- 1Physiotherapy Center Kiné, Cremona, Italy
- 2Neurology Unit, University Hospital of Verona, Verona, Italy
- 3Department of Medico-Surgical Sciences and Biotechnologies, Sapienza University of Rome Polo Pontino, Latina, Italy
- 4Unit of Neuro-Rehabilitation and Rehabilitation Medicine, Istituto di Ricovero e Cura a Carattere Scientifico (IRCCS) Casa Sollievo della Sofferenza, San Giovanni Rotondo, Italy
- 5Neurorehabilitation Unit, Department of Rehabilitation, HABILITA Zingonia, Ciserano, Italy
Introduction: Disturbances of balance control are common after stroke, affecting the quality of gait and increasing the risk of falls. Because balance and gait disorders may persist also in the chronic stage, reducing individual independence and participation, they represent primary goals of neurorehabilitation programs. For this purpose, in recent years, numerous technological devices have been developed, among which one of the most widespread is the Lokomat®, an actuated exoskeleton that guide the patient's limbs, simulating a symmetrical bilateral gait. Preliminary evidence suggests that beyond gait parameters, robotic assisted gait training may also improve balance. Therefore, the aim of this systematic review was to summarize evidence about the effectiveness of Lokomat® in improving balance in stroke patients.
Methods: Randomized controlled trials published between January 1989 and August 2020, comparing Lokomat® training to conventional therapy for stroke patients, were retrieved from seven electronic databases. Balance, assessed by means of validated clinical scales, was considered as outcome measure. The Physiotherapy Evidence Database (PEDro) scale was used to evaluate the methodological quality of the studies. The study protocol was registered on PROSPERO (no. CRD42020197531).
Results: After the removal of the duplicates, according to the inclusion criteria, 13 studies were selected, involving 445 subacute or chronic stroke patients. Eleven papers contributed to three meta-analyses. Favorable results for recovery of balance in stroke survivors treated with Lokomat® were shown using Timed Up and Go (pooled mean difference = −3.40, 95% CI −4.35 to −2.44; p < 0.00001) and Rivermead Mobility Index as outcome measures (pooled mean difference = 0.40, 95% CI 0.26–0.55; p < 0.00001). Inconclusive results were found when balance was measured by means of the Berg Balance Scale (pooled mean difference = 0.17, 95% CI −0.26 to 0.60; p = 0.44).
Conclusions: Overall, most studies have shown beneficial effects of Lokomat® on balance recovery for stroke survivors, at least comparable to conventional physical therapy. However, due to the limited number of studies and their high heterogeneity, further research is needed to draw more solid and definitive conclusions.
Introduction
Balance control is described as a complex motor skill that depends on interactions between multiple sensorimotor processes and environmental and functional contexts that in stroke survivors can be affected individually or in combination, producing balance disorders of different severity (1). Balance is an essential component of the quality of walking (2–4), and in stroke survivors, balance impairment may determine abnormal patterns of gait characterized by decreased walking speed and stride length and spatial and temporal asymmetry (5–9) that in turn increase the risk of falling (10–14). Because balance and gait disorders may persist also in the chronic stage (15), they represent one of the main limiting factors for individual independence and participation in social activities, reducing patients' quality of life (16–18).
As it is well-established that balance control strategies can become more efficient with training and practice, improving balance and gait represents a main goal of neurorehabilitation programs to restore effective and safe mobility (19–22). Although the development of robotic devices that mechanically guide patient's limbs through the gait cycle is a relatively novel approach, in recent years, many devices were developed and have become commercially available. Among these robot machines, the Lokomat® (Hocoma AG, Volketswil, Switzerland) is an actuated exoskeleton that simulates a symmetrical bilateral gait while the individual walks more or less actively on a treadmill; this device helps the patient reproduce the different phases of the physiological gait cycle, fragmenting the joints, symmetrically balancing the load, and transmitting proprioceptive afferents. Studies have shown that the rhythmic and repetitive step pattern provided by the robotic guidance combined with the active load of the limbs and kinematic coherence promotes the plasticity of the gait pattern generator, facilitating motor schemes and neural plasticity at the spinal cord and supraspinal level (23).
Most of the previous researches evaluating the effectiveness of Lokomat® focused on the kinematic parameters of gait in patients affected by stroke (24) or spinal cord injury (25), while only a few studies focused on balance as outcome measure.
Notwithstanding the small number of studies, two systematic reviews have been published that provided preliminary evidence about the effect of robot-assisted gait training (RAGT) and reported that rehabilitative robotic strategy can improve balance for stroke patients similarly to conventional gait rehabilitation (26, 27). However, these systematic reviews and meta-analyses considered variable kinds of robotic devices including both static exoskeletons and end-effector machine that may involve different neural mechanisms in the recovery process. Although RAGT/Lokomat® was also included, no data and remark could be obtained about the effect of this robotic instrument on balance.
Moreover, it should be noted that, contrary to what happens in the clinical pharmacological field, as already discussed in the review by Iosa et al. (28), the introduction of robotic technologies in rehabilitation does not provide for preclinical efficacy studies, thus allowing the marketing of devices for which the main purpose of use is generally known, but not the effects on aspects functionally related to the main purpose.
Therefore, with the aim to increase knowledge about the specific effect of Lokomat® on improving balance control in stroke survivors, as well as to favor a more appropriate use of this robot, we systematically synthesize evidence and discuss data on this issue.
Methods
This systematic review was reported according to the Preferred Reporting Items for Systematic reviews and Meta-Analysis statement (29, 30). All the analyses were performed on previous published papers, and the study was notified to the local ethics committee, according to current legislation. The study was performed in accordance with the Declaration of Helsinki, and the overview protocol was registered on PROSPERO (no. CRD42020197531).
Eligibility Criteria
Objectives were defined according to the PICO model (Population, Intervention, Comparison, Outcome type). The population of interest was adults post-stroke (both ischemic and hemorrhagic). Interventions considered were rehabilitation treatment performed by means of the Lokomat® robotic device, with no restrictions on the setting (inpatients and outpatients). Comparison groups included other rehabilitation methods (conventional physiotherapy and/or treadmill) (see Table 2 for a description of control therapies). Outcome considered was balance control, assessed by means of standardized clinical scales (44). Only studies written in English were considered.
Exclusion criteria were study design other than randomized controlled trial (RCT), studies involving subjects suffering from neurological diseases other than stroke, comparison of rehabilitation treatment performed by means of the Lokomat® with other unconventional therapies (e.g., electrical stimulation), studies with full text not available, non-peer-reviewed articles, congress abstracts/posters.
Exoskeleton Lokomat®
The Lokomat® (Hocoma AG, Volketswil, Switzerland) is a robotic bilateral orthosis used in neurological rehabilitation to automate locomotor function. Briefly, it is composed of a body weight support system used in combination with a treadmill that replicates the lower extremity biomechanics of walking overground, sometimes associated with an augmented reality system. Lokomat® can be classified as an “exoskeletal robot.” In this kind of robot, knee and hip are driven by linear electrical motors that guide the external orthosis applied to the body, while a foot lift induces passive dorsiflexion of the ankle during the swing phase (45). This facilitates a bilateral symmetrical gait, as the individual actively tries to advance each limb while walking on the treadmill. The preprogrammed gait model corresponds to the normal kinematics of gait that includes the synchronization of the gait cycle, the coordination between the limbs and joints, and the appropriate load of the limbs (46, 47).
Outcome Measures
For this meta-analysis, according to the International Classification of Functioning, Disability and Health (ICF), we considered balance as a level of activity reflecting functional abilities and postural control as a function of body structure reflecting both orientation and stabilization of the body (48). Therefore, in the included studies, the following standardized clinical scales were considered outcome measures:
- Berg Balance Scale (BBS): the scale that evaluates the patient's ability to safely maintain balance during a series of predetermined static and dynamic functional tasks. It is a 14-item list with each item consisting of a 5-point ordinal scale ranging from 0 (minimum performance) to 4 (best performance) with a maximum total score of 56 (≤ 45: need for travel assistance; 41–44: low risk of falling; 21–40: high risk; 0–20: very high risk of falling). It does not include the assessment of gait (49).
- Timed Up and Go (TUG): the test that determines fall risk and measures the progress of balance, sit to stand, and walking. The scale measures the time to get up from a chair, walk 3 m, turn around, go back to the chair, and sit down again. Subjects with a score ≥14 s are considered at risk of falling; the higher the score, the greater the risk (50).
- Rivermead Mobility Index (RMI): the 15-item questionnaire that investigates mobility in activity of daily living such as turning in bed, transfers, walking, going upstairs, running. The examiner is required to make one observation (standing unsupported >10 s), and all items are rated in a yes/no format with positive responses scoring a 1 for a maximal RMI score of 15. The higher the score, the better the performance (51).
- Modified Emory Functional Ambulation profile (mEFAP): the test that measures the time to ambulate through five common environmental terrains (floor, carpet, “up and go,” obstacles, and stairs) with or without an assistive device or manual assistance. The five timed subscores are added to derive a total score. The lower the score, the better the performance (52).
- Mobility Milestones (MM): the outcome measure that evaluates the time to achieve five mobility milestones: 1-min sitting balance, 10-s standing balance, a 10-step walk, a 10-m walk, a 6-min walk. The total score is obtained by adding up the time of each task. The higher the score, the longer the patient's recovery time (53).
- Postural Assessment Scale for Stroke (PASS): the 12-item performance-based scale used for assessing and monitoring postural control after stroke. The score of each item ranges from 0 to 3; therefore, the total score ranges from 0 to 36. The higher the score, the better the performance (54).
- Tinetti Performance-Oriented Mobility Assessment (POMA-B): the task-oriented test that measures an adult's balance abilities through an ordinal scale ranging from 0 (most impairment) to 2 (independence). The maximum score is 16. The lower the score, the greater the risk of falling (55).
- Short Physical Performance Battery (SPPB): the group of measures that combines the results of the gait speed, chair stand, and balance tests. The scores range from 0 (worst performance) to 12 (best performance) (56).
- Rivermead Motor Assessment (RMA): the evaluation that assesses functional mobility following stroke (e.g., gait, balance, transfers). It consists of three sections: gross function (RMA-gf), legs and trunk (RMA-lt), arm (RMA-a). Each item is scored either yes “1” or no “0.” The items in each section were ordered so that they were increasingly difficult for most patients, that is, a hierarchical scale. When a patient has failed one item, it is assumed that subsequent items will also be failed. For this reason, not all items need to be administered (57).
Search Strategy
A search of relevant studies was conducted in MEDLINE/PubMed, Physiotherapy Evidence Database (PEDro), the Cochrane Central Register of Controlled Trials, CINAHL, EMBASE, Web of Science, and Scopus databases. We included reports from the international literature published between January 1989 and August 2020. Search terms “included stroke,” “cerebrovascular,” “cerebrovascular disease,” “ischemic stroke,” “hemorrhagic stroke,” “brain injury,” “chronic stroke,” “Lokomat,” “robotic device,” “exoskeleton,” “balance,” “postural balance,” “equilibrium,” “postural control,” “balance control,” “gait,” “walking,” “step,” “weight-bearing,” “locomotion,” “balance training,” “gait training,” and “walking training.” We searched for “randomized controlled trial” as MeSH terms, keywords, or subject headings. Related terms were combined using the Boolean “OR” and “AND.” In order to avoid bias, the search was repeated over several days, also changing the order of the keywords.
Study Selection and Data Extraction
Two authors (FB, MB) independently screened titles and abstracts of the potentially relevant papers and removed the duplicates. Then, a careful check of the papers was performed in order to select only the studies that met the inclusion criteria. The reference lists of relevant papers were also inspected for additional studies potentially missed in the databases search. Any disagreement was solved by confrontation and consensus without involving a third author.
From each study included in the review, the same two authors (FB, MB) independently extracted the following data: title, authors, year of publication, journal of publication, participants (number, mean age, gender), study design, rehabilitative intervention details (frequency and duration of the sessions, Lokomat® parameters such as weight support and speed), outcome measures, results, follow-up.
Evaluation of the Methodological Quality of the Studies
The methodological quality of the studies included in this review was measured through the use of the PEDro scale (58, 59). The PEDro scale includes 10 items (random allocation, concealed allocation, similarity at baseline, subject blinding, therapist blinding, assessor blinding, >85% follow-up for at least one key outcome, intention-to-treat analysis, between-group statistical comparison for at least one key outcome, and point and variability measures for at least one key outcome), each scored as present (1) or absent (0). Criteria that are not specified must be considered as not met. The criteria classified as achieved can be added to obtain a score between 0 (minimum) and 10 (maximum), which indicates the general methodological quality of the study (≤ 4 poor, 4–5 acceptable, 6–8 good, 9–10 excellent) (60).
Data Analysis
A meta-analysis was performed to compare changes (pre- and post-intervention) between the study group (RAGT with Lokomat®) and the control group (conventional physiotherapy and/or treadmill). The studies were grouped according to the outcome measure, and for each meta-analysis, the standardized mean difference along with the 95% confidence interval (CI) was calculated. p < 0.05 was considered significance level. Heterogeneity was determined by the chi-square test and the I2 statistic. All statistical analyses were carried out by means of the Review Manager (RevMan) Software, Version 5.3 (The Cochrane Collaboration, The Nordic Cochrane Centre, London, UK).
Results
Database search identified 600 records; 565 papers were excluded because they were duplicates or not fulfilling the eligibility criteria. Among the remaining 35 papers that were obtained for full-text screening, 13 studies met the inclusion criteria and were included in the systematic review, while 11 studies were included in the quantitative synthesis (meta-analysis) (Figure 1).
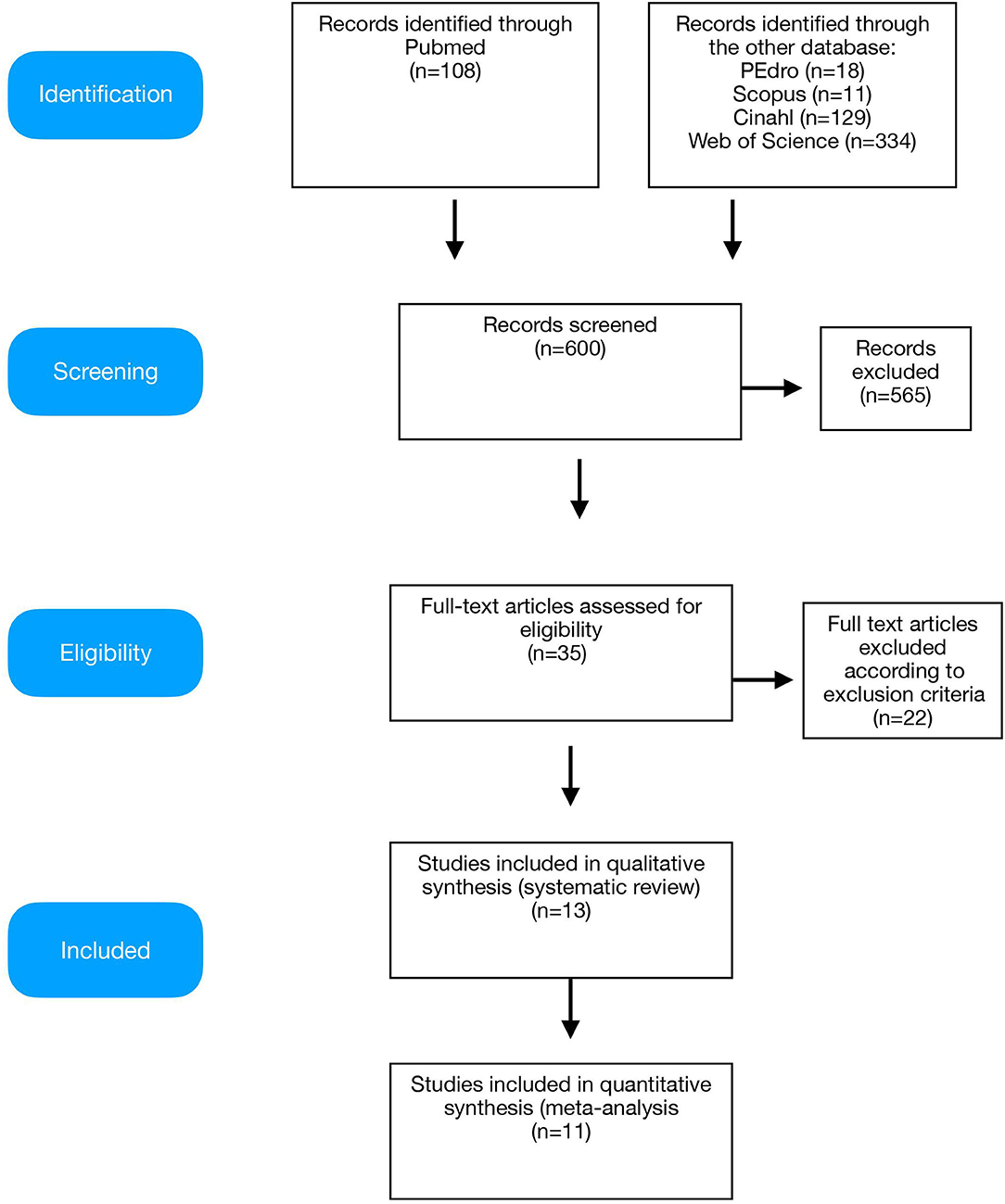
Figure 1. Preferred Reporting Items for Systematic reviews and Meta-Analysis (PRISMA) diagram of the study.
Methodological Quality of the Included Studies
The evaluation of each study, according to the PEDro criteria, is shown in Table 1. The methodological quality of the studies included in this review was globally more than acceptable (average total score 5.5 ± 1.0, range 4–7). Here, 46.2% of trial reports are moderate to high quality, scoring ≥6/10 on the PEDro scale (34, 37, 38, 40, 41, 43).
All participants were randomly assigned to the groups. In six studies (46.2%), the assignment of the subjects was carried out blindly (32, 34, 37, 38, 40, 43). In all papers (except for 55), the groups were similar at the beginning of the study regarding the most relevant prognostic indicators; of these, eight studies included blind assessors in evaluating at least one of the primary outcome measures of the study (31, 35, 37–42). In eight studies (61.5%), the results of at least one outcome measure were obtained in more than 85% of the subjects initially assigned to the groups (31, 33, 34, 37, 40–43). In all studies, the results of the intergroup comparison were reported for at least one of the main outcome measures. Mean and variability data for at least one of the main outcome measures were reported in 11 (84.6%) studies (31–34, 36–41, 43).
Characteristics of Participants
The included studies involved a total of 445 subjects, of which 239 (53.7%) were randomly assigned to the experimental group treated with the Lokomat® and 206 (46.3%) were assigned to the control group, receiving conventional neuromotor rehabilitation and/or treadmill training. In the total sample, 171 (38.4%) were female and 274 (61.6%) were male; the mean age was 58.16 ± 2.38 years in the study group and 64.38 ± 2.19 years in the control group, without statistically significant differences.
Chronic stroke patients were enrolled in seven studies (53.8%) (32, 34, 35, 37, 39, 41, 42), while six papers (46.2%) involved subacute patients.
The characteristics of the participants of each study are shown in Table 2.
Characteristics of the Interventions in the Study Groups (Lokomat® Assisted Gait Training)
In seven studies (53.8%), training with the Lokomat® was combined with conventional physiotherapy and compared to conventional therapy (neuromotor rehabilitation according to stroke guidelines) (35–37, 39–42), while in the remaining six studies, patients performed training with the Lokomat® only compared to conventional physiotherapy (32–35, 38, 43).
The setting of the Lokomat® parameters was very similar in the different studies: body weight support initially 40–50%, then decreased during treatment by 30–40% until no weight support; initial gait speed about 0.4/0.5 m/s, gradually increased up to 0.7/0.8 m/s; 100% guidance, progressively decreased to 0%.
In one study, training with Lokomat® was combined with an augmented reality program by means of a monitor placed in front of the patients that provided a feedback on their performance through an avatar (42). In four other studies (30.8%), patients performing RAGT were provided with a feedback by means of monitors or mirrors (32, 33, 35, 43) that showed their performance parameters.
On average, treatment lasted 5–6 weeks. The study by Belas Dos Santos et al. (39) reported the longest total duration of the treatment, which lasted 5 months. The duration of each individual session was on average 1 h/day, specifically, 15 min for Lokomat® parameters setting and 45 min for training. The frequency of the sessions was 3–5 times/week.
The main characteristics of all the interventions are summarized in Table 2.
Characteristics of the Interventions in the Control Groups (Conventional Rehabilitation Treatment)
Conventional treatment was very similar in all studies included in this review and was performed according to the main methods used in neurorehabilitation: Kabath, Bobath, and Perfetti. Specifically, the following activities were reported: stretching of the paretic lower limb, trunk control exercises, load balancing, exercises for static and dynamic balance, walking training. In four (30.8%) studies, the treadmill was used for locomotor training (33, 34, 37, 42), with speed settings similar to Lokomat® parameters. The duration of the treatment was on average 1 h/day, 3–5 times/week, for a total average duration of 5–6 weeks.
In all studies, intensity and frequency of rehabilitation treatment were comparable between the study group and the control group.
Effects on the Balance
The first study that supported the effectiveness of Lokomat® was performed in subacute stroke patients, demonstrating significantly more improvement than conventional physical therapy in gross coordination of walking (31). The superiority of Lokomat® was not confirmed 2 years later by the study of Hidler et al. (33) who did not find any difference in balance between study and control group; moreover, conventional gait training interventions were shown to be even more effective than Lokomat® for both walking speed and distance. Also Hornby et al. (32) reported greater improvements both in single-limb stance time on the impaired leg and in speed in chronic stroke survivors who received therapist-assisted training compared to Lokomat®. Specifically, larger speed improvements were reported in subjects with less severe gait deficits.
In the pilot study by Westlake and Patten (34) involving chronic stroke patients, even if statistically significant differences were not apparent between Lokomat® and conventional therapy, significantly greater training-related improvements were reported in the Lokomat® group when considering balance as well as gait symmetry, walking speed, and lower extremity motor impairment and function. Some years later, another study suggested that patients undergoing Lokomat® training demonstrated significantly greater improvement on both static and dynamic balance and overground walking speed than those undergoing conventional training (35).
Van Nunen et al. (36) showed that in non-ambulatory subacute stroke subjects, Lokomat® training was as effective as conventional therapy in recovering walking ability, considering walking speed, balance, and function, while higher gains for Lokomat® were reported in chronic stroke subjects in improving balance, activity-specific balance confidence, and spatiotemporal gait parameters (gait speed, cadence, and step length) (37).
Comparable results between Lokomat® and conventional therapy were described in other studies assessing balance, coordination, and functional independence in individuals with ataxia after stroke (39) or locomotion and mobility in subacute non-ambulatory stroke patients (40), respectively.
Other studies showed greater improvement with Lokomat® training both alone and combined with conventional therapy when considering balance, mobility, and fear of falling in ambulatory chronic post-stroke patients (41) as well as a greater persistent reduction of pusher behavior compared to non-robotic physiotherapy (38).
When combined with augmented reality, Lokomat® training induced higher gain in balance and gait abilities in chronic stroke patients when compared to conventional treatment (42). Finally, Lokomat® proved to be more effective than conventional physical therapy in improving lateropulsion and balance function in subacute stroke patients (43).
Data from all the studies are reported in Table 2.
Meta-Analysis
Pooling of results within meta-analyses was possible for the (i) BBS (n = 9), (ii) TUG (n = 5), and (iii) RMI (n = 4).
The characteristics of the different groups considered in the meta-analysis are reported in Table 3.
Analyzing the effect of Lokomat® on the first outcome measure (BBS), the results of the meta-analysis showed no significant difference between study and control group and a high heterogeneity of the included studies (Figure 2). When considering mobility and static and dynamic balance measured by means of TUG, although the studies were heterogeneous, the results showed that the Lokomat® training determined a significantly higher improvement than that in the control group (pooled mean difference = −3.40, 95% CI −4.35 to −2.44; p < 0.00001; I2 = 92%, five trials, 61 participants) (Figure 3). Also, when evaluating the effect of the interventions on the RMI, the meta-analysis revealed a significant difference in favor of the study group (pooled mean difference = 0.40, 95% CI 0.26–0.55; p < 0.00001; I2 = 0%, four trials, 97 participants) (Figure 4).
Discussion
The findings of this systematic review and meta-analysis showed that RAGT performed by means of Lokomat® might induce beneficial effects on balance in individuals affected by stroke similarly to conventional physical therapy. Moreover, data showed that the improvements occur in both acute and chronic stroke patients with different levels of impairment.
In general, as for other robotic exoskeletons, Lokomat® provides high-intensity and task-oriented exercise, allows to perform a gait cycle training also for not independent ambulators, and enhances motor re-education through a multisensory stimulation that involves patients in motivating activities (61, 62).
According to modern principles of gait rehabilitation, Lokomat® guides patients' legs through a preprogrammed physiological gait model that promotes the extension of the hip, encourages the start of the swinging phase, and promotes the step maximizing the load on the lower limbs. So the subjects experience an almost physiological proprioceptive input during walking (63, 64), transferring sensory–motor information to the central nervous system and inducing plastic changes (65–67). In fact, according to the basics of neuroplasticity and motor learning, robotic technologies, by providing controlled, repetitive, and task-specific stimulation, may induce adaptive modifications and reorganization of neural connections and networks, maximizing recovery and functional outcomes. Evidence suggests that robotic guided movements, in addition to typical motor areas, activate also deep neural centers such as the insula and the amygdala that are involved in movement memory and motivation to the movement and play a key role in inducing sensory processes related to the internal representation of the movements (68). Therefore, the simultaneous activation of sensory and motor systems could facilitate perceptual–motor skill learning and transfer (69).
As gait and balance are strictly related due to the nature of bipedal locomotion in which a majority of gait cycle is spent in single-limb support (70), it is not surprising that the clinical outcome of Lokomat®, in addition to gait parameters, also may involve balance. Indeed, the improvements in balance measures induced by Lokomat® suggest a transfer of skill from a task-specific locomotor training to non-walking functional balance parameters. The concept of “reverse transfer” was just proposed in relation to the idea that repeated practice of high-intensity walking training may improve non-walking tasks such as static balance and postural stability (71). The results of most of the papers included in this review support this view and hint that the dynamic, high-intensity, controlled, and repetitive training provided by Lokomat® may favor additional carryover to recovery parameters of postural and balance control beyond gait performance.
In the face of these favorable results, this review nevertheless highlights the presence of conflicting data: three studies in fact found that Lokomat® had the same effectiveness on balance as traditional physiotherapy (33, 36, 39), while one study has even indicated a smaller effect than conventional rehabilitation (32). Really, some doubts about the efficacy of RAGT had already been posed a few years ago, particularly regarding the scarce control of the patient over the initiation of each step, the lack of variability in visuospatial flow as opposed to overground walking, and the poor active contribution of the patient, crucial for activity-dependent learning (72–75).
It should also be emphasized that since the studies were few and often conducted on small populations, the results of this meta-analysis could have been polarized by the studies with wider sample sizes. That said, it is also true that the results of the larger studies also are usually the most reliable and generalizable, so in our opinion, the results of this review are completely reliable.
Furthermore, some general considerations must be made, first of all in relation to the device itself and to the setting parameters. The Lokomat® models have changed slightly over the years (e.g., Lokomat® models at the beginning maintained firm the pelvis, the successive models move also the pelvis of the patients), and in recent years, in some cases, further devices have been added to the robot (e.g., a monitor for augmented reality or visual feedback). Despite this, the basic structure and the preprogrammed walking pattern drove by the Lokomat® did not change significantly (gait cycle timing as stance vs. swing phase, inter-limb and inter-joint coordination, appropriate limb loading) and therefore, in our opinion, also the conditions of use and effects are yet comparable among different models.
One of the main advantages of robots consists of the possibility of freely setting the parameters according to each patient's characteristics; however, this implies a great heterogeneity in training protocols among clinical trials, as emerged also in this review, with consequent difficulties in comparing them. In principle, therapists seem to progressively adjust the level of motor assistance, resistance, and guidance as the patient's condition modifies, always providing the minimum amount of motor power required and maximizing patients' strength, motor function, and endurance (76). Despite that the scientific community pointed out the need to standardize rehabilitation protocols in order to optimize robotic use, to date, clear guidelines to select parameters are lacking (61) also for Lokomat®, and the usual approach promotes the customization of treatment, adapting the robots to the patient's clinical condition.
When considering the outcome measures, the results of this meta-analysis revealed that the BBS did not capture any difference between the intervention and the control group, unlike the other scales (TUG and RMI).
With respect to this issue, BBS can have floor and ceiling effects in evaluating balance in post-stroke subjects, and other balance measures adjunct or in conjunction with BBS have been suggested in evaluating balance in post-stroke subjects (77).
As about half of the studies included in this review involved chronic stroke patients, the ceiling effect may have resulted in an underestimation of therapy effects in the higher functioning individuals, without intergroup differences. Unfortunately, the variety of outcome measures used in the literature restricted the number of studies that could be considered in the meta-analysis.
When to start the treatment after the onset of the disease and for how long represent other crucial issues.
Although previous studies suggesting the presence of a “critical window for recovery” within the first 3–6 months post-stroke (78) seemed to justify to stop the treatments in the chronic phase (79), data from studies included in this review did not support this view, showing that Lokomat® was effective in improving balance and gait abilities also in chronic post-stroke subjects (33–35, 37, 41), consistently with more recent evidence suggesting that the time window for recovery may extend beyond 1 year post-stroke (80).
In addition to the heterogeneity of the studies, this review showed that the lack of longitudinal evaluations for most of the papers represents a main limitation; however, the limited data of the six studies with a follow-up assessment (follow-up range 2–36 weeks) showed substantial maintenance of the results at the post-training evaluation (32, 33, 35, 36, 38, 43). As no study reported side effects attributable to the use of Lokomat®, it seems legitimate to argue that this robotic device has been shown to be safe and feasible for use with subacute and chronic stroke patients.
Overall, the results of this meta-analysis elicit some comments and criticisms useful to address future research.
While powered exoskeletons hold promise, the literature surrounding their use for balance recovery is only just beginning to gather, with the majority focusing on gait. Given that this is a relatively new intervention for stroke, the objective of this meta-analysis was to map the current literature surrounding the use of Lokomat® for balance rehabilitation in post-stroke individuals and to identify gaps in the research. As expected, there are only a small number of published studies relevant to this topic. Moreover, studies were quite heterogeneous, which makes interpretation of the results challenging. First of all, no data are available regarding the optimal Lokomat® protocol. Larger controlled studies are required to determine the optimal timing and protocol design that will maximize efficacy and long-term outcomes in stroke patients. In addition, type, side, and severity of stroke and comorbid conditions were not considered in this review because of the scarcity of studies in this area: RCTs in which training with acute, subacute, and chronic stroke patients with specific categories of disability is compared might provide more specific indications for the use of Lokomat®. Finally, it is not definitely clear whether Lokomat® leads to a better outcome in balance-related outcome measurements compared with other more conventional gait rehabilitation methods. Also, the effects on static and dynamic balance, the impact of such intervention on functional goals that are meaningful to the patient, the transfer of the functional gains to real-world settings, and the effect on health-related quality of life have not yet received a satisfactory answer.
In conclusion, according to the results from this study, due to the small number of high-quality studies, the limited samples, the lack of follow-up evaluations, and the inconsistent results, at the moment, there is insufficient evidence to conclusively advocate in favor or against use of Lokomat® to improve balance control in stroke patients. However, the findings of most studies suggest that Lokomat® increasing therapy dosage, intensity, number of repetition, execution of task-oriented exercises, and combining top-down and bottom-up approaches can represent a useful tool for the physiotherapist to promote plasticity and functional recovery. In this sense, the present review and meta-analysis may represent a starting point for the development of new well-based research, continuing to advance the clinical application of powered exoskeletons.
Data Availability Statement
The original contributions presented in the study are included in the article/supplementary material, further inquiries can be directed to the corresponding author.
Author Contributions
The study has been designed by FB, MB, and CZ. Data have been gathered by FB and MB. Data have been analyzed by FB and CZ. The manuscript has been drafted by MB and CZ. MS and DI revised the manuscript for important intellectual content. All authors approved the final version of the manuscript.
Conflict of Interest
The authors declare that the research was conducted in the absence of any commercial or financial relationships that could be construed as a potential conflict of interest.
The reviewer MI declared a shared affiliation, with no collaboration, with the author MS at the time of the review.
References
1. Mehrholz J, Kugler J, Pohl M. Locomotor training for walking after spinal cord injury. Cochrane Database Syst Rev. (2012) 11:CD006676. doi: 10.1002/14651858.CD006676.pub3
2. Fulk GD, Reynolds C, Mondal S, Deutsch JE. Predicting home and community walking activity in people with stroke. Arch Phys Med Rehabil. (2010) 91:1582–6. doi: 10.1016/j.apmr.2010.07.005
3. Lee KB, Lim SH, Ko EH, Kim YS, Lee KS, Hwang BY. Factors related to community ambulation in patients with chronic stroke. Top Stroke Rehabil. (2015) 22:63–71. doi: 10.1179/1074935714Z.0000000001
4. Louie D, Eng J. Berg Balance Scale score at admission can predict walking suitable for community ambulation at discharge from inpatient stroke rehabilitation. J Rehabil Med. (2018) 50:37–44. doi: 10.2340/16501977-2280
5. Wall JC, Turnbull GI. Gait asymmetries in residual hemiplegia. Arch Phys Med Rehabil. (1986) 67:550–3.
6. Von Schroeder HP, Coutts RD, Lyden PD, Billings E. Gait parameters following stroke: a practical assessment. J Rehabil Res Dev. (1995) 32:25–31.
7. Olney SJ, Richards C. Hemiparetic gait following stroke. Part 1: Characteristics. Gait Posture. (1996) 4:136–48. doi: 10.1016/0966-6362(96)01063-6
8. Chen G, Patten C, Kothari DH, Zajac FE. Gait differences between individuals with post-stroke hemiparesis and non-disabled controls at matched speeds. Gait Posture. (2005) 22:51–6. doi: 10.1016/j.gaitpost.2004.06.009
9. Middleton A, Braun CH, Lewek MD, Fritz SL. Balance impairment limits ability to increase walking speed in individuals with chronic stroke. Disabil Rehabil. (2017) 39:497–502. doi: 10.3109/09638288.2016.1152603
10. Sackley CM. Falls, sway, and symmetry of weight-bearing after stroke. Int Disabil Stud. (1991) 13:1–4. doi: 10.3109/03790799109166267
11. Davenport RJ, Dennis MS, Wellwood I, Warlow CP. Complications after acute stroke. Stroke. (1996) 27:415–20. doi: 10.1161/01.STR.27.3.415
12. Hyndman D, Ashburn A, Stack E. Fall events among people with stroke living in the community: circumstances of falls and characteristics of fallers. Arch Phys Med Rehabil. (2002) 83:165–70. doi: 10.1053/apmr.2002.28030
13. Weerdesteyn V, de Niet M, van Duijnhoven HJ, Geurts AC. Falls in individuals with stroke. J Rehabil Res Dev. (2008) 45:1195–213. doi: 10.1682/JRRD.2007.09.0145
14. Xu T, Clemson L, O'Loughlin K, Lannin NA, Dean C, Koh G. Risk factors for falls in community stroke survivors: a systematic review and meta-analysis. Arch Phys Med Rehabil. (2018) 99:563–73. doi: 10.1016/j.apmr.2017.06.032
15. Eng JJ, Tang PF. Gait training strategies to optimize walking ability in people with stroke: a synthesis of the evidence. Expert Rev Neurother. (2007) 7:1417–36. doi: 10.1586/14737175.7.10.1417
16. Schmid AA, Van Puymbroeck M, Altenburger PA, Miller KK, Combs SA, Page SJ. Balance is associated with quality of life in chronic stroke. Top Stroke Rehabil. (2013) 20:340–6. doi: 10.1310/tsr2004-340
17. Wei WE, De Silva DA, Chang HM, Yao J, Matchar DB, Young SHY, et al. Post stroke patients with moderate function have the greatest risk of falls: a National Cohort study. BMC Geriatr. (2019) 19:373. doi: 10.1186/s12877-019-1377-7
18. Martino Cinnera A, Bonnì S, Pellicciari MC, Giorgi F, Caltagirone C, Koch G. Health-related quality of life (HRQoL) after stroke: positive relationship between lower extremity and balance recovery. Top Stroke Rehabil. (2020) 10:1–7. doi: 10.1080/10749357.2020.1726070
19. Perry J, Garret M, Gronkley JK, Mulroy SJ. Classification of walking handicap in the stroke population. Stroke. (1995) 26:982–89. doi: 10.1161/01.STR.26.6.982
20. French B, Thomas L, Leathley M, Sutton C, McAdam J, Forster A, et al. Does repetitive task training improve functional activity after stroke? A Cochrane systematic review and meta-analysis. J Rehabil Med. (2010) 42:9–14. doi: 10.2340/16501977-0473
21. Varoqui D, Froger J, Pélissier JY, Bardy BG. Effect of coordination biofeedback on (re)learning preferred postural patterns in post-stroke patients. Motor Control. (2011) 15:187–205. doi: 10.1123/mcj.15.2.187
22. Hessam M, Salehi R, Yazdi MJS, Negahban H, Rafie S, Mehravar M. Relationship between functional balance and walking ability in individuals with chronic stroke. J Phys Ther Sci. (2018) 30:993–6. doi: 10.1589/jpts.30.993
23. Winchester P, McColl R, Querry R, Foreman N, Mosby J, Tansey K, et al. Changes in supra spinal activation patterns following robotic locomotor therapy in motor- incomplete spinal cord injury. Neurorehabil Neural Repair. (2005) 19:313–24. doi: 10.1177/1545968305281515
24. Bruni MF, Melegari C, De Cola MC, Bramanti A, Bramanti P, Calabrò RS. What does best evidence tell us about robotic gait rehabilitation in stroke patients: a systematic review and meta-analysis. J Clin Neurosci. (2018) 48:11–7. doi: 10.1016/j.jocn.2017.10.048
25. Nam KY, Kim HJ, Kwon BS, Park JW, Lee HJ, Yoo A. Robot-assisted gait training (Lokomat) improves walking function and activity in people with spinal cord injury: a systematic review. J Neuroeng Rehabil. (2017) 14:24. doi: 10.1186/s12984-017-0232-3
26. Swinnen E, Beckwèe D, Meeusen R, Baeyens JP, Kerckhofs E. Does robot-assisted gait rehabilitation improve balance in stroke patients? A systematic review. Top Stroke Rehabil. (2014) 21:87–100. doi: 10.1310/tsr2102-87
27. Moucheboeuf G, Griffier R, Gasq D, Glize B, Bouyer L, Dehail P, et al. Effects of robotic gait training after stroke: a meta-analysis. Ann Phys Rehabil Med. (2020) 63:518–34. doi: 10.1016/j.rehab.2020.02.008
28. Iosa M, Morone G, Cherubini A, Paolucci S. The three laws of neurorobotics: a review on what neurorehabilitation robots should do for patients and clinicians. J Med Biol Eng. (2016) 36:1–11. doi: 10.1007/s40846-016-0115-2
29. Moher D, Liberati A, Tetzlaff J, Altman DG, PRISMA Group. Preferred reporting items for systematic reviews and meta-analyses: the PRISMA statement. PLoS Med. (2009) 6:e1000097. doi: 10.1371/journal.pmed.1000097
30. Hutton B, Salanti G, Caldwell DM, Chaimani A, Schmid CH, Cameron C, et al. The PRISMA extension statement for reporting of systematic reviews incorporating network meta-analyses of health care interventions: checklist and explanations. Ann Intern Med. (2015) 162:777–84. doi: 10.7326/M14-2385
31. Mayr A, Kofler M, Quirbach E, Matzak H, Fröhlich K, Saltuari L. Prospective, blinded, randomized crossover study of gait rehabilitation in stroke patients using the Lokomat gait orthosis. Neurorehabil Neural Repair. (2007) 21:307–14. doi: 10.1177/1545968307300697
32. Hornby TG, Campbell DD, Kahn JH, Demott T, Moore JL, Roth HR. Enhanced gait-related improvements after therapist- versus robotic-assisted locomotor training in subjects with chronic stroke: a randomized controlled study. Stroke. (2008) 39:1786–92. doi: 10.1161/STROKEAHA.107.504779
33. Hidler J, Nichols D, Pelliccio M, Brady K, Campbell DD, Kahn JH, et al. Multicenter randomized clinical trial evaluating the effectiveness of the Lokomat in subacute stroke. Neurorehabil Neural Repair. (2009) 23:5–13. doi: 10.1177/1545968308326632
34. Westlake KP, Patten C. Pilot study of Lokomat versus manual-assisted treadmill training for locomotor recovery post-stroke. J Neuroeng Rehabil. (2009) 6:18. doi: 10.1186/1743-0003-6-18
35. Uçar DE, Paker N, Bugdayci D. Lokomat: a therapeutic chance for patients with chronic hemiplegia. NeuroRehabilitation. (2014) 34:447–53. doi: 10.3233/NRE-141054
36. van Nunen MP, Gerrits KH, Konijnenbelt M, Janssen TW, de Haan A. Recovery of walking ability using a robotic device in subacute stroke patients: a randomized controlled study. Disabil Rehabil Assist Technol. (2015) 10:141–8. doi: 10.3109/17483107.2013.873489
37. Bang DH, Shin WS. Effects of robot-assisted gait training on spatiotemporal gait parameters and balance in patients with chronic stroke: a randomized controlled pilot trial. NeuroRehabilitation. (2016) 38:343–9. doi: 10.3233/NRE-161325
38. Bergmann J, Krewer C, Jahn K, Müller F. Robot-assisted gait training to reduce pusher behavior: a randomized controlled trial. Neurology. (2018) 91:e1319–e27. doi: 10.1212/WNL.0000000000006276
39. Belas Dos Santos M, Barros de Oliveira C, Dos Santos A, Garabello Pires C, Dylewski V, Arida RM. A comparative study of conventional physiotherapy versus robot-assisted gait training associated to physiotherapy in individuals with ataxia after stroke. Behav Neurol. (2018) 2018:2892065. doi: 10.1155/2018/2892065
40. Mayr A, Quirbach E, Picelli A, Kofler M, Smania N, Saltuari L. Early robot-assisted gait retraining in non-ambulatory patients with stroke: a single blind randomized controlled trial. Eur J Phys Rehabil Med. (2018) 54:819–26. doi: 10.23736/S1973-9087.18.04832-3
41. Mustafaoglu R, Erhan B, Yeldan I, Ersöz Hüseyinsinoglu B, Gündüz B, Razak Özdinçler A. The effects of body weight-supported treadmill training on static and dynamic balance in stroke patients: a pilot, single-blind, randomized trial. Turk J Phys Med Rehabil. (2018) 64:344–52. doi: 10.5606/tftrd.2018.2672
42. Park J, Chung Y. The effects of robot-assisted gait training using virtual reality and auditory stimulation on balance and gait abilities in persons with stroke. NeuroRehabilitation. (2018) 43:227–35. doi: 10.3233/NRE-172415
43. Yun N, Joo MC, Kim SC, Kim MS. Robot-assisted gait training effectively improved lateropulsion in subacute stroke patients: a single-blinded randomized controlled trial. Eur J Phys Rehabil Med. (2018) 54:827–36. doi: 10.23736/S1973-9087.18.05077-3
44. Schardt C, Adams M B, Owens T, Keitz S, Fontelo P. Utilization of the PICO framework to improve searching PubMed for clinical questions. BMC Med Inform Decis Mak. (2007) 7:16. doi: 10.1186/1472-6947-7-16
45. Maulden SA, Gassaway J, Horn SD, Smout RJ, DeJong G. Timing of initiation of rehabilitation after stroke. Arch Phys Med Rehabil. (2005) 86:S34–S40. doi: 10.1016/j.apmr.2005.08.119
46. Hsueh IP, Wang CH, Sheu CF, Hsieh CL. Comparison of psychometric properties of three mobility measures for patients with stroke. Stroke. (2003) 34:1741–5. doi: 10.1161/01.STR.0000075295.45185.D4
47. Jezernik S, Colombo G, Keller T, Frueh H, Morari M. Robotic orthosis lokomat: a rehabilitation and research tool. Neuromodulation. (2003) 6:108–15. doi: 10.1046/j.1525-1403.2003.03017.x
48. Amblard B, Crémieux J, Marchand AR, Carblanc A. Lateral orientation and stabilization of human stance: static versus dynamic visual cues. Exp Brain Res. (1985) 61:21–37. doi: 10.1007/BF00235617
49. Berg K, Wood-Dauphinee SL, Williams JL, Maki B. Measuring balance in the elderly: validation of an instrument. Can J Public Health. (1992) 83:S7–S11.
50. Mathias S, Nayak US, Isaacs B. Balance in elderly patients: the “Get-up and Go” test. Arch Phys Med Rehabil. (1986) 14:387–9.
51. Collen FM, Wade DT, Robb GF, Bradshaw CM. The Rivermead Mobility Index: a further development of the Rivermead Motor Assessment. Int Disabil Studies. (1991) 13:50–4. doi: 10.3109/03790799109166684
52. Baer HR, Wolf SL. Modified emory functional ambulation profile: an outcome measure for the rehabilitation of poststroke gait dysfunction. Stroke. (2001) 32:973–9. doi: 10.1161/01.STR.32.4.973
53. Smith MT, Baer GD. Achievement of simple mobility milestones after stroke. Arch Phys Med Rehabil. (1999) 80:442–7. doi: 10.1016/S0003-9993(99)90283-6
54. Benaim C, Perennou DA, Villy J, Rousseaux M, Pelissier JY. Validation of a standardized assessment of postural control in stroke patients: the Postural Assessment Scale for Stroke Patients (PASS). Stroke. (1999) 30:1862–8. doi: 10.1161/01.STR.30.9.1862
55. Tinetti ME, Williams TF, Mayewski R. Fall risk index for elderly patients based on number of chronic disabilities. Am J Med. (1986) 80:429–34. doi: 10.1016/0002-9343(86)90717-5
56. Guralnik J, Simonsick EM, Ferrucci L, Glynn RJ, Berkman LF, Blazer DG, et al. A short physical performance battery assessing lower extremity function: association with self-reported disability and prediction of mortality and nursing home admission. J Gerontol. (1994) 49:M85–94. doi: 10.1093/geronj/49.2.M85
57. Lincoln NB, Leadbitter D. Assessment of motor function in stroke patients. Physiotherapy. (1979) 65:48–51.
58. Maher CG, Sherrington C, Herbert RD, Moseley AM, Elkins M. Reliability of the PEDro scale for rating quality of randomized controlled trials. Phys Ther. (2003) 83:713–21. doi: 10.1093/ptj/83.8.713
59. Macedo LG, Elkins MR, Maher CG, Moseley AM, Herbert RD, Sherrington C. There was evidence of convergent and construct validity of Physiotherapy Evidence Database quality scale for physiotherapy trials. J Clin Epidemiol. (2010) 63:920–5. doi: 10.1016/j.jclinepi.2009.10.005
60. Foley NC, Teasell RW, Bhogal SK, Speechley MR. Stroke rehabilitation evidence-based review: methodology. Top Stroke Rehabil. (2003) 10:1–7. doi: 10.1310/Y6TG-1KQ9-LEDQ-64L8
61. Iosa M, Morone G, Bragoni M, De Angelis D, Venturiero V, Coiro P, et al. Driving electromechanically assisted Gait Trainer for people with stroke. J Rehabil Res Dev. (2011) 48:135–46. doi: 10.1682/JRRD.2010.04.0069
62. Chang WH, Kim MS, Huh JP, Lee PK, Kim YH. Effects of robot-assisted gait training on cardiopulmonary fitness in subacute stroke patients: a randomized controlled study. Neurorehabil Neural Repair. (2012) 26:318–24. doi: 10.1177/1545968311408916
63. He Y, Eguren D, Luu TP, Contreras-Vidal JL. Risk management and regulations for lower limb medical exoskeletons: a review. Med Devices (Auckl). (2017) 10:89–107. doi: 10.2147/MDER.S107134
64. Molteni F, Gasperini G, Cannaviello G, Guanziroli E. Exoskeleton and end-effector robots for upper and lower limbs rehabilitation: narrative review. PM R. (2018) 10:S174–S88. doi: 10.1016/j.pmrj.2018.06.005
65. Stroemer RP, Kent TA, Hulsebosch CE. Acute increase in expression of growth associated protein GAP-43 following cortical ischemia in rat. Neurosci Lett. (1993) 162:51–4. doi: 10.1016/0304-3940(93)90557-2
66. Li S, Carmichael ST. Growth-associated gene and protein expression in the region of axonal sprouting in the aged brain after stroke. Neurobiol Dis. (2006) 23:362–73. doi: 10.1016/j.nbd.2006.03.011
67. Nudo RJ. Postinfarct cortical plasticity and behavioral recovery. Stroke. (2007) 38:840–5. doi: 10.1161/01.STR.0000247943.12887.d2
68. Nocchi F, Gazzellini S, Grisolia C, Petrarca M, Cannatà V, Cappa P. Brain network involved in visual processing of movement stimuli used in upper limb robotic training: an fMRI study. J Neuroeng Rehabil. (2012) 9:49. doi: 10.1186/1743-0003-9-49
69. Müller S, Vallence AM, Winstein C. Investigation of perceptualmotor behavior across the expert athlete to disabled patient skill continuum can advance theory and practical application. J Mot Behav. (2018) 50:697–707. doi: 10.1080/00222895.2017.1408557
70. Beyaert C, Vasa R, Frykberg GE. Gait post-stroke: pathophysiology and rehabilitation strategies. Neurophysiol Clin. (2015) 45:335–55. doi: 10.1016/j.neucli.2015.09.005
71. Hornby TG, Straube DS, Kinnaird CR, Holleran CL, Echauz AJ, Rodriguez KS, et al. Importance of specificity, amount, and intensity of locomotor training to improve ambulatory function in patients post-stroke. Topics Stroke Rehabil. (2011) 18:293–307. doi: 10.1310/tsr1804-293
72. Lotze M, Braun C, Birbaumer N, Anders S, Cohen LG. Motor learning elicited by voluntary drive. Brain. (2003) 126:866–72. doi: 10.1093/brain/awg079
73. Dobkin BH. Neurobiology of rehabilitation. Ann N Y Acad Sci. (2004) 1038:148–70. doi: 10.1196/annals.1315.024
74. Kaelin-Lang A, Sawaki L, Cohen LG. Role of voluntary drive in encoding an elementary motor memory. J Neurophysiol. (2005) 93:1099–103. doi: 10.1152/jn.00143.2004
75. Dobkin BH, Duncan PW. Should body weight-supported treadmill training and robotic-assistive steppers for locomotor training trot back to the starting gate? Neurorehabil Neural Repair. (2012) 26:308–17. doi: 10.1177/1545968312439687
76. Fritz H, Patzer D, Galen SS. Robotic exoskeletons for reengaging in everyday activities: promises, pitfalls, and opportunities. Disabil Rehabil. (2019) 41:560–3. doi: 10.1080/09638288.2017.1398786
77. Blum L, Korner-Bitensky N. Usefulness of the Berg Balance Scale in stroke rehabilitation: a systematic review. Phys Ther. (2008) 88:559–66. doi: 10.2522/ptj.20070205
78. Carmichael ST, Archibeque I, Luke L, Nolan T, Momiy J, Li S. Growth-associated gene expression after stroke: evidence for a growth-promoting region in peri-infarct cortex. Exp Neurol. (2005) 193:291–311. doi: 10.1016/j.expneurol.2005.01.004
79. Biernaskie J, Chernenko G, Corbett D. Efficacy of rehabilitative experience declines with time after focal ischemic brain injury. J Neurosci. (2004) 24:1245–54. doi: 10.1523/JNEUROSCI.3834-03.2004
Keywords: balance, Lokomat®, exoskeleton, robotics, stroke, neurorehabilitation, gait
Citation: Baronchelli F, Zucchella C, Serrao M, Intiso D and Bartolo M (2021) The Effect of Robotic Assisted Gait Training With Lokomat® on Balance Control After Stroke: Systematic Review and Meta-Analysis. Front. Neurol. 12:661815. doi: 10.3389/fneur.2021.661815
Received: 31 January 2021; Accepted: 04 June 2021;
Published: 06 July 2021.
Edited by:
Giovanni Abbruzzese, University of Genoa, ItalyReviewed by:
Marco Iosa, Sapienza University of Rome, ItalyCarmelo Chisari, Pisana University Hospital, Italy
Anke Van Bladel, Ghent University, Belgium
Copyright © 2021 Baronchelli, Zucchella, Serrao, Intiso and Bartolo. This is an open-access article distributed under the terms of the Creative Commons Attribution License (CC BY). The use, distribution or reproduction in other forums is permitted, provided the original author(s) and the copyright owner(s) are credited and that the original publication in this journal is cited, in accordance with accepted academic practice. No use, distribution or reproduction is permitted which does not comply with these terms.
*Correspondence: Michelangelo Bartolo, YmFydG9sb21pY2hlbGFuZ2Vsb0BnbWFpbC5jb20=