- 1Department of Epidemiology, School of Public Health, University of Michigan, Ann Arbor, MI, United States
- 2Survey Research Center, Institute for Social Research, University of Michigan, Ann Arbor, MI, United States
- 3Population Studies Center, Institute for Social Research, University of Michigan, Ann Arbor, MI, United States
Background: Altered lipid metabolism may be a risk factor for dementia, and blood cholesterol level has a strong genetic component. We tested the hypothesis that dyslipidemia (either low levels of high-density lipoprotein cholesterol (HDL-C) or high total cholesterol) is associated with cognitive status and domains, and assessed causality using genetic predisposition to dyslipidemia as an instrumental variable.
Methods: Using data from European and African genetic ancestry participants in the Health and Retirement Study, we selected observations at the first non-missing biomarker assessment (waves 2006–2012). Cognition domains were assessed using episodic memory, mental status, and vocabulary tests. Overall cognitive status was categorized in three levels (normal, cognitive impairment non-dementia, dementia). Based on 2018 clinical guidelines, we compared low HDL-C or high total cholesterol to normal levels. Polygenic scores for dyslipidemia were used as instrumental variables in a Mendelian randomization framework. Multivariable logistic regressions and Wald-type ratio estimators were used to examine associations.
Results: Among European ancestry participants (n = 8,781), at risk HDL-C levels were associated with higher odds of cognitive impairment (OR = 1.20, 95% CI: 1.03, 1.40) and worse episodic memory, specifically. Using cumulative genetic risk for HDL-C levels as a valid instrumental variable, a significant causal estimate was observed between at risk low HDL-C levels and higher odds of dementia (OR = 2.15, 95% CI: 1.16, 3.99). No significant associations were observed between total cholesterol levels and cognitive status. No significant associations were observed in the African ancestry sample (n = 2,101).
Conclusion: Our study demonstrates low blood HDL-C is a potential causal risk factor for impaired cognition during aging in non-Hispanic whites of European ancestry. Dyslipidemia can be modified by changing diets, health behaviors, and therapeutic strategies, which can improve cognitive aging. Studies on low density lipoprotein cholesterol, the timing of cholesterol effects on cognition, and larger studies in non-European ancestries are needed.
Introduction
Dementia is a chronic and progressive syndrome beyond normal cognitive decline in aging. It affects memory, mental status, and activities of daily living, resulting in disability and dependency. There is no treatment currently available to cure or to alter the progressive course of dementia (1). Worldwide, over 50 million people are currently living with dementia, with 10 million new cases every year (1). The cost for global dementia treatment is expected to reach two trillion United States dollars by 2030 (2).
Altered lipid metabolism is implicated in dementia pathogenesis. Several epidemiological studies show associations between fundamental differences in cholesterol levels and cognitive decline risks. A meta-analysis reported a relative risk of 2.14 for participants with high total cholesterol (TC) in midlife to develop all-type dementia compared to participants with normal cholesterol (3). Another study found that risk of poor memory was associated with lower high-density lipoprotein cholesterol (HDL-C) (4). However, results have been inconsistent across studies and the causal relationship has not been rigorously assessed (5–7). Blood cholesterol levels have a strong inherited basis. A recent genome-wide association study (GWAS) identified 157 loci associated with blood cholesterol levels, which cumulatively explained over 50% of the inter-individual variation of blood cholesterol levels (8, 9). Given the large genetic component, genetic predisposition to increased blood cholesterol levels may be used as an instrumental variable to test the inferred causality of blood cholesterol levels on cognitive impairment.
Our current study hypothesized that blood cholesterol levels (HDL-C and TC) play an etiological role in dementia. This hypothesis posits that genetic variants that affect lipid metabolism would influence risk of cognitive impairment through changes in blood cholesterol levels. We applied an inverse-variance weighted Mendelian randomization framework analysis in European and African genetic ancestry samples in a United States based aging study, to investigate the causal nature of lipid dysregulation and cognitive impairment. Throughout this study, we use the term dementia to refer to the syndrome characterized by progressive memory loss that interferes with activities of daily living, but without specifying an underlying etiological mechanism.
Methods
Study Sample From the Health and Retirement Study
The Health and Retirement Study (HRS), funded by the National Institute on Aging and the Social Security Administration, is a publicly available longitudinal panel cohort study of people over age 50 in the United States. The study includes assessments of economic conditions, health, and other aspects of life surveyed in waves every 2 years since its inception in 1992. More than 43,000 people have participated in the study to date (10). Each wave interviews roughly 20,000 individuals.
Sample selection steps are shown in Supplementary Figure 1. We excluded participants who were younger than 50 or over 90 at their cholesterol measurement because the underlying neuropathological mechanisms and risk factors of dementia are considerably different in those age groups (11). To minimize misclassification of cognitive status, we also excluded individuals who presented with dementia at the prior wave and with a normal cognition measure in a subsequent wave (n = 29).
Exposure Assessments
HRS began collecting blood-based biomarkers, including HDL-C and TC, from individuals who participated in an enhanced face-to-face interview in 2006 (a randomly selected half of the sample) and 2008 (the other half). Similarly, new cohort members in 2010 were randomly assigned to one of the two groups. The HRS repeats blood sampling for biomarker measures for each group every 4 years (12–14). Special informed consent was obtained for the blood acquisition process (10). Cholesterol levels were retrieved from the HRS health sensitive data (15). We used the National Health and Nutrition Examination Survey equivalent assay values constructed by the HRS in our analyses (16). We selected the first non-missing blood assay results from participants (over the course of four waves with biomarkers 2006–2012) to maximize the sample size of non-missing data in biomarkers, genetics, and cognitive assessments across waves (n = 18,700).
We dichotomized blood cholesterol levels based on the 2018 guidelines published in the Journal of the American College of Cardiology (17): For HDL-C, normal levels were defined as: ≥40 mg/dL for males or ≥50 mg/dL for females, and at risk (low) levels were defined as: <40 mg/dL for males or <50 mg/dL for females. For TC, normal levels were defined as: <240 mg/dL, and at risk (high) levels were defined as: ≥240 mg/dL. Diverse lipid ratios (e.g., TC/HDL-C, LDL/HDL) have been used as atherogenic indexes for metabolic syndrome and cardiovascular disease. These indices have shown better predictive capacity for the aforementioned diseases than isolated lipid markers (18). Hence, we used the TC/HDL-C ratio as a sensitivity analysis in our study.
Outcome Assessments
HRS respondents who could participate in the interview themselves were asked to perform a series of cognition tests (Table 1). Their performance on each task was recorded in a continuous scale (19). Our main outcome, the Langa-Weir cognitive status, was classified in three levels based on a total score on a 27-point scale (normal: 12–27, cognitive impairment-non dementia (CIND): 7–11, and dementia: 0–6) (20). Few studies have specifically examined how cholesterol levels influence different cognitive domains, and while the majority have reported that lower HDL-C levels are tied to worse memory performance, some have reported a lack of association with other cognitive functions (21). Thus, we also used summary scores of three separate cognitive domains: word recall for episodic memory, serial 7 subtraction test for mental status, and an adaptation of the Wechsler Adult Intelligence Scale-revised vocabulary in our analyses (22).
Immediate and Delayed Recall
The interviewer read a list of 20 nouns (e.g., lake, car, army, etc.,) to the respondent, and asked the respondent to recall as many words as possible from the list in any order. After ~5 min of asking other survey questions (e.g., depression, and cognition items including backwards count, and serial 7's) the respondent was asked to recall the nouns previously presented as part of the initial recall task.
Serial 7's Test
The interviewer asked the respondent to subtract 7 from 100, and continue subtracting 7 from each subsequent number for a total of five trials. It was up to the respondent to remember the value from the prior subtraction without prompting.
Vocabulary
This measure was adapted from the Wechsler Adult Intelligence Scale-revised. Specifically, respondents were asked to define five words from one of two sets: (1) repair, fabric, domestic, remorse, plagiarize, and (2) conceal, enormous, perimeter, compassion, audacious. Respondents are randomly assigned to one set of words in the first wave and the sets are alternated in each wave thereafter (23).
All cognition variables were measured at the same wave as the corresponding cholesterol measure and retrieved from the HRS cross-wave imputation of cognitive functioning data (24).
Covariate Assessments
Other covariates used in our analysis included demographic characteristics, behavioral risk factors, and chronic health conditions. Age (years) at was calculated by subtracting the birth year from the cholesterol measurement year. Cholesterol measurement wave was an indicator of individual's first non-missing cholesterol measure. Sex (male/female), years of education, proxy status (self/proxy-respondent), body mass index (BMI, kilograms/meters2), lipid-lowering medication (yes/no), histories of stroke, hypertension, diabetes (yes/no), smoking status (current, former, never), and alcohol consumption (ever drinking yes/no) were self-reported. Average exercise level was an average score of three exercise variables (vigorous, moderate, mild) created from self-reported physical activity variables in the HRS. All covariates were assessed at the cholesterol measurement wave and retrieved from the RAND HRS Longitudinal File (25).
Genetic Data
HRS began collecting genetic data from respondents in 2006. Respondents provided saliva samples after reading and signing a consent form during an enhanced face-to-face interview. Details of the genotype collection and quality control can be found elsewhere (26). Genotyping was conducted by the Center for Inherited Disease Research using the Illumina HumanOmini2.5 BeadChip. Genotype data that passed initial quality control were released and analyzed by the Quality Assurance/Quality Control analysis team at the University of Washington. Raw genetic data on unrelated individuals, both genotyped and imputed to the 1,000 Genomes Project, is available from the National Center for Biotechnology Information's database of genotypes and phenotypes (dbGaP Study Accession: phs000428.v2.p2).
Genetic ancestry in HRS was identified through the union of self-reported race/ethnicity and principal component (PC) analysis on genome-wide single nucleotide polymorphisms (SNP) calculated across all participants plus HapMap controls (26). Participants who were self-identified non-Hispanic White and fell within the European ancestry genetic PC cluster were included in the European ancestry sample. Participants who self-identified as non-Hispanic Black and fell within the African ancestry genetic PC cluster were included in the African ancestry sample. The HRS releases ancestry-specific PCs created within each ancestry sample (27).
We used a polygenic score (PGS) constructed by the HRS as the instrumental variable in our Mendelian randomization analyses. A PGS is a weighted sum of cumulative genetic risk for a trait, which aggregates multiple individual loci across the human genome and weights them by effect sizes from a prior GWAS meta-analysis (27). The HDL-C and TC PGSs were created using weights from a 2013 GWAS by the Global Lipid Genetics Consortium (9). The general cognition PGS was created using weights from a 2015 GWAS by the Cohorts for Heart and Aging Research in Genomic Epidemiology consortium (28). All the PGSs were standardized (mean = 0, standard deviation = 1) within ancestry. We included APOE-ε4 allele carrier status along with the general cognition PGS in our sensitivity analyses as a precision variable to reduce standard errors in the regression models and to capture the large genetic component of dementia attributed to the APOE-ε4 variant. A binary variable of APOE-ε4 allele carrier (having at least one copy of the ε4 allele, yes/no), was retrieved from the HRS genetic data imputed to the worldwide 1,000 Genomes Project reference panel (phase I) (29). A promoter variant (rs3764261[A]) on the cholesteryl ester transfer protein (CETP) gene is consistently related to the metabolism of HDL-C (30). We retrieved the number of copies of this SNP from the HRS measured genotype files for sensitivity analyses.
Statistical Analysis
Distributions of baseline characteristics were compared between included and excluded samples, ancestry, and across exposure (cholesterol level) and outcome (cognitive status) groups. χ2 test and analysis of variance were used as appropriate to examine the homogeneity across groups. We used linear regressions to examine the associations between cholesterol PGSs and other covariates.
In our main analyses (European ancestry sample), we used multivariable logistic regressions and Wald-type ratio estimators to test the associations and inferred causality between dyslipidemia and cognitive status, using cholesterol PGSs as instrumental variables. Results from the Mendelian randomization and multivariable logistic regression were compared using a test of interaction to evaluate heterogeneity (31). Normal cognitive status, normal HDL-C levels, and normal TC levels were treated as reference groups. Our primary models were adjusted for age, sex, years of education, lipid-lowering medication, cholesterol measurement wave, and five ancestry-specific PCs. Additional adjustment included health risk factors that were associated with blood cholesterol levels. We also added APOE-ε4 allele carrier and general cognition PGS in our sensitivity analyses as further potential confounders of the association between lipid profile and dementia risk. We investigated continuous cognitive domain scores as outcomes with linear regression models to understand the associations between cholesterol levels and different cognitive domains.
Sensitivity Analyses
To assess the robustness of our findings, we performed several analyses. Because only the relevance assumption of Mendelian randomization is testable (32, 33), we performed improvement χ2 tests to evaluate the strength of the instrument, with values >10 being taken as evidence for strong instruments (34). We checked for pleiotropy to tentatively assess the violation of independence and exclusion restriction assumptions. Hence, we examined the associations between HDL or TC PGS and potential confounding factors using linear regression, as well as the associations between general cognition PGS and HDL PGS or TC PGS, adjusted for five-ancestry-specific PCs. To control for pleiotropy, we replaced the PGS with an indicator variable of variants in the CETP SNP (0/1/2 copies of risk allele[A]) as an instrumental variable. As a negative control, we also conducted analyses in subsets of individuals with normal HDL-C or TC levels who were not using any lipid-lowering medication.
We checked for non-linear associations between dyslipidemia and cognitive status by using a smooth function of continuous cholesterol. We examined the association between TC/HDL-C ratio (logarithmic transformed) and cognitive status. Finally, all analyses were additionally conducted in an African ancestry sample.
We reported odds ratios (OR) for logistic regression and coefficients for linear regression along with their 95% confidence intervals (CIs). Population attributable fractions were also calculated for significant associations. We considered P-value <0.05 for statistical significance if not specified. All analyses were carried out separately by genetic ancestry and adjusted for a set of five ancestry-specific PCs to adjust for population stratification. A heuristic model and study subsets are shown in Figure 1. Analyses were performed in R statistical software (version 3.6.1) (35). Code to produce all analyses in this manuscript are available online (https://github.com/bakulskilab).
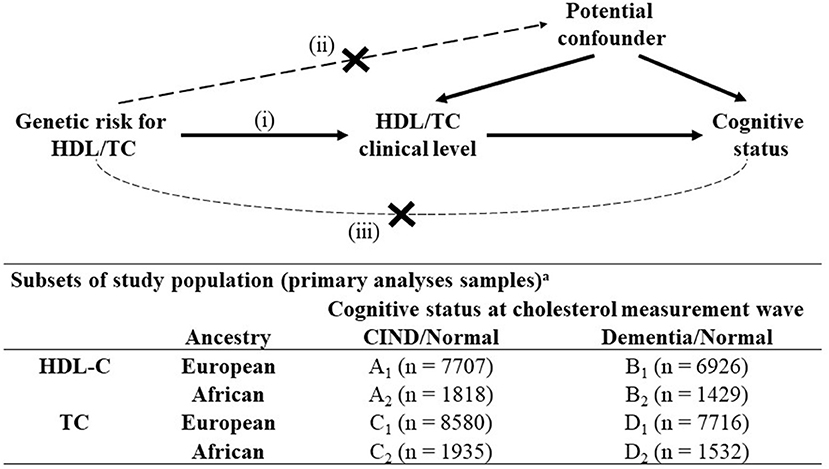
Figure 1. Mendelian randomization analyses structure and subsets of study population, Health and Retirement Study, (n = 10,882)a. CIND, Cognitive Impairment-Non-Dementia; HDL-C, High Density Lipoprotein Cholesterol; TC, Total Cholesterol. All variables were measured at the first instance of biomarker collection for a participant from Health and Retirement Study waves 2006–2012. Mendelian randomization assumptions: (i) the genetic variant must be associated with the exposure, (ii) the genetic variant must not be associated with any confounder of the exposure-outcome association and (iii) the genetic variant must be associated with the outcome only via the exposure. aSubsets used in standard logistic regression and Mendelian randomization analyses: participants with (A) non-missing values in HDL level + CIND or normal cognitive status; (B) non-missing values in HDL level + dementia or normal cognitive status; (C) non-missing values in TC level + CIND or normal cognitive status; (D) non-missing values in TC level + dementia or normal cognitive status.
Results
There were 10,882 participants included in our analytic sample. Compared to the excluded sample (n = 7,818), our included sample was older, more educated, more likely to have normal TC levels, and had better cognition (Supplementary Table 1). Among the included sample, the European and African ancestry groups had similar distributions of HDL-C and TC levels (Table 2). Participants in the European ancestry sample were older, more educated, performed better in cognition tests, and less likely to have chronic health conditions, relative to the African ancestry sample.
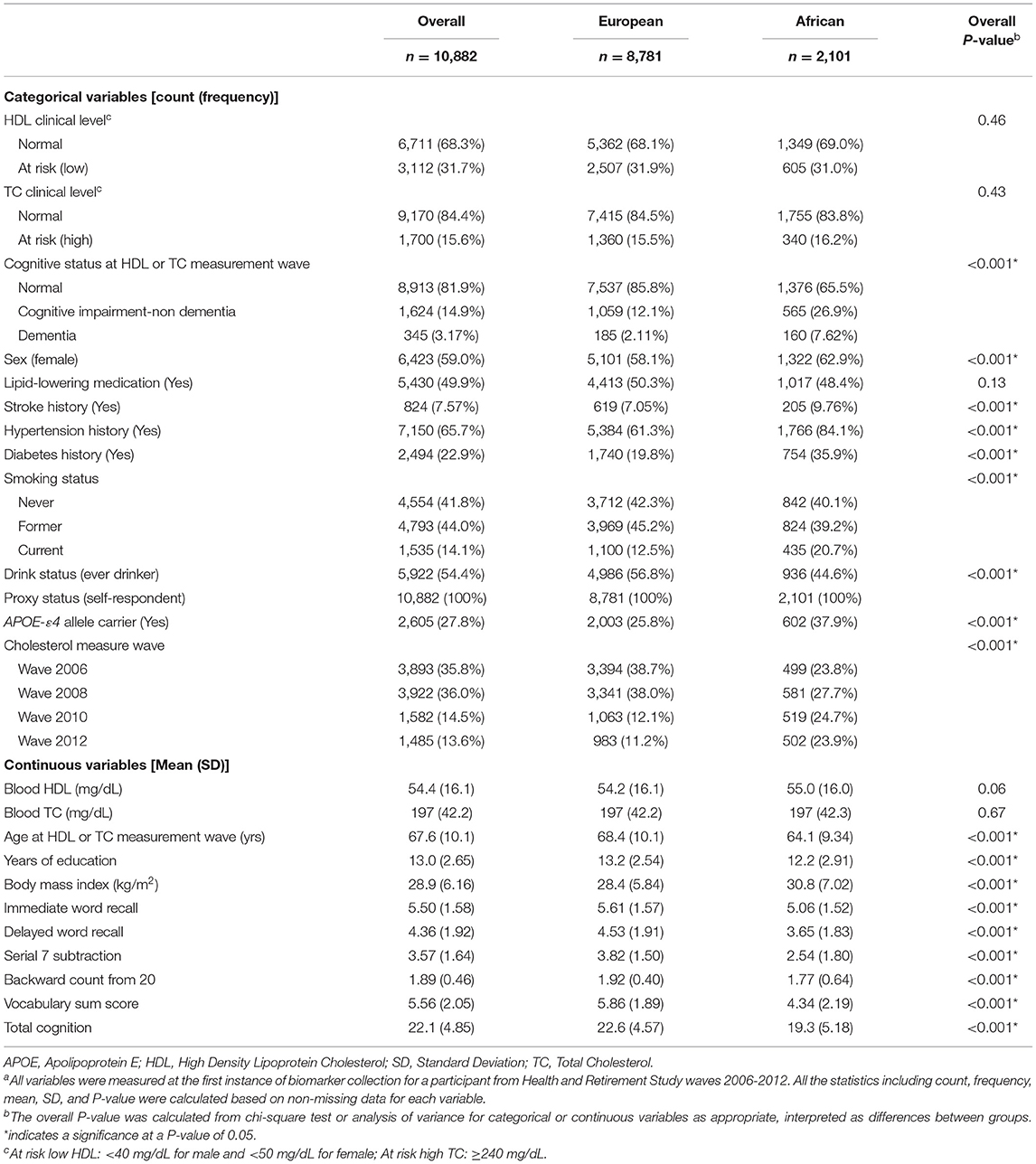
Table 2. Characteristics of sample participants (n = 10,882) stratified by ancestry, in the Health and Retirement Studya.
In the European ancestry sample (n = 8,781), health status including BMI, smoking, alcohol consumption, histories of stroke, hypertension, and diabetes were associated with both outcome and exposures. Thus, in our sensitivity models, we additionally adjusted for these variables. The average exercise level was also associated with cognitive impairment. But because it was correlated with BMI (r = −0.17, p < 0.001), hypertension (r = −0.12, p < 0.001), and diabetes (r = −0.16, p < 0.001), and may also be subject to reverse causation, we left those variables out for our analyses. Cognitive status was positively associated with the APOE-ε4 allele carrier status and general cognition PGS (Table 3).
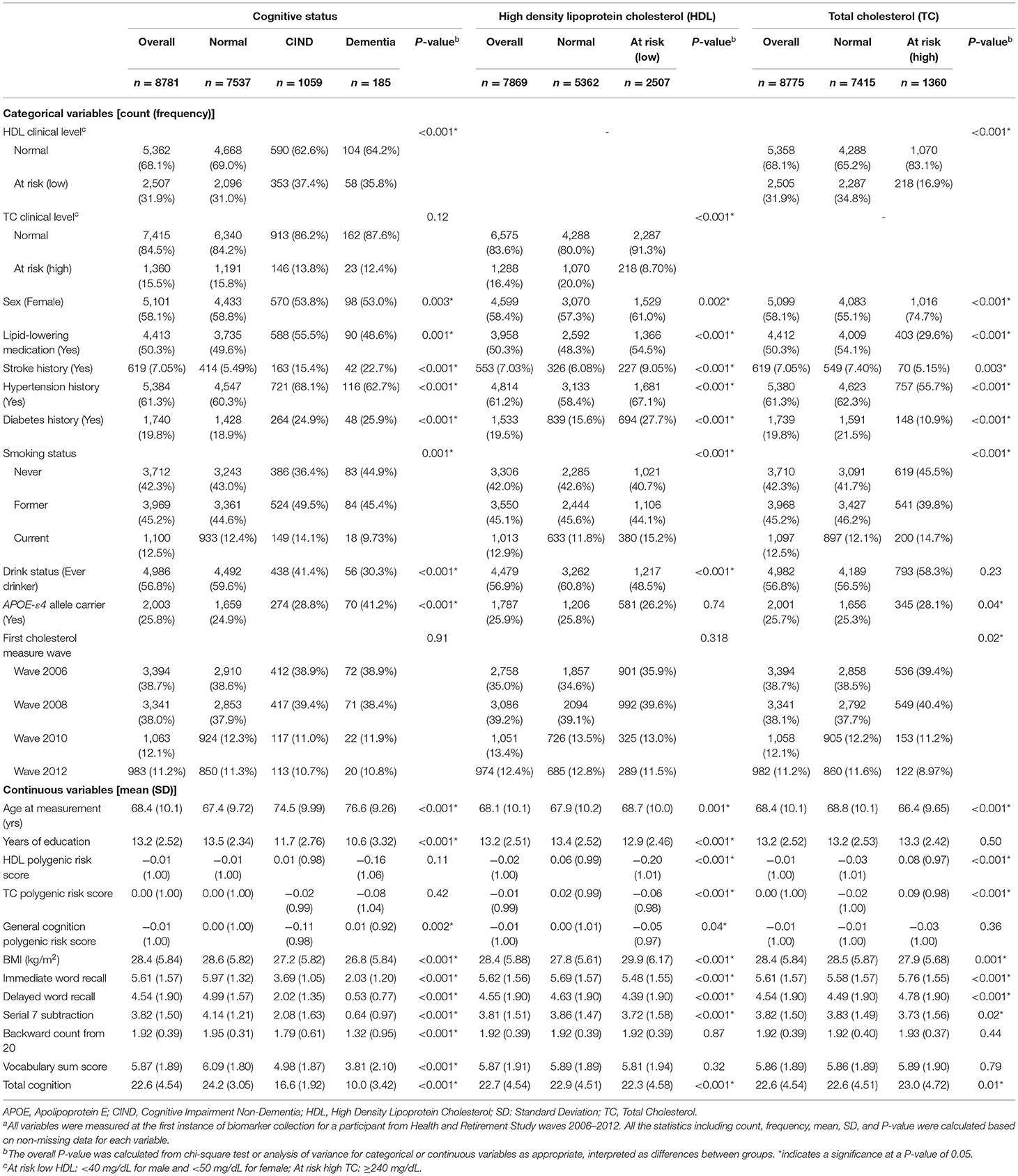
Table 3. Bivariate characteristics stratified by cognitive status or cholesterol clinical level, in the Health and Retirement Study, European ancestry sample (n = 8781)a.
Associations With Cumulative Genetic Risk of Dyslipidemia
In the European ancestry sample, cholesterol PGSs were highly associated with their corresponding blood cholesterol measures. After adjusting for age, sex, years of education, lipid-lowering medication, cholesterol measurement wave, and five ancestry-specific PCs, a one standard deviation increase in HDL PGS was associated with 0.78 (95% CI: 0.74, 0.82) lower odds of at risk relative to normal HDL-C level; a one standard deviation unit increase in TC PGS was associated with 1.21 (95% CI: 1.13, 1.29) times odds of at risk relative to normal TC level. Improvement χ2 test statistics confirmed both cholesterol PGSs as valid instruments for blood cholesterol levels in our sample (HDL PGS: χ2 = 98.7; TC PGS: χ2 = 32.6) (Table 4).
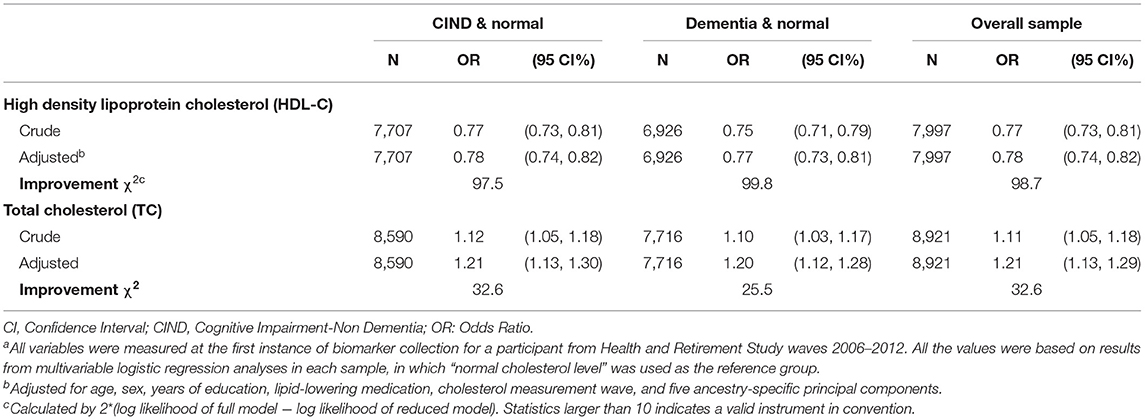
Table 4. Associations between polygenic risk score for cholesterol (HDL-C and TC) and blood cholesterol levels, in the Health and Retirement Study, European ancestry sample (n = 8,781)a.
In the European ancestry sample, HDL PGS was not associated with CIND but was associated with dementia (Table 5). After adjusting for age, sex, years of education, lipid-lowering medication, cholesterol measurement wave, and five ancestry-specific PCs, a one standard deviation increase in HDL PGS was associated with 0.81 (95% CI: 0.69, 0.96) lower odds of dementia relative to normal cognition. This association attenuated after additional adjustments of health status (Table 5). TC PGS was not directly or indirectly associated with the odds of CIND or dementia. No association was observed between HDL or TC PGS and any cognitive domain score (Supplementary Table 2).
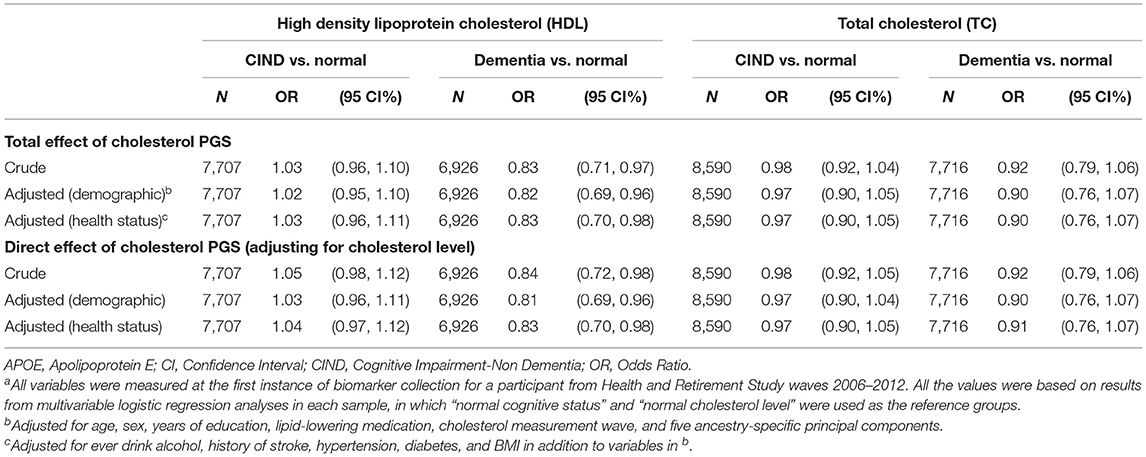
Table 5. Associations between polygenic risk score for cholesterol and cognitive status, in the Health and Retirement Study, European ancestry sample (n = 8,781)a.
Figure 2 presents the associations of HDL or TC PGSs with baseline characteristics in the European ancestry sample. Both cholesterol PGSs were associated with lipid-lowering medication usage and alcohol consumption. In addition, HDL PGS was also associated with history of hypertension, diabetes, smoking status, BMI, and years of education.
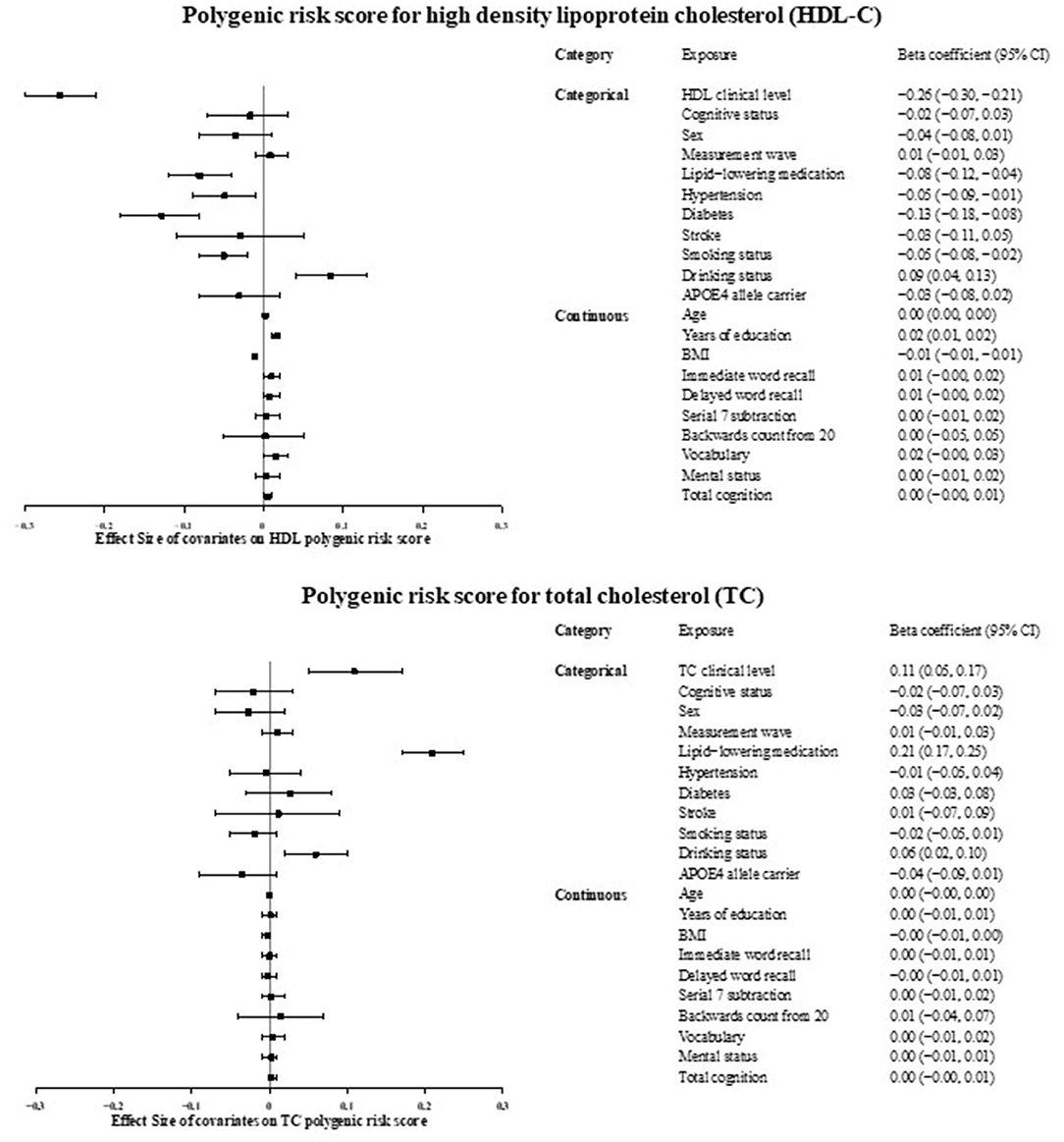
Figure 2. Associations between cholesterol polygenic risk score and factors potentially confounding the relation between cholesterol and cognitive status, in the Health and Retirement Study, European ancestry sample (n = 8,781). APOE, Apolipoprotein E; BMI, Body Mass Index; CI, Confidence Interval; HDL, High Density Lipoprotein Cholesterol; TC, Total Cholesterol.
Associations Between HDL-C Levels and Cognition
Multivariable logistic regression results showed at risk (low) HDL-C levels were associated with increased odds of CIND (Table 6). According to population attributable fraction results, 5% of the CIND cases were attributed to at risk (low) HDL-C levels. In the primary adjusted model, those with at risk (low) HDL-C levels had 1.20 (95% CI: 1.03, 1.40) times higher odds of CIND relative to normal cognition. This association attenuated to the null after additional adjustments for health status. No associations were observed between HDL-C levels and dementia.
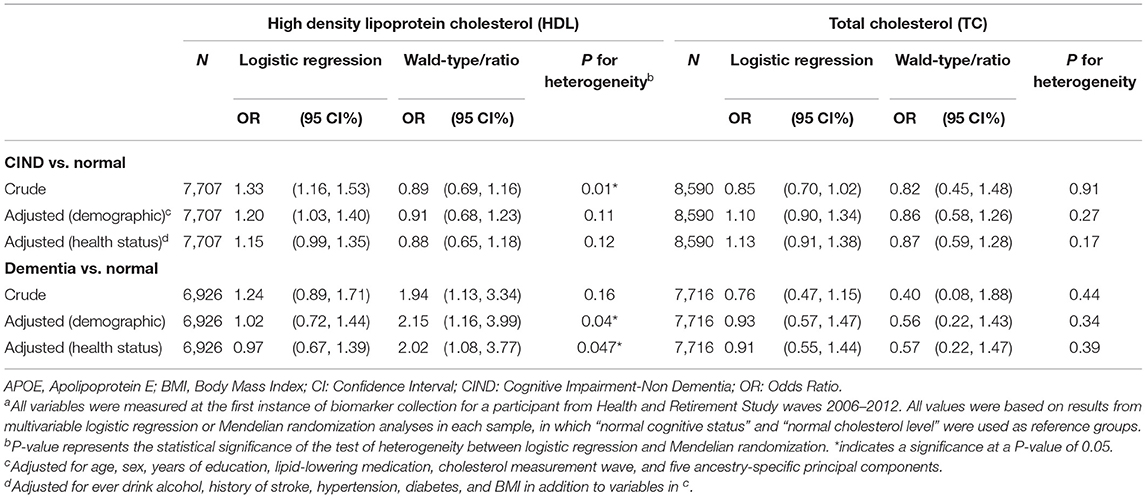
Table 6. Associations between blood cholesterol levels and cognitive status, in the Health and Retirement Study, European ancestry sample (n = 8781)a.
Mendelian randomization analyses showed no evidence of inferred causality between HDL-C levels and CIND (OR = 0.92, 95% CI: 0.69, 1.23; Table 6), despite significant logistic associations observed between HDL-C levels and CIND. However, an inferred causal relationship was observed between HDL-C levels and dementia. According to the Wald-type/ratio results, using HDL PGS as an instrumental variable, individuals with at risk (low) HDL-C levels had 2.15 (95% CI: 1.16, 3.99) times higher odds of dementia relative to normal cognition. This Mendelian randomization result was significantly different from the multivariable logistic regression result (P for heterogeneity = 0.04).
For specific cognitive domains, at risk (low) HDL-C levels were associated with worse performance in word recall tests (episodic memory) only in multivariable regression (Table 7). No association was observed between HDL-C levels and mental status or vocabulary scores. None of the associations between HDL-C levels and cognitive domain score could be considered causal through Mendelian randomization analyses.
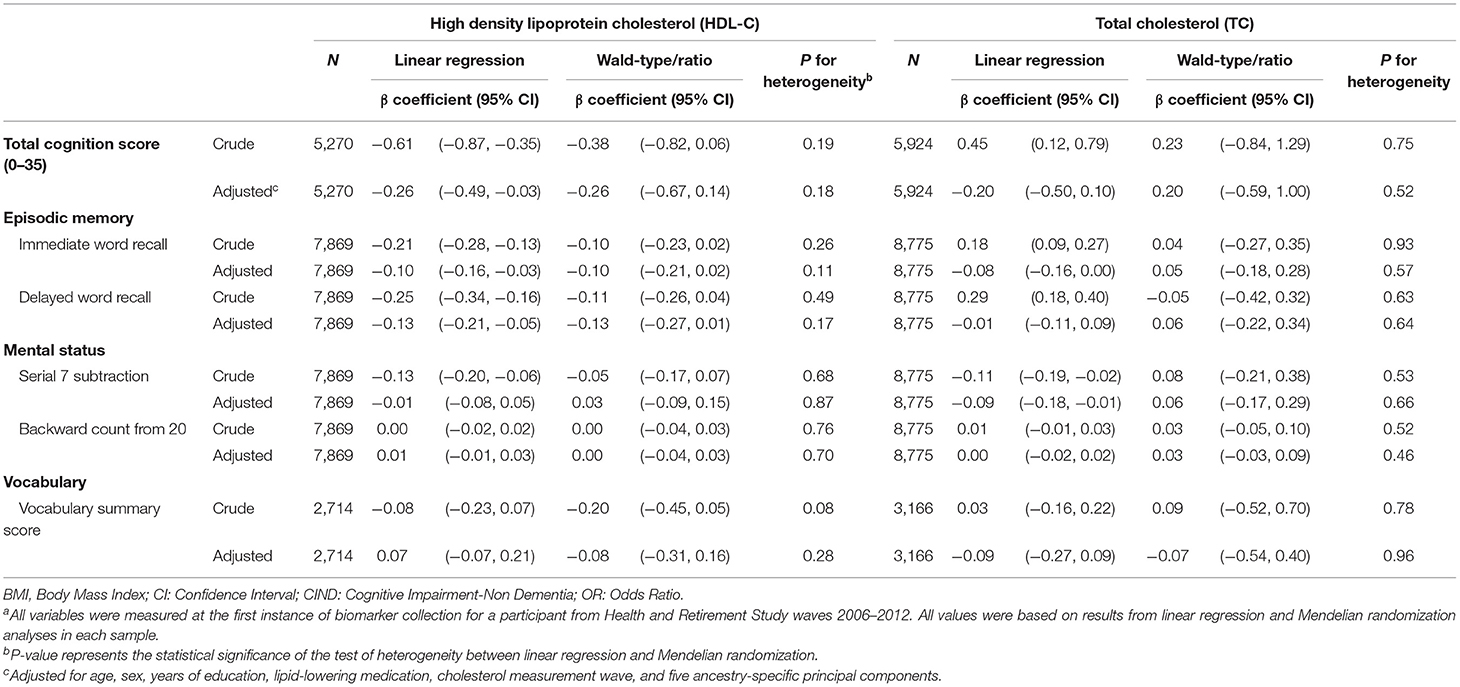
Table 7. Associations between blood cholesterol levels and cognitive domain scores, in the Health and Retirement Study, European ancestry sample (n = 8781)a.
Associations Between TC Levels and Cognition
Neither significant logistic regression associations nor causal inference were observed between TC levels and cognitive status in either multivariable logistic regression or Mendelian randomization analyses. Results did not differ between the logistic regression and Mendelian randomization estimates (P for heterogeneity: CIND/normal = 0.27; dementia/normal = 0.34) (Table 6). For specific cognitive domains, no association or causal relationship was observed between TC levels and any cognitive domain score (Table 7).
Sensitivity Analyses
In the European ancestry sample, after adjusting for five ancestry-specific PCs, general cognition PGS was not associated with HDL PGS ( = −0.02, P = 0.08), but was associated with TC PGS ( = −0.04, P = 0.005). Multivariable logistic regression and Mendelian randomization results were similar when we further adjusted for dementia genetic variables (APOE-ε4 allele carrier status and general cognition PGS).
Given that HDL PGS was associated with multiple baseline characteristics (Figure 2), there may be pleiotropic effects between cognition and blood HDL-C levels. To control for this potential pleiotropy, we used rs3764261 as a valid instrumental variable, but no causal relationship was found as above (Supplementary Table 3). In the subsample of participants with normal HDL-C and TC levels and not using any lipid-lowering medication, neither HDL PGS nor TC PGS was associated with cognitive status, showing lack of evidence for the violation of the independence assumption in our analysis (Supplementary Table 4). No association was observed between the log-transformed TC/HDL-C ratio on cognitive status (Supplementary Table 5).
Supplementary Figure 2 shows non-linear associations between continuous cholesterol and cognitive status, adjusting for age, sex, years of education, lipid-lowering medication, cholesterol measurement wave, and five ancestry-specific PCs. The probability of predicted CIND and dementia both decreased with increased HDL-C, which were consistent with our previous findings that low HDL-C level was associated with cognitive impairment. For TC, we observed an expected non-linear association with CIND and dementia: before the clinical cut point of 240 mg/dL, the probability of predicted CIND and dementia both decreases with the increase of TC concentration, while the probability increased after the cut point.
Among the African ancestry sample (n = 2,101), HDL and TC PGSs were also associated with blood HDL-C and TC levels, respectively; while improvement χ2 test statistics indicated neither cholesterol PGSs were valid instruments (HDL: 4.96 < 10; TC: 9.09 < 10), so no further Mendelian randomization analyses were performed (Supplementary Table 6a). In multivariable logistic regression, no associations were observed between HDL or TC polygenic score and CIND or dementia (Supplementary Table 6b, Model 1), or between HDL-C or TC level and cognitive status (Supplementary Table 6b, Model 2).
Discussion
The present study was conducted among a sample of older adults from the Health and Retirement Study participating in the 2006–2012 waves with biomarker and cognition data. To our knowledge, this is a novel study using a Mendelian randomization framework to investigate the inferred causal nature of dyslipidemia on cognitive status and separate cognitive domains. In the European ancestry sample, after adjusting for age, sex, years of education, lipid-lowing medication, cholesterol measurement wave, and five ancestry-specific PCs, at risk (low) HDL-C levels were associated with higher odds of CIND, lower episodic memory scores, and lower mental status scores. Using inverse-variance weighted Mendelian randomization methods, at risk (low) HDL-C level was inferred to be causally associated with dementia (OR = 2.25, 95% CI: 1.23, 4.09). No associations or causal inferences were observed between TC levels and cognitive status. These findings represent important new information supporting HDL-C management as a public health approach to prevent dementia.
Our observed associations between at risk (low) HDL-C levels and cognitive impairment in the European ancestry sample are consistent with previous studies (36, 37). HDL-C is involved in the deposition and clearance of beta-amyloid – a determining factor for endothelial inflammation and subsequent neurodegeneration in the brain (38). Animal models show a protective effect of HDL-C against memory deficits, neuroinflammation, and cerebral amyloid angiopathy (39). Several longitudinal studies also found a potential protective effect of midlife HDL-C on future cognitive decline (40, 41). For specific cognitive domains, at risk HDL-C and TC were both associated with worse episodic memory only, which suggests that dysregulation of lipid metabolism might be associated with certain cognitive functions. However, we were not able to identify specific neuropsychiatric or neuropathological mechanisms in this study. We did not observe an association between TC and cognitive status in either ancestry sample, which differs from some existing findings. For example, a prior meta-analysis reported adults with high TC had 2.14 times higher risk of developing dementia compared to those with normal TC (3). The difference may be attributed to variations across samples, in that our included study sample captured a lower proportion of abnormal TC participants (Supplementary Table 1). Additionally, we hypothesize that the lack of association between TC and dementia in our European sample may be due to a physiological decline of TC circulating levels in older adults. Studies have noted that TC may not be an adequate marker to characterize lifetime exposure to dyslipidemia or atherogenic risk due to the fact that TC levels decline with age (42, 43). However, HDL-C circulating levels are relatively stable over time and may be a better indicator of atherogenic risk among older adults (44). Therefore, our null causal inferred association between TC and dementia may correspond to a physiological decline of TC levels due to aging; and hence, a misclassification of the lifetime atherogenic risk. In contrast, the non-null association between HDL-C levels and cognitive impairment may be a reflection of more stable circulating levels of this lipoprotein in older adults.
It is worth noticing that in our study of older adults, we only observed significant associations between HDL-C and CIND, but not with dementia. One possible explanation on that is that in our sensitivity analyses, we adjusted for potential mediators, which could be part of the causal chain between the cholesterol level and cognitive impairment. Adjusting for potential mediators can bias those associations (45). The attenuated association between HDL-C and CIND after additional adjustments for health conditions also supports this assumption (Table 6).
By contrast, the causal inference was only observed for at risk (low) HDL-C levels with dementia. No causal relationships were observed between HDL-C levels and CIND or any cognitive domain scores, including episodic memory. We were limited to cross-sectional HDL-C measurements and were unable to capture repeated cholesterol measures prior to impaired cognition or cumulative lifetime HDL-C levels. It is possible that the polygenic score for HDL is a better proxy for lifetime HDL-C levels because genetic risk is established early in life and maintained throughout the life course. Under this scenario, a lower polygenic score for HDL might be a marker of decreased lifetime HDL-C levels, which could increase the risk of dementia in later life.
To assess the validity of our Mendelian randomization models, several tests were performed to evaluate the assumptions of the testing framework. The relevance assumption is met by providing evidence of both cholesterol PGSs as valid instruments using improvement χ2 tests. A limitation of our analysis is the potential violation of the independence and exclusion restriction assumptions. Even though we tested for potential violations of pleiotropy, implemented negative controls, and as a sensitivity analysis used the SNP rs3764261 as a valid instrumental variable, our study cannot rule out the presence of pleiotropy. As such, the inferred causality between HDL-C and cognitive status should be interpreted with caution.
No association was found between cholesterol levels and cognitive status in the African ancestry sample. African ancestry participants are traditionally underrepresented in genetic research. Because the method for computing the cholesterol PGSs depended on summary statistics from GWASs focused exclusively on participants of the European ancestry, results may have limited generalizability to other ancestral groups (46). Furthermore, we had a relatively small sample of the African ancestry (nAfrican = 2,101 vs. nEuropean = 8,781). A similar study should be replicated in an African ancestry with a larger sample size and PGSs based on GWASs among the African ancestry specifically. Previous studies have found that African American men and women have better lipid profiles than their White counterparts, including higher levels of HDL-C, lower levels of LDL-C, TC, and triglycerides (TG) (47, 48). Despite this beneficial lipid profile, African Americans, as a collective group, are at greater risk for chronic conditions that are physiologically related to an unfavorable lipid imbalance, such as: coronary heart disease (49), stroke (50), and dementia (3). In our sample, participants of African ancestry have comparable lipid profiles to those with European ancestry. However, our results indicate that an adverse lipid profile is not predictive of dementia nor CIND among African ancestry participants. Therefore, future research should focus on understanding and identifying clinical biomarkers of cognitive decline for participants with non-European ancestry.
There are several strengths and limitations in our current study. First, studies examining blood cholesterol levels and cognitive function in the context of genetics are limited, and thus our findings contribute to an important, yet sparse, literature. Second, Mendelian randomization has a powerful control for confounding and reverse causation, which often impede or mislead epidemiological studies of causation (51). We used summary scores (PGSs) of cumulative variation across multiple genetic loci to capture a larger fraction of variability of blood cholesterol, and thus increased the power of testing. The lack of data on other lipid markers such as LDL-C and triglycerides (TG) is an important limitation of our study. We do not know to what extent the exclusion of these two markers may affect the robustness of our results, as LDL-C and TG are important atherogenic risk factors often associated with stroke and vascular dementia. To address this limitation, we implemented in our sensitivity analysis a ratio measure (TC/HDL-C) which may be a measure with better atherogenic predictive power (18, 52, 53). However, using the ratio measure, our results did not substantively change. Further, studies with a more complete lipid panel data are warranted. Our findings should be considered in light of potential selection bias. Individuals with dyslipidemia may die prematurely from dyslipidemia-related diseases, such as cardiovascular diseases, before developing dementia. Thus, our sample may have been biased toward healthier individuals – those that survived – which may not be representative in the general older population. In such a case, our estimates are conservative and represent an underestimation of the true causal odds ratio. In this study, we were able to broadly classify dementia status. Additional studies will be able to examine dementia subtypes for specificity of associations. Furthermore, although, the dementia and CIND cut points have been clinically validated with an estimated sensitivity of 78% (20), the classification of cognitive status is not as well-defined as other more explicitly defined variables. However, our analysis with multiple domains of cognition suggests that our main finding of a potential causal association between HDL-C and dementia among participants of European ancestry may be robust. We argue that any potential misclassification of dementia by the Langa-Weir cognitive status' algorithm is non-differential by HDL-C status. Therefore, our estimates are conservative and biased toward the null. Finally, we used concurrent assessments of cholesterol and cognition in our current study, but measurements of early-life cholesterol level were not available. Results of causal tests could be strengthened by further studies with time-specific variables. Similar studies should be replicated with larger sample sizes and in multiple ancestries to promote generalizability.
Dementia is a major public health concern worldwide. Blood cholesterol levels, unlike genetic factors, can be modified by changing diets and health behaviors. Findings in our study underscore the protective effects of increased blood HDL-C and its role in maintaining cognition during aging. Thus, therapeutic strategies aimed at controlling cholesterol levels could be a converging target to mitigate cognitive deficits.
Data Availability Statement
Publicly available datasets were analyzed in this study. This data can be found here: Phenotype and covariate data are publicly available through the Health and Retirement Study, public use dataset. Produced and distributed by the University of Michigan with funding from the National Institute on Aging (grant number NIA U01AG009740). https://hrs.isr.umich.edu/data-products. The data/analyses presented in the current publication have been deposited in and are available from the dbGaP database under dbGaP accession phs000428.v2.p2.
Ethics Statement
The Health and Retirement Study is sponsored by the National Institute on Aging (NIA U01AG009740) and is conducted by the University of Michigan, where informed consent was approved by the Institutional Review Board. This analysis was exempt and not regulated as determined by the Institutional Review Board at the University of Michigan (HUM00128220). The patients/participants provided their written informed consent to participate in this study.
Author Contributions
MF, KB, and EW: conceived and designed the analysis plan. MF: managed the dataset and analyzed the data. CH: shadow analysis and code review. MF, KB, EW, and CH: contributed to the interpretation and writing and editing of the manuscript. KB and EW: provided funding and resources. All authors contributed to the article and approved the submitted version.
Funding
All authors were supported by grants from the National Institute on Aging (R01 AG055406 and R01 AG067592). KB was supported by grants from the National Institute for Environmental Health Sciences and the National Institute for Minority Health and Health Disparities (R01 ES025531; R01 ES025574; and R01 MD013299). EW was supported by a grant from the National Institute on Aging (R01 AG055654). This work was supported by the Michigan Center for the Demography of Aging (P30 AG012846) and the Michigan Alzheimer's Disease Center (P30 AG053760). The funders did not have any role in the design, analysis, interpretation, or writing of the manuscript.
Conflict of Interest
The authors declare that the research was conducted in the absence of any commercial or financial relationships that could be construed as a potential conflict of interest.
Acknowledgments
The authors would like to thank the participants of the Health and Retirement Study. We would additionally like to thank the scientific community for helpful comments on our medRxiv posting.
Supplementary Material
The Supplementary Material for this article can be found online at: https://www.frontiersin.org/articles/10.3389/fneur.2021.660212/full#supplementary-material
Abbreviations
BMI, body mass index; CI, confidence intervals; CIND, cognitive impairment-non dementia; GWAS, genome-wide association study; HDL-C, high-density lipoprotein cholesterol; HRS, Health and Retirement Study; OR, odds ratios; PC, principal component; PGS, polygenic score; SNP, single nucleotide polymorphisms; TC, total cholesterol.
References
1. World Health Organization. Dementia. World Health Organization. Available online at: https://www.who.int/news-room/fact-sheets/detail/dementia (accessed September 27, 2019).
2. Statista. Dementia Treatment Cost Forecast Globally 2018-2030. Statista. Available online at: https://www.statista.com/statistics/471323/global-dementia-economic-impact-forecast/ (accessed July 20, 2020).
3. Anstey KJ, Ashby-Mitchell K, Peters R. Updating the evidence on the association between serum cholesterol and risk of late-life dementia: review and meta-Analysis. J Alzheimers Dis. (2017) 56:215–28. doi: 10.3233/JAD-160826
4. Komulainen P, Lakka TA, Kivipelto M, Hassinen M, Helkala EL, Haapala I, et al. Metabolic syndrome and cognitive function: a population-based follow-up study in elderly women. Dement Geriatr Cogn Disord. (2007) 23:29–34. doi: 10.1159/000096636
5. Yaffe K, Bahorik AL, Hoang TD, Forrester S, Jacobs DR Jr, Lewis CE, et al. Cardiovascular risk factors and accelerated cognitive decline in midlife: the CARDIA study. Neurology. (2020) 95:e839–46. doi: 10.1212/WNL.0000000000010078
6. Koch M, Jensen MK. HDL-cholesterol and apolipoproteins in relation to dementia. Curr Opin Lipidol. (2016) 27:76–87. doi: 10.1097/MOL.0000000000000257
7. Kivipelto M, Helkala EL, Laakso MP, Hänninen T, Hallikainen M, Alhainen K, et al. Apolipoprotein E epsilon4 allele, elevated midlife total cholesterol level, and high midlife systolic blood pressure are independent risk factors for late-life Alzheimer disease. Ann Intern Med. (2002) 137:149–55. doi: 10.7326/0003-4819-137-3-200208060-00006
8. Kathiresan S, Manning AK, Demissie S, D'Agostino RB, Surti A, Guiducci C, et al. A genome-wide association study for blood lipid phenotypes in the Framingham Heart Study. BMC Med Genet. (2007) 8(Suppl. 1):S17. doi: 10.1186/1471-2350-8-S1-S17
9. Willer CJ, Schmidt EM, Sengupta S, Peloso GM, Gustafsson S, Kanoni S, et al. Discovery and refinement of loci associated with lipid levels. Nat Genet. (2013) 45:1274–83. doi: 10.1038/ng.2797
10. HRS. HRS Data Book | Health and Retirement Study. Available online at: https://hrs.isr.umich.edu/about/data-book (accessed December 11, 2019).
11. Bullain SS, Corrada MM. Dementia in the oldest old. Continuum. (2013) 19(2 Dementia):457–69. doi: 10.1212/01.CON.0000429172.27815.3f
12. Crimmins EM, Faul JD, Kim JK, Guyer HM, Langa KM, Ofstedal MB, et al. Documentation of Biomarkers in the 2006 and 2008 Health and Retirement Study. Ann Arbor, MI: Institute for Social Research, University of Michigan (2013). doi: 10.7826/ISR-UM.06.585031.001.05.0018.2009
13. Crimmins EM, Faul JD, Kim JK, Weir DR. Documentation of Biomarkers in the 2010 and 2012 Health and Retirement Study. Ann Arbor, MI: Survey Research Center, University of Michigan (2015).
14. Crimmins E, Kim JK, McCreath H, Faul J, Weir D, Seeman T. Validation of blood-based assays using dried blood spots for use in large population studies. Biodemography Soc Biol. (2014) 60:38–48. doi: 10.1080/19485565.2014.901885
15. HRS. Sensitive Health Data | Health and Retirement Study. Available online at: http://hrsonline.isr.umich.edu/index.php?p=healthdat&_ga=2.253318798.625031607.1576100757-1101446680.1569101058 (accessed December 13, 2019).
16. HRS. Data Product Detail | Health and Retirement Study. Available online at: http://hrsonline.isr.umich.edu/index.php?p=shoavail&iyear=XV (accessed December 13, 2019).
17. Grundy SM, Stone NJ, Bailey AL, Beam C, Birtcher KK, Blumenthal RS, et al. 2018 AHA/ACC/AACVPR/AAPA/ABC/ACPM/ADA/AGS/APhA/ASPC/NLA/PCNA guideline on the management of blood cholesterol: a report of the American College of Cardiology/American Heart Association Task Force on Clinical Practice Guidelines. Circulation. (2019) 139:e1182–6. doi: 10.1161/CIR.0000000000000698
18. Millán J, Pintó X, Muñoz A, Zúñiga M, Rubiés-Prat J, Pallardo LF, et al. Lipoprotein ratios: physiological significance and clinical usefulness in cardiovascular prevention. Vasc Health Risk Manag. (2009) 5:757–65. doi: 10.2147/VHRM.S6269
19. Ofstedal MB, Fisher G. Documentation of Cognitive Functioning Measures in the Health and Retirement Study. Ann Arbor, MI: Institute for Social Research, University of Michigan (2005) doi: 10.7826/ISR-UM.06.585031.001.05.0010.2005
20. Crimmins EM, Kim JK, Langa KM, Weir DR. Assessment of cognition using surveys and neuropsychological assessment: the health and retirement study and the aging, demographics, and memory study. J Gerontol B Psychol Sci Soc Sci. (2011) 66B(Suppl. 1):i162–71. doi: 10.1093/geronb/gbr048
21. Leritz EC, McGlinchey RE, Salat DH, Milberg WP. Elevated levels of serum cholesterol are associated with better performance on tasks of episodic memory. Metab Brain Dis. (2016) 31:465–73. doi: 10.1007/s11011-016-9797-y
22. McArdle JJ, Fisher GG, Kadlec KM. Latent variable analyses of age trends of cognition in the health and retirement study, 1992-2004. Psychol Aging. (2007) 22:525–45. doi: 10.1037/0882-7974.22.3.525
23. Ofstedal MB, Fisher GG, Herzog AR. Documentation of Cognitive Functioning Measures in the Health and Retirement Study. Institute for Social Research, University of Michigan (2005). Available online at: http://hrsonline.isr.umich.edu/sitedocs/userg/dr-006.pdf (accessed November 1, 2020).
24. Fisher G, Hassan H, Faul J, Rogers W, Weir D. Health and Retirement Study Imputation of Cognitive Functioning Measures: 1992-2014. (2017). Ann Arbor, MI: Institute for Social Research, University of Michigan. Available online at: https://hrs.isr.umich.edu/sites/default/files/biblio/COGIMPdd.pdf
25. Monica 1776 Main Street Santa, California 90401-3208. RAND HRS Longitudinal File. (2016). (V1). Available online at: https://www.rand.org/well-being/social-and-behavioral-policy/centers/aging/dataprod/hrs-data.html (accessed October 22, 2019).
26. David RW. Quality Control Report for Genotypic Data. (2013). Available online at: http://hrsonline.isr.umich.edu/sitedocs/genetics/HRS2_qc_report_SEPT2013.pdf?_ga=2.160262008.498457094.1571249480-1101446680.1569101058 (accessed November 1, 2020).
27. Ware EB, Schmitz LL, Gard A, Faul J. HRS Polygenic Scores 2006-2012 Genetic Data - Release 3 | Health and Retirement Study. Available online at: https://hrs.isr.umich.edu/news/hrs-polygenic-scores-2006-2012-genetic-data-release-3 (accessed October 18, 2019).
28. Davies G, Armstrong N, Bis JC, Bressler J, Chouraki V, Giddaluru S, et al. Genetic contributions to variation in general cognitive function: a meta-analysis of genome-wide association studies in the CHARGE consortium (N=53949). Mol Psychiatry. (2015) 20:183–92. doi: 10.1038/mp.2014.188
29. Health and Retirement Study, Combined Phases 1-3 Imputation Report - 1000 Genomes Reference Panel. (2013). Available online at: https://hrsonline.isr.umich.edu/sitedocs/genetics/1000G_IMPUTE2report_HRS2_2006_2008_2010.pdf?_ga=2.125105226.1552876390.1623162680-1324948855.1614619191&_ga=2.125105226.1552876390.1623162680-1324948855.1614619191
30. Altman DG, Bland JM. Interaction revisited: the difference between two estimates. BMJ. (2003) 326:219. doi: 10.1136/bmj.326.7382.219
31. Palmer TM, Sterne JAC, Harbord RM, Lawlor DA, Sheehan NA, Meng S, et al. Instrumental variable estimation of causal risk ratios and causal odds ratios in Mendelian randomization analyses. Am J Epidemiol. (2011) 173:1392–403. doi: 10.1093/aje/kwr026
32. Hernán MA, Robins JM. Instruments for causal inference: an epidemiologist's dream? Epidemiology. (2006) 17:360–. doi: 10.1097/01.ede.0000222409.00878.37
33. Davies NM, Holmes MV, Davey Smith G. Reading Mendelian randomisation studies: a guide, glossary, and checklist for clinicians. BMJ. (2018) 362:k601. doi: 10.1136/bmj.k601
34. Schierer A, Been LF, Ralhan S, Wander GS, Aston CE, Sanghera DK. Genetic variation in Cholesterol Ester Transfer Protein (CETP), Serum CETP activity, and Coronary Artery Disease (CAD) risk in Asian Indian diabetic cohort. Pharmacogenet Genomics. (2012) 22:95–104. doi: 10.1097/FPC.0b013e32834dc9ef
35. R. The R Project for Statistical Computing. Available online at: https://www.r-project.org/ (accessed October 18, 2019).
36. Sparks DL, Kryscio RJ, Connor DJ, Sabbagh MN, Sparks LM, Lin Y, et al. Cholesterol and cognitive performance in normal controls and the influence of elective statin use after conversion to mild cognitive impairment: results in a clinical trial cohort. NDD. (2010) 7:183–6. doi: 10.1159/000295660
37. Landi F, Russo A, Cesari M, Pahor M, Bernabei R, Onder G. HDL-cholesterol and physical performance: results from the ageing and longevity study in the sirente geographic area (ilSIRENTE Study). Age Ageing. (2007) 36:514–20. doi: 10.1093/ageing/afm105
38. Holtzman DM. Role of apoe/Abeta interactions in the pathogenesis of Alzheimer's disease and cerebral amyloid angiopathy. J Mol Neurosci. (2001) 17:147–55. doi: 10.1385/JMN:17:2:147
39. Button EB, Robert J, Caffrey TM, Fan J, Zhao W, Wellington CL. HDL from an Alzheimer's disease perspective. Curr Opin Lipidol. (2019) 30:224–34. doi: 10.1097/MOL.0000000000000604
40. Song F, Poljak A, Crawford J, Kochan NA, Wen W, Cameron B, et al. Plasma apolipoprotein levels are associated with cognitive status and decline in a community cohort of older individuals. PLoS ONE. (2012) 7:e34078. doi: 10.1371/journal.pone.0034078
41. Singh-Manoux A, Gimeno D, Kivimaki M, Brunner E, Marmot MG. Low HDL cholesterol is a risk factor for deficit and decline in memory in midlife: the Whitehall II study. Arterioscler Thromb Vasc Biol. (2008) 28:1556–62. doi: 10.1161/ATVBAHA.108.163998
42. Castelli WP, Garrison RJ, Wilson PWF, Abbott RD, Kalousdian S, Kannel WB. Incidence of coronary heart disease and lipoprotein cholesterol levels: the framingham study. JAMA. (1986) 256:2835–8.
43. Gordon T, Castelli WP, Hjortland MC, Kannel WB, Dawber TR. Predicting coronary heart disease in middle-aged and older persons: the framington study. JAMA. (1977) 238:497–9.
44. Wilson PW, Anderson KM, Harris T, Kannel WB, Castelli WP. Determinants of change in total cholesterol and HDL-C with age: the framingham study. J Gerontol. (1994) 49:M252–7.
45. Corraini P, Olsen M, Pedersen L, Dekkers OM, Vandenbroucke JP. Effect modification, interaction and mediation: an overview of theoretical insights for clinical investigators. Clin Epidemiol. (2017) 9:331–8. doi: 10.2147/CLEP.S129728
46. Martin AR, Gignoux CR, Walters RK, Wojcik GL, Neale BM, Gravel S, et al. Human demographic history impacts genetic risk prediction across diverse populations. Am J Hum Genet. (2017) 100:635–49. doi: 10.1016/j.ajhg.2017.03.004
47. Cook CB, Erdman DM, Ryan GJ, Greenlund KJ, Giles WH, Gallina DL, et al. The pattern of dyslipidemia among urban African-Americans with type 2 diabetes. Diabetes Care. (2000) 23:319. doi: 10.2337/diacare.23.3.319
48. Cowie CC, Howard BV, Harris MI. Serum lipoproteins in African Americans and whites with non-insulin-dependent diabetes in the US population. Circulation. (1994) 90:1185–93. doi: 10.1161/01.CIR.90.3.1185
49. Leigh JA, Alvarez M, Rodriguez CJ. Ethnic minorities and coronary heart disease: an update and future directions. Curr Atheroscler Rep. (2016) 18:9. doi: 10.1007/s11883-016-0559-4
50. Kleindorfer D. Sociodemographic groups at risk: race/ethnicity. Stroke. (2009) 40(Suppl. 1):S75–8. doi: 10.1161/STROKEAHA.108.534909
51. Olena Y. Mendelian Ramdomization. Available online at: https://www.ncbi.nlm.nih.gov/projects/gap/cgi-bin/study.cgi?study_id=phs000428.v2.p2 (accessed October 19, 2019).
52. Lemieux I, Lamarche B, Couillard C, Pascot A, Cantin B, Bergeron J, et al. Total cholesterol/HDL cholesterol ratio vs LDL cholesterol/HDL cholesterol ratio as indices of ischemic heart disease risk in men: the Quebec Cardiovascular Study. Arch Intern Med. (2001) 161:2685–92.
Keywords: blood cholesterol, polygenic score, dementia, Mendelian randomization, cognitive domain
Citation: Fu M, Bakulski KM, Higgins C and Ware EB (2021) Mendelian Randomization of Dyslipidemia on Cognitive Impairment Among Older Americans. Front. Neurol. 12:660212. doi: 10.3389/fneur.2021.660212
Received: 29 January 2021; Accepted: 24 May 2021;
Published: 23 June 2021.
Edited by:
Rosanna Tortelli, University College London, United KingdomReviewed by:
Judith Aharon Peretz, Rambam Health Care Campus, IsraelDavid Conversi, Sapienza University of Rome, Italy
Copyright © 2021 Fu, Bakulski, Higgins and Ware. This is an open-access article distributed under the terms of the Creative Commons Attribution License (CC BY). The use, distribution or reproduction in other forums is permitted, provided the original author(s) and the copyright owner(s) are credited and that the original publication in this journal is cited, in accordance with accepted academic practice. No use, distribution or reproduction is permitted which does not comply with these terms.
*Correspondence: Erin B. Ware, ZWJha3NoaXNAdW1pY2guZWR1