of cognitive function.
Results: After three months of follow-up, 87 (23.7%) patients were diagnosed with PSCI. The NLR was significantly higher in PSCI patients than in non-PSCI patients (P < 0.001). Patient age, sex, body mass index, NIHSS scores, and high-density lipoprotein levels also differed in the univariate analysis. In the logistic regression analysis, the NLR was an independent risk factor associated with the patients with PSCI after adjustment for potential confounders (OR = 1.67, 95%CI: 1.21–2.29, P = 0.002). The nomogram based on patient sex, age, NIHSS score, and NLR had good predictive power with an AUC of 0.807. In the validation group, the AUC was 0.816.
Conclusion: An increased NLR at admission is associated with PSCI, and the model built with NLR as one of the predictors can increase prognostic information for the early detection of PSCI.
Introduction
Stroke is the second most important cause of declining acquired cognitive ability and dementia worldwide, with a high rate of disability (1–3). The incidence of poststroke cognitive impairment (PSCI) varies from 17–92% per year (4–6). As people pay more attention to disease and health, cognitive and psychological hazards are receiving increasing attention. Therefore, the recognition and early diagnosis of PSCI are of great significance for the functional recovery of stroke patients.
The existing body of research on PSCI has been reported, including white matter changes, brain microbleeds, silent infarcts, and lacunes (7–9). In addition, age, years of education, and National Institutes of Health Scale (NIHSS) scores were also reported (10–13). Neurodegenerative and vascular mechanisms can cause significant increases in inflammatory markers, including C-reactive protein, IL-1, IL-18, IL-6, and TNF-α, which may be the reason for PSCI (14, 15). Extensive studies have also shown that inflammatory pathophysiology plays a crucial role in regulating cognitive impairment (16–18). However, as an inflammatory marker, studies on the effects of the neutrophil-to-lymphocyte ratio (NLR) on PSCI are limited (19).
The NLR is a peripheral inflammatory marker that is freely received from circulation (10). Recently, the NLR has become a research hotspot for various diseases, such as cardiovascular diseases (20, 21), cancer (22, 23), and some inflammatory diseases (24). Existing studies have also have recognized the critical role played by the NLR in cognitive impairment and dementia. Studies have illustrated that the NLR has high predictive value in patients with Alzheimer's disease, and is also associated with cognitive dysfunction after carotid endarterectomy (25, 26). To date, the attention paid to the NLR and PSCI has been far from sufficient. The NLR, as an inflammatory marker, is associated with cognitive impairment (25, 26). There are few studies on the association between the NLR and PSCI, except for the study by Minwoo Lee et al. (19) who found that the NLR in acute ischemic stroke was independently associated with PSCI at 3 months poststroke. Nomograms have been widely used to predict the prognosis of oncology patients, predicting patient survival based on a simple score. So far, no nomogram has been developed for PSCI. The aim of this study is to further deepen the research on the relationship between the NLR and PSCI and to build a relevant nomogram to predict the probability of stroke patients developing PSCI. This prediction model was not previously available.
Materials and Methods
Subjects
This study was approved by the Ethics Committee of the Second People's Hospital of Hefei, and conformed to the Helsinki Declaration. From January 2012 to January 2017, 367 stroke patients were admitted to the hospital within 7 days of onset. The criteria for selecting the subjects were as follows: (1) patients aged between 18 and 80 years; (2) patients with diagnoses confirmed by computerized tomography (CT) or magnetic resonance imaging (MRI) at the time of admission; (3) patients with complete information including neutrophil and lymphocyte levels; and (4) patients who completed the Chinese version of the Mini Mental State Examination (MMSE) assessment at the 3 month follow-up at hospital discharge.
The criteria for excluding the subjects were as follows: (1) patients with acute or chronic inflammatory disease; (2) patients with transient ischemic attack; (3) patients who cannot be assessed for severe aphasia or dysarthria, or visual or auditory impairments at baseline or at follow-up (demonstrated by the exclusion of certain patients with severe stroke, high NIHSS scores); (4) patients with Parkinson's disease, severe cognitive impairment, or dementia; and (5) factors that severely affect inflammation indicators, including severe infection or antibiotic use before admission, blood disease, immunosuppression use, glucocorticoid use, or severe liver and/or kidney disease, as well as recent trauma and/or major surgery.
Clinical Measurements
Patient baseline data include sex, age, body mass index (BMI), years of education, drinking history and smoking history, hypertension history, diabetes history, hyperlipidemia history, coronary artery disease history, and etiology of stroke. The patients underwent CT or MRI examination within 72 h of admission. Inflammation is associated with many diseases, and eliminating the inflammatory response of these diseases is very important for the study. According to the classification of ICD-10, diseases are divided into 21 categories. We considered endocrine, nutritional, and metabolic diseases, diseases of the circulatory system, mental and behavioral disorders, and diseases of the nervous system in the study in order to clarify the impact of inflammation associated with patients' complications on PSCI (these are the most frequently used diseases in previous studies, and the incidence rate is relatively high).
Assessment
After admission and 3 months after discharge, trained neurologists used the MMSE to assess cognitive function. Studies have proven that the MMSE has high sensitivity and specificity for the diagnosis of cognitive impairment in the community setting and primary hospitals (23). Lower MMSE scores indicate more serious cognitive impairment. Due to the specific application of the cultural and social background of the Chinese elderly population, PSCI was defined as MMSE score ≤19 (illiterate), ≤22 (primary education), and ≤26 (secondary and above) depending on the patient's years of education (27, 28). The NIHSS is usually completed by two physicians independently within 8 h of admission, followed by a score comparison, with a third physician intervening to assess if there is a discrepancy. We used the NIHSS to assess the severity of stroke.
Laboratory Test
In our hospital's laboratory, the researchers were blinded to the clinical outcomes and measured neutrophil and lymphocyte counts. The NLR was defined as the absolute neutrophil count divided by the absolute lymphocyte count within 24 h of hospital admission.
Statistical Analyses
Continuous variables are expressed as the mean ± standard deviation and categorical variables are presented in terms of frequencies and percentages. Univariate analysis was performed by Student's t test (if the data did not obey a normal distribution, a Kruskal-Wallis test was used) or the chi-square test. Factors with a P < 0.05 were then analyzed by multivariate logistic regression. In multivariate logistic regression, we divided all stroke participants into PSCI and non-PSCI groups at the 3 month follow-up, adjusted for potential confounding factors, and analyzed the odds ratios (ORs) and 95% confidence intervals (CIs) for PSCI risk. To better reflect the clinical significance of changes in variables, we standardized the continuous variables in the logistic regression. The OR obtained in this way means that the probability of PSCI is several times higher/lower than before for the increase of one standard deviation unit. The variables for which we obtained a P < 0.05 by multivariate logistic regression were used as one of the predictors in the prediction model.
The correlation between the NLR and stroke severity (NIHSS score) was tested using the Pearson correlation test, and the correlation between the NLR and the possibility of PSCI occurrence was demonstrated using a continuous probability curve.
The nomogram used the ROC curve to assess the accuracy of the model's predictions, with a larger area under the curve (AUC) indicating a higher accuracy of the model. Calibration plots were usually used to assess the degree of fit between the predicted and actual scenarios; the higher the overlap between the predicted and actual curves, the closer the predicted scenario to the actual scenario.
We used internal validation to verify the stability of the model. A third of the patients were randomly selected by R as an internal validation cohort and the model was likewise assessed by ROC curves and calibration plots. All statistics were analyzed by SPSS 22.0 and R version 4.0.
Results
A total of 367 eligible patients were included in the study cohort. Among the 367 patients, 38.3% were women and 61.7% were men, with a mean age 61.8 years. The differences between the PSCI and non-PSCI patients are highlighted in Table 1. At the 3 month follow-up, 87 out of the 367 patients were diagnosed with PSCI (23.7%). Compared with the non-PSCI patients, PSCI patients were more likely to be women (32.90 vs. 57.50%) and older (60.12 vs. 67.25). They also showed significant differences in stroke severity on admission, with a mean NIHSS score of 3.94 for the PSCI patients and 2.40 for the non-PSCI patients.
The NLR, the hypothetical marker in this paper, also showed significant differences between the PSCI and non-PSCI patients. As shown in Table 1, the mean NLR for the PSCI patients was 2.73, compared to 2.14 for the non-PSCI patients. The probability of PSCI was also closely linked to the NLR. Figure 1 shows the risk probability of PSCI (95 CI%). When a patient's NLR was elevated, then the probability of that patient developing PSCI was subsequently elevated. In addition to this, the NLR showed a strong correlation with stroke severity, as shown in Figure 2, where patients' NLR on admission was correlated with their NIHSS score (P < 0.001, r = 0.24, 95% CI: 0.14–0.34).
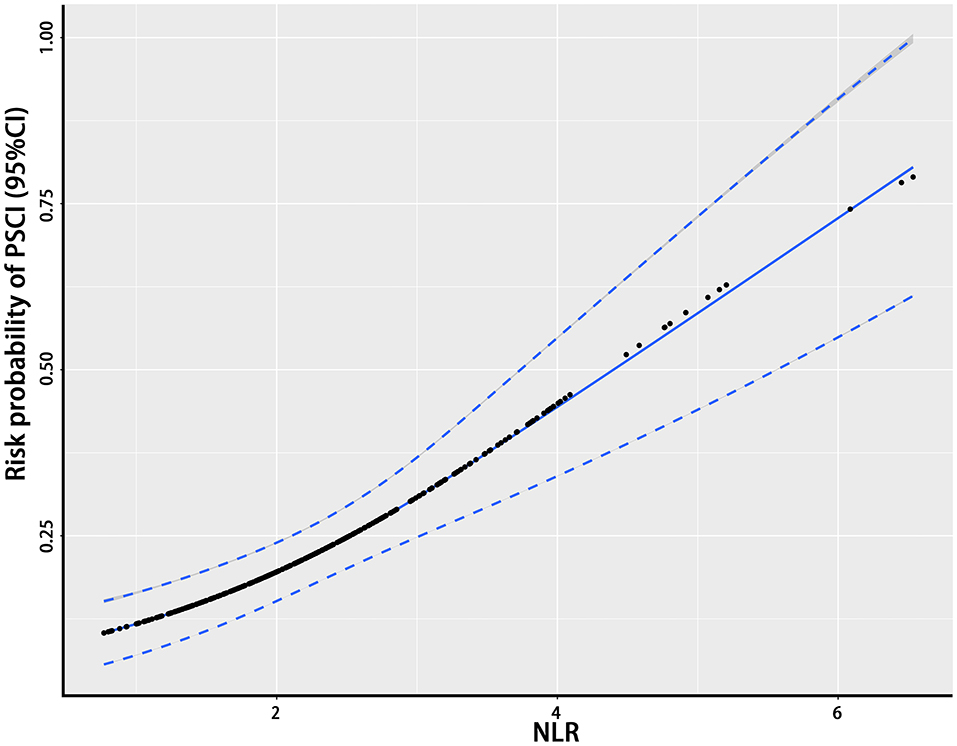
Figure 1. The relationship between the NLR and the probability of PSCI. This figure shows the association between the NLR and the probability of PSCI. The black dots represent the probability of the occurrence of PSCI corresponding to the given NLR level and the blue curves on either side are their 95% confidence intervals. NLR, neutrophil-to-lymphocyte ratio; PSCI, poststroke cognitive impairment.
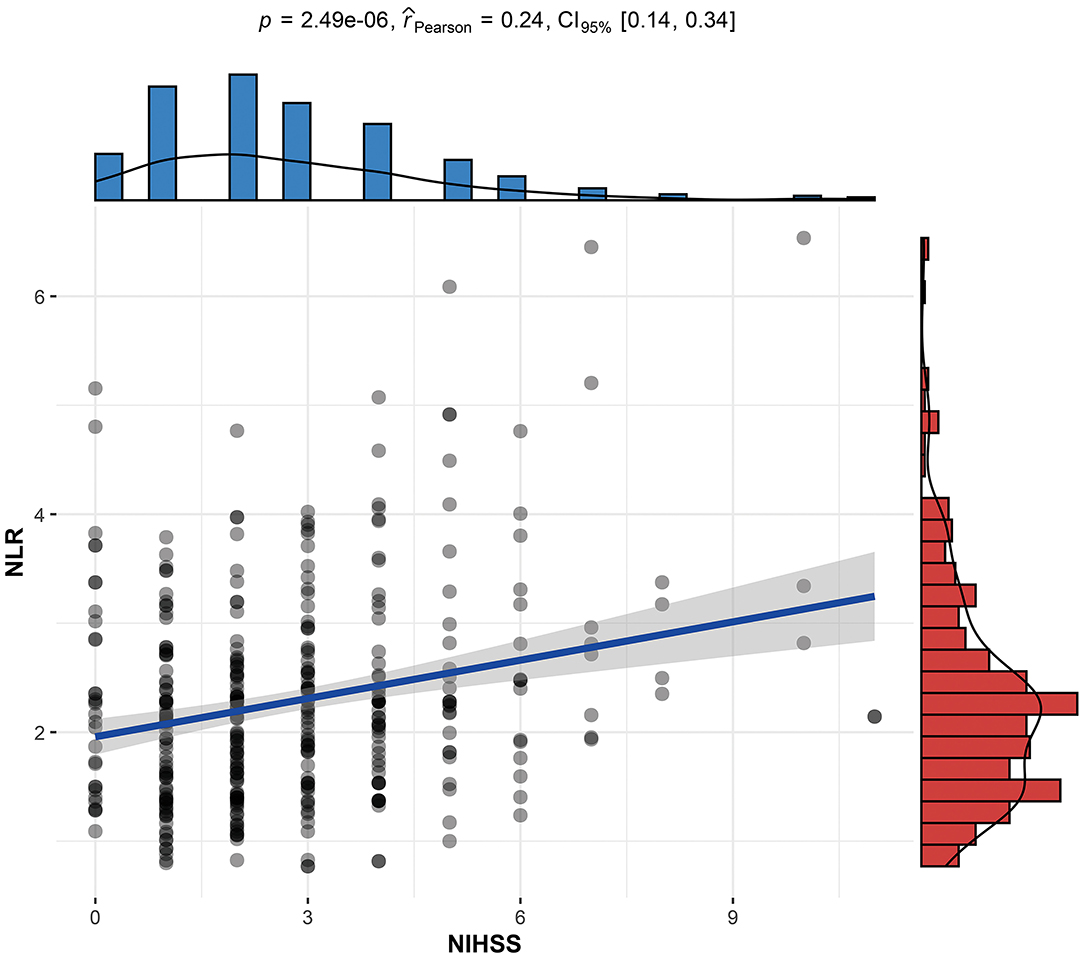
Figure 2. Linear correlation between the stroke patient's NLR and NIHSS score. NLR, neutrophil-to-lymphocyte ratio; NIHSS, National Institutes of Health Stroke Scale on admission; PSCI, poststroke cognitive impairment.
In the logistic regression analysis, after adjusting for multiple confounding factors (age, sex, high-density lipoprotein level, NIHSS score, body mass index), higher NLR values were independently associated with the occurrence of PSCI at 3 months with an adjusted OR of 1.67 (95%CI: 1.21–2.29, P = 0.002). Additionally, patients' age (OR = 1.06, 95%CI: 1.03–1.10, P = 0.001), sex (male as the reference, OR = 2.94, 95%CI: 1.58–5.45, P = 0.001), and NIHSS score at admission (OR = 1.42, 95%CI: 1.22–1.64, P < 0.001) remained independently associated with the occurrence of PSCI (Table 2). MMSE at baseline may have an impact on cognitive function at the 3 month follow-up, so we independently explored its impact on the final outcome. As shown in Table 1, there was no difference in MMSE at baseline between the two groups of patients in the univariate analysis. As shown in Table 2, in model 2 we performed logistic regression analysis on the variables that were statistically different in the univariate analysis described above. To further control the MMSE, we included it in model 1. As shown in model 1, the MMSE did not show a statistical difference in the logistic regression analysis (P: 0.769, OR: 1.013, 95% CI: 0.930–1.103; Z-MMSE: P: 0.769,OR: 1.05,95%CI: 0.77–1.42). And, the OR of NLR changed from 1.63 to 1.60, showing a limited effect of baseline MMSE on NLR. After controlling for MMSE, NLR remained strongly associated with PSCI (P: 0.003, 95%CI: 1.18–2.25; Z-NLR: P: 0.003, 95%CI: 1.17–2.18). Hence, baseline MMSE was not included in the nomogram. Finally, the four predictors screened by the regression analysis were incorporated into the nomogram to predict the probability of PSCI occurrence. Figure 3 shows the nomogram, which was constructed by incorporating the four predictors. As shown, each predictor has its own corresponding score, and the sum of the scores of these factors corresponds to the probability of PSCI occurring in this case.
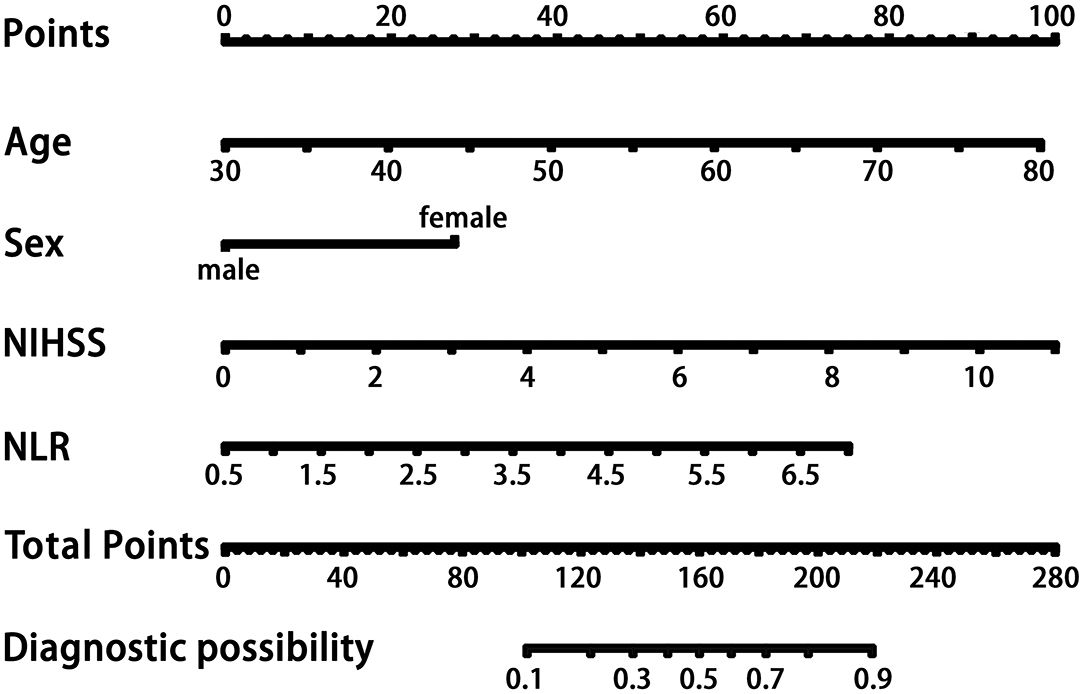
Figure 3. The nomogram for patients with PSCI. Each variable corresponds to its own score, and the sum of these scores corresponds to the probability of PSCI occurring on the probability axis. NLR, neutrophil-to-lymphocyte ratio; NIHSS, National Institutes of Health Stroke Scale on admission; PSCI, poststroke cognitive impairment.
We assessed the accuracy of the nomogram by ROC curves. In the development group, the AUC was 0.807, demonstrating good predictive power. Internal validation was used to assess the stability of the model. In the internal validation, the AUC of the model was 0.816, which was close to that of the development group, again demonstrating good predictive power (Figure 4). Except for the AUC, calibration plots are one of the tools that are commonly used to assess the consistency of a predicted situation against an actual situation. As shown in Figure 5, the predicted curves showed the best agreement with the actual curves in both the development and validation groups.
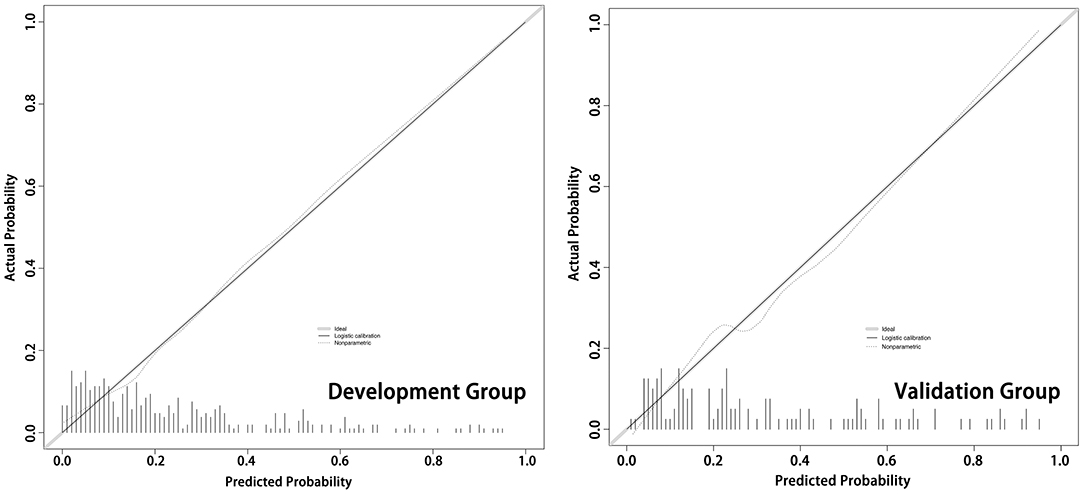
Figure 5. The calibration plots of the development cohort and validation cohort. As shown in the calibration plots, the predicted probability of the model is plotted on the x-axis; the actual overall event is plotted on the y-axis. The higher the degree of overlap between the predicted curve and the diagonal (actual situation), the more accurate the model.
Discussion
Patient sex, age, and the NIHSS score were considered to be associated with the occurrence of PSCI in previous studies (29–31). Few studies have considered using the NLR as an easily available peripheral inflammatory factor, and its relationship to PSCI (19). Our results suggest that higher NLR levels at admission are associated with the presence of PSCI. This is the first study to build a nomogram on the PSCI, filling a gap in this area.
In a review by Sun et al., demographically, age and literacy were associated with the risk of cognitive impairment after stroke (29). Age is a risk factor not only for stroke, but also for cognitive decline. There is evidence that the prevalence of cognitive decline after stroke increases exponentially with age after the age of 65 years (1). In a study by Chen et al., female sex was identified as a risk factor for cognitive decline (30). Sharma et al. found a strong correlation between the occurrence of PSCI and the patient's NIHSS (31).
A considerable amount of literature has already reported that cognitive impairment or dementia is related to inflammatory factors (14, 32–34). Diniz et al. found that increased expression of sTNFR1, which represents the TNF-α signaling system, is associated with a higher risk of progression from MCI to AD (35). Patanella AK et al. investigated the differential impact of BDNF and IL-6 on cognitive impairment and showed that low BDNF and high IL-6 levels are associated with poor performance in cognitive tasks in patients with relapsing-remitting multiple sclerosis. Although these inflammatory factors show significant correlations with cognitive function, these markers are uncommon in clinical work and often require additional testing to obtain them. Therefore, the NLR was selected as a target marker for this study as a readily available and clinically used peripheral inflammatory factor. In our study, we found that the NLR was positively correlated with stroke severity at admission and that high NLR levels predicted a higher likelihood of PSCI.
The NLR reflects the balance between neutrophil and lymphocyte levels and is a widely available, easily derived, and reproducible marker of inflammation. In accordance with the present results, previous studies have emphasized that the NLR is associated with atherosclerosis and is an independent risk factor for ischemic stroke (36, 37). Studies over the past decade have provided valuable information about the NLR and cognitive impairment or dementia. Hadi et al. found that the NLR at admission is associated with cognitive dysfunction after carotid endarterectomy (26). Mehmet et al. found that the NLR has a high sensitivity, specificity, and predictive value for AD patients (25). Due to the multifactorial pathophysiology of poststroke cognitive decline, the precise mechanisms remain unclear. Vascular mechanisms, which include lesions on small cerebral vessels, lead to cerebral microbleeds (CMBs), and white matter lesions may be the pathogenesis of PSCI (29, 38). Extensive research has shown that when the brain develops microbleeds, it has an inflammatory response to the hemorrhage (39–42). Inflammatory cytokines, including IL-6, IL-10, CRP, MCP-1, ICAM-1, and TNF-α, play an important role in the development of poststroke cognitive decline (14, 15, 43). One study reported that the NLR is positively correlated with inflammatory cytokines (44). Another study, a correlation analysis showed that hsCRP was positively correlated with the NLR in patients with Behcet disease (24). As a marker of inflammation, anti-inflammatory treatment can reduce neuroinflammation, AD-like pathology, and cognitive deficits (45).
Animal models with disturbed endothelial dysfunction have demonstrated that blood-brain barrier (BBB) breakdown either initiates or exacerbates neurodegeneration, which could lead to PSCI (46). Destruction of the BBB can disrupt the flow of oxygen and nutrients to the brain and allow toxins from around the brain to enter the central nervous system (47, 48). The NLR is a marker of endothelial dysfunction (49), and the accumulation of neutrophils may cause chronic BBB damage (50). Neutrophils have been shown to be an important source of matrix metalloprotein-9 that can breakdown the BBB or be absorbed by endothelial cells and act on the basement membrane (51). In a rat model of cerebral ischemia, treatment to prevent neutrophil infiltration reduces the release of matrix metalloprotein-9 in the brain (52). Moreover, the infiltration of neutrophils in the central nervous system can induce a cascade of neuroinflammatory reactions, aggravating cognitive decline. This shows that the NLR is closely related to the development of PSCI.
There are still several limitations in this study. First, this was a retrospective study and is subject to recall bias. During the follow-up process, selection bias was an inevitable result as a proportion of patients did not meet the inclusion criteria and were excluded. Second, this study used a single center's internal validation to verify the accuracy of the model, and whether the model can be generalized to other levels of medical centers is still not well validated. Despite its limitations, the highlight of this study is the creation of a relevant nomogram for PSCI patients by using a sample of a certain amount of data, which has not been done before. The indicators that were selected are also clinically accessible, so that clinicians can easily determine the likelihood of PSCI in a particular stroke patient and take appropriate precautions for it.
Conclusion
This study suggests that a higher NLR may be associated with the occurrence of PSCI, and the constructed nomogram can help predict the probability of PSCI in stroke patients.
Data Availability Statement
The raw data supporting the conclusions of this article will be made available by the authors, without undue reservation.
Ethics Statement
The studies involving human participants were reviewed and approved by the Ethics Committee of the second people's Hospital of Hefei. Written informed consent for participation was not required for this study in accordance with the national legislation and the institutional requirements.
Author Contributions
LY designed this study. FZ participated in the design and completed the statistical part of this article. CC, XJ, ML, and YW participated in the collection of data and the writing of the article. All authors contributed to the article and approved the submitted version.
Conflict of Interest
The authors declare that the research was conducted in the absence of any commercial or financial relationships that could be construed as a potential conflict of interest.
Publisher's Note
All claims expressed in this article are solely those of the authors and do not necessarily represent those of their affiliated organizations, or those of the publisher, the editors and the reviewers. Any product that may be evaluated in this article, or claim that may be made by its manufacturer, is not guaranteed or endorsed by the publisher.
Acknowledgments
We would like to thank all the authors who participated in this research.
References
1. Iadecola C, Duering M, Hachinski V, Joutel A, Pendlebury ST, Schneider JA, et al. Vascular Cognitive Impairment and Dementia: JACC Scientific Expert Panel. J Am Coll Cardiol. (2019) 73:3326–44. doi: 10.1016/j.jacc.2019.04.034
2. Jorm AF, Jolley D. The incidence of dementia: a meta-analysis. Neurology. (1998) 51:728–33. doi: 10.1212/WNL.51.3.728
3. Lobo A, Launer LJ, Fratiglioni L, Andersen K, Di Carlo A, Breteler MM, et al. Prevalence of dementia and major subtypes in Europe: A collaborative study of population-based cohorts. Neurology. (2000) 54:S4–9.
4. Qu Y, Zhuo L, Li N, Hu Y, Chen W, Zhou Y, et al. Prevalence of post-stroke cognitive impairment in china: a community-based, cross-sectional study. PLoS ONE. (2015) 10:e0122864. doi: 10.1371/journal.pone.0122864
5. Casolla B, Caparros F, Cordonnier C, Bombois S, Henon H, Bordet R, et al. Biological and imaging predictors of cognitive impairment after stroke: a systematic review. J Neurol. (2018) 266:2593–604. doi: 10.1007/s00415-018-9089-z
6. Barba R, Martinez-Espinosa S, Rodriguez-Garcia E, Pondal M, Vivancos J, Del Ser T. Poststroke dementia: clinical features and risk factors. Stroke. (2000) 31:1494–501. doi: 10.1161/01.STR.31.7.1494
7. Zhao H, Cheng J, Jiang J, Zuo L, Zhu W, Wen W, et al. Geometric microstructural damage of white matter with functional compensation in post-stroke. Neuropsychologia. (2021) 160:107980. doi: 10.1016/j.neuropsychologia.2021.107980
8. Chi NF, Hu HH, Chan L, Wang CY, Chao SP, Huang LK, et al. Impaired cerebral autoregulation is associated with poststroke cognitive impairment. Ann Clin Trans Neurol. (2020) 7:1092–102. doi: 10.1002/acn3.51075
9. Kandiah N, Chander RJ, Lin X, Ng A, Poh YY, Cheong CY, et al. Cognitive impairment after mild stroke: development and validation of the SIGNAL2 risk score. J Alzheimer's disease: JAD. (2016) 49:1169–77. doi: 10.3233/JAD-150736
10. Pohjasvaara TI, Jokinen H, Ylikoski R, Kalska H, Mantyla R, Kaste M, et al. White matter lesions are related to impaired instrumental activities of daily living poststroke. J Stroke Cerebrovasc Dis. (2007) 16:251–8. doi: 10.1016/j.jstrokecerebrovasdis.2007.08.001
11. Wang Z, Wong A, Liu W, Yang J, Chu WC, Au L, et al. Cerebral microbleeds and cognitive function in ischemic stroke or transient ischemic attack patients. Dement Geriatr Cogn Disord. (2015) 40:130–6. doi: 10.1159/000379744
12. Vermeer SE, Prins ND, Tom DH, Albert H, Koudstaal PJ, Breteler MMB. Silent brain infarcts and the risk of dementia and cognitive decline. N Engl J Med. (2010) 348:1215–22. doi: 10.1056/NEJMoa022066
13. Gottesman RF, Hillis AE. Predictors and assessment of cognitive dysfunction resulting from ischaemic stroke. Lancet Neurol. (2010) 9:895–905. doi: 10.1016/S1474-4422(10)70164-2
14. Rothenburg LS, Herrmann N, Swardfager W, Black SE, Tennen G, Kiss A, et al. The relationship between inflammatory markers and post stroke cognitive impairment. J Geriatr Psychiatry Neurol. (2010) 23:199–205. doi: 10.1177/0891988710373598
15. Saleem M, Herrmann N, Swardfager W, Eisen R, Lanctôt KL. Inflammatory markers in mild cognitive impairment: a meta-analysis. J Alzheimers Dis. (2015) 47:669–79. doi: 10.3233/JAD-150042
16. Shen XN, Niu LD, Wang YJ, Cao XP, Liu Q, Tan L, et al. Inflammatory markers in Alzheimer's disease and mild cognitive impairment: a meta-analysis and systematic review of 170 studies. J Neurol Neurosurg Psychiatry. (2019) 90:590–8. doi: 10.1136/jnnp-2018-319148
17. Heneka MT, Carson MJ, El Khoury J, Landreth GE, Brosseron F, Feinstein DL, et al. Neuroinflammation in Alzheimer's disease. Lancet Neurol. (2015) 14:388–405. doi: 10.1016/S1474-4422(15)70016-5
18. Darweesh SKL, Wolters FJ, Ikram MA, de Wolf F, Bos D, Hofman A. Inflammatory markers and the risk of dementia and Alzheimer's disease: a meta-analysis. Alzheimer's Dementia. (2018) 14:1450–9. doi: 10.1016/j.jalz.2018.02.014
19. Lee M, Lim JS, Kim CH, Lee SH, Kim Y, Hun Lee J, et al. High neutrophil-lymphocyte ratio predicts post-stroke cognitive impairment in acute ischemic stroke patients. Front Neurol. (2021) 12:693318. doi: 10.3389/fneur.2021.693318
20. Balta S, Celik T, Mikhailidis DP, Ozturk C, Demirkol S, Aparci M, et al. The relation between atherosclerosis and the neutrophil-lymphocyte ratio. Clin Appl Thromb Hemost. (2016) 22:1076029615569568. doi: 10.1177/1076029616639701
21. Mozos I, Malainer C, Horbanczuk J, Gug C, Stoian D, Luca CT, et al. Inflammatory markers for arterial stiffness in cardiovascular diseases. Front Immunol. (2017) 8:1058. doi: 10.3389/fimmu.2017.01058
22. Toya Y, Endo M, Nakamura S, Akasaka R, Yanai S, Kawasaki K, et al. Long-term outcomes and prognostic factors with non-curative endoscopic submucosal dissection for gastric cancer in elderly patients aged >/= 75 years. Gastric Cancer. (2019) 22:838–44. doi: 10.1007/s10120-018-00913-9
23. Zaragoza J, Caille A, Beneton N, Bens G, Christiann F, Maillard H, et al. High neutrophil to lymphocyte ratio measured before starting ipilimumab treatment is associated with reduced overall survival in patients with melanoma. Br J Dermatol. (2016) 174:146–51. doi: 10.1111/bjd.14155
24. Cengiz O, Sevket B, Ilknur B, Sait D, Turgay C, Turker T, et al. Neutrophil-lymphocyte ratio and carotid-intima media thickness in patients with Beh? Et disease without cardiovascular involvement. Angiol. (2015) 66:291–6. doi: 10.1177/0003319714527638
25. Kuyumcu ME, Yesil Y, Oztürk ZA, Kizilarslanoglu C, Etgül S, Halil M, et al. The evaluation of neutrophil-lymphocyte ratio in Alzheimer's disease. Dementia Geriat Cogn Diso. (2012) 34:69–74. doi: 10.1159/000341583
26. Halazun HJ, Mergeche JL, Mallon KA, Connolly ES, Heyer EJ. Neutrophil-lymphocyte ratio as a predictor of cognitive dysfunction in carotid endarterectomy patients. J Vasc Surg. (2014) 59:768–73. doi: 10.1016/j.jvs.2013.08.095
28. Mitchell AJ, A. meta-analysis of the accuracy of the mini-mental state examination in the detection of dementia and mild cognitive impairment. J Psychiatr Res. (2009) 43:411–31. doi: 10.1016/j.jpsychires.2008.04.014
29. Sun JH, Tan L, Yu JT. Post-stroke cognitive impairment: epidemiology, mechanisms and management. Ann Transl Med. (2014) 2:80. doi: 10.3978/j.issn.2305-5839.2014.08.05
30. Chen X, Duan L, Han Y, Tian L, Dai Q, Wang S, et al. Predictors for vascular cognitive impairment in stroke patients. BMC Neurol. (2016) 16:115. doi: 10.1186/s12883-016-0638-8
31. Sharma R, Mallick D, Llinas RH, Marsh EB. Early post-stroke cognition: in-hospital predictors and the association with functional outcome. Front Neurol. (2020) 11:613607. doi: 10.3389/fneur.2020.613607
32. Granic I, Dolga AM, Nijholt IM, van Dijk G, Eisel UL. Inflammation and NF-kappaB in Alzheimer's disease and diabetes. Journal of Alzheimer's Dis. (2009) 16:809–21. doi: 10.3233/JAD-2009-0976
33. Casserly I, Topol EJ. Convergence of atherosclerosis and Alzheimer's disease: inflammation, cholesterol, and misfolded proteins. Lancet. (2004) 363:1139–46. doi: 10.1016/S0140-6736(04)15900-X
34. Yu D, Corbett B, Yan Y, Zhang GX, Reinhart P, Cho SJ, et al. Early cerebrovascular inflammation in a transgenic mouse model of Alzheimer's disease. Neurobiol Aging. (2012) 33:2942–7. doi: 10.1016/j.neurobiolaging.2012.02.023
35. Diniz BS, Teixeira AL, Ojopi EB, Talib LL, Mendonca VA, Gattaz WF, et al. Higher serum sTNFR1 level predicts conversion from mild cognitive impairment to Alzheimer's disease. J Alzheimer's Dis. (2010) 22:1305–11. doi: 10.3233/JAD-2010-100921
36. Nam KW, Kwon HM, Jeong HY, Park JH, Kim SH, Jeong SM. High neutrophil to lymphocyte ratios predict intracranial atherosclerosis in a healthy population. Atherosclerosis. (2018) 269:117–21. doi: 10.1016/j.atherosclerosis.2017.12.035
37. Suh B, Shin DW, Kwon HM, Yun JM, Yang HK, Ahn E, et al. Elevated neutrophil to lymphocyte ratio and ischemic stroke risk in generally healthy adults. PLoS ONE. (2017) 12:e0183706. doi: 10.1371/journal.pone.0183706
38. Wardlaw JM, Smith C, Dichgans M. Small vessel disease: mechanisms and clinical implications. Lancet Neurol. (2019) 18:684–96. doi: 10.1016/S1474-4422(19)30079-1
39. Ahn SJ, Anrather J, Nishimura N, Schaffer CB. Diverse inflammatory response after cerebral microbleeds includes coordinated microglial migration and proliferation. Stroke. (2018) 49:1719–26. doi: 10.1161/STROKEAHA.117.020461
40. Jung S, Aliberti J, Graemmel P, Sunshine MJ, Kreutzberg GW, Sher A, et al. Analysis of fractalkine receptor CX_3CR1 function by targeted deletion and green fluorescent protein reporter gene insertion. Molec Cell Biol. (2000) 20:4106–14. doi: 10.1128/MCB.20.11.4106-4114.2000
41. Pologruto TA, Sabatini BL, Svoboda K. ScanImage: flexible software for operating laser scanning microscopes. Biomed Eng Online. (2003) 2:1–9. doi: 10.1186/1475-925X-2-13
42. Low A, Mak E, Rowe JB, Markus HS, O'Brien JT. Inflammation and cerebral small vessel disease: a systematic review. Ageing Res Rev. (2019) 53:100916. doi: 10.1016/j.arr.2019.100916
43. Thiel A, Cechetto DF, Heiss WD, Hachinski V, Whitehead SN. Amyloid burden, neuroinflammation, and links to cognitive decline after ischemic stroke. Stroke. (2014) 45:2825–9. doi: 10.1161/STROKEAHA.114.004285
44. Sen N, Afsar B, Ozcan F, Buyukkaya E, Isleyen A, Akcay AB, et al. The neutrophil to lymphocyte ratio was associated with impaired myocardial perfusion and long term adverse outcome in patients with ST-elevated myocardial infarction undergoing primary coronary intervention. Atherosclerosis. (2013) 228:203–10. doi: 10.1016/j.atherosclerosis.2013.02.017
45. Grilli M, Goffi F, Memo M, Spano P. Interleukin-1beta and glutamate activate the NF-kappaB/Rel binding site from the regulatory region of the amyloid precursor protein gene in primary neuronal cultures. J Biol Chem. (1996) 271:15002–7. doi: 10.1074/jbc.271.25.15002
46. Bell RD, Zlokovic BV. Neurovascular mechanisms and blood-brain barrier disorder in Alzheimer's disease. Acta Neuropathol. (2009) 118:103–13. doi: 10.1007/s00401-009-0522-3
47. Bowman GL, Dodge H, Frei B, Calabrese C, Oken BS, Kaye JA, et al. Ascorbic acid and rates of cognitive decline in Alzheimer's disease. J Alzheimer's Dis. (2009) 16:93–8. doi: 10.3233/JAD-2009-0923
48. Kisler K, Nelson AR, Montagne A, Zlokovic BV. Cerebral blood flow regulation and neurovascular dysfunction in Alzheimer disease. Nat Rev Neurosci. (2017) 18:419–34. doi: 10.1038/nrn.2017.48
49. Balta S, Demirkol S, Celik T, Kucuk U, Unlu M, Arslan Z, et al. Association between coronary artery ectasia and neutrophil-lymphocyte ratio. Angiology. (2013) 64:627–32. doi: 10.1177/0003319713480424
50. Abdul Muneer PM, Alikunju S, Szlachetka AM, Haorah J. Inhibitory effects of alcohol on glucose transport across the blood-brain barrier leads to neurodegeneration: preventive role of acetyl-L: -carnitine. Psychopharmacology. (2011) 214:707–18. doi: 10.1007/s00213-010-2076-4
51. Jickling GC, Dazhi L, Boryana S, Ander BP, Xinhua Z, Aigang L, et al. Hemorrhagic transformation after ischemic stroke in animals and humans. J Cereb Blood Flow Metab. (2014) 34:185–99. doi: 10.1038/jcbfm.2013.203
52. Justicia C, Panes J, Sole S, Cervera A, Deulofeu R, Chamorro A, et al. Neutrophil infiltration increases matrix metalloproteinase-9 in the ischemic brain after occlusion/reperfusion of the middle cerebral artery in rats. J Cereb Blood Flow Metab. (2003) 23:1430–40. doi: 10.1097/01.WCB.0000090680.07515.C8
Keywords: cognitive impairment, neutrophil to lymphocyte ratio (NLR), poststroke cognitive impairment, prognosis, nomogram
Citation: Zha F, Zhao J, Chen C, Ji X, Li M, Wu Y and Yao L (2022) A High Neutrophil-to-Lymphocyte Ratio Predicts Higher Risk of Poststroke Cognitive Impairment: Development and Validation of a Clinical Prediction Model. Front. Neurol. 12:755011. doi: 10.3389/fneur.2021.755011
Received: 07 August 2021; Accepted: 08 December 2021;
Published: 17 January 2022.
Edited by:
Johannes Boltze, University of Warwick, United KingdomReviewed by:
Chenlu Gao, Brigham and Women's Hospital and Harvard Medical School, United StatesPeng Li, Harvard Medical School, United States
Copyright © 2022 Zha, Zhao, Chen, Ji, Li, Wu and Yao. This is an open-access article distributed under the terms of the Creative Commons Attribution License (CC BY). The use, distribution or reproduction in other forums is permitted, provided the original author(s) and the copyright owner(s) are credited and that the original publication in this journal is cited, in accordance with accepted academic practice. No use, distribution or reproduction is permitted which does not comply with these terms.
*Correspondence: Li Yao, ylirn168@163.com
†These authors share first authorship